A pro-innovation approach to AI regulation: government response
Updated 6 February 2024
Command Paper: CP 1019
ISBN: 978-1-5286-4565-2
Unique Reference: E03019481 02/24
Presented to Parliament by the Secretary of State for Science, Innovation and Technology by Command of His Majesty on 6 February 2024.
1. Ministerial foreword
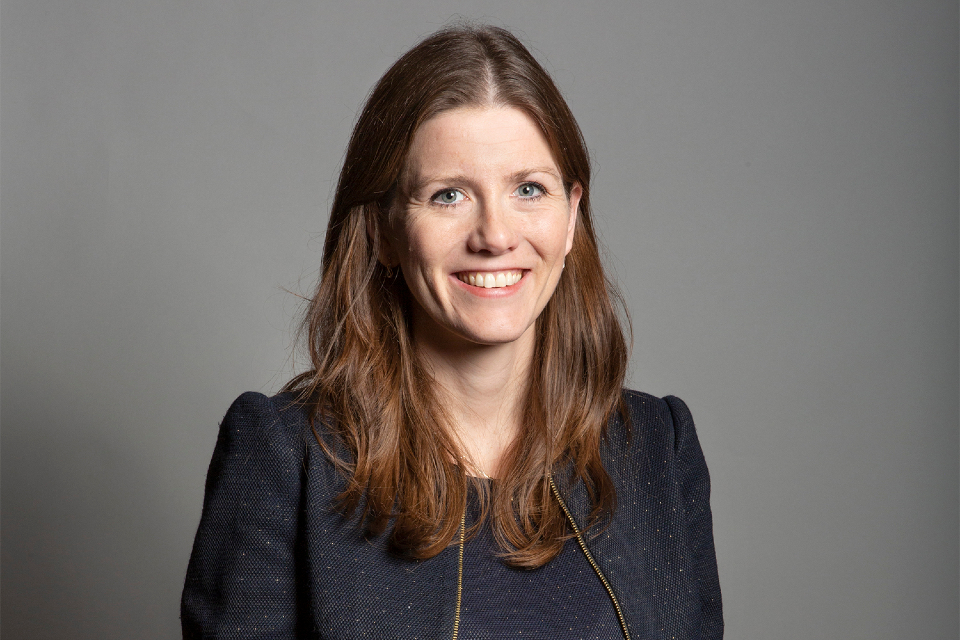
The Rt Hon Michelle Donelan MP, Secretary of State for Science, Innovation and Technology.
The world is on the cusp of an extraordinary new era driven by advances in Artificial Intelligence (AI). I see the rapid improvements in AI capabilities as a once-in-a-generation opportunity for the British people to revolutionise our public services for the better and to deliver real, tangible, long-term results for our country.
The UK AI market is predicted to grow to over $1 trillion (USD) by 2035[footnote 1] – unlocking everything from new skills and jobs to once unimaginable life saving treatments for cruel diseases like cancer and dementia. My ambition is for us to revolutionise the way we deliver public services by becoming a global leader in safe AI development and deployment.
We have done more than any government in history to make that a reality, and our plan is working. Last year, we hosted the world’s first AI Safety Summit, bringing industry, academia, and civil society together with 28 leading AI nations and the EU to agree the Bletchley Declaration – a landmark commitment to share responsibility on mitigating the risks of frontier AI, collaborate on safety and research, and to promote its potential as a force for good in this world.
We were the first government in the world to formally publish our assessment of the capabilities and risks presented by advanced AI. Research-driven reports produced by DSIT and the Government Office for Science[footnote 2] laid the groundwork for an international agreement on evaluating the scientific basis for AI safety.
We brought together a powerful consortium of experts in our AI Safety Institute, the first government-backed organisation of its kind anywhere in the world, committed to advancing AI safety in the public interest.
With the publication of our AI regulation white paper in March 2023, I wanted to take a bold and considered approach that is strongly pro-innovation and pro-safety. I knew that our approach had to remain agile enough to deal with the unprecedented speed of development, while also remaining robust enough in each sector to address the key concerns around potential societal harms, misuse risks, and autonomy risks that our thought leadership exercises have revealed.
This agile, sector-based approach has empowered regulators to create bespoke measures that are tailored to the various needs and risks posed by different sections of our economy. The white paper proposed five clear principles for existing UK regulators to follow, and set out our expectations for responsible AI innovation.
This common sense, pragmatic approach has been welcomed and endorsed both by the companies at the frontier of AI development and leading AI safety experts. Google DeepMind, Microsoft, OpenAI and Anthropic all supported the UK’s approach, as did Britain’s budding AI start-up scene, and many leading voices in academia and civil society.
In considering our response to the consultation, I have sought to double-down on this success and drive forward our plans to make Britain the safest and most innovative place to develop and deploy AI in the world, backed by over £100 million to support AI innovation and regulation. Building on feedback from the consultation, we have set up a central function to drive coherence in our regulatory approach across government, including by recruiting a new multidisciplinary team to conduct cross-sector risk assessment and monitoring to guard against existing and emerging risks in AI.
With the Digital Regulation Cooperation Forum (DRCF), we have launched the AI and Digital Hub, a pilot scheme for a brand-new advisory service to support innovation run by expert regulators including Ofcom, the CMA, the FCA and the ICO[footnote 3]. We are also investing in new support for regulators to build their practical, technical expertise and backing the launch of nine new research hubs across the UK to harness the power of AI in everything from mathematics to healthcare.
Advancing our thought-leadership on safety, we also lay out the case for a set of targeted, binding requirements on developers of highly capable general-purpose AI models in the future to ensure that powerful, sophisticated AI develops in a way which is safe. And our targeted consultations on our cross-economy AI risk register and monitoring and evaluation framework will engage with leading voices from regulators, academia, civil society, and industry.
The AI Safety Institute’s technical experts will have a crucial role to play here as we develop our approach on the regulation of highly capable general-purpose systems. We will work closely with AI developers, with academics and civil society members who can provide independent expert perspectives, and also with our international partners ahead of the next AI Safety Summits in the Republic of Korea and France.
Finally, my thinking on the UK’s AI leadership role goes well beyond the immediate horizon. We will need to lead fields of research that will help us build a more resilient society ready for a world where advanced AI technology and the means to develop it are widely accessible. That means improving our defensive capabilities against bad actors seeking to use AI to do harm, it means designing new internet infrastructure for a digital world full of agentic AI systems, and it also means leveraging AI to improve critical aspects of our society such as democratic deliberation and consensus. AI can and must remain a force for the public good, and we will ensure that is the case as we develop our policy approach in this area.
This response paper is another clear, decisive step forward for the UK’s ambitions to lead in safe AI and to be a Science and Technology Superpower by the end of the decade. Whether you are an AI developer, user, safety researcher or you represent civil society, we all have a shared interest in realising the opportunities of safe AI development. I am personally driven by a mission to improve the lives of the British people through technology and innovation, and our response paper sets out exactly how that mission will become a reality.
2. Executive summary
-
The pace of progress in Artificial Intelligence (AI) has been unlike any previous technology and the benefits are already being realised across the UK: AI is helping to make our jobs safer and more satisfying, conserve our wildlife and fight climate change, and make our public services more efficient. Not only do we need to plan for the capabilities and uses of the AI systems we have today, but we must also prepare for a near future where the most powerful systems are broadly accessible and significantly more capable[footnote 4].
-
The UK is leading the world in how to respond to this challenge. Our approach to preparing for such a future is firmly pro-innovation. To realise the immense benefits of these technologies, we must ensure AI’s trustworthiness and public adoption through a strong pro-safety approach. As the Prime Minister set out in a landmark speech in October 2023, “the future of AI is safe AI. And by making the UK a global leader in safe AI, we will attract even more of the new jobs and investment that will come from this new wave of technology”[footnote 5]. To achieve this, the UK is investing more in AI safety than any other country in the world. Today we are announcing over £100 million to help realise new AI innovations and support regulators’ technical capabilities.
-
Our regulatory framework builds on the existing strengths of both our thriving AI industry and expert regulatory ecosystem. We are focused on ensuring that regulators are prepared to face the new challenges and opportunities that AI can bring to their domains. By working closely with regulators to ensure cohesion across the landscape, we are ensuring that innovators can bring new products to market safely and quickly. Today we are announcing several new initiatives to make the UK an even better place to build and use AI including £10 million to jumpstart regulator’s AI capabilities; a new commitment by UK Research and Innovation (UKRI) that future investments in AI research will be leveraged to support regulator skills and expertise; and a £9 million partnership with the US on responsible AI as part of our International Science Partnerships Fund[footnote 6]. Through this and other work on AI across government, the UK will continue to respond to risks proportionately and effectively, striving to lead thinking on AI in the years to come. Through this and other work on AI across government, the UK will continue to respond to risks proportionately and effectively, striving to lead thinking on AI in the years to come.
-
In March 2023, we published our AI regulation white paper, setting out initial proposals to develop a pro-innovation regulatory framework for AI. The proposed framework outlined five cross-sectoral principles for the UK’s existing regulators to interpret and apply within their remits. We also proposed a new central function to bring coherence to the regime and address regulatory gaps. This flexible and adaptive regulatory approach has enabled us to act decisively and respond to technological progress.
-
Our context-based framework received strong support from stakeholders across society and we have acted quickly to implement it. We are pleased that a number of regulators are already taking action in line with our proposed approach, from the Competition and Market Authority’s (CMA) review of foundation models to the updated guidance on data protection and AI by the Information Commissioner’s Office (ICO). We are asking a number of regulators to publish an update outlining their strategic approach to AI by 30 April 2024.
-
We have already started developing the central function to support effective risk monitoring, regulator coordination, and knowledge exchange. Our new £10 million package to boost regulators’ AI capabilities, mentioned above, will help our regulators develop cutting-edge research and practical tools to build the foundations of their AI expertise and everyday ability to address AI risks in their domains. Today, we are also publishing new guidance to support regulators to implement the principles effectively and the Digital Regulation Cooperation Forum (DRCF) is sharing details on the eligibility criteria for the support to be offered by the AI and Digital Hub pilot.
-
We are backing this approach with wider support for the AI ecosystem, including committing over £1.5 billion in 2023 to build the next generation of supercomputers in the public sector and today announcing an £80 million boost in AI research through the launch of nine new research hubs across the UK to propel transformative innovations. In November 2023, the Prime Minister brought together leading global actors in AI for the first AI Safety Summit where they discussed and agreed actions to address emerging risks posed by the development and deployment of the most powerful AI systems. Leading AI developers set out the steps they are already taking to make models safe and committed to sharing the most powerful AI models with governments for testing so that we can ensure safety today and prepare for the risks of tomorrow.
-
Our initial technical contribution to this international effort is through the creation of an AI Safety Institute to lead evaluations and safety research in the UK government, in collaboration with partners across the world including in the US. The AI Safety Summit underscored the global nature of AI development and deployment, demonstrating the need for further work towards a coherent and collaborative approach to international governance.
-
Our overall approach – combining cross-sectoral principles and a context-specific framework, international leadership and collaboration, and voluntary measures on developers – is right today as it allows us to keep pace with rapid and uncertain advances in AI. However, the challenges posed by AI technologies will ultimately require legislative action in every country once understanding of risk has matured. In this document, we build on our pro-innovation framework and pro-safety actions by setting out our early thinking and the questions that we will need to consider for the next stage of our regulatory approach.
-
As AI systems advance in capability and societal impact, it is clear that some mandatory measures will ultimately be required across all jurisdictions to address potential AI-related harms, ensure public safety, and let us realise the transformative opportunities that the technology offers. However, acting before we properly understand the risks and appropriate mitigations would harm our ability to benefit from technological progress while leaving us unable to adapt quickly to emerging risks. We are going to take our time to get this right – we will legislate when we are confident that it is the right thing to do.
-
We have placed a particular emphasis on the challenges that highly capable general-purpose AI systems pose to a context-based framework. Here we lay out a pro-innovation case for further targeted binding requirements on the small number of organisations developing highly capable general-purpose AI systems to ensure that they are accountable for making these technologies sufficiently safe. This can be done while allowing our expert regulators to provide effective rules for the use of AI within their remits.
-
In the coming months, we will formally establish our activities to support regulator capabilities and coordination, including a new steering committee with government and regulator representatives to support coordination across the AI governance landscape. We will conduct targeted consultations on our cross-economy AI risk register and plan to assess the regulatory framework. We will continue our work to address the key issues of today, from electoral interference to discrimination to intellectual property law, and the most pressing risks of tomorrow, such as biosecurity and AI alignment. We will also continue to lead international conversations on AI governance across a range of fora and initiatives in the lead up to the next AI Safety Summits in the Republic of Korea and France.
3. Glossary
Adaptivity: The ability to see patterns and make decisions in ways not directly envisioned by human programmers.
Artificial General Intelligence (AGI): A theoretical form of advanced AI that would have capabilities that compare to or exceed humans across most economically valuable work[footnote 7]. A number of AI companies have publicly stated their aim to build AGI and believe it may be achievable within the next twenty years. Other experts believe we may not build AGI for many decades, if ever.
AI agents: Autonomous AI systems that perform multiple sequential steps – sometimes including actions like browsing the internet, sending emails, or sending instructions to physical equipment – to try and complete a high-level task or goal.
AI deployers: Any individual or organisation that supplies or uses an AI application to provide a product or service to an end user.
AI developers: Organisations or individuals who design, build, train, adapt, or combine AI models and applications.
AI end user: Any intended or actual individual or organisation that uses or consumes an AI-based product or service as it is deployed.
AI life cycle: All events and processes that relate to an AI system’s lifespan, from inception to decommissioning, including its design, research, training, development, deployment, integration, operation, maintenance, sale, use, and governance.
AI risks: The potential negative or harmful outcomes arising from the development or deployment of AI systems.
Alignment: The process of ensuring an AI system’s goals and behaviours are in line with human values and intentions.
Application Programming Interface (API): A set of rules and protocols that enables integration and communication between AI systems and other software applications.
Autonomous: Capable of operating, taking actions, or making decisions without the express intent or oversight of a human.
Capabilities: The range of tasks or functions that an AI system can perform and the proficiency with which it can perform them.
Compute: Computational processing power, including Central Processing Units (CPUs), Graphics Processing Units (GPUs), and other hardware, used to run AI models and algorithms.
