The benefits and harms of algorithms: a shared perspective from the four digital regulators
Updated 23 September 2022
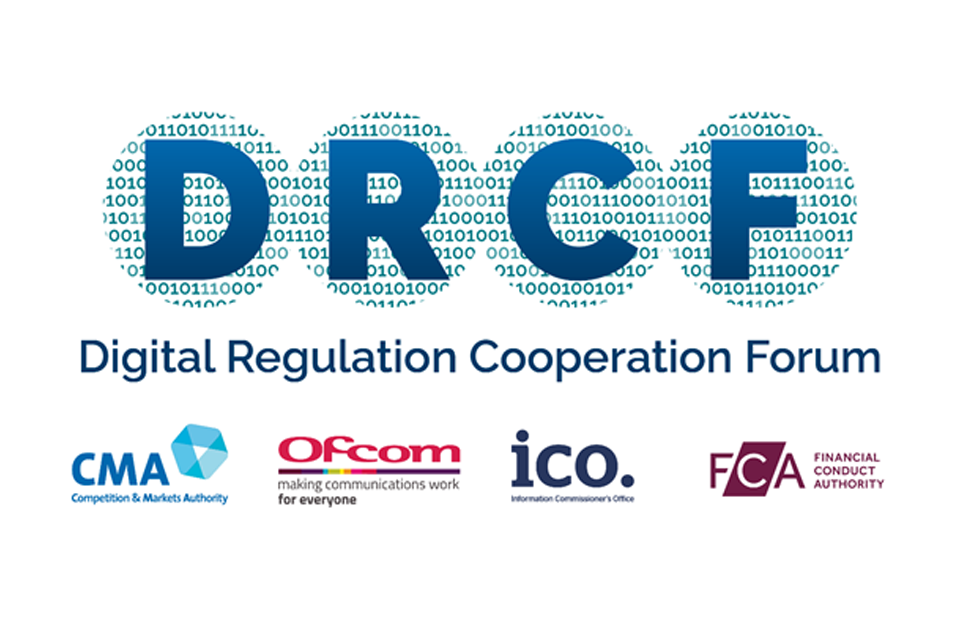
Note: This discussion paper is intended to foster debate and discussion among our stakeholders. It should not be taken as an indication of current or future policy by any of the member regulators of the Digital Regulation Cooperation Forum (DRCF).
Executive summary
Every day, we use a wide variety of automated systems that collect and process data. Such “algorithmic processing” is ubiquitous and often beneficial, underpinning many of the products and services we use in everyday life. From detecting fraudulent activity in financial services to connecting us with friends online or translating languages at the click of a button, these systems have become a core part of modern society.
However, algorithmic systems, particularly modern Machine Learning (ML) approaches, pose significant risks if deployed and managed without due care. They can amplify harmful biases that lead to discriminatory decisions or unfair outcomes that reinforce inequalities. They can be used to mislead consumers and distort competition. Further, the opaque and complex nature by which they collect and process large volumes of personal data can put people’s privacy rights in jeopardy.
It is important for regulators to understand and articulate the nature and severity of these risks. In doing so, they can help empower businesses to develop and deploy algorithmic processing systems in safe and responsible ways that are pro-innovation and pro-consumer. When it comes to addressing these risks, regulators have a variety of options available, such as producing instructive guidance, undertaking enforcement activity and, where necessary, issuing financial penalties for unlawful conduct and mandating new practices.
Over the past year, the Digital Regulation Co-operation Forum (DRCF) has enabled our 4 regulatory bodies (CMA, FCA, ICO and Ofcom) to collaborate in defining common areas of interest and concern. From this foundation, we can act more effectively in this space, identifying potential initiatives for individual regulators while recognising areas where joint initiatives and collaboration may have significantly more impact than individual interventions.
This paper is one of 2 initial publications by the DRCF on algorithmic processing[footnote 1]. In this paper we set out 6 common areas of focus among the DRCF members: transparency, fairness, access to information, resilience of infrastructure, individual autonomy and healthy competition. These areas were developed by DRCF members in conjunction with stakeholders from academia, civil society, government, industry, public sector and consumer groups. We then outline the current and potential harms and some of the current and future benefits of algorithmic processing that relate to our focus areas. Finally, we explore possible roles for UK regulators, the DRCF in particular, and outline suggestions for future work.
The key takeaways from this paper are:
- algorithms offer many benefits to individuals and society, and these benefits can increase with continued responsible innovation
- harms can occur both intentionally and inadvertently
- those procuring and/or using algorithms often know little about their origins and limitations
- there is a lack of visibility and transparency in algorithmic processing, which can undermine accountability
- a “human in the loop” is not a foolproof safeguard against harms
- there are limitations to DRCF members’ current understanding of the risks associated with algorithmic processing
As the DRCF continues to evolve, there are opportunities for members to co-ordinate and collaborate in a manner that would enable greater impact than individual regulatory action. These could include:
- Working with industry to improve companies’ understanding of the impact algorithms can have on individuals and society, including identifying and promoting best practice.
- Supporting the development of algorithmic assessment practices (as discussed in our companion paper[footnote 2] ), which can identify inadvertent harms, improve transparency, and provide confidence in the deployment of an algorithmic processing system
- Helping organisations communicate more information to consumers about where and how algorithmic systems are being used, for example via transparency guidelines and algorithmic registers.
- Engaging with researchers in the field of “human-computer interaction” (HCI) to explore ways to better understand issues with human-in-the-loop oversight, such as automation bias.
- Conducting or commissioning further research where appropriate, or drawing the attention of external researchers to important open questions. This could include exploring futures methodologies (for example horizon scanning and scenario planning) to identify emerging trends in the development and adoption of algorithms.
Through the process of researching and writing these papers, we have developed a better mutual understanding of members’ capabilities, remits and powers. This includes perceived areas of tension, such as those that between pro-privacy and pro-competition activities. We believe that through continued collaboration and co-ordination we can continue to resolve some of these tensions and have greater positive impact than acting solely as individual regulatory bodies.[footnote 3]
In the next financial year, we intend to undertake further activity in the field of algorithmic processing. We are now launching a call for input alongside the publication of these 2 papers to inform the future work of the DRCF, and we welcome and encourage all interested parties to engage with us in helping shape our agenda.
Introduction
What do we mean by ‘algorithmic processing’?
This discussion paper examines the benefits and harms posed by algorithmic processing. Here, we understand algorithmic processing as the processing of data (both personal and non-personal) by automated systems. This includes artificial intelligence (AI) applications, such as those powered by machine learning (ML) techniques, but also simpler statistical models. Our interest covers the processing of data, as well as the context in which that processing occurs, such as the means used to collect and store that data, and the ways humans interact with the results of any processing. We are also interested in both the positive and negative impacts on individuals and society that algorithms cause, as well as how different algorithmic systems interact with each other.
Algorithmic processing can be used both to produce an output (for example video or text content) and to make or inform decisions that have a direct bearing on individuals. It is already being woven into many digital products and services, resulting in efficiency gains across the public and private sectors. It can and does enable innovation and can unlock significant benefits for individuals, consumers, businesses, public services, and society at large. Examples of the benefits it provides include:
- detecting whether there has been fraud in someone’s bank account
- translating a foreign news site into English
- prioritising comedy films over sci-fi films through recommender systems on streaming services
- advertising travel insurance to someone after they’ve booked a holiday
- providing public safety messages, such as when someone can receive a coronavirus booster jab
However, algorithmic processing can also be a source of harm if not managed responsibly. It may, for example, produce biased outputs/predictions, leading some groups to be treated less favourably than others (for example algorithms used in CV screening software have the potential to unfairly discriminate against job applicants of one gender over another if not deployed or managed with due care).[footnote 4] Algorithmic processing could also lead to society-wide harms (for example by promoting disinformation through social media recommender systems). Algorithmic harms can emerge as an outcome of use, as in the case of the above examples, or through the development of these systems (for example on account of the energy costs of training an AI model [footnote 5]). These examples represent a small fraction of harms that can be caused.
Algorithmic processing can pose significant risks for several reasons. It can be used:
- To make automated decisions that can potentially vary the cost of, or even deny an individual’s access to, a product, service, opportunity or benefit. For example, a recruitment aptitude test may automatically reject a large volume of job applications for a given role.
- To process sensitive data on a large scale. For example, using live facial recognition at a stadium on matchday could impact rights relating to freedom of assembly.
- To track an individual’s behaviour online, which may infringe their right to privacy. For example, social media firms tracking what people look at online, without them being aware.
What is the purpose of this discussion paper?
The CMA, FCA, ICO and Ofcom collectively believe that we can, and should, play a role in identifying and mitigating these risks within the industries we regulate. In terms of algorithmic processing, for example, the data protection legislation the ICO oversees includes provisions that restrict the circumstances in which organisations can make solely automated decisions that have legal or significant effects on individuals. While the remits and powers of members vary [footnote 6], between us we have the ability to produce advice and guidance, set standards in the form of codes of practice, and commend responsible behavior. Our independence means we can provide robust oversight, scrutinising both the public and private sectors.
The DRCF is able to provide a coordinated regulatory approach to algorithmic processing. Collaboration is particularly important for addressing issues that cut across our regulatory remits, such as the use of personal data for real-time bidding in the advertising industry, and financial scams on social media.[footnote 7] The DRCF is the first forum in the world where 4 regulators, representing a range of perspectives, can pool insights and expertise on algorithmic processing, as well as conduct joint initiatives on topics of common interest. Working together will allow us to develop consistent messaging, and provide regulatory clarity for those using algorithmic systems.
This discussion paper provides an initial assessment of the benefits and harms that can arise from the use of algorithmic processing in the delivery of digital services. Our goal is to better understand how algorithmic processing takes place to help organisations achieve the benefits without causing the harms, laying the groundwork for future action in DRCF’s 2022 to 23 workplan. The paper covers the following topics:
- where and how algorithmic processing is being deployed in the sectors we regulate
- the benefits and harms associated with those applications
- the extent to which those harms are currently being mitigated
- the type of issues that may arise in the future as the use of algorithmic processing evolves
While we look here at the harms and benefits associated with all types of algorithmic processing, much of the research and stakeholder comments we cite relate specifically to the use of machine learning (ML) algorithms. This reflects the fact that ML-trained algorithms pose novel and sometimes more significant risks, which are only beginning to be understood. For example, they can surface, reward and amplify underlying harmful patterns that did not scale in the past. It is important, however, not to discount the impact of algorithmic systems built using conventional statistical methods, for example the Ofqual algorithm used to decide A-level grades for students in 2020.[footnote 8] In addition, this discussion paper will largely, although not exclusively, focus on the direct harms and benefits caused by the use and development of algorithms as they relate to our regulatory remits.
What domestic and international work has been conducted relating to algorithmic processing so far?