Developers of highly capable general-purpose systems: A subsection of AI developers, these organisations invest large amounts of resource into designing, building, and pre-training the most capable AI foundation models. These models can underpin a wide range of AI applications and may be deployed directly or adapted by downstream AI developers.
Disinformation: Deliberately false information spread with the intent to deceive or mislead.
Foundation models: Machine learning models trained on very large amounts of data that can be adapted to a wide range of tasks.
Frontier AI: For the AI Safety Summit, we defined frontier AI as models that can perform a wide variety of tasks and match or exceed the capabilities present in today’s most advanced models. In this paper, we focus on highly capable general-purpose AI model developers to target our proposals for new responsibilities.
Misinformation: Incorrect or misleading information spread without harmful intent.
Safety and security: The protection, wellbeing, and autonomy of civil society and the population[footnote 8]. In this publication, safety is often used to describe prevention of or protection against AI-related harms. AI security refers to protecting AI systems from technical interference such as cyber-attacks[footnote 9].
Superhuman performance: When an AI model demonstrates capabilities that exceed human ability benchmarking for a specific task or activity.
Box 1: Different types of AI systems
In our discussion paper on frontier AI capabilities and risks[footnote 10], we noted that definitions of AI are often challenging due to the quick advancements in the technology.
For the purposes of developing a proportionate regulatory approach that effectively addresses the risks posed by the most powerful AI systems, we currently distinguish between:
-
Highly capable general-purpose AI: Foundation models that can perform a wide variety of tasks and match or exceed the capabilities present in today’s most advanced models. Generally, such models will span from novice through to expert capabilities with some even showing superhuman performance across a range of tasks.
-
Highly capable narrow AI: Foundation models that can perform a narrow set of tasks, normally within a specific field such as biology, with capabilities that match or exceed those present in today’s most advanced models. Generally, such models will demonstrate superhuman abilities on these narrow tasks or domains.
-
Agentic AI or AI agents: An emerging subset of AI technologies that can competently complete tasks over long timeframes and with multiple steps. These systems can use tools such as coding environments, the internet, and narrow AI models to complete tasks.
4. Introduction
1. The UK’s AI sector is thriving. The AI industry in the UK employs over 50,000 people and contributes £3.7 billion to economy[footnote 11]. Our universities produce some of the best AI research and talent, and the UK is home to the third largest number of AI unicorns and start-ups in the world[footnote 12].
2. Our goal is to make the UK a great place to build and use AI that changes our lives for the better. AI is the defining technology of our time and the UK is leading the world with our response.
3. In March 2023, we published a white paper setting out our proposals to establish a regulatory framework for AI to drive safe, responsible innovation[footnote 13]. We set five principles for regulators to interpret and apply within their domains. We also included proposals for a central function within government to conduct a range of activities such as risk assessment and regulatory coordination to support the adaptability and coherence of our approach.
4. We held a 12-week public consultation on our proposals[footnote 14]. We have now analysed the evidence (see Annex A for details) which has informed our approach. We thank everyone for their submissions. We have also built into our response the key achievements from the AI Safety Summit in November 2023, as well as themes from our engagement ahead of the Summit.
AI White Paper consultation and AI Summit activities
AI White Paper consultation and AI Summit activities.
5. The pace of AI development continues to accelerate. In the run up to the AI Safety Summit, we published a discussion paper on AI risks and capabilities that showed these trends are likely to continue in line with companies building these technologies using more compute, more data, and increasingly efficient algorithms[footnote 15]. Some frontier AI labs have stated their goal to build AI systems that are more capable than humans at a range of tasks[footnote 16].
6. Enhanced capabilities bring new opportunities. AI is already changing the way that we live and work. Workers using AI in sectors ranging from manufacturing to finance have reported improvements to their job enjoyment, performance, and health[footnote 17]. AI will change the tasks we do at work and the skills we need to do them well[footnote 18]. Recent AI developments are also changing how we spend our leisure time, with powerful AI systems underpinning the chatbots and image generators that have become some of the fastest growing consumer applications in history[footnote 19]. Highly capable AI is already transforming sectors, from helping us to conserve our wildlife[footnote 20] to changing the ways that we identify and treat disease[footnote 21].
7. However, more powerful AI also poses new and amplified risks. For example, AI chatbots may make false information more prominent[footnote 22] or a highly capable AI system may be misused to enable crime. For instance, a model designed for drug discovery could potentially be accessed maliciously to create harmful compounds[footnote 23].
8. AI may also fundamentally transform life in ways that are hard to predict. For instance, future agentic AI systems may be able to pursue complex goals with limited human supervision, raising questions around how AI agents remain attributable, ask for approval before taking action, and can be interrupted.
9. AI technologies present significant uncertainties that require an agile regulatory approach that supports innovation whilst adapting to address new risks. In this consultation response, we show how our flexible approach is already addressing key AI-related risks and how we are further strengthening this framework (section 5.1). We also set out initial thinking on potential new responsibilities on the developers of highly capable general-purpose AI systems alongside the voluntary commitments secured at the AI Safety Summit (section 5.2). In section 6, we provide a summary of the evidence we received to our consultation along with our formal response.
5. A regulatory framework to keep pace with a rapidly advancing technology
10. In the AI regulation white paper, we proposed five cross-sectoral principles for existing regulators to interpret and apply within their remits in order to drive safe, responsible AI innovation[footnote 24]. These are:
- Safety, security and robustness.
- Appropriate transparency and explainability.
- Fairness.
- Accountability and governance.
- Contestability and redress.
11. We welcome the strong support for these principles through the consultation. They are the foundation of our approach. We remain committed to a context-based approach that avoids unnecessary blanket rules that apply to all AI technologies, regardless of how they are used. This is the best way to ensure an agile approach that stands the test of time.
12. We are pleased to see how regulators are already independently implementing our principles. In the white paper we highlighted the importance of a central function to support regulator capabilities and coordination. We have made good progress establishing this function within the government. We set out below how we are further strengthening it, including new funding, in section 5.1. We also show how regulators and the government are addressing some of the most important issues facing us today.
13. In section 5.2, we set out some of the regulatory challenges posed by the rapid development of highly capable general-purpose systems; how we are currently tackling these through voluntary measures, including those agreed at the AI Safety Summit; and which additional responsibilities may be required in the future to address risks effectively.
5.1. Delivering a proportionate, context-based approach to regulate the use of AI
5.1.1. Regulators are taking active steps in line with the framework
14. Since the publication of the AI regulation white paper, a number of regulators have set out work in line with our principles-based approach. For example, the Competition and Markets Authority (CMA) published a review of foundation models to understand the opportunities and risks for competition and consumer protection[footnote 25]. The Information Commissioner’s Office (ICO) updated guidance on how data protection laws apply to AI systems to include fairness[footnote 26]. To ensure the safety of AI, regulators such as the Office of Gas and Electricity Markets (Ofgem) and Civil Aviation Authority (CAA) are working on AI strategies to be published later this year. This builds on regulator work that led the way on clarifying how existing frameworks apply to AI risks in their domain, such as the Medicines and Healthcare products Regulatory Agency (MHRA) Software and AI as a Medical Device Change Programme 2021 on requirements for software and AI used in medical devices[footnote 27].
15. It is important that the public have full visibility of how regulators are incorporating the principles into their work. The government has written to a number of regulators impacted by AI to ask them to publish an update outlining their strategic approach to AI by 30 April [footnote 28]. We are encouraging regulators to include:
- An outline of the steps they are taking in line with the expectations set out in the white paper.
- Analysis of AI-related risks in the sectors and activities they regulate and the actions they are taking to address these.
- An explanation of their current capability to address AI as compared with their assessment of requirements, and the actions they are taking to ensure they have the right structures and skills in place.
- A forward look of plans and activities over the coming 12 months.
16. When we published the AI regulation white paper, we proposed that the principles would be established on a non-statutory basis. Many consultation respondents noted the potential benefits of a statutory duty on regulators, but some acknowledged that implementing the regime on a non-statutory basis in the first instance would allow for important flexibilities. We think a non-statutory approach currently offers critical adaptability – especially while we are still establishing our approach – but we will keep this under review. Our decision will be informed in part by our review of the plans published by regulators, as set out above; our review of regulator powers, as set out below; and in line with our wider approach to AI legislation, such as the introduction of targeted binding measures (see section 5.2).
5.1.2 Supporting regulatory capability and coordination
17. The systemic changes driven by AI demand a system-wide response – our individual regulators cannot successfully address the opportunities and risks presented by AI technologies within their remits by acting in isolation. In the AI regulation white paper, we proposed a new central function, established within government, to monitor and assess risks across the whole economy and support regulator coordination and clarity.
18. The proposal for a central function was widely welcomed by stakeholders who noted it is critical to the effective delivery of the AI regulation framework. Many stressed that, without such a function, there is a risk of regulatory overlaps, gaps, and poor coordination as multiple regulators consider the impact of AI in their domains.
19. We have already started to establish this function in a range of ways:
i. Risk assessment: We have recruited a new multidisciplinary team to undertake cross-sectoral risk monitoring within the Department for Science, Innovation and Technology (DSIT), bringing together expertise in risk, regulation, and AI with backgrounds in data science, engineering, economics, and law. This team will provide continuous examination of cross-cutting AI risks, including evaluating the effectiveness of interventions by both the government and regulators. In 2024, we will launch a targeted consultation on a cross-economy AI risk register to ensure it comprehensively captures the range of risks. It will provide a single source of truth on AI risks which regulators, government departments, and external groups can use. It will also support government work to identify any risks that fall across or in between the remits of regulators so we can identify where there are gaps or existing regulation is ineffective and prioritise further action. In addition to the risk register, we are considering the added value of developing a risk management framework, similar to the one developed in the US by the National Institute of Standards and Technology (NIST).
ii. Regulator capabilities: Effective regulation relies on regulators having the right skills, tools, and expertise. While some regulators have been able to put the right expertise in place to address AI, others are less prepared. We are announcing £10 million for regulators to develop the capabilities and tools they need to adapt and respond to AI. We are investing in regulators today to future-proof their capabilities for tomorrow. The funding will enable regulators to collaborate to create, adapt, and improve practical tools to address AI risks and opportunities within and across their remits. It will enable regulators to carry out research and development to produce novel, actionable insights that will set the foundation of their approaches for years to come. We will work closely with regulators in the coming months to identify the most promising opportunities to leverage this funding. This builds on the recent announcement that the government will explore how to further support regulators to develop the specialist skills necessary to regulate emerging technologies, including options for increased flexibility on pay and conditions[footnote 29].
iii. Regulator powers: We recognise the need to assess the existing powers and remits of the UK’s regulators to ensure they are equipped to address AI risks and opportunities in their domains and implement the principles in a consistent and comprehensive way. We will, therefore, work with government departments and regulators to analyse and review potential gaps in existing regulatory powers and remits.
iv. Coordination: In the coming months we will formalise our regulator coordination activities. To support and guide this work, we will establish a steering committee with government representatives and key regulators to support knowledge exchange and coordination on AI governance by spring 2024. We continue to support regulatory coordination more widely, including working with bodies such as the Digital Regulation Cooperation Forum (DRCF). Today we have published new guidance for regulators to support them to interpret and apply our principles.
v. Research and innovation: We are working closely with UK Research and Innovation (UKRI) to ensure the government’s wider investments in AI R&D can support the government’s safety agenda. This includes a new commitment by UKRI to improve links between regulators and the skills, expertise, and activities supported by UKRI investments in AI research such as Responsible AI UK, the Trustworthy Autonomous Systems hub, the UKRI AI Centres for Doctoral Training, and the Alan Turing Institute. This will ensure the UK’s strength in AI research is fully utilised in our regulatory framework. This work builds on our previous commitment of £250 million through the UKRI Technology Missions Fund to secure the UK’s global leadership in critical technologies[footnote 30]. UKRI is today announcing that £19 million of the Technology Missions Fund will support Phase 2 of the Accelerating Trustworthy AI competition, supporting 21 projects delivered through the Innovate UK BridgeAI programme, to accelerate the adoption of trusted and responsible AI and machine learning.
vi. Ease of compliance: Regulation must work for innovators. We are supporting innovators and businesses to get new products to market safely and efficiently by funding a pilot multi-agency advisory service delivered by the DRCF[footnote 31]. This will particularly help innovators navigate the legal and regulatory requirements they need to meet before launch. The online portal for the pilot 1. DRCF AI and Digital Hub and the application window are due to launch in the spring. Insights from the pilot will inform the implementation of our regulatory approach. Further details on the eligibility criteria for the support to be offered by the pilot have been published by the DRCF today alongside this consultation response.
vii. Public trust: We want businesses, consumers, and the public to have confidence in AI technologies. We will build trust by continuing to support work on assurance techniques and technical standards. The UK AI Standards Hub, launched in 2022, provides practical tools and guides for businesses, organisations, and individuals to effectively use digital technical standards and participate in their development[footnote 32] In 2023, the government collaborated with techUK to launch the Portfolio of AI Assurance Techniques announced in the AI regulation white paper[footnote 33]. In spring 2024, we will publish an “Introduction to AI assurance” to further promote the value of AI assurance and help businesses and organisations build their understanding of the techniques for safe and trustworthy systems. Alongside this, we undertake regular research with the public to ensure the government’s approach to AI is aligned with our wider values[footnote 34].
viii. Monitoring and Evaluation: We are developing a monitoring and evaluation plan that allows us to continuously assess the effectiveness of our regime as AI technologies change. We will conduct a targeted consultation with a range of stakeholders on our proposed plan to assess the regulatory framework in spring 2024. As part of this, we will seek detailed views on our proposed metrics and data sources.
20. AI regulation will only work within a wider ecosystem that champions the industry. In 2023, the government committed over £1.5 billion to build public sector supercomputers, including the AI Research Resource and an exascale computer. We are also working closely with the private sector to support investment, such as Microsoft’s announcement of £2.5 billion for AI-related data centres in November 2023. The £80 million investment in AI hubs that we are announcing today will enable AI to evolve and tackle complex problems across applications from healthcare treatments to power-efficient electronics. The government is also conducting a wider review of the UK AI supply chain to ensure we maintain our strategic advantage as a world leader in these technologies.