This discussion paper is being published at a time of growing interest – both domestically and internationally – in the effects of algorithmic processing, particularly that powered by AI and ML methods. In 2021 the UK government published a National AI Strategy [footnote 9], setting out its ambition to position the UK as an AI ‘superpower’. Among its commitments were to launch a National AI Research and Innovation Programme, an AI Standards Hub, and a new visa regime to attract AI talent to the UK. The government is expected to follow up with a separate AI White Paper later this year that sets out a national position for governing AI, as well as a National AI Strategy for Health and Social Care and a Defence AI Strategy. The government has also put forward a series of proposals to amend the UK data protection framework, including aspects that relate to AI.[footnote 10]
Other governments around the world have issued similar policy blueprints, including France, Germany, Canada, the US and China. So too have international and supranational bodies, among them the Ad Hoc Committee on AI at the Council of Europe (CAHAI), which is working on a legal framework for the development, design and application of AI. The European Commission, meanwhile, has proposed its own Artificial Intelligence Act, which – as presently conceived - would introduce new rules such as mandatory “conformity assessments” for high-risk applications of algorithmic processing.[footnote 11]
Regulators at home and overseas have also begun to examine the impact of algorithmic processing, with some issuing new directives to support the responsible use of algorithms in their sectors:
- In the US, the Federal Trade Commission (FTC) has issued guidance to businesses on the use of “AI and algorithms”,[footnote 12] and has conducted a public hearing on how algorithmic processing could impact competition and consumer protection.[footnote 13] Rebecca Kelly Slaughter – a Commissioner at the FTC – has also produced a report on algorithms and economic justice, which includes a taxonomy of harms.[footnote 14]
- In France, the data protection regulator, CNIL, has published research on the ethical implications of algorithmic processing, noting its impact on bias, exclusion, and free will, among other issues.[footnote 15]
- In Australia, the Australian Information Commissioner (AIC) has issued guidance for organisations on how to responsibly use modern data analytics techniques[footnote 16], while the Australian Competition and Consumer Commission (ACCC) has conducted an inquiry into the workings of digital platforms, including the use of algorithms to profile vulnerable users.
- In the UK, the CMA has published a paper on the potential effects of algorithms on competition and consumers.[footnote 17] This adds to the work already done by the ICO on explaining decisions made by AI [footnote 18] and Ofcom’s work on the use of AI in online content moderation.[footnote 19] The ICO has also produced guidance on AI and data protection,[footnote 20] an accompanying risk toolkit,[footnote 21] and its taxonomy of harms as part of its Regulatory Policy Methodology Framework.[footnote 22] The FCA is similarly active in this space[footnote 23]: FCA researchers have collaborated with the Alan Turing Institute to consider AI transparency needs [footnote 24] and have carried out research on algorithmic explainability and fairness.[footnote 25] The FCA has also carried out cross-firm reviews on themes relating to algorithmic trading highlighting examples of good and poor practice. [footnote 26] Separately, the FCA and Bank of England have led on an AI Public-Private Forum (AIPPF) to further the dialogue between the public sector, the private sector, and academia on AI.[footnote 27]
- In financial services, the international standards-setting organisation IOSCO has set out non-legally binding guidance relating to how regulators may best address conduct risks associated with the development, testing and deployment of artificial intelligence and machine learning. [footnote 28] The OECD recently consulted on revisions to the Principles on Financial Consumer Protection, with reference to the increasing use of artificial intelligence, machine learning and algorithms.[footnote 29]
The rest of this paper should be read with this wider context in mind. Indeed, there is much that DRCF members can learn from the research and experiences of other regulators and policymakers, particularly where they have successfully addressed the harms we outline in the following pages. However, this paper is unique in that it captures perspectives from 4 different digital regulators.
How was this paper produced?
The production of this discussion paper involved 3 stages.
Stage 1: Identify Shared Priorities
As digital regulation is a complex landscape that cuts across the remit of various regulators, it is important to provide clarity on the priorities that will guide the DRCF’s work in this area. To do this the DRCF convened a series of internal workshops which led us to identify the following high-level regulatory priorities:
- Protect individuals from harm.
- Uphold individual rights.
- Enable participation in online markets.
- Encourage consumer trust and innovation.
- Promote effective competition.
- Promote resilient infrastructure and systems.
Stage 2: Identify Shared Areas of Focus
In the second stage, DRCF members grouped a range of algorithmic harms and benefits into several shared areas of focus that are of mutual interest. The identification of these areas helped us to consider if and where a co-regulatory, collaborative approach may be effective in mitigating the potential harms arising from algorithmic processing.
It should be noted that we see accountability as an overarching concept that is a key motivator for DRCF members. It was not specifically identified as a shared focus area since it is fundamental to all the areas, particularly transparency.
The main areas of focus are:
- transparency of algorithmic processing
- fairness for individuals affected by algorithmic processing
- access to information, products, services and rights
- resilience of infrastructure and algorithmic systems
- individual autonomy for informed decision-making and participating in the economy
- healthy competition to foster innovation and better outcomes for consumers
The relationship between the working group priorities and shared areas of focus is shown in the chart on the following page. Each area is explained in detail in Current and Potential Harms of Algorithmic Processing
Stage 3: Stakeholder Engagement
In the third stage, DRCF members produced a summary report to share with stakeholders from academia, civil society, government, industry, public sector and consumer groups, as well as a list of questions for their consideration. Stakeholders provided feedback in a series of bilateral engagements with DRCF members. This discussion paper reflects the DRCF’s foundational thinking in the first 2 stages and the feedback we received from the list of stakeholders in the third stage. Stakeholders generally agreed that the working group priorities and 6 shared areas of focus were important. In addition, several other potential shared areas of focus were suggested which are discussed later in the paper.
Working Group Priorities and Shared areas of focus in algorithmic processing systems
Shared DRCF focus areas for algorithmic processing | Protect individuals from harm | Uphold individual rights | Enable participation in online markets | Encourage trust and innovation | Promote effective competition | Promote resilient infrastructure |
---|---|---|---|---|---|---|
Transparency of algorithmic processing | yes | yes | yes | yes | yes | no |
Fairness for individuals affected by algorithmic processing | yes | yes | yes | yes | no | no |
Access to information, products and services | yes | yes | yes | no | yes | no |
Resilience of infrastructure and algorithmic systems | no | no | no | no | no | yes |
Individual autonomy for informed decision-making and participating in the economy | yes | yes | yes | no | no | no |
Healthy competition to foster innovation and better outcomes for consumers | no | no | yes | yes | yes | no |
Current and potential harms of algorithmic processing
In this section we explain each of the shared areas and their importance. We then give examples of harms that can occur within each of these areas, however we acknowledge that some of these harms can affect more than one area.
Transparency of algorithmic processing
Transparency refers to the act of providing information about how and where algorithmic processing takes place. This information could relate to the technical features of the algorithm, including the data used to train it and the type of outputs it generates. Or the information could relate to the wider context in which the algorithm is deployed, such as the protocols and procedures that govern its use, whether it is overseen by a human operator, and whether there are any mechanisms through which people can seek redress. Transparency serves a number of purposes:
- it enables citizens and consumers to exercise their rights and make an informed judgement about if and how to engage with an algorithmic system
- it enables human operators of the algorithm to understand its strengths and limitations, and make better decisions about how to act on its outputs
- it helps buyers to scrutinise the claims made by vendors, which in turn supports competition in the market of algorithmic systems
- it helps regulators to monitor the use of algorithms in the industries that fall within their remits, allowing them to intervene before significant harm can occur
The areas in which algorithmic processing should be transparent, include:
- Purpose: being clear to the user about both the purpose and the nature of the system (for example whether it is entirely automated or includes input from a human)
- Knowledge: about how and where the system is used, including the data being processed
- Accountability: regarding the extent of human involvement, and where human accountability lies
- Justifiability: communicating where a decision is made, and the justification for that decision
- Impact: the likely impacts of the algorithmic processing for the individual It is important to note that transparency can sometimes result in unintended consequences, with algorithmic models being gamed or exploited if people know too much about the processes underlying their outputs.[footnote 30] Algorithmic transparency therefore needs to be viewed in context, with different levels of transparency provided to different individuals or organisations as appropriate.
Transparency: Where accountability lies
Algorithmic processing often involves multiple parties, each playing a different role in the journey from the creation of an algorithm through to its deployment. One party may collect data, another may label and clean it, and another still may use it to train an algorithm. There is concern that the number of players involved in algorithmic supply chains is leading to confusion over who is accountable for their proper development and use. A study looking at business-to-business AI services, for example, found that the roles of data “processor” and “controller” as expressed in data protection legislation are not always clearly identified, meaning those building, selling and using algorithms may not be fulfilling their obligations under the UK GDPR.[footnote 31] This confusion also means that citizens and consumers are left unsure of where they should turn for support in cases where they feel algorithmic processing is being misused. Stakeholders were particularly concerned about the potential for confusion where organisations purchase algorithms “off the shelf”, and stressed that developers and deployers must be clear on their responsibilities at the point of procurement. Vendors of algorithmic systems should also inform customers of the limitations and risks associated with their products.
Transparency: Exercising one’s rights and seeking redress
While there is often a lack of transparency regarding who is accountable for the outcomes of algorithmic processing, on occasion there is also a lack of transparency about the very use of those algorithms. So-called “invisible processing”[footnote 32] describes circumstances where personal data is obtained and processed without the direct participation or knowledge of individuals. Indeed, there are many reported cases of personal data being collected for one purpose but then being used for another. The ICO, for example, has taken enforcement action against a number of credit reference agencies, which were processing customer data for purposes that were beyond those originally agreed, including to build marketing products that help commercial firms predict people’s ability to afford different goods and services.[footnote 33] The ICO has also identified invisible processing in the advertising technology (adtech) ecosystem, where personal data (including behavioural data) has been used to build intricate profiles of internet users, often without their knowledge.[footnote 34]
While data subjects may technically provide consent for the reuse of their data, they may not always understand what this means in practice (hence it is not informed consent). As well as being potentially unfair, this lack of transparency makes it more difficult for individuals to exercise their rights in relation to the processing of their personal data. Under UK GDPR, this includes the right to rectify any errors in personal data, the right for personal data to be erased (also known as the right to be “forgotten”), the right to obtain and reuse personal data for their own purposes, and the right to object to the processing of data under certain circumstances. Without knowing that an algorithm is processing their personal data, individuals are also unable to seek redress for any harms that may have occurred as a result of that processing. They may not even be aware that they are being harmed. For example, those who face unlawful discrimination by a pricing algorithm may not realise they are paying a higher price than someone with similar circumstances in a different demographic group (for example a customer of one ethnicity paying more than a customer of another).
Even when individuals are aware that their personal data is being processed, and have made a decision to raise a complaint, they may not know where to turn to begin this process. While the ICO allows people to raise concerns about how an organisation is using their data, the stakeholders we spoke with suggested that public awareness of this option was low. Some stakeholders also believed that stronger measures of redress were required, such as introducing an easier route for people to seek financial compensation where their data protection rights have been infringed, in a manner akin to the small claims court system. Others we spoke with emphasised the need to lower the cost to civil society actors and private individuals of bringing legal action against those misusing algorithmic systems.
In general, it is important that regulators work together to simplify the process of raising complaints, enabling people to seek redress without having to navigate separate regulatory systems.
Transparency: Providing an explanation of a decision
In some cases, it is not enough simply to know that an algorithm is present and is processing data. It may also be important to understand how that algorithm has arrived at a particular decision or output (for example to know why someone has received a poor credit score, or why a photo posted on social media has been flagged as inappropriate). Indeed, the ability of individuals to have access to the ‘logic’ of a system is a requirement under UK data protection law for solely automated decisions that significantly affect them (with certain exceptions). However, the complexity and dynamic nature of some algorithmic systems - particularly those developed using machine learning techniques - can make it difficult to acquire an explanation. By definition, machine learning algorithms are not programmed by human hand but rather learn from data, which can result in models that are difficult to interrogate.
This in turn makes it harder for individuals and consumers to understand why an algorithm has made the recommendation or decision it has, and therefore what they should do differently in future to achieve a different result. It also makes it more difficult for those interpreting the results of an algorithm - for example a social media content moderator - to properly act on its outputs, which in turn undermines the quality of decision-making. This is especially the case where operators lack technical expertise. Two of the academic stakeholders we spoke with stressed the importance of “justifiability” in the context of an explanation - the idea that those on the receiving end of an algorithmic decision or output should understand the rationale behind that decision, as well as why it was appropriate to use an algorithm in that context.
Transparency: Algorithmic mis/disinformation
A lack of transparency regarding where and how algorithmic systems operate can also lead to harmful behaviour in a population. Members of the public may not know, for example, that what they are seeing and reading online is in fact produced by, or being recommended by, an algorithmic system, leading them to be less discerning about that content than they should be. One example is the use of algorithms by so-called troll farms to produce fake social media posts during elections - posts which are then shared between real users, facilitating the spread of disinformation. Another example is the use of algorithms to facilitate high-speed trading, which can result in “herding” behaviour where individual traders unknowingly mimic the actions of automated trading tools. This can in turn lead to erratic and unstable movements in financial markets.
Another issue falling under the banner of algorithmic transparency is the production of “synthetic media”. Synthetic media describes audio and visual content that either replicates the behaviours and characteristics of real people, or which alters how real people and environments are presented.[footnote 35] This type of content has long been used in the entertainment industry, including to enhance films in post-production, however there are growing concerns that it is now being used to deliberately mislead the public, who are unaware that the content is fabricated by an algorithmic system. A number of stakeholders flagged the risks posed by “deepfake” videos on social media, which falsely portray individuals as doing and saying things that are embarrassing, offensive, or in some other way inappropriate. As well as damaging personal reputations [footnote 36], synthetic media also risks undermining user trust in online content of all kinds, making it more difficult for the public to distinguish what is true from what is false.[footnote 37] This in turn could undermine democratic institutions, including news outlets and criminal and civil courts that rely on audio, visual and text-based media as evidence.[footnote 38]
Fairness for individuals affected by algorithmic processing
For algorithmic systems to win the trust of consumers and citizens, they need to be shown as operating fairly. To some, fairness means that people experience the same outcomes, while to others it means that people are treated in the same way, even if that results in different outcomes for different groups. What counts as “fair” in the context of algorithmic processing varies from context to context, and can even vary within a single industry.[footnote 39] However, fairness is not just a subjective ethical value, it is also a legal requirement. The UK GDPR for example mandates that organisations only process personal data fairly and in a transparent manner. Separately, the Equality Act prohibits organisations from discriminating against people on the basis of protected characteristics, including in cases where they are subject to algorithmic processing. The Consumer Rights Act, meanwhile, includes a “fairness test”, whereby a contract term will be unfair if “contrary to the requirement of good faith, it causes a significant imbalance in the parties’ rights and obligations to the detriment of the consumer”. This applies to contracts between traders and consumers, including those which involve algorithmic processing.
Fairness: Discriminating on the basis of sensitive characteristics
With a small number of exceptions, most observers agree it is unfair to discriminate against people on the basis of sensitive characteristics, such as their socio-economic status or accent.[footnote 40] Indeed, discrimination on the basis of protected characteristics (for example age, sexual orientation or race) is prohibited in specific contexts, such as employment or education, under the Equality Act.[footnote 41] It is therefore concerning that a number of algorithmic systems have been shown to produce biased or discriminatory results, from facial recognition technology that is better at recognising male and white faces,[footnote 42] to recruitment screening software that penalises job applications from female candidates.[footnote 43] Researchers have differentiated between 2 main categories of harm caused by biased algorithms: allocative and representational.[footnote 44] Allocative harms are those where particular groups are denied access to important goods and services. Representational harms occur when systems reinforce the subordination of groups through stereotyping, under-representation, and denigration.
Few of those who build and use algorithms deliberately set out to unfairly discriminate against people. However, there are many ways that bias can be inadvertently embedded within algorithms. One of these is by using training data that reflects historical bias. For example, if a predictive policing model is trained on the arrest data of police forces that have historically discriminated against black residents, that model is likely to reproduce those same biases in its patrol recommendations. These historical biases can also result in feedback loops, with biased models leading to biased outcomes, which are subsequently fed back into model training exercises. Other sources of bias include model optimisation, human interpretation, and even how a problem has been framed. [footnote 45] It is important to emphasise that those deploying algorithms do not need to intend to discriminate for their conduct to be unlawful.
Fairness: Dataset and Model Design Considerations
Those building and deploying algorithms often try to address bias by removing information about sensitive characteristics from their data (a technique known as “fairness through unawareness”). However, other information can act as a proxy for sensitive or protected characteristics, such as postcode acting as a proxy for ethnicity because of the correlation between those 2 variables. That means that depending on the context, simply removing sensitive or protected characteristics may not be the solution. These proxies as correlations are conceptually different to proxies intentionally used in model design when what you want to measure is not observable. For instance, in order to measure individuals’ risk of re-offending (unobserved quality), developers building recidivism models often use a score based on past arrests as a proxy since that has been recorded. The validity-reliability of these proxies for unobserved information in model design can affect the fairness of the outcome. A good example of how this can play out unexpectedly comes from the US healthcare system, where an algorithm used to refer patients to specialist healthcare programmes was recently found to systematically discriminate against black people.[footnote 46] The researchers investigating the algorithm found that healthcare costs accrued in a year were being used as a proxy for patient risk scores that would inform referral decisions. However, because healthcare costs were on average lower for black people than for white people with the same chronic conditions, black patients were less likely to be referred to specialist care than white patients, despite having the same support needs.
In some situations, however, there may be an operational need to use data points that happen to also correlate with sensitive attributes when building and running an algorithm. To take another example from the insurance industry, car engine size is known to be correlated with gender, yet it is also a material factor in determining the premiums of customers, given that larger engines are more costly to replace and that they result in more serious incidents.[footnote 47] Organisations may benefit from regulatory guidance to understand what counts as a legitimate use of proxy data, particularly in circumstances where they are under an obligation to treat their stakeholders fairly. This is the case, for instance, in the financial services industry, where the FCA has asked firms to demonstrate that “fair treatment of customers is at the heart of their business model”.[footnote 48]
Fairness: Price personalisation
In addition to these issues of demographic discrimination, several stakeholders highlighted how algorithmic processing could be used to discriminate against people on the basis of their purchasing power or willingness to pay. “Price personalisation” is not a new activity; many types of business have long attempted to set prices according to what individual customers are willing and able to pay, from cars to holidays, to household goods. However, algorithmic processing could amplify the ability of sellers to predict what people are willing to pay, drawing on data about those individuals which has not previously been available. In theory, this type of practice could be described as fair, since it may lead to lower income customers paying less and higher income customers paying more, possibly resulting in more people being able to access those goods and services. This practice may not be perceived as fair across the board, however. Additionally, others may view this practice as inherently unfair regardless of the outcome, as it would mean sellers are scrutinising the behaviour and characteristics of buyers without their knowledge.[footnote 49]
Another reason personalisation might be considered unfair is because it could result in people being penalised for circumstances outside of their control. People living in less affluent areas, for example, may be more likely to be the victim of a burglary, and therefore could face higher premiums for their home insurance - a pricing practice that one of our stakeholders described as a “poverty premium”.[footnote 50] This example also highlights the difficulty of defining fairness, as it could also be argued that the practice is fair with regards to the insurer in terms of increased premium for increased risk. Less affluent and more vulnerable consumers may also be unaware that some businesses engage in this type of pricing strategy, leaving them more exposed to its effects. Indeed, qualitative research undertaken by Ofcom in 2020 found that participants had very limited awareness and knowledge of personalised pricing, which is consistent with findings in the wider literature, that many consumers are surprised that their online behaviour might influence the prices they are offered for products and services.[footnote 51] The study also found that, with the exception of lower prices for low-income households, consumers were sceptical of the benefits of personalisation, with some saying that the practice was “disempowering”. By its nature personalised pricing is difficult to spot, and the extent and nature of this practice outside insurance and credit markets is not clearly understood.
Fairness: Redeploying algorithms in other contexts
Another practice that can sometimes result in unfair outcomes is the repurposing of algorithms. While some organisations are able to develop bespoke models that are attuned to their specific needs, others must rely on “off the shelf” systems purchased from third party vendors, which may have been trained in a very different context. These models can suffer from lower accuracy levels, and may harm individuals whose data is being analysed.[footnote 52] For example, an algorithm that has been developed to identify hate speech in one region of the world is likely to perform worse when deployed in another region, owing to differences in common parlance and cultural attitudes. The dangers of repurposing algorithms have also been well documented in the world of healthcare, where hospitals (notably in the US) have unsuccessfully sought to export their in-house diagnostic models to other settings.[footnote 53] Those procuring algorithms may be able to work with vendors to retrain systems to suit their own context, however this depends on the buyer having sufficient resources and bargaining power.
Access to digital markets, including information, products, services, and rights
From targeted job adverts to recommendation systems on social media sites, algorithmic processing is transforming how consumers and citizens access information, products and services online. By enabling a degree of personalisation, they are helping people to both seek out and be exposed to content and opportunities that are more relevant to their interests and appropriate for their needs. However, algorithms also pose several risks in this context, potentially closing people off from alternative viewpoints, as well as depriving some groups from seeing valuable economic opportunities.
Access: Limiting people’s exposure to alternative viewpoints
The use of algorithms to target content online, particularly on social media platforms, could result in internet users being repeatedly exposed to the same type of information. As has been well documented, many of today’s platforms deploy sophisticated recommendation algorithms, which adapt as they learn more about the type of content users tend to engage with. In many cases, this results in users being presented with more of the same innocuous content, such as a favourite TV show or a friend’s social media posts. However, in other cases this type of targeting can result in people being repeatedly shown content that is misleading or damaging, such as antivaxx conspiracy theories, or even violent content. Toxic online environments, polarisation or online aggressive behaviour may result from exposing internet users to this kind of emotionally charged content, potentially leading to physical harm in the real world.[footnote 54] This phenomenon can affect both individuals (for example if a person acts on misleading health information) and society (for example with so-called online echo chambers fostering political and cultural polarisation).