21. Finally, to drive coordinated action across government we have established lead AI Ministers across all departments to bring together work on risks and opportunities driven by AI in their sectors and to oversee implementation of frameworks and guidelines for public sector usage of AI. We are also establishing a new Inter-Ministerial Group to drive effective coordination across government on AI issues. Further to this, we are strengthening the team working on AI within the DSIT. In February 2023, we had a team of around 20 people working on AI issues. This had grown to over 160 across the newly established AI Policy Directorate and the AI Safety Institute by the end of 2023, with plans to expand to more than 270 people in 2024. In recognition of the fact that AI is a top priority for the Secretary of State and has become central to the wider work of the department and government, we will no longer maintain the branding of a separate Office for AI. Similarly, the Centre for Data Ethics and Innovation (CDEI) is changing its name to the Responsible Technology Adoption Unit to more accurately reflect its mission. The name highlights the directorate’s role in developing tools and techniques that enable responsible adoption of AI in the private and public sectors, in support of the department’s central mission.
AI governance landscape
AI regulation landscape.
DSIT - the government department responsible for overall responsibility for AI policy, including regulation.
Image 1: A diagram of the AI regulation landscape showing the relationships between the government, regulators, industry, and the wider ecosystem.
5.1.3 Tackling specific risks
22. There are three broad categories of AI risk: societal harms; misuse risks; and autonomy risks[footnote 35]. Below we outline examples of how the government and regulators are responding to specific risks in line with our principles. This summary illustrates the wide range of work already happening to ensure the benefits of AI innovation can be realised safely and responsibly. It is not intended to be exhaustive or prioritise certain risks over others.
23. In addition to the work to address specific risks outlined below, we are today announcing £2 million of Arts and Humanities Research Council (AHRC) funding to support translational research that will help to define responsible AI across sectors such as education, policing, and creative industries. These projects, part of the AHRC’s Bridging Responsible AI Divides (BRAID) work[footnote 36], will produce recommendations to inform future work in this area and demonstrate how the UK is at the forefront of embedding AI across key sectors. In addition to the scoping projects, AHRC are confirming a further £7.6 million to fund a second phase of the BRAID programme, extending activities to 2027/28. The next phase will include a new cohort of large-scale demonstrator projects, further rounds of BRAID Fellowships, and new professional AI skills provisions, co-developed with industry and other partners.
Societal harms
Preparing UK workers for an AI enabled economy
24. AI is revolutionising the workplace. While the adoption of these technologies can bring new, higher quality jobs, it can also create and amplify a range of risks, such as workplace surveillance and discrimination in recruitment, that the government and regulators are already working to address. We want to harness the growth potential of AI but this must not be at the expense of employment rights and protections for workers. The UK’s robust system of legislation and enforcement for employment protections, including specialist labour tribunals, sets a strong foundation for workers. To ensure the use of AI in HR and recruitment is safe, responsible, and fair, the Department for Science, Innovation and Technology (DSIT) will provide updated guidance in spring 2024.
25. Since 2018 we have funded a £290 million package of AI skills and talent initiatives to make sure that AI education and awareness is accessible across the UK. This includes funding 24 AI Centres for Doctoral Training which will train over 1,500 PhD students. We are also working with Innovate UK and the Alan Turing Institute to develop guidance that sets out the core AI skills people need, from ‘AI citizens’ to ‘AI professionals’. We published draft guidance for public comment in November 2023 and we intend to publish a final version and a full skills framework in spring 2024[footnote 37].
26. It is hard to predict, at this stage, exactly how the labour market will change due to AI. Some sectors are concerned that AI will displace jobs through automation[footnote 38]. The Department for Education (DfE) has published initial work on the impact of AI on UK jobs, sectors, qualifications, and training pathways[footnote 39]. We can be confident that we will need new AI-related skills through national qualifications and training provision. The government has invested £3.8 billion in higher and further education in this parliament to make the skills system employer-led and responsive to future needs. Along with DfE’s Apprenticeships[footnote 40] and Skills Bootcamps[footnote 41], the new Lifelong Learning Entitlement reforms[footnote 42] and Advanced British Standard[footnote 43] will put academic and technical education in England on an equal footing and ensure our skills and education system is fit for the future.
Enabling AI innovation and protecting intellectual property
27. The AI technology and creative sectors, as well as our media, are strongest when they work together in partnership. This government is committed to supporting these sectors so that they continue to flourish and are able to compete internationally. The Department for Culture, Media and Sport (DCMS) is working closely with publishers, the music industry, and other creative businesses to understand the impact of AI on these sectors, with a view to mitigating risks and capitalising on opportunities. Significant funding highlighted in the Creative Industries Sector Vision[footnote 44] will help enable AI-based R&D and innovation in the creative industries.
28. Creative industries and media organisations have particular concerns regarding copyright protections in the era of generative AI. Creative industries and rights holders are concerned at the large-scale use of copyright protected content for training AI models and have called for assurance that their ability to retain autonomy and control over their valuable work will be protected. At the same time, AI developers have emphasised that they need to be able to easily access a wide range of high-quality datasets to develop and train cutting-edge AI systems in the UK.
29. The Intellectual Property Office (IPO) convened a working group made up of rights holders and AI developers on the interaction between copyright and AI. The working group has provided a valuable forum for stakeholders to share their views. Unfortunately, it is now clear that the working group will not be able to agree an effective voluntary code.
30. DSIT and DCMS ministers will now lead a period of engagement with the AI and rights holder sectors, seeking to ensure the workability and effectiveness of an approach that allows the AI and creative sectors to grow together in partnership. The government is committed to the growth of our world-leading creative industries and we recognise the importance of ensuring AI development supports, rather than undermines, human creativity, innovation, and the provision of trustworthy information.
31. Our approach will need to be underpinned by trust and transparency between parties, with greater transparency from AI developers in relation to data inputs and the attribution of outputs having an important role to play. Our work will therefore also include exploring mechanisms for providing greater transparency so that rights holders can better understand whether content they produce is used as an input into AI models. The government wants to work closely with rights holders and AI developers to deliver this. Critical to all of this work will also be close engagement with international counterparts who are also working to address these issues. We will soon set out further proposals on the way forward.
Protecting UK citizens from AI-related bias and discrimination
32. AI has the potential to entrench bias and discrimination[footnote 45], possibly leading to unfairly negative outcomes for different populations across a range of sectors. For example, unaccounted for bias in an AI-enabled automated decision making process could result in discriminatory outcomes against specific demographic characteristics in areas such as credit applications[footnote 46] or recruitment[footnote 47]. In line with our fairness principle, the department is working closely with the Equality and Human Rights Commission (EHRC) and ICO to develop new solutions to address bias and discrimination in AI systems[footnote 48].
33. Both regulators and public sector bodies are acting to address AI-related bias and discrimination in their domains. The ICO has updated guidance on how our strong data protection laws apply to AI systems that process personal data to include fairness and has continued to hold organisations to account, for example through the issuing of enforcement notices[footnote 49]. The Office of the Police Chief Scientific Adviser published a Covenant for Using AI in Policing[footnote 50] which has been endorsed by the National Police Chiefs’ Council and should be given due regard by all developers and users of the technology in the sector.
Reforming data protection law to support innovation and privacy
34. Data is the foundation for modelling, training, and developing AI systems. But it is critical to respect relevant individual rights and data protection principles should be complied with when processing personal data in AI systems. The ICO has demonstrated how they can use data protection law to hold organisations to account through regulatory action and public communications where AI systems are processing personal data. The UK’s data protection framework, which is being reformed through the Data Protection and Digital Information Bill (DPDI), will complement our pro-innovation, proportionate, and context-based approach to regulating AI.
35. Current rules on automated decision-making are confusing and complex, undermining confidence to develop and use innovative technologies. The DPDI Bill will expand the lawful bases on which solely automated decisions that have significant effects on individuals can take place and provide a boost in confidence to organisations looking to use the technologies responsibly. It will continue to ensure that data subject rights are protected with safeguards in place. For example, data subjects will be provided with information on such decisions, have the opportunity to make representations, and can request human intervention or contest the decision. This will support innovation and reduce burdens on people and businesses, while maintaining data protection safeguards in line with the UK’s high standards of data protection.
Ensuring AI generated online content is trusted and safe
36. The government is committed to ensuring that people have access to accurate information and is supporting all efforts to promote verifiable sources to tackle the spread of false or misleading information. AI technologies are increasingly able to provide individuals with cheap ways to generate realistic content that can falsely portray people and events. Similarly, AI may increase volumes of unintentionally false, biased, or harmful content[footnote 51]. This may drive negative public perceptions of information quality and lower overall trust in information sources[footnote 52].
37. We have published emerging practices to protect trust in online information including watermarking and output databases[footnote 53]. We will shortly launch a call for evidence on AI-related risks to trust in information to develop our understanding of this fast moving and nascent area of technological development, including possible mitigations. This will be aimed at researchers, academics, and civil society organisations with relevant expertise. We will also explore research into the wider and systemic impacts on the information ecosystem and potential solutions. We also continue to engage with news publishers and broadcasters, as vital channels for trustworthy and verifiable information, on the risks of AI to journalism.
Ensuring AI driven digital markets are competitive
38. AI is creating huge opportunities for innovation that benefits businesses and consumers across the economy. The markets for both the underlying AI technologies, such as foundation models, and products that use AI in new and innovative ways, are growing quickly.
39. Where these markets are competitive they will drive innovation and better outcomes for businesses and consumers. Successful firms will rightly grow and increase their market share, but it will be important that market power does not become entrenched by only a small number of firms.
40. The CMA will take steps to ensure that AI markets work well for all. In September 2023, the regulator published an initial review into the market for foundation models[footnote 54]. The report found that, while there will be many benefits to consumers from AI, these technologies could enable firms to gain or entrench market power. The Digital Markets, Competition and Consumers Bill, which is currently progressing through Parliament, will give the CMA additional tools to identify and address any competition issues in AI markets and other digital markets affected by recent developments in AI.
Ensuring AI best practice in the public sector
41. AI poses enormous opportunities for transforming productivity in the public sector. The UK is already leading the way, ranked third in the Government AI Readiness Index.[footnote 55] In November 2023, we announced that we are tripling the number of technical AI engineers and developers within the Cabinet Office to create a new AI Incubator for the government. These experts will design and implement AI solutions across government departments to drive improvements in public service delivery. This potential productivity improvement could, for example, save police up to 38 million hours per year and 750,000 hours every week[footnote 56].
42. We are seizing the opportunities presented by AI to deliver better public services including health, education, and transport. For example, last year the Department of Health and Social Care (DHSC) and NHS launched the £21 million AI Diagnostic Fund to deploy these technologies in key, high demand areas such as chest X-rays and CT scans[footnote 57]. DfE has been examining how to maximise the benefits of AI in the education sector, including publishing a policy paper and a call for evidence on generative AI in education[footnote 58], as well as running a hackathons project to further understand possible use cases. The findings of the hackathons will be published in spring of this year. The Department for Transport (DfT) is focused on the new Automated Vehicles Bill, designed to put the UK at the forefront of regulation of self-driving technology and in a strong position to realise an estimated £42 billion share of the global self-driving market. DfT also plans to publish its first Transport AI Strategy in 2024, to help both the department and the wider sector to grasp the opportunities and risks presented by new AI capabilities. Alongside this, the department continues to fund innovative Small and Medium sized Enterprises (SMEs) through its Transport Research and Innovation Grants scheme to support the next generation of AI tools and applications as well as trialling AI to support fraud identification in its grant-making processes.
43. Cabinet Office (CO) is leading on establishing the necessary underpinnings to drive AI adoption across the public sector, by improving digital infrastructure and access to data sets, and developing centralised standards. The government is also using the procurement power of the public sector to drive responsible and safe AI innovation. The Central Digital and Data Office (CDDO) has published guidance on the procurement and use of generative AI for the UK government[footnote 59]. Later this year, DSIT will launch the AI Management Essentials scheme, setting a minimum good practice standard for companies selling AI products and services. We will consult on introducing this as a mandatory requirement for public sector procurement, using purchasing power to drive responsible innovation in the broader economy.
44. This builds on the Algorithmic Transparency Recording Standard (ATRS), which established a standardised way for public sector organisations to proactively publish information about how and why they are using algorithmic methods in decision-making. Following a successful pilot of the standard, and publication of an approved cross-government version last year, we will now be making use of the ATRS a requirement for all government departments and plan to expand this across the broader public sector over time.
45. To inform the secure use of AI across government, the public sector, and beyond, the National Cyber Security Centre (NCSC) has published a range of guidance products on the cyber security considerations around using and developing AI[footnote 60].
Misuse risks
Safeguarding democracy from electoral interference
46. The government is committed to strengthening the integrity of elections to ensure that our democracy remains secure, modern, transparent, and fair. AI has the potential to increase the reach of actors spreading disinformation online, target new audiences more effectively, and generate new types of content that are more difficult to detect[footnote 61]. Our Defending Democracy Taskforce is helping to reduce the threat of foreign interference in our democracy by bringing together a wide range of expertise across government, the intelligence community, and industry. In 2024, the Taskforce will be increasing its engagement with partners, collaborating with devolved governments, the police, local authorities, tech companies, and international partners.
47. We will always respond firmly to any threats to the UK’s democracy. The Elections Act 2022 introduced the new digital imprints regime, which will increase the transparency of digital political advertising (including AI-generated material), by requiring those promoting eligible digital campaigning material targeted at the UK electorate to include an imprint with their name and address. This will empower voters to know who is promoting political material online and on whose behalf. The Elections Act 2022 also revised the offence of undue influence. This will better protect voters from improper influences to vote in a particular way, or to not vote at all, and includes activities that deceive a person in relation to the administration of an election (such as the date of an electoral event or the location of a polling station).
48. The Online Safety Act 2023 will capture specific activity aimed at disrupting elections where it is a criminal offence in scope of the regulatory framework. This includes content that contains incitement to violence against electoral candidates and public figures, and the offence of undue influence. The foreign interference offence from the National Security Act 2023 has been added to the Online Safety Act as a “priority offence”, putting new responsibilities on online service providers and capturing attempts by foreign state actors to manipulate our information environment and undermine our democratic, political, and legal processes (including elections). The Online Safety Act has also updated Ofcom’s statutory media literacy duty, requiring the regulator to heighten the public’s awareness of, and resilience to, misinformation and disinformation online.