Access: Limiting people’s exposure to economic opportunities
As well as changing the type of content people see online, algorithms can also shape the economic opportunities that internet users are exposed to. Many businesses today use targeted adverts to channel their products and services at desired audiences, saving them time and money, and benefiting consumers who want to see those goods. However, not everyone who has an interest in seeing those adverts is shown them. Researchers from Northeastern University, for example, ran an experiment on Facebook in 2019 which suggested that some online adverts for housing opportunities were being shown to black and white users at differing levels of frequency.[footnote 55] A separate study, also looking at Facebook, found that online job adverts for STEM careers were less frequently displayed to women.[footnote 56] The researchers hypothesised that this was partly because the underlying algorithms were designed to optimise for cost, and women tend to be more costly to advertise to (in part because they are seen as more likely to make a purchase). This is linked to how algorithmic processing can lead to unfair outcomes for some demographic groups, as was explained in [Fairness for individuals affected by algorithmic processing](https://www.gov.uk/government/publications/findings-from-the-drcf-algorithmic-processing-workstream-spring-2022/the-benefits-and-harms-of-algorithms-a-shared-perspective-from-the-four-digital-regulators#Fairness-for-individuals-affected-by-algorithmic-processing]
Resilience of infrastructure and algorithmic systems
Resilience refers to the capability of algorithmic systems to withstand shocks and perform consistently when exposed to different conditions. This includes being able to cope with adversarial attacks, such as when bad actors seek to “poison” datasets or extract personal information from an organisation’s training datasets. Algorithms can themselves be weaponised in order to inflict damage, for example by automating spear phishing [footnote 57] activity and scaling up denial of service (DoS) operations. These are issues of concern to all DRCF members.
Resilience: Bad actors targeting algorithms
As algorithmic processing has become more important to the functioning of public services and industry, so too has it become an increasingly attractive target for those eager to cause disruption. There are many ways that algorithmic systems can be undermined. One of these is by poisoning training data, resulting in models with lower levels of accuracy. Cyber criminals could, for example, seek to corrupt the training data used to build a bank’s fraud detection model, making it less likely that fraudulent activity is noticed. Another way criminals can wrongfoot algorithmic systems is by deploying “adversarial examples”.[footnote 58] This is where inputs to a model are deliberately manipulated in order to be misclassified or unrecognised, even if that manipulation is imperceptible to the human eye. Terrorist organisations, for instance, could try to evade the content moderation algorithms of social media platforms by making minute changes to the pixel patterns of their images and videos.
While these are cases of algorithms being manipulated in order to cause mistakes, cybersecurity experts have also highlighted how algorithms can be manipulated in order to leak sensitive information.[footnote 59] One practice of particular concern is “model inversion”, where personal information can be inferred about individuals who are featured in training datasets. A report from the US-based Center for Security and Emerging Technology highlights the example of a facial recognition system, where a model’s attackers start with a randomly generated image of a face, and make repeated edits to that image until they arrive at a version that the model matches to the name of their target individual.[footnote 60]
Stakeholders also raised more general concerns about the ability of organisations to safely manage the data they collect to train and run algorithmic systems. Despite the secure processing of personal data being a key principle under the UK GDPR [footnote 61], a DCMS Cyber Security Breaches Survey produced in 2021 found that 4 in 10 businesses experienced a “cyber security breach or attack” in the last 12 months.[footnote 62] The survey also suggested that businesses found it harder to implement cyber security measures during the pandemic, with fewer businesses now deploying security monitoring tools or undertaking any form of user monitoring than was the case a year ago.
Resilience: Bad actors using algorithms
Just as bad actors can seek to undermine algorithmic systems, so too can they weaponise them for their own purposes. A number of cyber security experts have documented how machine learning algorithms can be used to scale up criminal activity online.[footnote 63] This includes by automating and improving the quality of spear phishing attacks, which are personalised messages designed to extract information or money from their victims. In a recent experiment in Singapore, researchers from the Government Technology Agency used a deep learning natural language model in conjunction with other AI-as-a-service tools to craft bespoke phishing emails tailored to people’s backgrounds and personality traits.[footnote 64] Sending these emails to colleagues at the Government Technology Agency as an experiment, the researchers say they were impressed by the quality of the synthetic messages and the rate of click-throughs they were able to generate, when compared to messages that were drafted by humans.
As well as scaling up existing threats, algorithms could be used to introduce new ones. In a first of its kind incident, it was reported in 2019 that fraudsters used deepfake technology to mimic the voice of a senior executive from a German energy company, allowing them to request a transfer of several hundred thousand pounds from its UK subsidiary.[footnote 65] A report produced by a consortium of organisations including the Universities of Oxford and Cambridge predicts that novel cyber threats such as these will continue to emerge over the coming years.[footnote 66] This includes the use of algorithms to predict which individuals are most likely to respond to scams, thereby improving target selection.[footnote 67] The report also argues that the advent of machine learning tools is lowering the barriers to entry for cyber criminals, for instance by enabling attackers to perform phishing attacks in multiple languages with little additional effort required. These developments raise questions about the future resilience of traditional cyber security tools used by organisations.
Individual autonomy
Individual autonomy is about citizens and consumers having control over their lives, which includes being able to make informed choices about what they buy, the media they consume, and the people they interact with online. As we have already seen, algorithmic processing is allowing firms to target information, products and services with increasing precision, as well as to build more sophisticated “choice architectures” that determine how options are presented to users online. These practices offer tremendous benefits, yet when deployed inappropriately they can also undermine people’s autonomy, encouraging them to do things, buy things and believe things that are damaging to themselves and/or wider society. They can also impact on people’s freedom to determine their identity, including how they choose to present themselves to the world. [footnote 68] Vulnerable people are especially exposed to these risks.
Individual autonomy: Manipulation through unrestrained targeting
Targeting (for example via the use of recommender systems) is essential in helping people to navigate the large volume of content online; without it, it would be impossible for search engines to function or for music and video streaming sites to serve up the content we want to see and hear. Yet targeting can sometimes err into manipulation, resulting in people making decisions and altering their beliefs in a way they would otherwise not, given time, space and more options at their disposal. The Centre for Data Ethics and Innovation’s Online Targeting Review argued that targeting, when left unchecked, could exploit people’s impulses and emotions. The CDEI also expressed concern that targeting could be a driver of “internet addiction”, with recommender systems being designed to maximise endless engagement and clicks. Several stakeholders suggested these risks were greater when people had a “false sense of control” over their online interactions, since those who are unaware that targeting is taking place are also less likely to scrutinise what they are seeing and why.
Stakeholders also expressed concern that algorithmic targeting may be making consumer preferences more ‘sticky’. At any one point in time, consumers will have an affinity for a particular set of brands, products and services, which would typically be expected to change over time as societal tastes evolve, new goods arrive on the market, and brands launch new advertising campaigns. However, algorithmic recommendations (for example those provided via search results or targeted adverts) may serve to limit people’s exposure to alternative goods, potentially hardening their preferences. The extent to which people are aware of this practice, consent to it and can escape it in order to access wider options, will determine how much it impacts on individual autonomy.
Some groups in society are particularly vulnerable to the effects of targeting. This includes children, older people, people with learning disabilities, and people with addictions. An investigation by the Gambling Commission, for instance, found that 45% of online gamblers were encouraged to spend money on gambling activity due to the adverts they saw.[footnote 69] While there is no formula for determining what types of targeting are harmful, it is clear that user manipulation is a present risk online and one regulators will need to pay close attention to. Given the technical sophistication of some algorithms and the fact they may be deployed behind the scenes in ways that individuals affected may not appreciate, conceptions of who is ’vulnerable’ in this context may be broader than when thinking about vulnerability in other regulatory dimensions.
Individual autonomy: Manipulation through harmful choice architectures
A broader grouping of online practices that can undermine the autonomy of citizens and consumers is the use of harmful “choice architectures”.[footnote 70] User experience and interaction designers, content designers and marketers can be thought of as choice architects, and the design of the environment they create is the choice architecture.[footnote 71] Common examples of choice architecture include how products are ordered in search results, browsing buttons available to users on social media platforms, the number of steps needed to cancel a subscription, or whether an application is selected by default for tasks on mobile devices.
Choice architecture is a neutral term. A well-designed website, app or digital service built with users’ interests in mind will help consumers choose between suitable products, make transactions faster, and result in suggestions for more relevant products and services. Websites and platforms often spend significant time and resources refining their choice architectures, resulting in a better user experience and reduced friction for users. However, a CMA study identified that firms can design their user interfaces utilising algorithms in a manner that goes against users’ interests by exploiting their innate biases, such as loss aversion, inertia and their tendency to choose default options.[footnote 72] Some websites, for example, present consumers with potentially misleading scarcity messages, which aim to convince them that there is only so much time to buy a particular product, or that there is more limited stock than there is in reality. [footnote 73] Furthermore, both search algorithms and personalisation underpinned by algorithms can drive the choice architecture encountered by users. [footnote 74]
The CMA has also highlighted the practice of firms using algorithms to predict the likely rating that users would give to their service. Makers of apps, for example, have been shown to use algorithms to determine when users are more likely to leave positive reviews – a tactic that some fear is leading to ”ratings inflation”.[footnote 75]
Researchers have coined new phrases to describe particularly harmful forms of choice architecture. These include: dark patterns,[footnote 76] a set of (deliberate) manipulative practices identified by user experience (UX) designers; sludge [footnote 77], which makes it hard for consumers to take action in their interests; and dark nudges [footnote 78], which make it easy for consumers to take action that is not in their interests. Dark patterns have also been observed in the “consent management platforms” that are used by websites and apps to acquire consent from internet users to collect, share and sell their personal data. Some of these practices are unlikely to be compliant with data protection regulation.
Analysis of choice architecture is already central to some areas of regulatory intervention. For example, qualitative and quantitative analysis of the choice architecture of default applications are key parts of the CMA’s recent interim report on mobile eco-systems. [footnote 79] As we go on to discuss in the section below, choice architecture is also highly relevant to the control of personal data.
Individual autonomy: Control and protection of personal data
The complexity of algorithmic systems’ supply chains (including data collection and annotation) and how they operate across domains may lead to some loss of user control in how and where personal data is shared. In its Online Platforms and Digital Advertising Market Study, the CMA recommended that the new Digital Markets Unit (DMU) has the power to compel platforms to give consumers more control over their data. [footnote 80] Under this arrangement, the DMU would have the ability to introduce a “choice requirement”, which would require platforms to give consumers the choice to receive non-personalised advertising; [footnote 81] as well as to introduce a “fairness by design” duty, which would set out for platforms the choice architecture they should utilise to present effective choices to consumers. Furthermore, the CMA recommended the government consider giving the DMU powers to ask platforms to trial how such choices are presented to consumers given this is a complex area where unintended impacts are possible. The government is currently consulting on empowering the CMA to order trialing of potential remedies in the course of a Market Investigation Reference (MIR), [footnote 82] as well as giving similar powers to the Digital Markets Unit which could support its approach to implementing codes of conduct and pro-competitive interventions. [footnote 83]
Healthy competition to foster innovation and better outcomes for consumers
Strong competition helps to push down costs and prices, drive up service standards and quality, and increase access to products and services. It also creates incentives for innovation, productivity and economic growth. Effective competition means that markets are open to new firms that can offer better deals and products, while firms that cannot keep up either have to change or go out of business. Promoting competition is a priority statutory objective shared by the FCA, Ofcom and the CMA. The ICO is committed to supporting innovation and economic growth which is one aspect of competition.[footnote 84]
Healthy competition: Issues with anti-competitive behaviour in recommender systems and search engines
Recommender systems or ranking systems may be designed to promote a platform’s own products, content or services above those of its competitors. Self-preferencing can also occur where companies exploit default effects or saliency, such as where their own products and services are shown as the default option to consumers, rather than in a list of options. The CMA’s 2021 report on algorithms outlined this issue and the risks it poses to competition. [footnote 85]
Where own-brand content is recommended on video-on-demand services, there is a risk that the diversity of content is reduced for viewers, and public service material, for example, may become less prominent in search results. Algorithms that are used for information retrieval and ranking in search engines may be designed to up-rank certain sponsored links and own-brand content. Some users may be unaware of this preferencing and be unwittingly steered towards products or services that are more profitable to the company. A good example of where this practice has been shown to play out is the online hotel booking industry, where an investigation by the CMA between 2017 to 19 found that search results on some booking sites were affected by the amount of commission a hotel pays to the site. [footnote 86] Such practices may in turn impede competition, for example the ability of challengers to compete in concentrated markets such as search engines, as well as fairness concerns for consumers.
Healthy competition: The risk of connected algorithmic systems
Algorithms and the infrastructure around them are evolving and becoming increasingly complex, often with a multitude of interacting components, which could make it hard to explain or reverse engineer the output. Interconnectedness of algorithms developed by multiple organizations can also pose a risk. They could propagate and amplify issues within a system and make it challenging to isolate the root cause(s). For example, the “Flash Crash” [footnote 87] on 6 May 2010 has highlighted the risks of automated algorithmic trading. [footnote 88]
Some developers have also highlighted the challenges of integrating dynamic machine learning models with software that has been programmed using conventional methods. This is because the behaviour of the ML model will change as it is re-trained, which can cause issues with downstream applications.
Connected algorithmic processing systems could also facilitate collusion and lead to higher prices for consumers. A firm might develop a certain part of their product or service in-house and source other algorithmic components from third parties, for example to set prices, through which they could exchange information. There are concerns that algorithms could also lead to new forms of tacit collusion – where there is no explicit agreement between businesses to collude, but where pricing algorithms effectively deliver the same result.[footnote 89] At the extreme end, pricing algorithms drawing on ML technology could autonomously learn to collude. [footnote 90] They can be used to automatically detect and respond to price deviations by competitors, which could make explicit collusion between firms more stable, as there is less incentive for those involved to cheat or defect from the cartel. An example of this was the CMA’s Trod/GB eye decision in the online posters markets, where the parties agreed that they would not undercut each other’s prices for posters and frames sold on Amazon’s UK website. They implemented the agreement by using automated repricing software that they each configured to give effect to the illegal cartel. [footnote 91] A possible avenue to address concerns about autonomous learning by algorithms may be increased transparency from businesses, both around pricing behaviour and their rationale for using price matching algorithms.
Healthy competition: Data power [footnote 92]
Online platforms and search engines collect individuals’ data to train their algorithmic systems, allowing content to be personalised to user interests and needs. This personalisation of content can in turn drive more engagement on those platforms and engines, resulting in the collection of even more personal data with which to further refine their algorithms. This dynamic leads to network effects, with these products or services gaining additional value as more people use them. While this is in one sense a consequence of an effective and attractive service offering, it can also result in barriers to new entrants, who often lack the necessary user data to train comparable algorithmic systems. A study undertaken by the CMA found that this was a particular barrier to new entrants in digital advertising, with Google and Facebook benefiting from rich data sources that are well beyond those available to smaller companies in this market. [footnote 93] For example, a dominant search engine can use its volume and variety of activity to develop a deeper understanding of consumer interests than a competitor with lower market share. This allows the engine to provide more effective search advertising services as well as opportunities for advertisers to target niche search terms.
Additionally, mass personal data collection also potentially violates the principle in UK data protection law that requires firms to minimise the amount of personal data they collect. AI development has exacerbated the issue because it creates a heightened demand for data, including personal data. Organisations with data power accumulate granular information on individuals across their online journey that they then use to personalise their offerings, which can exacerbate information asymmetry between consumers and service providers.
Additional focus areas suggested by stakeholders
In addition to the 6 shared areas discussed above, stakeholders suggested the following 3 topics for the DRCF to consider.
Human ‘in’ or ‘on’ the loop
Stakeholders drew attention to the important role played by human practitioners who operate and interpret the results of algorithmic systems. These practitioners - who range from social media content moderators to the employees of financial services firms - are often seen as providing an additional line of defence against the potential harms that might be caused by algorithms. Applying common sense and more contextual knowledge, they may be able to spot, for example, where a social media post has been mistakenly flagged as containing hate speech, or where a financial transaction has been wrongly interpreted as being fraudulent.
However, a growing number of commentators are cautioning against viewing human involvement as a foolproof safeguard. Specialists in human computer interaction (HCI) have highlighted the problem of “automation bias”, where practitioners uncritically accept the recommended decision of an algorithm, rather than meaningfully engage with that output. [footnote 94] This is a concern the ICO has also identified in its AI guidance, as data protection law prohibits solely automated decisions that significantly impact individuals without a meaningful human review. Practitioners could also become distracted while in command of a system, or be unable to interpret its technical outputs, for example the different types of statistical accuracy indicators that a facial recognition model might produce when it flags a positive match.
For these reasons, it is important that users of algorithmic systems implement a wider set of oversight and governance arrangements. This includes establishing effective decision-making procedures for approving new systems, as well as regularly reviewing the accuracy of those systems once they are live.
Impact of algorithmic processing on climate
There is intense ongoing debate about the potential impact of algorithmic systems including AI and machine learning on climate change. There are several ways in which AI can help with reducing climate change, however the computational resources required for developing and maintaining this technology can also have a negative impact. Research shows that AI may act as an enabler on 134 targets (79%) across all Sustainable Development Goals developed by United Nations (UN). [footnote 95] For example, machine learning could help optimize energy supply and demand in real time, with increased efficiency. AI can also help retailers reduce their environmental footprint through waste reduction, better optimization of their supply chain to improve how they respond to market demands. [footnote 96] At a consumer level, algorithms can play a positive role by helping users make sustainable choices. The potential of AI to combat climate change is an active topic of research that has been explored by various organisations. [footnote 97] Several agencies are calling upon governments to develop appropriate policies to tap into the full potential of these technologies. [footnote 98]
But despite AI’s promise, research suggests 35% [footnote 99] of targets across all Sustainable Development Goals may experience a negative impact from its development. [footnote 100] Algorithmic systems, especially advanced ML systems, require very high computational resources, particularly in their training and development phases. For example, research estimated that the carbon footprint of training a single big Natural Language Processing (NLP) model is equal to around 300,000 kg of carbon dioxide emission. [footnote 101]
Data governance
Stakeholders told us that regulators should pay close attention to the way organisations handle data, given that the quality of data is a major determinant in shaping how an algorithmic system performs. Incomplete or outdated training datasets, for example, are likely to result in poorly performing models. Unrepresentative datasets, meanwhile, could result in models that are less accurate when processing the data of particular demographic groups, whether that is for the purposes of screening CVs or targeting consumer adverts. A recent business survey undertaken by Ipsos MORI for the Centre for Data Ethics and Innovation revealed that 23% of UK firms using “AI and data-driven technology” saw challenges in accessing quality data.[footnote 102] Of these, 74% cited the problem of collating data from fragmented data sources, while 32% said there was a lack of historical data available on which to train their systems.
Even when organisations have access to high quality data, they may not be aware of how to store that data responsibly. Depending on the nature of the data, good data governance may mean storing data in a standardised format, creating metadata to ensure other users understand how it should be used, putting security controls around who has access to that data, and keeping a record of who is using that data and how. Some organisations have taken to creating “data catalogues” to monitor their data inventory and ensure its proper use. In the same CDEI-Ipsos MORI survey, 86% of firms who use AI and data-driven technology said they felt able to “store and manage data responsibly through well-defined governance and data protection protocols”.[footnote 103] While this is reassuring, the survey also identifies room for improvement in several areas. This includes the ability of firms to handle unstructured data (for example videos and images), with only 45% of respondents saying they do this well.
The UK government has documented and sought to address a number of these issues in its National Data Strategy, which highlights the recent creation of a government Data Quality Hub to promote best practice methods for maintaining data quality. [footnote 104] Effective algorithmic auditing may also be a way to help address these issues in some settings, with auditors looking not just at how algorithms perform but also how organisations are managing the datasets that underpin them. Auditing of this nature could potentially serve a related purpose of assuring that datasets have been developed responsibly, for example that the data they contain has been labelled by individuals who have been adequately compensated for their time.
Current and Potential Benefits of Algorithmic Processing
Algorithmic processing has the potential to bring about huge, positive impacts on people’s lives. Some examples include:
- Machine learning being used in hearing aid design to improve the clarity of speech in the presence of background noise. [footnote 105] Elsewhere in healthcare, machine learning has been used to create artificial voices for people with motor neurone disease. [footnote 106]
- Algorithms being used to summarise complex information for a person to easily understand such as in news media, financial research, search engine optimisations, or analysing legal documents. [footnote 107]
- AI being used by local authorities to analyse how active travel schemes are being used [footnote 108] or to detect new land for housing in response to increasing housing needs. [footnote 109]
In this section we provide a discussion about how algorithmic processing can provide benefits within our 6 shared focus areas, both now and in the near future.
Transparency of algorithmic processing
Algorithms are often discussed as being difficult (or impossible) to interpret or explain, especially when more complex machine learning such as neural networks or deep learning are used. However, algorithms themselves can sometimes be used to assist in creating valuable interpretations and explanations. There is growing interest in ‘counterfactual algorithms’ that can generate explanations based on what could have happened if the input of a model was different. [footnote 110] For example, an algorithm could inform an individual who had been rejected for a loan that if their income was higher, or their level of debt was lower, that their loan application would have been accepted. Understanding how to achieve a better decision can help foster greater trust.
Algorithms can also be used for dimension reduction in models, [footnote 111] removing excessive and irrelevant features from machine learning models and thus making them simpler and potentially more explainable and interpretable. This could lead to better human-computer interactions and making AI accessible to a broader audience. Future benefits could see algorithms assist with better decision-making. New developments in interpretable AI and visualisation of AI are making it easier for human experts to put complex data together to draw actionable insights. For example, in a medical research context, AI-assisted summarisation could one day help clinicians see the most important information and patterns about a patient leading to better treatment.[footnote 112]
Fairness for individuals affected by algorithmic systems
Algorithms can also be used to detect bias and discrimination. Some suggest that models and so-called ‘causal graphs’ (graphical representations of the causal relationship between features and outputs) can be used to detect and explain the causal pathways that lead to potential unfairness. [footnote 113] Research in this area is evolving and tools are being developed to assist in detecting unfair bias and discriminatory decision-making.
Access to digital markets
Algorithmic processing can assist in widening access to digital markets. For example, price personalisation can enable certain customers to access goods or services by lowering the price and thereby widening access. [footnote 114] Browser plug-ins enable users to control their browsing data and help them to understand how they are being tracked and what is informing the recommendations being made to them. [footnote 115] This may help empower users and increase user inclusion in online services.
In credit underwriting, there may be opportunities to improve the efficiency and inclusiveness of lending if some algorithmic systems can help assess the creditworthiness of customers with limited credit histories (‘thin-files’). [footnote 116] There is also an opportunity to empower consumers with unique insights into their financial needs, reducing matching frictions and supporting effective decision-making.