49. We will consider the tools available to verify election-related content. This could include using watermarks to give people confidence in the content they are viewing. It is not just the government that needs to act. We will continue to work with tech companies to ensure that it is possible to report and remove fakes quickly. Building on discussions at the AI Safety Summit, we are collaborating with international and industry partners to address the shared risk of election interference.
Preventing the misuse of AI technologies
50. AI capabilities may be used maliciously, for example, to perform cyberattacks or design weapons[footnote 62]. Developments in AI can amplify existing risks by enabling less sophisticated threat actors to carry out more substantial attacks at a larger scale[footnote 63]. We are working with industry, academia, and international partners to find proportionate, practical mitigations to these risks. The 2023 refreshed Biological Security Strategy will ensure that by 2030 the UK is resilient to a spectrum of biological risks and a world leader in responsible innovation[footnote 64]. As set out in the National Vision for Engineering Biology, the government has identified screening of synthetic DNA as a responsible innovation policy priority for 2024[footnote 65]. Prioritising this will allow us to continue reaping the economic rewards of engineering biology in the UK whilst improving the safety of the supply chain.
51. Some of the risks presented by AI systems are manifesting today as these technologies are misused to increase the scale, speed, and success of criminal offences. As discussed above, AI can provide users with increasing capability to produce false or misleading content. This can include material that constitutes a criminal offence such as fraud, online child sexual abuse, and intimate image abuse. The government has already moved to address some of these issues in the Online Safety Act 2023. Some AI technologies could be misused to commit identity-related fraud, such as producing false documentation used for immigration purposes. These capabilities present potential risks related to fraudulent access to public funds.
52. In order to address the potential criminal use of AI, we are reviewing the extent to which existing criminal law provides coverage of AI-enabled offending and harmful behaviour. AI may also present systemic risks to police capacity, institutional trust, and the evidential process. The government will make amendments to existing legal frameworks as required in order to protect law and order. AI also poses more opportunities for law enforcement to become more efficient at detecting and preventing crime. As such, these technologies may help mitigate some of the risks of AI-enabled criminal offences. For example, we are investing in AI models that allow police to detect and categorise the severity of child abuse images more effectively. We are also exploring how AI might enable officers to redact large amounts of text evidence more quickly.
53. To help organisations develop and use AI securely, the NCSC published guidelines for secure AI system development in November 2023. The government is now looking to build on this and other important publications by releasing a call for views in spring 2024 to obtain further input on our next steps in securing AI models, including a potential Code of Practice for cyber security of AI, based on NCSC’s guidelines. International collaboration in this area is vital if we are to see meaningful change to the security of AI models, and we will be exploring ways to promote international alignment, such as via international standards.
54. This builds on our work to secure personal devices and critical infrastructure. The security regime in the Product Security and Telecommunications Infrastructure (“PSTI”) Act, scheduled to come into effect in 2024, will require manufacturers of consumer connectable products, such as AI-enabled smart speakers, to comply with minimum security requirements underpinned by the secure by design principle. This means no consumer connectable products in scope of the regime can be made available to UK customers unless the manufacturer has minimum security measures in place covering the product’s hardware and software, and, where appropriate, associated AI solutions. Beyond this, the National Protective Security Authority (NPSA) conducts research to understand how AI can, and will, enhance physical and personnel security. NPSA advises a wide range of organisations, including critical national infrastructure companies, on how to address AI-related threats and delivers campaigns to help protect valuable AI-related intellectual property for emerging technology companies.
Autonomy risks
55. In our discussion paper on frontier AI capabilities and risks[footnote 66], we outlined potential future risks linked to the increasing autonomy of advanced AI systems. Some experts are concerned that, as AI systems become more capable across a wider range of tasks, humans will increasingly rely on AI to make important decisions. Some also believe that, in the future, agentic AI systems may have the capabilities to actively reduce human control and increase their own influence. New research on the advancing capabilities of agentic AI demonstrates that we may need to consider potential new measures to address emerging risks as the foundational AI technologies that underpin a range of applications continue to develop[footnote 67].
56. In section 5.2, we set out proposals for new future responsibilities on developers of highly capable general-purpose AI. While the likelihood of autonomy risks is debated, we believe that our proposals introduce accountability, governance, and oversight for these developers as well as testing and benchmarking powerful AI systems to address these risks now and in the future. In particular, the testing conducted by the AI Safety Institute will identify systems with potentially hazardous capabilities (see sections 5.2 and 5.3 for more details on the role of the Institute). Testing has already begun and will increase in pace over the following months. These initial steps build the UK’s technical capability to assess and respond to emerging AI risks, ensuring our resilience to future technological developments.
5.2. Examining the case for new responsibilities for developers of highly capable general-purpose AI systems
57. As noted above, we are seeing rapid progress in the performance of highly capable general-purpose AI systems. We expect this to continue as organisations develop them with more compute, more data, and more efficient algorithms. Developers do not always know which capabilities a model may exhibit before testing[footnote 68]. Some companies have publicly stated their goal to build AI systems that are more capable than humans at a range of tasks[footnote 69]. With agentic AI capabilities on the horizon, we expect further transformative changes to our societies[footnote 70].
58. The Prime Minister set out the government’s approach to managing risk at the frontier of AI development in October 2023. He stated: “My vision, and our ultimate goal, should be to work towards a more international approach to safety, where we collaborate with partners to ensure AI systems are safe before they are released[footnote 71].”
59. We set out below how the UK has led the way with a technical approach, securing voluntary agreements on AI safety with key countries and companies. The new AI Safety Institute will work with its partners to test the most powerful new AI systems pre- and post- deployment. As the Prime Minister set out, we will not “rush to regulate” and potentially implement the wrong measures that may insufficiently balance addressing risks and supporting innovation.
60. Clearly, if the exponential growth of AI capabilities continues, and if – as we think could be the case – voluntary measures are deemed incommensurate to the risk, countries will want some binding measures to keep the public safe. Some countries, such as the United States are beginning to explore this through mandatory reporting requirements for the most powerful systems. We have seen significant interventions from leading figures in industry, science, and civil society, highlighting how governments should consider responding to the development[footnote 72] and we welcome continued close collaboration with these expert voices.
61. The UK will continue to lead the conversation on effective AI governance. In the section below, we set out some of the key questions that countries will have to grapple with when deciding how best to manage the risks of highly capable general-purpose AI systems, such as how to allocate liability across the supply chain and negotiate the open release of the most powerful systems. We will continue to discuss these questions with civil society, industry, and international partners to prepare for the future.
Box 2: What do we mean by highly capable general-purpose AI systems?
In the AI regulation white paper, we defined “foundation models” as “a type of AI model that is trained on a vast quantity of data and is adaptable for use on a wide range of tasks. Foundation models can be used as a base for building more specific AI models[footnote 73].”
For the purposes of the AI Safety Summit, the UK defined “frontier AI” as highly capable general-purpose AI models that can perform a wide variety of tasks and match or exceed the capabilities present in today’s most advanced models.
Today, this can include the cutting-edge foundation models that underpin consumer facing applications. However, it is important to note that, both today and in the future, highly capable AI systems could be underpinned by another technology.
In this consultation response, we focus our discussion on future responsibilities for the developers of highly capable general-purpose AI systems. Developers of these systems currently face the least clear legal responsibilities. The systems have the least coverage by existing regulation while presenting some of the greatest potential risk. This means some of those risks may not be addressed effectively. In the future, our regulatory approach might need to also allocate new responsibilities to developers of highly capable narrow systems as the framework continues to adapt to reflect new technological developments, different risks, or further analysis of accountability across the AI life cycle.
5.2.1. The regulatory challenges of highly capable general-purpose AI
62. The AI regulation white paper outlined a regulatory approach designed to adapt and keep pace with the rapid developments in AI technology. For the large majority of AI systems, our view is still that it is more effective to focus on how AI is used within a specific context than to regulate specific technologies. This is because the level of risk will be determined by where and how AI is used.
63. However, some highly capable AI systems can present substantial risks. Risk may increase when a highly capable system is general-purpose and can be used in a wide range of applications across different sectors. If a general-purpose AI system presents a risk of harm, this could mean that multiple sectors or applications could be at risk. This means that a single feature or flaw in one model might result in multiple harms across the whole economy. For example, if an AI system is used to underpin complex automated processes in both healthcare and recruitment, but the model’s outputs demonstrate bias in a way that is not sufficiently transparent or with impacts that are not adequately mitigated, this could result in discriminatory practices in these different services.
64. Highly capable general-purpose AI systems challenge a context-based approach to regulation as some of the risks that they contribute to may not be effectively mitigated by existing regulation. For example, the cross-sectoral impact of these systems may prevent harms from being sufficiently addressed. Even though some regulators can enforce existing laws against the developers of the most capable general-purpose systems within their current remits[footnote 74], the wide range of potential uses means that general-purpose systems do not currently fit neatly within the remit of any one regulator, potentially leaving risks without effective mitigations[footnote 75].
65. While some regulators demonstrate advanced approaches to addressing AI within their remits, many of our current legal frameworks and regulator remits may not effectively mitigate the risks posed by highly capable general-purpose AI systems. Many regulators in the UK can struggle to enforce existing rules on those actors designing, training, and developing the most powerful general-purpose AI systems. Similarly, it is not always clear how existing rules can be applied to effectively address the risks that highly capable general-purpose models can present. Existing rules and laws are frequently applied to the deployment or application level of AI, but the organisations deploying or using these systems may not be well placed to identify, assess, or mitigate the risks they can present. If this is the case, new responsibilities on the developers of highly capable general-purpose models may more effectively address risks.
66. Our ongoing work analysing life cycle accountability for AI, outlined in the white paper, may eventually need to consider the role of other actors across the value chain, such as data or cloud hosting providers, to determine how legal responsibility for AI may be distributed most fairly and effectively. This analysis will also consider how the unpredictable way future capabilities and risks may emerge could also expose further gaps in the regulatory landscape.
Case study 1: Liability as a barrier to AI adoption in the UK
“Count Your Pennies Ltd”, a fictional accountancy firm, purchases an “off the shelf” AI recruitment tool developed by a fictional UK company called “Quantum Talent Technologies”. The tool automatically shortlists candidates based on their application forms.
One fictional candidate, Ms Smith, queries why her application was rejected for a certain position given her clear suitability for the role. After receiving an unsatisfactory response from the recruiting manager, she files a discrimination claim. Through the investigation, it becomes clear that the AI tool is discriminatory. It was built using a powerful foundation model that was developed by a non-UK company and trained on biased historic employment data.
It’s common for the law to allocate liability to the last actor in the chain (in this case, “Count Your Pennies Ltd”). In limited circumstances, the law may also allocate liability to the actor immediately above in the supply chain (in this case, “Quantum Talent Technologies”)[footnote 76].
For example, it can be difficult for equality law – which is the statutory framework designed to legally protect people against discrimination in the workplace and in wider society[footnote 77] – to allocate liability to anyone other than the end deployer. This could ultimately lead to harmful outcomes (if the actors most able to address risks and harms are not incentivised or held accountable) and undermine AI adoption and dampen innovation across the UK economy. We will continue to analyse challenges such as these as part of our ongoing policy work on life cycle accountability for AI.
67. While highly capable narrow AI systems are in scope of the regulatory framework for AI, these systems may require a different set of interventions if they present potentially dangerous capabilities. Narrow systems are more likely than general-purpose systems to be subject to effective regulation within the remit of an existing regulator. We will continue to gather evidence on whether the specialised nature of highly capable narrow systems demands a different approach to general-purpose systems
5.2.2. The role of voluntary measures in initially building an effective and targeted regulatory approach
68. We have already started to make the world safer today by securing commitments from leading AI companies on voluntary measures. Building on voluntary commitments brokered by the White House, the Secretary of State for Science, Innovation and Technology wrote to seven frontier AI companies prior to the AI Safety Summit requesting that they publish their safety policies. All seven companies published their policies before the AI Safety Summit, increasing transparency within the AI community and encouraging safe industry practice[footnote 78]. We also published a report on emerging processes for frontier AI safety to inform the future development of safety policies (see Box 3)[footnote 79]. In 2024, we will encourage AI companies to develop their AI safety and responsible capability scaling policies[footnote 80]. As part of this work, we will update our emerging processes guide by the end of the year.
Box 3: Emerging Processes for Frontier AI Safety
Ahead of the AI Safety Summit, the UK government outlined a set of emerging safety processes to provide information to companies on how they can ensure and maintain the safety of AI technologies.
The document covers nine emerging processes:
1. Responsible Capability Scaling - a framework for managing risk as organisations scale the capability of frontier AI systems, enabling companies to prepare for potential future, more dangerous AI risks before they occur.
2. Model Evaluations and Red Teaming - methods to assess the risks AI systems pose and inform better decisions about training, securing, and deploying them.
3. Model Reporting and Information Sharing - practices that increase government visibility of frontier AI development and deployment and enable users to make well-informed choices about whether and how to use AI systems.
4. Security Controls including Securing Model Weights - measures such as cyber security and other security controls that underpin AI system security.
5. Reporting Structure for Vulnerabilities - a process to enable outsiders to identify safety and security issues in an AI system.
6. Identifiers of AI-generated Material - tools to mitigate the creation and distribution of deceptive AI-generated content by providing information about whether content has been AI generated or modified.
7. Prioritising Research on Risks Posed by AI - research processes to identify and address the emerging risks posed by frontier AI.
8. Preventing and Monitoring Model Misuse - practices to identify and prevent intentional misuse of AI systems.
9. Data Input Controls and Audits - measures to identify and manage training data that is likely to increase the dangerous capabilities their frontier AI systems possess, and the risks they pose.
The document consolidated emerging thinking in AI safety from research institutes and academia, companies, and civil society, who the UK government collaborated and engaged with throughout its development. AI safety is an ongoing project and the processes and practices will continue to evolve through research and dialogue between governments and the broader AI ecosystem. The document provides a useful starting point for future frameworks for action both in the UK and globally.
69. Alongside these voluntary measures, at the AI Safety Summit, governments and AI companies agreed that both parties have a crucial role to play in testing the next generation of AI models, to ensure AI safety – both before and after models are deployed. In the UK, the newly established AI Safety Institute (see Box 4) leads this work. Leading AI tech companies have pledged to provide the Institute with priority access to their systems. The Institute has already begun testing, and is committed to doing so in partnership with other countries and their respective safety institutes. We will shortly provide an update on the AI Safety Institute’s approach to evaluations. Our assessment of the capabilities and risks of AI will also be underpinned by a new International Report on the Science of AI Safety[footnote 81], chaired by leading AI pioneer Yoshua Bengio (see paragraph 87).
Box 4: The AI Safety Institute (AISI)
At present, frontier AI developers are building powerful systems that outpace the ability of government and regulators to make them safe. As such, the government’s first challenge is one of knowledge: we do not fully understand what the most powerful systems are capable of and we urgently need to plug that gap. This will be the task of the new AI Safety Institute. It will advance the world’s knowledge of AI safety by carefully examining, evaluating, and testing new frontier AI systems. In addition, it will research new techniques for understanding and mitigating AI risk, and conduct fundamental research on how to keep people safe in the face of fast and unpredictable progress in AI.
The AI Safety Institute’s work will be fundamental to informing the UK’s regulatory framework. It will provide foundational insights to our governance regime and help ensure that the UK takes an evidence-based, proportionate approach to regulating the risks of AI. It will initially perform three core functions:
- Develop and conduct evaluations on advanced AI systems, aiming to characterise safety-relevant capabilities, understand the safety and security of systems, and assess their societal impacts.
- Drive foundational AI safety research. The Institute’s research will support short and long-term AI governance. It will ensure the UK’s iterative regulatory framework for AI is informed by the latest expertise and lay the foundation for technically grounded international governance of advanced AI. Projects will range from rapid development of tools to inform governance, to exploratory AI safety research which may be underexplored by industry.
- Facilitate information exchange, including by establishing – on a voluntary basis and subject to existing privacy and data regulation – clear information-sharing channels between the Institute and other national and international actors, such as policymakers, international partners, private companies, academia, civil society, and the broader public.
The goal of the Institute’s evaluations will not be to designate any particular AI system as “safe”; it is not clear that available techniques could justify such a definitive determination. The AI Safety Institute is not a regulator; its role is to develop the technical expertise to understand the capabilities and risks of AI systems, informing the government’s broader actions. Nevertheless, we expect progress in system evaluations to enable better informed decision making by governments and companies and act as an early warning system for some of the most concerning risks. If the AI Safety Institute identifies a potentially dangerous capability through its evaluation of advanced AI systems, the Institute will, where appropriate, address risks by engaging the developer on suitable safety mitigations and collaborating with the government’s established AI risk management and regulatory architecture.
The Institute is focused on the most advanced current AI capabilities and any future developments. It will consider open source systems as well as those deployed with various forms of access controls.
70. These voluntary actions allow us to test and learn what works in order to adapt our regulatory approach. We will strengthen our technical understanding to build wider consensus on key interventions, such as whether there should be conditions in which it would be right to pause the development of specific systems, as some have proposed.
71. While voluntary measures help us make AI safer now, the intense competition between companies to release ever-more-capable systems means we will need to remain highly vigilant to meaningful compliance, accountability, and effective risk mitigation. It may be the case that commercial incentives are not always aligned with the public good. If the market evolves such that there are a larger number of firms that are building highly capable systems, the governance of voluntary approaches will be much harder[footnote 82]. It will also be increasingly important to ensure the right accountability mechanisms and corporate governance frameworks are in place for companies building the most powerful systems.
5.2.3. The case for future binding measures
72. The section above highlights how the context-based approach may miss significant risks posed by highly capable general-purpose systems and leave the developers of those systems unaccountable. Whilst voluntary measures are a useful tool to address risks today, we anticipate that all jurisdictions will, in time, want to place targeted mandatory interventions on the design, development, and deployment of such systems to ensure risks are adequately addressed.
Foundation model supply chain
Foundation model supply chain.
Note: This is one possible model (there will not always be a separate or single company at each layer).
Image 2: A diagram of the foundation model supply chain taken from the Ada Lovelace Institute’s ‘Foundation Models Explainer’.
While there are many different ways to understand and describe the often complex life cycles of AI technologies, this diagram illustrates that our proposed future measures would be clearly targeted at the small number of companies that work in the foundation model developer layer building highly capable general-purpose AI.
73. Predicting which systems are capable enough to lead to significant risk is not straightforward. In line with our proportionate approach, any future regulation would be targeted at the small number of developers of the most powerful general-purpose systems. We propose to do this by establishing dynamic thresholds that can quickly respond to advances in AI development. Our preliminary analysis indicates that initial thresholds could be based on forecasts of capabilities using a combination of two proxies: compute (i.e. the amount of compute used to train the model) and capability benchmarking (i.e. assessing capabilities in certain risk areas to identify where we think high capabilities result in high risk). At least for the time being, the combination of these proxies can predict AI capabilities reasonably well, however there might need to be a range of thresholds.
74. Any new obligations would ensure that the developers of the in-scope systems adhere to the principles set out in the AI regulation white paper including safety, security, transparency, fairness, and accountability. This could include transparency measures (for example, relating to the data that systems are trained on); risk management, accountability, and corporate governance related obligations; or actions to address potential harms, such as those caused by misuse or unfair bias before or after training.
75. The open release of AI has, overall, been beneficial for innovation, transparency, and accountability. A degree of openness in AI is, and will continue to be, critical to scientific progress, and we recognise that openness is core to our society and culture. However, while we are committed to defending the value of openness, we note that there is a balance to strike as we seek to mitigate potential risks. In this regard, we see an emerging consensus on the need to explore pre-deployment capability testing and risk assessment for the most powerful AI systems, including where systems might be released openly. Pre-deployment testing could inform the deployment options available for a model and change the risk prevention steps required of organisations prior to the model’s release. Recognising the complexity of the debate, we are working closely with the open source community and AI developers to understand their needs. Our engagement with those developing and using AI models that are highly capable, general-purpose, and open access will allow us to explore the need for nuanced and targeted policy options that minimise any negative impacts on valuable open source activity, whilst mitigating risks.
76. The challenges posed by AI will ultimately require legislative action in every country once understanding of risk has matured. Introducing binding measures too soon, even if highly targeted, could fail to effectively address risks, quickly become out of date, or stifle innovation and prevent people from across the UK from benefiting from AI in line with the adaptable approach set out in the AI regulation white paper, the government would consider introducing binding measures if we determined that existing mitigations were no longer adequate and we had identified interventions that would mitigate risks in a targeted way. As with any decision to legislate, the government would only consider introducing legislation if we were not sufficiently confident that voluntary measures would be implemented effectively by all relevant parties and if we assessed that risks could not be effectively mitigated using existing legal powers. Finally, prior to legislating, the government would need to be confident that we could mandate measures in a way that would significantly mitigate risk without unduly dampening innovation and competition.
77. We know there is more work to do to refine our approach to regulating the most capable AI systems and the actors that design, develop, and deploy them. We look forward to developing our proposals by working closely with industry, academia, civil society, and the wider public. In Box 5, below, we set out the key questions that will guide our policy development.
Box 5: Key questions for policy development on the future regulation of highly capable general-purpose systems
Building on the evidence we received to our AI regulation white paper consultation on the topic of life cycle accountability and foundation models, over the coming months we will work closely with a range of experts and international partners to examine the questions below. We will publish findings from this engagement in a series of expert discussion papers. We will also publish the next iteration of our thinking and the steps we are taking in relation to the most capable AI systems.
-
Which specific risks should be addressed through future regulatory interventions targeted at highly capable AI systems? How do we ensure the regime is resilient to future developments?
-
When should the government and regulators intervene? Which systems should we be targeting? What would a compound threshold for intervention look like? Is compute a useful proxy for now, if thresholds remain dynamic? What about capability benchmarking?
-
Which obligations should be imposed on developers? Should the obligations be linked to our AI regulation principles? How do we ensure that the obligations are flexible but clear? At what stage could it be necessary to pause model development?
-
What, if any, new regulatory powers are required? How would this work alongside the existing regulatory landscape?
-
What should enforcement of any new regulation look like? What legal responsibilities should developers of in-scope systems have? Are updates to civil or criminal liability frameworks needed?
-
How do we provide regulatory certainty to drive responsible AI innovation while retaining an adaptable regime that can accommodate fast technical developments? How do we avoid creating barriers to market entry and scale-up?
-
Should certain capabilities trigger controls on open release? What would the negative consequences be? How should thresholds be set? What controls could be imposed?
-
What are the roles of existing transparency and accountability frameworks? How can strong transparency and good accountability be encouraged or assured to support responsible development of the most capable AI systems?
-
Should developers of highly capable AI systems be subject to specific corporate governance requirements? Is there a role for requirements on developers of highly capable AI systems to consider and mitigate risks to society or humanity at large?
-
How do potential new measures on highly capable AI systems link to wider life cycle accountability for AI? Are other actors in the AI value chain also hard for regulators to reach in a way that hampers our ability to address risk and support AI innovation and adoption?
78. As we set out in the AI regulation white paper, our intention is for our regulatory framework to apply to the whole of the UK subject to existing exemptions and derogations for unique operating requirements, such as defence and national security. However, we recognise that AI is used across a wide variety of sectors, some of which are reserved and some of which are devolved. As our policy develops and we consider the introduction of binding requirements on the developers of the most capable general-purpose systems, we will continue to assess any devolution impacts and need for extraterritorial reach.
79. We are committed to engaging the territorial offices and devolved administrations on both the design and delivery of the regulatory framework, so that businesses and citizens across the UK benefit from our regulatory approach.
5.3. Working with international partners to promote effective collaboration on AI governance
80. AI knows no borders and its impact will shape societies and economies in all corners of the world: AI developed in one nation will increasingly affect the lives of citizens living in others. Effective governance of AI will therefore require equally impactful international cooperation, which must build on the work of existing multilateral and multi-stakeholder fora and initiatives.
81. The UK is an established global leader in AI with a history of driving forward the international conversation and taking clear, decisive action to build bilateral and multilateral agreement. Our focus to date has been on collaborative action to support the development of AI in line with the context-based framework and principles set out in the AI regulation white paper[footnote 83]. This involves working alongside different groups of countries in accordance with need and acting in a targeted and proportionate manner. Our goal remains to work with others to build an international community that is able to realise the opportunities of AI on a global scale. We promote our values and collaborate where suitable to address the most pressing current and future AI-related risks. We carefully balance safety and innovation, acting alongside our partners to promote the international design, development, deployment, and use of the highest potential AI systems.
82. We will continue to act through bilateral partnerships and multilateral initiatives – including future AI Safety Summits – to promote safe, secure, and trustworthy AI, underpinned by effective international AI governance. Throughout this we will adopt a multistakeholder approach: We will collaborate with our international partners by working with representatives from industry, academia, civil society, and government to ensure we can reap the extraordinary benefits afforded by these technologies[footnote 84].
83. Working with these networks, we will unlock the opportunities presented by AI while addressing potential risks. In support of this, we maintain close relationships with our international partners across the full range of issues detailed in section 5.1, as well as on our respective emerging domestic approaches.
84. Domestic and international approaches must develop in tandem. In developing our own approach to AI regulation we will, therefore, both influence and respond to international developments. We will continue to proactively engage with the international landscape to ensure the appropriate degree of cooperation required for effective AI governance. We will achieve appropriate levels of coherence with other regulatory regimes, promote safety, and minimise potential barriers to trade – maximising opportunities for individuals and businesses across the UK and beyond. We will continue to work with our international partners to drive the development and adoption of tools for trustworthy AI, such as assurance techniques and global technical standards, in order to promote interoperability and avoid fragmentation.
85. We will continue to recognise the critical nature of safety in underpinning, but not supplanting, all other aspects of international AI collaboration. As the Prime Minister Rishi Sunak set out, our “vision, and our ultimate goal, should be to work towards a more international approach to safety”[footnote 85]. As noted above, the UK hosted the first ever AI Safety Summit in November 2023 and secured the Bletchley Declaration, a landmark agreement between 29 parties, including 28 countries from across the globe and the European Union[footnote 86]. The Declaration builds a shared understanding of the opportunities and risks that AI presents and the need for collaborative action to ensure the safety of the most powerful AI systems now and in the future. A number of countries and companies developing frontier AI also agreed to state-led testing of the next generation of systems, including through partnerships with newly announced AI Safety Institutes (see Box 4 for more detail)[footnote 87].
86. The pace of AI development shows no sign of slowing down, so the UK is committed to establishing enduring international collaboration on AI safety, building on the foundations of the AI Safety Summit agreements. To maintain this momentum and ensure that action is taken to secure AI safety, the Republic of Korea has agreed to co-host the next AI Safety Summit with the UK. France has agreed to host the following summit.
87. The UK’s AI Safety Institute represents one of our key contributions to international collaboration on AI. The Institute will partner with other countries to facilitate collaboration between governments on AI safety testing and governance, and develop their own capability. The Institute will facilitate international collaboration in three key ways:
-
Partnerships: the AI Safety Institute has agreed a partnership with the US AI Safety Institute and with the government of Singapore to collaborate on AI safety testing and is in regular dialogue on AI safety issues with international partners.
-
International Report on the Science of AI Safety[footnote 88]: The report was first unveiled as the State of the Science Report at the UK AI Safety Summit in November, where represented countries agreed to the development of an internationally authored report on the capabilities and risks of advanced AI. Rather than producing new material, it will summarise the best of existing research and identify priority research areas, providing a synthesis of the existing knowledge of risks from advanced AI.
-
Information Exchange: the AI Safety Institute’s evaluations and research are the first step in addressing the insight gaps between industry, governments, academia, and the public. This will ensure relevant parties, including international partners, receive the information they need to inform the development of shared protocols.
88. The UK also plays a proactive role through a range of multilateral initiatives to drive forward our ambition to promote the safe and responsible design, development, deployment, and use of AI. This includes:
-
G7: Working in cooperation with our partners in this forum, the UK has made significant progress to quickly respond to new technological developments and drive work on effective international AI governance. In December 2023, under Japan’s Presidency, G7 Leaders welcomed the Hiroshima AI Process Comprehensive Policy Framework that includes international guiding principles for all AI actors and a Code of Conduct for organisations developing advanced AI systems, as well as a work plan to further advance these outcomes[footnote 89]. We encourage AI actors, and especially AI developers, to further engage and support these outcomes. We look forward to collaborating further on AI under Italy’s G7 Presidency in 2024.