There may be ways to promote legal inclusion too, such as through automated advice - also known as robo-justice. For example, individuals can receive automated advice on whether they are eligible for legal aid. [footnote 117]
Resilience of infrastructure and users to cyber threats, scams and fraud
As well as creating and exacerbating security risks, algorithmic processing can be used to enhance resilience of infrastructure and users to cyber threats, scams and fraud. For example, algorithms are used for triaging, monitoring and blocking spam/fraudulent activity, which supports consumers, benefits business, and helps to avert data breaches. AI can be used to flag erroneous commercial transactions, and to train systems that detect synthetic media content designed to mimic real individuals. They can be deployed in the financial markets for Anti-Money Laundering (AML) purposes, fraud detection and countering the financing of terrorism purposes. They can also assist in anti-corruption efforts; for example, Microsoft announced its Anti-Corruption Technology and Solutions initiative in late 2020, which will leverage technologies to enhance transparency and to detect and deter corruption. Early applications of this initiative have helped to bring greater transparency to how the use of COVID-19 economic stimulus funds has been spent. [footnote 118]
Individual autonomy
Algorithms can be used to enhance the user experience and enable individuals to make better choices via specific design choices on social media platforms. This can be done at multiple stages of the user journey and allows users to consciously control how and when they share their personal data, or determine what they see (such as filtering results in recommender systems). Context-aware recommender systems [footnote 119] may be used to provide information and services that take into account the users’ needs and context.
A future trend could see greater personalisation of how individuals interact with algorithmic systems. For example, if a user is partially sighted, an algorithm could adjust the size or font of some text automatically to enable greater autonomy.
Healthy Competition
Algorithmic processing can foster competition by helping customers connect with a greater number of providers, as well as helping firms to access consumers, hence reducing the barrier to entry in some markets. Search engines, for example, are algorithmic systems that allow people to find hundreds if not thousands of products that match their search terms. Price comparison websites use similar techniques to collate information and present consumers with up-to-date prices on a range of goods and services, from flights to car insurance to broadband. Algorithmic processing has also helped to power the growth of the sharing economy, including ride-hailing and home rental services. P2P platforms have opened up more choice for consumers and increased pressure on traditional industries to improve their offerings (for example with Airbnb disrupting the traditional hotel industry).
There are strong indications that the increase in use of algorithmic systems will lead to economic growth and efficiency optimisation. It has been predicted that AI, for example, could deliver a 22% boost to the UK economy by 2030. [footnote 120] More economic growth, driven by algorithmic processing, could mean better incentives to invest in the sector experiencing growth. In turn, this leads to greater incentives for new organisations to enter the market, creating greater competition amongst firms. This could produce benefits for consumers as they will have more choices. Implementing algorithmic systems could also reduce the supply costs of goods and services, with savings passed on to customers in the form of lower prices.
Implications for regulators
Role for Regulators and the DRCF
The DRCF was established to build on the strong working relationships between its members and to enhance this cooperation and the effectiveness of individual regulatory approaches, given the unique challenges posed by the regulation of digital services and products. This discussion paper illustrates some of the benefits and harms that could arise from the current use of algorithmic processing, as well as how these issues might evolve in the near future. We have integrated the views of different agencies to help firms and other stakeholders understand common concerns. Our findings suggest many areas where there is shared interest and therefore opportunities for a greater level of cooperation.
We recognise the influential role we can play to shape the algorithmic processing landscape to benefit individuals, consumers, businesses, and society more broadly. The DRCF is pioneering in that it can address issues from 4 different perspectives. We can be inspired by the interventions that individual regulators have made to think of ways of collaborating in the future. Through guidance and thought leadership, we can provide greater clarity for organisations so they can confidently innovate. For example, the ICO and The Alan Turing Institute’s co-badged guidance on ‘Explaining Decisions Made with AI’ guides organisations in ways to make their use of AI systems more transparent. As DRCF members, we may consider ways to build and expand on this to provide further clarity to the organisations we regulate to ensure they are transparent about who is accountable and what the allocation of accountability within the AI pipeline entails. We can also explore ways of clarifying the similarities and differences over the concept of transparency across the different DRCF members.
A more hands-on cooperative intervention could be achieved through the increased use of regulatory sandboxes. The FCA’s regulatory sandbox allows firms to test products and services in a controlled environment, and to reduce the time-to-market at potentially lower cost. The ICO is an active mentor in the FCA Digital Sandbox, and also runs its own regulatory sandbox programme on a rolling basis. These sandboxes are not exclusively open to organisations developing algorithms, although many of the entrants do use them. We could explore ways of running sandboxes where 2 or more DRCF members can (subject to their particular powers) offer advice and the ability to test products and services that use algorithmic processing in a controlled environment.
As well as interventions that are targeted at organisations during the pre-deployment stages, regulators can exercise their powers to take enforcement action against actors who have not complied with the law and caused harm. Appropriate enforcement action can be a powerful tool to deter organisations from ignoring compliance issues. We can explore ways to collaborate in investigations where algorithmic processing is causing harms that span the mandate of more than one regulator. There may also be opportunities for valuable joint work on supporting individuals and consumers in seeking redress over harms they believe they have incurred.
The DRCF could also establish greater consistency in the way we engage with citizens about algorithms to enable them to better understand what algorithms are, where they’re used, and the choices available to consumers. This includes consistency about the language and terminology we use, as this can easily create or increase confusion. Cooperation can be wider than just between DRCF members, it can include other regulators as well as wider society. For example, engaging with the Equality and Human Rights Commission when we conduct further work on algorithmic processing and fairness. We can also engage with technology providers and professional users (for example media organisations, retail firms, and public services) to better understand how algorithmic processing takes place and how to achieve the benefits while minimising harms. Finally, not every issue that is identified in the context of algorithmic processing will require joint action from DRCF members, and regulatory approaches may well vary in important aspects, reflecting the specific regulatory context and mandate. Many potential benefits and harms related to algorithms are also context dependent and require a tailored approach from an individual regulator that is sensitive to the specifics of a particular sector.
Conclusions and Next Steps for the DRCF
Although the 4 regulators within the DRCF have different remits, there are overlapping areas of mutual interest. The DRCF have identified the following 6 cross-cutting focus areas in the context of algorithmic processing:
- Transparency of algorithmic processing
- Fairness for individuals affected by algorithmic processing
- Access to information, products, services, and rights 4.Resilience of infrastructure and algorithmic systems 5.Individual autonomy for informed decision-making and participating in the economy 6.Healthy competition to foster innovation and better outcomes for consumers
One aim of the DRCF is that future regulatory guidance and thought leadership in these areas is approached in a more joined up way. This approach is important for businesses - particularly in terms of guidance and standard setting. Algorithmic processing systems have the potential to deliver many benefits and harms as identified in this document. We will work together where appropriate to ensure that the harms are mitigated in a proportionate way, and help businesses to innovate so that they can realise the benefits.
There was a broad set of answers when stakeholders were asked to identify which area should be prioritised: transparency received the most support with fairness and resilience coming joint second. Some stakeholders also suggested that pursuing some priorities may require balance with others: for example, the pandemic has shown that there can be perceived tensions between protecting individuals from harm and protecting individual rights. There may also be perceived tensions between the aims of competition law and data protection, although these tensions can be resolved. We believe that the ICO and CMA’s joint statement provides a blueprint for how tensions or unintended effects across different types of digital regulation can be negotiated between regulators and allow synergies to emerge. [footnote 121] Where firms make “privacy preserving” claims in the context of defending their exclusive access to large volumes of data flows, regulators may test those claims as substantial access to data may be a source of market power.
Going forward there are a number of potential areas we could focus on, and, of these, transparency and fairness have been shown to be particularly significant. Similarly, actively supporting access to redress is important, as is recognising the role DRCF members can play in helping citizens/users better understand what algorithms are, where they’re used and the choices available to them. Many of the issues identified are exacerbated in situations where there are multiple parties involved in the development, deployment and use of systems, for example in AI-as-a-Service tools We have identified the following points as the key takeaways from our work.
Key Takeaways
1 . Algorithms offer many benefits for individuals and society and these benefits can increase with continued responsible innovation.
Companies that innovate responsibly can use algorithms to create benefits for individuals and society in a virtuous cycle. When consumers see evidence of and/or experience benefits they trust and support firms facilitating those benefits. This can create and stimulate markets and drive economic growth. Benefits may include increased productivity; the development of tools for disadvantaged groups; and improved methods of summarising, organising and finding information and content.
DRCF members could (where appropriate) work together to identify best practice in different areas of algorithmic design, testing and governance, and disseminate these lessons to help industry innovate responsibly for the benefit of all. There may also be opportunities to help businesses demonstrate compliance where they deploy algorithms, making sure this process is as simple and cost-effective as possible.
2 . Harms can occur both intentionally and inadvertently
As explained in this paper, algorithmic processing can be deliberately used to inflict damage, whether that is by automating spear phishing attacks or enabling the creation of subversive deepfake content. Yet much of the harm that results from the use of algorithmic processing may be inadvertent, perhaps caused not by malice but by insufficient understanding on the part of those who deploy these systems. Some users may not appreciate, for example, that harmful bias can be embedded within algorithms, nor that some algorithms may affect vulnerable users differently to the wider population.
Thus, it may not be appropriate for DRCF members to assume that organisations understand the risks of algorithmic processing, nor that they are aware of methods to mitigate those risks. DRCF members, as well as producing clear guidance and policies, could therefore look at ways of improving industry’s baseline knowledge of the impact algorithms can have on individuals and society.
3 . Those procuring and/or using algorithms often know little about their origins and limitations
Those purchasing algorithmic systems often do so with little knowledge of how they have been built and how they perform in different contexts. This makes it more difficult for purchasers to identify and mitigate risks (for example algorithmic bias), and to ascertain whether the systems they are using were developed responsibly (for example built with the support of data labelers who were adequately compensated for their work).
DRCF members could support the development of algorithmic auditing practices, and consider appropriate minimum standards in relation to some areas of algorithmic deployment. We could, for example, set standards for third party auditors, or investigate the merits of tools like bias tests. Algorithmic auditing is the subject of another DRCF paper [footnote 122] being published alongside this one.
4 . The lack of visibility in algorithmic processing can undermine accountability
Algorithmic processing may take place without the knowledge of those affected by it (for example someone rejected for a credit card may not realise their application was processed by an algorithm, just as those viewing videos on a streaming site may not realise that content has been personalised by an algorithm). In some cases this lack of transparency may make it more difficult for people to exercise their rights - including those under the GDPR. It may also mean algorithmic systems face insufficient scrutiny in some areas (for example from the public, the media and researchers).
DRCF members could help organisations communicate more information to consumers about where and how algorithms are being deployed. This could mean issuing new transparency guidelines, such as the ICO’s Explaining Decisions Made with AI guidance [footnote 123] or the government’s algorithmic transparency standard for the use of high impact algorithms by public bodies. We could also explore the costs and benefits of “algorithmic registers”, which serve as a public log that anyone can access.