-
G20: In September 2023, as part of India’s G20 Presidency, the UK Prime Minister agreed to and endorsed the New Delhi Leaders’ Declaration alongside all other G20 Members[footnote 90]. The Declaration reaffirmed the UK’s commitment to the 2019 G20 AI Principles and emphasised the importance of a governance approach that balances the benefits and risks of AI and promotes responsible AI for achieving the UN Sustainable Development Goals[footnote 91]. The UK will work closely with Brazil on their AI ambitions as part of their 2024 G20 Presidency, which will centre on AI for inclusive sustainable development.
-
Global Partnership on AI (GPAI): The UK continues to actively shape GPAI’s multi-stakeholder project-based activities to guide the responsible development and use of AI grounded in human rights, inclusion, diversity, innovation, and economic growth. The UK was pleased to attend the December 2023 GPAI Summit in New Delhi, represented by the Minister for AI, Viscount Camrose, and to both endorse the GPAI New Delhi Ministerial Declaration[footnote 92] and host a side-event on outcomes and next steps following the AI Safety Summit. The UK has also begun a two-year mandate as a Steering Committee member and will work with India’s Chairmanship to ensure GPAI is reaching its full potential.
-
Council of Europe: The UK is continuing to work closely with like-minded nations on the proposed Council of Europe Convention on AI to help protect human rights, democracy, and rule of law. The Convention offers an opportunity to ensure these important values are codified internationally as one part of a wider approach to effective international governance.
-
Organisation for Economic Co-operation and Development (OECD): The UK is an active member of the Working Party on AI Governance (AIGO) and recognises the forum’s role in supporting the implementation of the OECD AI Principles and enabling the exchange of experience and best practice across member countries. In 2024, the UK will support the revision of the OECD AI Principles[footnote 93] and continue to provide case studies from the UK’s Portfolio of AI Assurance Techniques[footnote 94] to the OECD’s Catalogue of Tools and Metrics of Tools for Trustworthy AI[footnote 95].
-
United Nations (UN) and its associated agencies: Given the organisation’s unique role in convening a wide range of nations, the UK recognises the value of the UN-led discussions on AI and engages regularly to shape global norms on AI. In July 2023, the UK initiated and chaired the first UN Security Council briefing session on AI, and the Deputy Prime Minister chaired a session on frontier AI risks at UN High Level Week in September 2023. The UK continues to collaborate with a range of partners across UN AI initiatives, including negotiations for the Global Digital Compact, which aims to facilitate the Sustainable Development Goals through technologies such as AI, monitoring the implementation of the UNESCO Recommendation on the Ethics of AI[footnote 96], and engaging constructively at the International Telecommunication Union, which hosted the ‘AI for Good’ Summit in July 2023. The UK will also continue to work closely with the UN AI Advisory Body and is closely reviewing its interim report: Governing AI for Humanity[footnote 97].
-
Global Standards Development Organisations (SDOs): The UK is engaging directly with SDOs, such as the ISO and IEC, and is supporting developments in technical AI standards. The UK champions a global digital standards ecosystem that is open, transparent, and consensus-based. The UK also aims to support innovation and strengthen a multi-stakeholder, industry-led model for the development of technical AI standards, including through initiatives such as the UK’s AI Standards Hub[footnote 98]. We support UK stakeholders to participate in SDOs to both leverage the benefits of global technical standards here in the UK and deliver global digital technical standards shaped by democratic values.
89. Additionally, the UK is committed to ensuring that the benefits of AI are widely accessible. This includes working with international partners to fund safe and responsible AI projects for development around the world. As announced at the AI Safety Summit, the UK is contributing £38 million through its new AI for Development programme to support safe, responsible and inclusive AI innovation to accelerate progress on development challenges, focused initially in Africa[footnote 99]. This is part of an £80 million boost in AI programming to combat inequality and boost prosperity in Africa, with the UK working alongside Canada, the Bill and Melinda Gates Foundation, the USA, Google, Microsoft, and African partners, including Kenya, Nigeria, and Rwanda among others.
90. AI is now also fundamental to our bilateral relationships and, in some cases, it is suitable to build deeper and more committed bilateral partnerships alongside multilateral engagement to further our shared interests. We have therefore pursued bilateral agreements on areas including responsibly developing and deploying AI with key international partners, to build the foundation for further collaboration on AI governance. For example, as part of the DSIT International Science Partnerships Fund[footnote 100], UKRI will invest £9 million to bring together researchers and innovators in bilateral research partnerships with the US. These partnerships will focus on developing safer, responsible, and trustworthy AI as well as AI for scientific uses. Since the publication of the AI regulation white paper in March 2023 we have signed:
-
The Atlantic Declaration with the US[footnote 101]: which develops our strong partnership on AI, underpinned by our shared democratic values and our ambition to promote safe and responsible AI innovation across the world. Work under the 2023 Atlantic Declaration will ensure that our unique alliance is reinforced for the challenges of new technological developments.
-
The Hiroshima Accord with Japan[footnote 102]: which commits to focus on promoting human-centric and trustworthy AI and interoperability between our AI governance frameworks.
-
The Downing Street Accord with the Republic of Korea[footnote 103]: which builds on the progress achieved on safe, responsible AI development, including at the AI Safety Summit – the next edition of which will be co-hosted by the Republic of Korea and the UK.
-
The Joint Declaration on a Strategic Partnership with Singapore[footnote 104]: which harnesses expertise in new technologies such as AI from the UK and Singapore. DSIT also signed a Memorandum of Understanding (MoU) on Emerging Technologies in June 2023 with Singapore’s Infocomm Media Development Authority (IMDA). In this MoU, both parties agreed to collaborate on AI governance and to facilitate the development of effective and interoperable AI assurance mechanisms.
91. We have a number of other important bilateral relationships on AI with countries across the world and we intend, where suitable, to further build such agreements to strengthen these partnerships, such as through bilateral MoUs and Free Trade Agreements.
92. Only through effective global collaboration will the UK and our partners worldwide unlock the opportunities and mitigate the associated risks of AI. We will continue to engage our international partners to support responsible AI innovation that effectively and proportionately addresses potential AI harms and aligns with the principles established in the AI regulation white paper. We will also work together to promote coherence between our AI governance frameworks to ensure that businesses can operate effectively in both the UK and wider global markets and to ensure that AI developments benefit people around the world.
5.4. An AI regulation roadmap of our next steps
93. In 2024, we will:
-
Continue to develop our domestic policy position on AI regulation by:
-
Engaging with a range of experts on interventions for highly capable AI systems, including questions on open release, in the summer.
-
Publishing an update on our work on new responsibilities for developers of highly capable general-purpose AI systems by the end of the year.
-
Collaborating across government and with regulators to analyse and review potential gaps in existing regulatory powers and remits on an ongoing basis.
-
Working closely with the AI Safety Institute, which will provide foundational insights to our central AI risk assessment activities and inform our approach to AI regulation, on an ongoing basis. The AI Safety Institute will ensure that the UK takes an evidence-based, proportionate response to regulating the risks of AI.
-
-
Progress action to promote AI opportunities and tackle AI risks by:
-
Conducting targeted engagement on our cross-economy AI risk register and plan to assess the regulatory framework from the spring onwards.
-
Releasing a call for views in spring to obtain further input on our next steps in securing AI models, including a potential Code of Practice for cyber security of AI, based on NCSC’s guidelines.
-
Establishing a new international dialogue to defend democracy and address shared risks related to electoral interference ahead of the next AI Safety Summit.
-
Launching a call for evidence on AI-related risks to trust in information and related issues such as deepfakes.
-
Exploring mechanisms for providing greater transparency, including measures so that rights holders can better understand whether content they produce is used as an input into AI models.
-
Phasing in the mandatory requirement for central government departments to use the Algorithmic Transparency Recording Standard (ATRS) over the course of the year.
-
-
Build out the central function and support regulators by:
-
Launching a new £10 million programme to support regulators to identify and understand risks in their domain and to develop their skills and approaches to AI.
-
Establishing a steering committee to support and guide the activities of a formal regulator coordination structure within government in the spring.
-
Asking key regulators to publish updates on their strategic approach to AI by 30 April.
-
Collaborating with regulators to iterate and expand our initial cross-sectoral guidance on implementing the principles, with further updates planned by summer.
-
-
Encourage effective AI adoption and provide support for industry, innovators, and employees by:
-
Launching the pilot AI and Digital Hub with the DRCF in the spring.
-
Publishing an Introduction to AI Assurance in spring.
-
Publishing updated guidance on the use of AI within HR and recruitment in spring.
-
Publishing a full AI skills framework that incorporates feedback to our consultation and supports employers, employees, and training providers to identify upskilling routes for AI in spring.
-
Launching the AI Management Essentials scheme to set a minimum good practice standard for companies selling AI products and services by the end of the year.
-
Publishing an update on our emerging processes guide by the end of the year.
-
-
Support international collaboration on AI governance by:
-
Actioning our newly announced £9 million partnership with the US on responsible AI as part of the DSIT International Science Partnerships Fund.
-
Publishing the first iteration of the International Report on the Science of AI Safety in spring.
-
Sharing new knowledge with international partners through the AI Safety Institute on an ongoing basis.
-
Supporting the Republic of Korea and France on the next AI Safety Summits on an ongoing basis, and considering the possible role of AI Safety Summits beyond these.
-
Continuing bilateral and multilateral partnerships on AI, including the G7, G20, Council of Europe, OECD, United Nations, and GPAI, on an ongoing basis.
-
6. Summary of consultation evidence and government response
94. This chapter provides a summary of the written evidence we received in response to our consultation followed by the government response. This chapter is structured by the 10 categories that we used to group our 33 consultation questions:
- The revised cross-sectoral AI principles.
- A statutory duty to regard.
- New central functions to support the framework.
- Monitoring and evaluation of the framework.
- Regulator capabilities.
- Tools for trustworthy AI.
- Final thoughts.
- Legal responsibility for AI.
- Foundation models and the regulatory framework.
- AI sandboxes and testbeds.
95. In total, we received 409 written consultation responses from organisations and individuals. Annex A provides an overview of who we received responses from and outlines our method of analysis. We also proactively engaged with 364 individuals through roundtables, technical workshops, bilaterals, and a programme of ongoing regulator engagement. While we weave insights from this engagement throughout our analysis, Annex A provides a detailed overview of our engagement findings.
6.1. The revised cross-sectoral AI principles
1. Do you agree that requiring organisations to make it clear when they are using AI would improve transparency?
2. Are there other measures we could require of organisations to improve transparency for AI?
3. Do you agree that current routes to contest or get redress for AI-related harms are adequate?
4. How could current routes to contest or seek redress for AI-related harms be improved, if at all?
5. Do you agree that, when implemented effectively, the revised cross-sectoral principles will cover the risks posed by AI technologies?
6. What, if anything, is missing from the revised principles?
Summary of questions 1-6:
96. Over half of respondents agreed that, when implemented effectively, the revised principles would cover the key risks posed by AI technologies. The revised principles included safety, security and robustness; appropriate transparency and explainability; fairness; accountability and governance; and contestability and redress. However, respondents also advocated for the explicit inclusion of human rights, operational resilience, data quality, international alignment, systemic risks and wider societal impacts, sustainability, and education and literacy.
97. Respondents wanted to see further detail on the implementation of the principles, regulator capability, and interactions with existing law. Respondents consistently stressed the fast pace of technological change and reflected that the framework should be adaptable and supported by monitoring and evaluation. Some respondents were concerned that the principles would not be sufficiently enforceable, citing a lack of statutory backing.
98. There was strong support for a range of transparency measures from respondents. Respondents emphasised that transparency was key to building public trust, accountability, and an effective and verifiable regulatory framework. A majority of respondents agreed that a requirement for organisations to make it clear when they are using AI would improve transparency. Those who disagreed felt that labelling AI use would be either insufficient or disproportionately burdensome. Respondents suggested a range of transparency measures including the public disclosure of inputs like compute and data; labelling AI use and outputs; opt-ins and human alternatives to automated processing; explanations for AI outcomes, impacts and limitations; public or organisational AI registers; disclosure of model details to regulators; and independent assurance tools including audits and technical standards.
99. Most respondents reported that current routes to contest or seek redress for AI-related harms through existing legal frameworks are not adequate. Respondents noted that it can be difficult to identify AI-related harms and the high costs of litigation often prevents individuals from seeking redress. Many respondents wanted to see the government clarify the legal rights and responsibilities relating to AI, with many suggesting doing so through regulatory guidance. Some endorsed the introduction of statutory requirements. Respondents recommended establishing accessible redress routes, with some advocating for a central, cross-sector redress mechanism such as a dedicated AI ombudsman. Respondents also noted that international agreements would be needed to ensure effective routes to contest or seek redress for AI-related harms across borders. Respondents emphasised that better AI transparency would help make redress more accessible across a broad range of potential harms, including intellectual property infringement.
Response:
100. The government wants to ensure that the UK maintains its position as a global leader in AI. This means promoting safe, responsible innovation to ensure that we maximise the benefits AI can bring across the country. Our cross-sectoral principles set out our expectations for the responsible design, development, and application of AI to help guide businesses and organisations building and using these technologies. We are encouraged to see that most respondents agree that the revised cross-sectoral principles will cover the risks posed by AI when implemented effectively.
101. We expect regulators to apply the principles within their existing remits and in line with our existing laws and values, respecting the UK’s long history of democracy, strong rule of law, and commitments to human rights and environmental sustainability. As aspects of these values and rules are enshrined in the law that regulators are bound to follow, we do not think it is necessary to include democracy, human rights, the rule of law, or sustainability specifically within the principles themselves. The guidance we are publishing alongside this consultation response will support regulators to implement the principles within their respective domains.
102. The principles already cover issues raised by respondents linked to both operational resilience (safety, security, and robustness) and data protection (transparency, fairness, and accountability). We expect all actors across the AI life cycle to adhere to existing legal frameworks, including data protection law. The UK’s existing data protection legislation (UK GDPR and the Data Protection Act 2018) regulates the development of AI systems and other technologies where personal data is involved. The Data Protection and Digital Information Bill will clarify the rights of data subjects to specific safeguards when subject to solely automated decisions that have significant effects on them. Furthermore, the Information Commissioner’s Office (ICO) has created specific guidance on how to use data for AI in compliance with data protection law. Beyond the scope of data protection law[footnote 105]. Beyond the scope of data protection law, the government is assessing a range of possible interventions aligned with the principles as part of our work to encourage the responsible and safe development of highly capable AI. For example, we are exploring if and how to introduce targeted measures on developers of highly capable general-purpose AI systems related to transparency requirements (for example, on training data), risk management, and accountability and corporate governance related obligations. Similarly, our central risk assessment activities will identify and monitor a range of risks, providing cross-economy oversight that will capture systemic risks and wider societal impacts.
103. We acknowledge the broad support for transparency and we will continue our work assessing whether and which measures provide the most meaningful transparency for AI end users and actors across the AI life cycle. It is important that we take an evidence-based approach to transparency. The Algorithmic Transparency Recording Standard (ATRS) is a practical mechanism for transparency that was developed through public engagement and has been piloted across the UK[footnote 106]. The ATRS helps public sector organisations provide clear information about algorithmic tools they use in decision-making. As mentioned in section 5.1, we will now be making use of the ATRS a requirement for all government departments and plan to expand this across the broader public sector over time. While measures like watermarking can help users identify AI generated content, we need to ensure that proposed interventions are robust, cannot be easily overridden, and achieve positive outcomes. To establish greater transparency on AI outputs, we published an “Emerging processes for frontier AI safety” document that outlines three areas of practice related to identifying AI generated content, including research techniques, watermarking, and AI output databases[footnote 107]. As mentioned in section 5.2.2, we will update this guide by the end of the year and continue to encourage AI companies to develop best practices.
104. Our expert regulators are already using their existing remits to implement the AI principles, including the contestability and redress principle which includes expectations about clarifying existing routes to redress. We recognise the link between the fair and effective allocation of liability throughout the AI life cycle and the availability and clarity of routes to redress. Our work to explore existing liability frameworks and accountability through the value chain is ongoing and includes analysis of the existence of redress mechanisms. As a first step towards ensuring fair and effective allocation of accountability and liability, the government is considering introducing targeted binding requirements on developers of highly capable general-purpose AI systems which may involve creating or allocating new regulatory powers.
6.2. A statutory duty to regard
7. Do you agree that introducing a statutory duty on regulators to have due regard to the principles would clarify and strengthen regulators’ mandates to implement our principles, while retaining a flexible approach to implementation?
8. Is there an alternative statutory intervention that would be more effective?
Summary of questions 7-8:
105. Most respondents somewhat or strongly agreed that introducing a statutory duty on regulators to have due regard to the principles set out in the AI regulation white paper would clarify and strengthen regulators’ mandates to implement the principles while retaining a flexible approach to implementation. However, nearly a quarter noted that regulators would need enhanced resources and capabilities in order to enact a statutory duty effectively.
106. Around a third of respondents argued that additional, targeted statutory measures would be necessary to effectively implement the regulatory framework. Many suggested expanding regulator powers, noting that the existing statutory remits of some regulators would limit their ability to implement the framework. In particular, respondents raised the need to review and potentially expand the investigatory powers and capabilities of regulators in regard to AI.
107. Some advocated for wider, horizontal statutory measures such as specific AI legislation, a new AI regulator, and strict rules about the use of AI in certain contexts.
108. Other respondents felt that, if rushed, the implementation of a duty to regard could disrupt regulation, innovation, and trust. These respondents recommended that the duty should be reviewed after a period of non-statutory implementation, particularly to observe interactions with existing law and regulatory remits. Some respondents 1. noted that the end goal and timeframes for the AI regulatory framework were not clear, causing uncertainty.
Response:
109. We are encouraged that respondents to this question are enthusiastic about the proper and effective implementation of our cross-sectoral AI principles. We welcome the broad support for a statutory duty on regulators, recognising that respondents also gave conditions and alternatives that could be used to implement the framework effectively. As set out in the AI regulation white paper, we anticipate introducing a statutory duty on regulators requiring them to have due regard to the principles after reviewing an initial period of non-statutory implementation.
110. We acknowledge concerns from respondents that rushing the implementation of a duty to regard could cause disruption to responsible AI innovation. We will not rush to legislate but will evaluate whether it is necessary and effective to introduce a statutory duty to have due regard to the principles on regulators. We currently think that a non-statutory approach offers critical adaptability but we will keep this under review, for example by assessing the updates on strategic approaches to AI that the government has asked a number of regulators to publish by 30 April 2024. We will also work with government departments and regulators to analyse and review potential gaps in existing regulatory powers and remits.
111. We are pleased to see that many regulators are taking proactive steps to address AI and implement the principles within their remits. This includes work by the Competition and Markets Authority (CMA), Advertising Standards Authority (ASA), and Office of Communications (Ofcom)[footnote 108]. Others are progressing their existing plans in ways that align with these principles, such as the ICO and Medicines and Healthcare products Regulatory Agency (MHRA)[footnote 109].
112. We continue to work closely with regulators to develop the framework, ensure coherent implementation, and build regulator capability. To support a coherent approach across sectors, we are publishing initial guidance to regulators alongside this response on how to apply the cross-sectoral AI principles within their existing remits. We will update this guidance over time to ensure that it reflects developments in our regime and technological advances in AI. We will establish a steering committee by spring 2024 to support and guide the activity of the central regulator coordination function (see section 5.1.2 for details).
113. We note respondents’ concerns across the consultation that any new rules for AI should not contradict or duplicate existing laws. We will continue to evaluate any potential gaps or frictions within the existing statutory remits of regulators and current legislative frameworks. In the white paper, we said that we would keep the wider AI landscape under review in order to inform future iterations of the regulatory framework, including whether further interventions on foundation models may be required. We will consult on our plan for monitoring and evaluating the regulatory framework in 2024 (see our response to questions on monitoring and evaluation in section 6.4 for more detail).
6.3. New central functions to support the framework
9. Do you agree that the functions outlined in section 3.3.1 would benefit our AI regulation framework if delivered centrally?
10. What, if anything, is missing from the central functions?
11. Do you know of any existing organisations who should deliver one or more of our proposed central functions?
12. Are there additional activities that would help businesses confidently innovate and use AI technologies?
12.1. If so, should these activities be delivered by government, regulators, or a different organisation?
13. Are there additional activities that would help individuals and consumers confidently use AI technologies?
13.1. If so, should these activities be delivered by government, regulators, or a different organisation?
14. How can we avoid overlapping, duplicative, or contradictory guidance on AI issued by different regulators?
Summary of questions 9-14:
114. Nearly all respondents agreed that delivering the proposed functions centrally would benefit the AI regulation framework, with many praising the approach for ensuring that the government can monitor and iterate the framework.
115. While respondents widely supported the proposed central functions, many wanted more detail on each function and its activities. Some respondents felt there should be a greater emphasis on partnerships and collaboration to deliver the activities. Respondents also wanted more detail on international collaboration. Some suggested that the government should prioritise building the central risk function. Of these responses, a few noted that more consideration should be given to ethical and societal risks.
116. Respondents emphasised that the regulatory functions should build from the existing strengths of the UK’s regulatory landscape, with approximately a third identifying regulators as organisations who should deliver one or more central functions. Overall, respondents emphasised that effective delivery would require collaboration between government, regulators, industry, civil society, academia, and the general public. Over a quarter of respondents felt that technology-focused research institutes and think tanks could help deliver the central functions.
117. Respondents suggested a range of additional activities that government and regulators could offer to support industry. Around a third of respondents felt that training products and educational resources would help organisations to apply the principles to everyday business practices. Nearly a quarter suggested that regulators should produce guidance to allow businesses to innovate confidently. Some noted the importance of internationally interoperable frameworks for AI regulation to ensure a low compliance burden on organisations building, selling, and using AI technologies. Respondents also argued that more work is needed to ensure that businesses have access to high-quality, diverse, and ethically-sourced data to support their AI innovation efforts.
118. When thinking about additional activities for individuals and consumers, respondents prioritised transparency from the cross-sectoral principles, with nearly half arguing that individuals and consumers should be able to identify when and how AI is being used by a service or organisation. More than a third of respondents felt that education and training would enable consumers to use AI products and services safely and more effectively.
119. Around a third suggested that the proposed central functions would be the most effective mechanism to avoid overlapping, duplicative, or contradictory guidance.
Response:
120. We welcome the strong support for the central functions proposed in the AI regulation white paper to coordinate, monitor, and adapt the AI framework. Together, these functions will provide clarity, ensure the framework works as intended, and future-proof the UK’s regulatory approach. That is why we have already started to establish the central function within government to undertake the activities proposed in the white paper (see section 5.1.2 for details).
121. We note respondents’ concerns around the potential risks posed by the rapid developments in AI technology. We have already established the risk monitoring and assessment activities of the central function within DSIT, reflecting the strong recommendation from respondents to operationalise cross-economy AI risk management as a priority. Our centralised risk assessment activities will identify, measure, and monitor existing and emerging AI risks using expertise from across government, industry, and academia, including the AI Safety Institute. This will allow us to monitor risks holistically and identify any potential gaps in our approach. Horizon scanning will extend our central risk assessment activities, monitoring emerging AI trends and opportunities to maximise benefits while taking a proportionate approach to AI risks. This year, we will conduct targeted engagement on our cross-economy AI risk register.
122. Reflecting respondents’ views that the proposed central function will help regulators avoid producing overlapping, duplicative, or contradictory guidance, we are developing a coordination function to support regulators to interpret and apply the principles within their remits (see section 5.1.2 for detail). As part of this, we will establish a steering committee in the spring with government representatives and key regulators to support knowledge exchange and coordination on AI governance. To further support regulators and ensure that the UK’s strength in AI research is fully utilised in our regulatory framework, we have also announced a £10 million package to support regulator AI capabilities and a new commitment by UK Research and Innovation (UKRI) to improve links between regulators and the skills, expertise, and activities supported by their future investments in AI research.
123. To ensure appropriate levels of cohesion with emerging approaches to AI regulation in other jurisdictions, we will continue to work with international partners on regulatory interoperability, including technical standards and assurance techniques, to make it easier for UK companies to attract overseas investment and trade internationally. For more detail, see section 5.3 and our response to questions on tools for trustworthy AI in 6.6.
124. Alongside this, we have announced a new pilot regulatory service to be hosted by the Digital Regulation Cooperation Forum (DRCF) to make it easier for AI and digital innovators to navigate the regulatory landscape (see our response to questions on AI sandboxes for more detail: section 6.10).
125. We remain committed to the iterative approach set out in the white paper, anticipating that our framework will need to evolve as new risks or regulatory gaps emerge. Our monitoring and evaluation activities will assess if, when, and how we make changes to our framework, gathering evidence from a wide range of sources. We provide more detail in our response to questions on monitoring and evaluation in section 6.4.
126. We are encouraged that respondents endorsed a wide range of organisations in the UK as useful partners to deliver the proposed centralised activities. As we said in the white paper, the government will deliver the central function initially, working in partnership with regulators and other key actors in the AI ecosystem. The government’s primary role will be to leverage existing activities where possible and ensure that all the necessary activities to promote responsible AI innovation are taking place.
6.4. Monitoring and evaluation of the framework
15. Do you agree with our overall approach to monitoring and evaluation?
16. What is the best way to measure the impact of our framework?
17. Do you agree that our approach strikes the right balance between supporting AI innovation; addressing known, prioritised risks; and future-proofing the AI regulation framework?
Summary of questions 15-17:
127. A majority of respondents agreed with the overall approach to monitoring and evaluation, commending the proposed feedback loop with industry and civil society as a means to gain insights about the effectiveness of the framework.
128. Just over a quarter of respondents emphasised that engaging with a diverse range of stakeholders would create the most valuable insights. Many advocated for the inclusion of wider civil society and consumer representatives to ensure that voices outside of the tech industry are heard, as well as regular engagement with industry and research experts. Respondents also stressed that international engagement would be key to effectively harmonise approaches across jurisdictions.
129. Respondents wanted to see more detail on the practicalities of the monitoring and evaluation framework, including how data will be collected and used to measure success. Nearly a third of respondents suggested the impact of the framework should be measured through a range of data sources, and recommended collecting data on key indicators as well as using impact assessments.
130. Half of respondents agreed that the approach appears to strike the right balance between supporting AI innovation; addressing known, prioritised risks; and future-proofing the AI regulation framework. However, some respondents disagreed and argued that the approach prioritised AI innovation and economic growth over safety and the mitigation of AI-related risks.
Response:
131. We are pleased to note the positive feedback on our proposed approach to the monitoring and evaluation of the framework. Monitoring and evaluation activities will allow us to review the implementation of the AI regulation framework across the economy and is at the heart of our iterative approach. It will ensure that the regime is working as intended: actively responding to prioritised risks, supporting innovation, and maximising the benefits of AI across the UK. We agree with respondents that, as we implement the framework set out in the AI regulation white paper, monitoring and evaluation will allow the government to spot potential issues and adapt the framework in response if needed.
132. We acknowledge growing concerns that we may face more safety risks related to AI as these technologies are increasingly used. We recognise that many of these concerns focus on the advanced capabilities of the most powerful AI systems. That is why we remain committed to an adaptable approach that will evolve as new risks or regulatory gaps emerge. Our initial thinking on potential new measures targeted at the developers of highly capable general-purpose AI models is presented in section 5.2. The AI Safety Institute will advance AI safety capabilities for the public interest, allowing the government to respond to the cutting-edge of technological development. Our monitoring and evaluation will build on work by the Institute, our cross-sectoral risk assessment, and feedback from stakeholders to understand how the regulatory framework is performing. Our evaluation will consider whether the framework is effectively achieving the objectives set out in the white paper, including building public trust by addressing potential risks appropriately.
133. We note the emphasis from respondents on using the right data, metrics, and sources to evaluate how well the regulatory framework is performing. We agree that it is key to the effectiveness of the framework to get the measures of success right, and we are actively working on this as we develop our monitoring and evaluation framework for publication. We will conduct a targeted consultation on our proposed plan to assess the framework with a range of stakeholders in spring. As part of this, we will seek detailed views on our proposed metrics and data sources.