5 . A “human in the loop” is not a foolproof safeguard against harms
Having a human review the outcomes of an algorithmic system has been suggested by some AI commentators to be an essential safeguard, and indeed data protection law includes specific protections for individuals from being subject to decisions made by solely automated means. Yet research suggests human operators often struggle to interpret the results of algorithmic processing, with some misunderstanding the different ways that accuracy can be measured. Some also place too much faith in the effectiveness of algorithmic processing, insufficiently scrutinising their outputs (for example that of a positive match provided by a content moderation tool used by a social media platform).
DRCF members could further investigate the concept a “human in the loop” and explore opportunities to help firms understand better the strengths and limitations of this and other approaches to risk mitigation. Appropriate human oversight and accountability will be essential to mitigate potential harms, whatever the technology deployed. DRCF members may find that further engagement with researchers in the field of “human-computer interaction” (HCI) is valuable in deepening collective understanding of potential issues related to human oversight and may wish to share emerging insights in this space with the industries they regulate.
6 . There are limitations to DRCF members’ current understanding of the risks associated with algorithmic processing
Recent years have seen a spate of innovations in algorithmic processing, from the arrival of powerful language models like GPT-3, to the proliferation of facial recognition technology in commercial and consumer apps. [footnote 124] As the number of use cases for algorithmic processing grows, so too will the number of questions concerning the impact of algorithmic processing on society. Already there are many gaps in our knowledge of this technology, with myths and misconceptions commonplace.
DRCF members could conduct or commission further research on algorithmic processing where appropriate, and otherwise draw the attention of external researchers to important open questions. There may be additional opportunities to liaise with organisations funding research, like UK Research and Innovation, to help inform their funding priorities. We may also consider using futures methodologies (for example horizon scanning and scenario planning) to identify emerging trends in the development and adoption of algorithms and work through the implications of these.
Call for input
Having presented our view on the most prominent risks and benefits associated with algorithmic processing, we are eager to hear views from a wide range of stakeholders on these matters. The DRCF is therefore launching a call for input on the findings of this report and our related paper on algorithmic auditing. We are particularly interested in hearing the views of stakeholders on the questions set out in Annex A. The call for input will last until Wednesday 8th June. Stakeholders can submit views via email at drcf.algorithms@cma.gov.uk.
Annex A – Call for input questions
We would welcome views from stakeholders on the following questions:
- What are your overall reflections on the findings of this paper?
- What other issues could the DRCF focus on?
- Which area of focus does the DRCF have the most potential to influence and which would you prefer the DRCF prioritised?
- What outputs would consumers and individuals find useful from the DRCF to assist them in navigating the algorithmic processing ecosystem in a way that serves their interests?
- Do you have any evidence on the harms and benefits of algorithmic systems you would like to share with the DRCF?
-
DRCF (2022) Auditing algorithms: the existing landscape, role of regulators and future outlook. ↩
-
Ibid. ↩
-
See for example ICO and CMA (2021) ‘CMA-ICO Joint Statement on Competition and Data Protection Law’. ↩
-
Reuters (2018), ‘Amazon scraps secret AI recruiting tool that showed bias against women’. 11 October. ↩
-
Taddeo, M., Tsamados, A., Cowls, J., and Floridi, L., (2021) ‘Artificial intelligence and the climate emergency: Opportunities, challenges, and recommendation’, One Earth, Vol 4, Issue 6, pp.776-779. 18 June. ↩
-
Such powers may include analysing systems at code-level; interrogating existing policies; interviewing stakeholders; issuing fines and taking other enforcement action where they find unlawful activity involving algorithmic processing. ↩
-
Real-time bidding is an automated digital auction process that allows advertisers to bid on ad space from publishers. ↩
-
BBC (2020) ‘A-levels: Ofqual’s ‘cheating’ algorithm under review’. 20 August. ↩
-
Office for AI, DCMS and BEIS (2021) ‘National AI Strategy’. 22 September. ↩
-
Department for Digital, Culture, Media and Sport (2021), ‘Data: a new direction’. 10 September. See also: ICO (2021), ‘ICO response to DCMS consultation “Data: a new direction”’. 7 October. ↩
-
European Commission (2021), ‘Laying down harmonised rules on artificial intelligence (Artificial Intelligence Act) and amending certain union legislative acts’. 21 April ; See the ICO’s response: ICO (2021), ‘Proposal for a regulation of the European Parliament and of the Council laying down harmonised rules on artificial intelligence (Artificial Intelligence act) and amending certain union legislative acts’. 6 August. ↩
-
Federal Trade Commission (2020), ‘Using Artificial Intelligence and Algorithms’. 8 April. ↩
-
Federal Trade Commission (2018) ‘FTC Hearing #7: The Competition and Consumer Protection Issues of Algorithms, Artificial Intelligence, and Predictive Analytics’ 13 to 14 November. ↩
-
Slaughter, R. K., Kopec, J., and Batal, M., (2021), ‘Algorithms and Economic Justice: A Taxonomy of Harms and a Path Forward for the Federal Trade Commission’, Yale Journal of Law and Technology, Special Publication. August. ↩
-
CNIL (2018), ‘Algorithms and artificial intelligence: CNIL’s report on the ethical issues’. 25 May. ↩
-
Office of the Australian Information Commissioner (2018), ‘Guide to data analytics and the Australian Privacy Principles’. 21 March. ↩
-
CMA (2021), ‘Algorithms: how they can reduce competition and harm consumers’. 19 January. See also CMA (2018) ‘Pricing algorithms: Economic working paper on the use of algorithms to facilitate collusion and personalised pricing‘. 8 October. ↩
-
ICO and The Alan Turing Institute (2020), ‘Explaining decisions made with AI’. No date. ↩
-
Ofcom (2019), ‘Use of AI in online content moderation’. 18 July. ↩
-
ICO (2020), ‘Guidance on AI and data protection’. No date. ↩
-
ICO (2021), ‘AI and data protection risk toolkit’. No date. ↩
-
ICO (2021), ‘Regulatory Policy Methodology Framework’. 5 May. ↩
-
The FCA has an outcomes-focused, technology neutral approach to regulation and sets clear expectations around accountability for FSMA authorised firms through the Senior Managers and Certification Regime. Accountability for the outcomes of algorithmic decisions remains with the Senior Manager accountable for the relevant business activity whatever technology is deployed. For instance, where firms use ‘robo advisory’ services, the Senior Manager accountable for advice would be accountable for the advice given and the end outcomes for consumers. Senior Managers should ensure that there are adequate systems and controls around the use of an algorithm. ↩
-
Mueller, H., and Ostmann, F., (2020), ‘AI transparency in financial services’. 18 February. ↩
-
Examples include Bracke, P., Croxson K., and Jung, C. (2019) ‘Explaining why the computer says no’, FCA Insight, and Bono, T., Croxson, K., and Giles, A (2021) `Algorithmic fairness in Credit Scoring’, Oxford Review of Economic Policy. ↩
-
FCA (2018) Algorithmic Trading Compliance in Wholesale Markets. ↩
-
Bank of England and FCA (2022) The AI Public-Private Forum: Final Report ↩
-
The Board of the International Organization of Securities Commissions (2020): The use of artificial intelligence and machine learning by market intermediaries and asset managers: Consultation Report ↩
-
OECD (2022) Public consultation on draft proposed revisions to the Recommendation on G20/OECD High-Level Principles on Financial Consumer Protection ↩
-
Tsamados, A., Aggarwal, N., Cowls, J., Morley, J., Roberts, H., Taddeo, M., and Floridi, L. (2022) ‘The ethics of algorithms: key problems and solutions’, AI & Soc. ↩
-
Cobbe, J. and Singh, J. (2021), ‘Artificial Intelligence as a Service: Legal Responsibilities, Liabilities, and Policy Challenges’. Forthcoming in Computer Law & Security Review. 9 June. ↩
-
ICO (2020), ‘ICO takes enforcement action against Experian after data broking investigation’. 27 October. ↩
-
ICO (2019) ‘Update report into adtech and real time bidding’. 20 June. ↩
-
The Royal Society (2022), ‘The online information environment: Understanding how the internet shapes people’s engagement with scientific information’. January. ↩
-
MIT Technology Review (2021), ‘A horrifying new AI app swaps women into porn videos with a click’. 13 September. ↩
-
The Royal Society (2022), ‘The online information environment: Understanding how the internet shapes people’s engagement with scientific information’. January. See also, Paris, B. and Donovan, J. (2019), ‘Deepfakes and cheap fakes: The manipulation of audio and visual evidence’. Data and Society. 18 September. ↩
-
Paris, B. and Donovan, J. (2019), ‘Deepfakes and cheap fakes: The manipulation of audio and visual evidence’. Data & Society. 18 September. ↩
-
Binns, R. (2018) ‘Fairness in Machine Learning: Lessons from Political Philosophy’. Proceedings of Machine Learning Research. See also CDEI (2020) ‘Review into bias in algorithmic decision-making’. 27 November. ↩
-
For example, while the law deems it fair for insurers to discriminate against people on the basis of their age (with older drivers often paying lower premiums than younger ones), it does not allow discrimination on the basis of gender or ethnicity ↩
-
UK Government (2010), ‘Equality Act 2010’. 1 October. ↩
-
Goode, L. (2018), ‘Facial recognition software is biased towards white men, researcher finds’. The Verge. 11 February. ↩
-
Reuters (2018), ‘Amazon scraps secret AI recruiting tool that showed bias against women’. 11 October. ↩
-
Kiat, L. S. (unknown), ‘Machines gone wrong’. No date. ↩
-
Bias from model optimisation occurs when models are designed to take into account features (e.g. price) which result in some groups being unfavourably treated. For example, MIT and London Business School researchers found in 2018 that online job adverts for STEM careers were less frequently displayed to women, in part because the underlying algorithms were designed to optimise for cost, and women tend to be more costly to advertise to. Bias from model generalisation occurs when organisations fail to use a single model to produce reliable results from multiple groups. In healthcare, for example, symptoms and biomedical markers for some diseases (for example diabetes) can vary by ethnic group, meaning that multiple models may be required to support diagnosis in the population. ↩
-
Nature (2019), ‘Millions of black people affected by racial bias in health-care algorithms’. 26 October. ↩
-
CDEI (2019), ‘Snapshot Paper – AI and Personal Insurance’. 12 September. ↩
-
FCA (2021), ‘Fair treatment of customers’. 24 March. See also: FCA (2021), ‘FCA to introduce new Consumer DUty to drive a fundamental shift in industry mindset’. 7 December. ↩
-
CMA (2021), ‘Algorithms: how they can reduce competition and harm consumers’. 19 January. ↩
-
See for example Pandey, A., and Caliskan, A. (2021) ‘Disparate Impact of Artificial Intelligence Bias in Ridehailing Economy’s Price Discrimination Algorithms’. Proceedings of the 2021 AAAI/ACM Conference on AI, Ethics, and Society. July. ↩
-
Ofcom (2020), ‘Personalised pricing for communications: Making data work for consumers’. 4 August. ↩
-
Danks, D., and London, A.J. (2017) ‘Algorithmic Bias in Autonomous Systems’. Proceedings of the 26th International Joint Conference on Artificial Intelligence (IJCAI 2017). ↩
-
Yu, A. C. and Eng, J. (2020), ‘One algorithm may not fit all: How selection bias affects machine learning performance’. RadioGraphics, 40 (7). 25 September. ↩
-
Hao, K. (2021) ‘How Facebook got addicted to spreading misinformation MIT Technology Review’. 11 March. ↩
-
M. Ali et al. (2019), ‘Discrimination through optimization: How Facebook’s ad delivery can lead to skewed outcomes’. Proceedings of the ACM on Human-Computer Interaction, Volume 3, Issue CSCW, November 2019, Article No.: 199, pp 1. ↩
-
Lambrecht, A and Tucker, C E (2019) ‘Algorithmic Bias? An Empirical Study of Apparent Gender-Based Discrimination in the Display of STEM Career Ads’. Management Science, 65 (7). pp. 2966-2981. ↩
-
Spear phishing is an email or electronic communications scam targeted towards a specific individual, organisation or business. Although often intended to steal data for malicious purposes, cybercriminals may also intend to install malware on a targeted user’s computer. ↩
-
Belfer Center for Science and International Affairs (2019), ‘Attacking Artificial Intelligence: AI’s Security Vulnerability and What Policymakers Can Do About It’. August. ↩
-
ICO (2019), ‘Privacy attacks on AI models’. 12 August. ↩
-
CSET(2020), ‘Hacking AI - A Primer for Policymakers on Machine Learning Cybersecurity’. December. ↩
-
ICO, ‘Guide to the UK General Data Protection Regulation (UK GDPR) – Security’ ↩
-
DCMS (2021), ‘Cyber Security Breaches Survey 2021’. 24 March. ↩
-
CSER (2018), ‘The Malicious Use of Artificial Intelligence: Forecasting, Prevention, and Mitigation’. 21 February. ↩
-
WIRED (2021), ‘AI Wrote Better Phishing Emails Than Humans in a Recent Test’. 7 August. ↩
-
WSJ (2019), ‘Fraudsters Used AI to Mimic CEO’s Voice in Unusual Cybercrime Case’. 30 August. ↩
-
CSER (2018), ‘The Malicious Use of Artificial Intelligence: Forecasting, Prevention, and Mitigation’. 21 February. ↩
-
These algorithms might equally in future be used by anti-fraud agencies to identify those most likely to be targeted by fraudsters, allowing to provide advance warning to these individuals that they are at risk. ↩
-
ICO (2021), ‘Data protection and privacy expectations for online advertising proposals’. 25 November. ↩
-
CDEI (2020), ‘Online targeting: Final report and recommendations’. 4 February. ↩
-
CMA (2022) ‘Online Choice Architecture: How digital design can harm competition and consumers’. 5th April. ↩
-
Thaler, R. H., Sunstein, C. R., & Balz, J. P. (2013), ‘Choice architecture. The behavioral foundations of public policy’, Princeton University Press. (pp. 428-439); Johnson, E. (2022). The Elements of Choice: Why the Way We Decide Matters. Oneworld Publications. ↩
-
Competition and Markets Authority (2021), ‘Algorithms: How they can Reduce Competition and Harm Consumers.’ 19 January. ↩
-
For example, the CMA discussed online hotel booking websites which used a combination of partitioned pricing, reference pricing, paid for ranking and scarcity claims to influence customer decision-making. Fung, S. S., Haydock, J., Moore, A., Rutt, J., Ryan, R., Walker, M., & Windle, I. (2019). Recent Developments at the CMA: 2018–2019. Review of Industrial Organization, 55(4), 579-605. ↩
-
CMA (2021), ’ Algorithms: How they can reduce competition and harm consumers ’. 19 January.) ↩
-
FT (2020), ‘Apple: how app developers manipulate your mood to boost ranking’. 7 September. ↩
-
The term “dark patterns” was coined by Harry Brignull: for examples of dark patterns, see What are Dark Patterns?. ↩
-
Sunstein, C. R. (2020), ’Sludge audits’. Behavioural Public Policy, 1–20. ↩
-
Campione, Chiara (A.A. 2018/2019), ‘The dark nudge era: Cambridge analytica, digital manipulation in politics, and the fragmentation of society’. Tesi di Laurea in Nudging: behavioral insights for regulation and public policy, Luiss Guido Carli, , Luiss Guido Carli, relatore Giacomo Sillari, pp. 55. [Bachelor’s Degree Thesis] Giacomo Sillari, pp. 55. [Bachelor’s Degree Thesis] ↩
-
Competition and Markets Authority (2021), ‘Mobile ecosystems market study’. 15 June. ↩
-
Competition and Markets Authority (2019), ‘Online platforms and digital advertising market study’. 3 July. ↩
-
Such consumer choice has now been implemented in China. See Vernotti, C. (2022), ‘Digital policy experts weigh in on China’s new algorithm regulation’. Technode. 5 April. ↩
-
BEIS (2021), ‘Reforming Competition and Consumer Policy’. 1 October. ↩
-
DCMS (2021), ‘A new pro-competition regime for digital markets.’ 20 July. Separately, the ICO’s Age Appropriate Design Code requires that ”information society services” set the highest privacy settings as default for child users. See: ICO (2019), ‘Age-appropriate design: a code of practice for online services’. ↩
-
The ICO has a statutory duty under the Deregulation Act 2015 to take into account the desirability of promoting economic growth. ↩
-
CMA (2021) ‘Algorithms: How they can reduce competition and harm consumers’. 19 January. ↩
-
CMA (2019). ‘Online hotel booking ‘. 13 September. ↩
-
The flash crash was a United States trillion-dollar stock market crash, which lasted for approximately 360 minutes. ↩
-
Buchanan, Bonnie. (2019), ‘Artificial intelligence in finance’.2 April. ↩
-
CMA (2021) ’Algorithms: How they can reduce competition and harm consumers’. 18 June. ↩
-
HM Treasury (2019), ‘Unlocking digital competition, Report of the Digital Competition Expert Panel’. 13 March. ↩
-
CMA (2016), ‘Online seller admits breaking competition law’. 21 July. ↩
-
Lynskey, Orla (2019), ‘Grappling with “data power”: normative nudges from data protection and privacy’. Theoretical Inquiries in Law, 20 (1). 189 - 220. ↩
-
CMA (2019), ‘Online platforms and digital advertising market study’. 3 July. ↩
-
Strauß, Stefan. 2021. “Deep Automation Bias: How to Tackle a Wicked Problem of AI?” Big Data and Cognitive Computing 5, no. 2: 18. ↩
-
Vinuesa, R., Azizpour, H., Leite, I. et al. (2020), ‘The role of artificial intelligence in achieving the Sustainable Development Goals’. Nature Communications 11, Article number 233. ↩
-
Australian Retail Association(2020), ‘How AI and ML can enhance sustainability for fresh retailers‘. 13 January. ↩
-
The Royal Society (2020), ‘Digital technology and the planet Harnessing computing to achieve net zero’. December. ↩
-
GPAI (2021), ‘Climate change and AI: Recommendations for government action’. November. ↩
-
The positive and negative impacts do not sum to 100% as AI could have both a positive and a negative impact on some of the targets depending on the scenario. ↩
-
Vinuesa R., Azizpour H., Leite I. et al.(2020), ‘The role of artificial intelligence in achieving the Sustainable Development Goals’. Nature Communications, 11, 233. ↩
-
Emma S., Ananya G., Andrew M. (2019), ‘Energy and Policy Considerations for Deep Learning in NLP’. 57th Annual Meeting of the Association for Computational Linguistics (ACL). ↩
-
CDEI (2021), ‘AI Barometer 2021’. 17 December. ↩
-
CDEI (2021), ‘AI Barometer 2021’. 17 December. ↩
-
DCMS (2019), ‘National Data Strategy’.8 July. ↩
-
Graetzer, SN , Barker, J, Cox, TJ , Akeroyd, M, Culling, JF, Naylor, G, Porter, E and Viveros Munoz, R (2021), ‘Clarity-2021 challenges : machine learning challenges for advancing hearing aid processing’. Interspeech 2021, 30th August - 3rd September. ↩
-
CDEI (2019), ‘Snapshot Paper - Deepfakes and Audiovisual Disinformation’. 12 September. ↩
-
Michael L. Littman, Ifeoma Ajunwa, Guy Berger, Craig Boutilier, Morgan Currie, Finale Doshi-Velez, Gillian Hadfield, Michael C. Horowitz, Charles Isbell, Hiroaki Kitano, Karen Levy, Terah Lyons, Melanie Mitchell, Julie Shah, Steven Sloman, Shannon Vallor, and Toby Walsh (2021). “Gathering Strength, Gathering Storms: The One Hundred Year Study on Artificial Intelligence (AI100) 2021 Study Panel Report.” Stanford University, Stanford, CA, September. ↩
-
SmartCitiesWorld (2021), ‘Manchester uses artificial intelligence to gain more insight into active travel’. 13 August. ↩
-
PLANNING (2021), ‘Birmingham Council to use artificial intelligence to help it find more housing land’. 30 July. ↩
-
Verma, S., Dickerson, J., & Hines, K. (2020). ’Counterfactual explanations for machine learning: A review‘. arXiv preprint. October. ↩
-
VentureBeat (2021), ‘Understanding dimensionality reduction in machine learning models’. 16 May. ↩
-
Michael L. Littman, Ifeoma Ajunwa, Guy Berger, Craig Boutilier, Morgan Currie, Finale Doshi-Velez, Gillian Hadfield, Michael C. Horowitz, Charles Isbell, Hiroaki Kitano, Karen Levy, Terah Lyons, Melanie Mitchell, Julie Shah, Steven Sloman, Shannon Vallor, and Toby Walsh (2021). “Gathering Strength, Gathering Storms: The One Hundred Year Study on Artificial Intelligence (AI100) 2021 Study Panel Report.” Stanford University, Stanford, CA, September. ↩
-
Nature (2020), ‘The long road to fairer algorithms’. 04 February. ↩
-
HM Treasury (2019), ‘Unlocking digital competition’. 13 March. ↩
-
Such as plug-ins that block ads and trackers like Pymk Inspector - Open Source Agenda and Ghostery. ↩
-
Ostmann, F., and Dorobantu C. (2021), ‘AI in financial services’. The Alan Turing Institute. ↩
-
Zeleznikow, J. (2017), ’Don’t fear robo-justice. Algorithms could help more people access legal advice’. The Conversation. 23 October. ↩
-
Microsoft (2020), ’Microsoft launches Anti-Corruption Technology and Solutions (ACTS)’. 9 December. ↩
-
Adomavicius, G., Mobasher, B., Ricci, F., & Tuzhilin, A. (2011). ’Context-Aware Recommender Systems‘. AI Magazine, 32(3), 67-80. ↩
-
McKinsey Global Institute (2019), ‘Artificial intelligence in the United Kingdom: Prospects and challenges’. 10 June. ↩
-
CMA&ICO (2021), ‘Competition and data protection in digital markets: a joint statement between the CMA and the ICO’. 19 May. ↩
-
DRCF (2022) ’Auditing algorithms: the existing landscape, role of regulators and future outlook‘. [INSERT HYPERLINK TO OTHER PAPER] ↩
-
ICO and The Alan Turing Institute (2020), ‘Explaining decisions made with AI’. No date. ↩
-
GPT-3 is a language model that performs a wide variety of natural language tasks, including autocompleting sentences ↩