6.5. Regulator capabilities
18. Do you agree that regulators are best placed to apply the principles and government is best placed to provide oversight and deliver central functions?
19. As a regulator, what support would you need in order to apply the principles in a proportionate and pro-innovation way?
20. Do you agree that a pooled team of AI experts would be the most effective way to address capability gaps and help regulators apply the principles?
Summary of questions 18-20:
134. Nearly all respondents agreed that regulators are best placed to lead the implementation of the principles, and that the government is best placed to provide oversight and delivery of the central functions. However, respondents argued that the government would need to improve regulator capability in order for this approach to be effective. Some respondents were concerned at the lack of a specific body to support the implementation and oversight of the proposed framework, with some asking for AI legislation and a new AI regulator.
135. While regulators are broadly supportive of the proposed approach, over a quarter of those that responded to Q19 suggested that increased AI expertise would help them effectively apply the principles within their existing remits. Overall, regulators reported different levels of technical expertise and AI capability. Some felt that greater organisational capacity and additional resources would help them undertake new responsibilities related to AI and understand where and how AI is used in their domains.
136. Regulators also noted that AI presents coordination challenges across domains and sectors, with some emerging risks related to AI not falling clearly within a specific existing remit. Just over a quarter of regulators that responded to Q19 emphasised that close collaboration between regulators and the proposed central functions would help build meaningful sector-specific requirements and prevent duplication.
137. A majority of respondents agreed that a pooled team of AI experts would be the most effective way to address the different levels of capability across the regulatory landscape. Respondents advocated for a diverse and multi-disciplinary pool to bring together technical AI expertise with sector-specific regulatory knowledge, industry specialists, and civil society. Respondents argued that this would ensure that regulators are considering a broad range of perspectives in their application of the cross-sectoral AI principles.
Response:
138. We are encouraged that respondents broadly agree with the proposed regulator-led approach for the implementation of the principles, with the government providing oversight and delivering the central function. As outlined in the AI regulation white paper, our existing expert regulators are best placed to conduct detailed risk analysis and enforcement activities within their areas of expertise. We will continue to work closely with regulators to ensure that potential risks posed by AI are sufficiently covered by our rule of law. In keeping with our iterative approach, we will seek to adapt the framework, including the regulatory architecture, if analysis proves this is necessary and effective.
139. As pointed out by respondents across the consultation, to regulate AI effectively our regulators must have the right skills, tools, and expertise. To support regulator’s ability to adapt and respond to the risks and opportunities that AI presents in their domains, we are today announcing a £10 million investment to build technical upskilling. We will work closely with regulators to identify the most promising opportunities to leverage this funding, including designing a delivery model that can achieve the intended objectives more effectively than the central pool of expertise proposed in the AI regulation white paper. In particular, regulator feedback has shown that we need to support them to develop tools and skills within their specific domains – albeit working collaboratively where appropriate – and deliver support that aligns with and supports their independence. As capability and resource varies across regulators, our intention is that this fund will particularly enable those regulators with less mature AI expertise to conduct research and uncover foundational insights to develop or adapt practical tools to ensure compliance in an AI-enabled future.
140. Further, as set out in the response to Professor Dame Angela McLean’s cross-cutting review of pro-innovation regulation of technologies[footnote 110], the government is also exploring how to further support regulators to develop the specialist skills necessary to regulate emerging technologies, including increased flexibility on pay and conditions. This builds on schemes already in place to support secondments between government departments, regulators, academia, and industry.
141. We acknowledge regulator’s concerns that AI can pose coordination challenges. In the white paper we proposed a number of centralised activities to support regulators and ensure that the regulatory landscape for AI is consistent and cohesive. To facilitate cross-cutting collaboration and ensure that the overall regulatory framework functions as intended, we are developing our regulatory coordination activities. These coordination activities will sit in our central function in government alongside our AI risk assessment activities (see more detail in section 5.1.2). To support a coherent approach across sectors, we are also publishing initial guidance to regulators alongside this response on how to apply the cross-sectoral AI principles within their existing remits.
142. We note respondents’ emphasis on transparency and the need for industry and civil society to have visibility of the AI regulation framework. We agree that establishing feedback loops with industry, academia and civil society will be key to measuring the effectiveness of the framework. Our central function will engage stakeholders to ensure that a wide range of voices are heard and considered: providing clarity, building trust, ensuring interoperability, and informing the government of the need to adapt the framework.
6.6. Tools for trustworthy AI
21. Which non-regulatory tools for trustworthy AI would most help organisations to embed the AI regulation principles into existing business processes?
Summary of question 21:
143. There was strong support for the use of technical standards and assurance techniques, with some respondents agreeing that both would help organisations to embed the AI principles into existing business processes. Many respondents praised the UK AI Standards Hub and the Centre for Data Ethics and Innovation’s (CDEI) work on AI assurance. While some respondents noted that businesses would have a smaller compliance burden if tools and processes were consistent across sectors, others noted the importance of additional sector-specific tools and processes. Respondents also suggested supplementing technical standards with case studies and examples of good practice.
144. Respondents argued that standardised tools and techniques for identifying and mitigating potential risks related to AI would also support organisations to embed the AI principles. Some identified assurance techniques such as impact and risk assessments, model performance monitoring, model uncertainty evaluations, and red teaming as particularly helpful for identifying AI risks. A few respondents recommended assurance techniques that can be used to detect and prevent issues such as drift to mitigate risks related to data. While commending the role of tools for trustworthy AI, a small number of respondents also expressed a desire for more stringent regulatory measures, such as statutory requirements for high risk applications of AI or a watchdog for foundation models.
145. Respondents felt that tools and techniques such as fairness metrics, transparency reports, and organisational AI ethics guidelines can support the responsible use of AI while growing public trust in the technology. Respondents expressed the desire for third-party verification of AI models through bias audits, consumer labelling schemes, and external certification against technical standards.
146. A few respondents noted the benefits of international harmonisation across AI governance approaches for both organisations and consumers. Some endorsed interoperable technical standards for AI, commending global standards development organisations (SDOs) such as the International Organization for Standardization (ISO) and Institute of Electrical and Electronics Engineers (IEEE). Others noted the strength of a range of international work on AI including that by individual countries, such as the USA’s National Institute of Standards and Technology (NIST) AI Risk Management Framework (RMF) and Singapore’s AI Verify Foundation, along with work on international governance by multilateral bodies such as the Organisation for Economic Co-operation and Development (OECD), United Nations (UN), and G7.
Response:
147. We are pleased to see such strong support for the continued development and adoption of technical standards and assurance techniques for AI. These tools will help organisations put our proposed regulatory principles into practice, innovate responsibly, and build public confidence. We recognise that, in some instances, it will be important to have assurance techniques and technical standards that are specific to a particular context, application, or sector. That is why, in the AI regulation white paper, we set out a layered approach to technical standards, encouraging regulators to build on widely applicable sector-agnostic tools where appropriate[footnote 111].
148. We welcome praise for the UK AI Standards Hub and CDEI. Launched in October 2022, the Hub brings together the UK’s technical expertise on AI standards, including the Alan Turing Institute, British Standards Institution, and National Physical Laboratory, to provide training and information on the complex international AI standards landscape. The CDEI published a Portfolio of AI Assurance Techniques in June 2023 with examples from the real world to support the development of trustworthy AI, which respondents indicated would be helpful[footnote 112]. The Portfolio is also part of the OECD’s Catalogue of Tools and Metrics for Trustworthy AI, which shares the CDEI case-studies to an international audience. The CDEI also launched the “Fairness Innovation Challenge” in October to support the development of new socio-technical solutions to address bias and discrimination in AI systems[footnote 113]. Today we are announcing that the Centre for Data Ethics and Innovation (CDEI) is changing its name to the Responsible Technology Adoption Unit to more accurately reflect its role within the Department for Science, Innovation and Technology (DSIT) to develop tools and techniques that enable responsible adoption of AI in the private and public sectors. This year, DSIT will publish an “Introduction to AI assurance” to further promote the value of AI assurance.
149. We note that respondents would like to see more standardised tools and techniques to identify and manage AI risk. Ahead of the AI Safety Summit in November 2023, we published “Emerging processes for frontier AI safety” to help prompt a debate about good safety processes for advanced AI systems look like[footnote 114]. The document provides a snapshot of promising ideas, emerging processes, and associated practices in AI safety. It is intended as a point of reference to inform the development of frontier AI organisations’ safety policies as well as a companion for readers of these policies. It outlines early thinking on practices for innovation in frontier AI development, including model evaluations and red teaming, responsible capability scaling, and model reporting and information sharing. In 2024, we will encourage AI companies to develop their AI safety and responsible capability scaling policies. As part of this work, we will update our emerging processes guide by the end of the year. More widely, we note the development of relevant global technical standards which provide guidance on risk management related to AI. For example, standard ISO 42001 will help organisations manage their AI systems in a trustworthy way.
150. In the white paper, we note that responding to risk and building public trust are key drivers for regulation. We therefore understand respondents’ emphasis on tools for building public trust as a key way to ensure responsible AI innovation. The Responsible Technology Adoption Unit (formerly CDEI) within DSIT has a specialist Public Insights team that regularly engages with the general public and affected communities to build a deep understanding of public attitudes towards AI[footnote 115]. These insights are used by DSIT and wider government to align our regulatory approaches to AI with public values and foster trust in these technologies. DSIT and the Central Digital and Data Office (CDDO) have also developed the ATRS to help public sector organisations provide clear information about algorithmic tools they use to support decisions[footnote 116]. Following a successful pilot of the standard, and publication of an approved cross-government version last year, we will now be making use of the ATRS a requirement for all government departments and plan to expand this across the broader public sector over time.
151. We agree with respondents that international cooperation on AI governance will be key to successfully mitigating AI-related risks and building public trust in AI. The first ever AI Safety Summit convened a group of representatives from around the globe to set a new path for collective international action to navigate the opportunities and risks of frontier AI. We also continue to collaborate internationally on AI governance, both bilaterally and through several multilateral fora. For example, the UK plays an important role in AI discussions at the UN, Council of Europe, OECD, G7, Global Partnership on AI (GPAI), and G20. Notably, the UK worked closely with G7 partners in negotiating the Codes of Conduct and Guiding Principles for the development of advanced AI systems, as part of the Hiroshima AI Process. The UK fully supports developing AI policy and technical standards in a globally inclusive, multi-stakeholder, open, and consensus-based way. We support UK stakeholders to participate in Standards Development Organisations (SDOs) to both leverage the benefits of global technical standards here in the UK and deliver global digital technical standards shaped by democratic values.
6.7. Final thoughts
22. Do you have any other thoughts on our overall approach? Please include any missed opportunities, flaws, and gaps in our framework.
Summary of question 22:
152. Some respondents felt that the AI regulation framework set out in the white paper would benefit from more detailed guidance on AI-related risks. Some wanted to see more stringent measures for severe risks, particularly related to the use of AI in safety-critical contexts. Respondents suggested that the framework would be clearer if the government provided risk categories for certain uses of AI such as law enforcement and places of work. Other respondents stressed that AI can pose or accelerate significant risks related to privacy and data protection breaches, cyberattacks, electoral interference, misinformation, human rights infringements, environmental sustainability, and competition issues. A few respondents were concerned about the potential existential risk posed by AI. Many respondents felt that AI technologies are developing faster than regulatory processes.
153. Some respondents argued that the success of the framework relies on sufficient coordination between regulators in order to provide a clear and consistent approach to AI across sectors and markets. Respondents also noted that different sectors face particular AI-related benefits and risks, suggesting that the framework would need to balance the consistency provided by cross-sector requirements with the accuracy of sector-specific approaches. In particular, respondents flagged that any new rules or bodies to regulate AI should build from the existing statutory remits of regulators and relevant regulatory standards. Respondents also noted that regulators would need to be adequately resourced with technical expertise and skills to implement the framework effectively.
154. Respondents consistently emphasised the importance of international harmonisation to effective AI regulation. Some respondents suggested that the UK should work towards an internationally aligned regulatory ecosystem for AI by developing a gold standard framework and promoting best practice through key multilateral channels such as the OECD, UN, GPAI, G7, G20, and the Council of Europe. Respondents noted that divergent or overlapping approaches to regulating AI would cause significant compliance burdens. Respondents argued that international cooperation can support responsible AI innovation in the UK by creating clear and certain rules that allow investments to move across multiple markets. Respondents also suggested establishing bilateral working groups with key strategic partners to share expertise. Some respondents stressed that the UK’s pro-innovation approach should be delivered at pace to remain competitive with a fast-moving international landscape.
Response:
155. We acknowledge that many respondents would like more detail on the implementation of the framework set out in the AI regulation white paper, particularly regarding AI-related risks. We have already started to deliver the proposals set out in the white paper, working quickly to establish centralised, cross-economy risk assessment activities within the government to identify, measure, and mitigate risks. Building from this work, we published research on frontier AI capabilities and risks for discussion at the AI Safety Summit[footnote 117]. It outlined initial evidence on the most advanced AI systems and how their capabilities and risks may continue to develop. The significant uncertainty in the evidence highlights the need for further research.
156. This year, we will consult on a cross-economy risk register for AI, seeking expert views on our risk assessment methodology and whether we have comprehensively captured AI-related risks. The AI Safety Institute will advance the world’s knowledge of AI safety by carefully examining, evaluating, and testing advanced AI systems. It will conduct fundamental research on how to keep people safe in the face of fast and unpredictable technological progress.
157. In the white paper, we proposed an adaptable, principles-based approach to regulating AI in order to keep pace with rapid technological change. We will use our risk assessment and monitoring and evaluation activities to continue to assess measures for the targeted, proportionate, and effective prevention and mitigation of any new and accelerated risks related to AI, including those potentially posed by the development of the most powerful systems.
158. We agree that an effective framework for regulating AI will need to carefully balance cross-sector consistency with sector specific needs in order to support responsible innovation. Our context-focused framework builds from the domain expertise of the UK’s regulators, ensuring that different industries benefit from existing regulatory knowledge. While this approach streamlines compliance within specific sectors, we recognise the need for consistency and coordination between regulators to create an easily navigable regulatory landscape for businesses and consumers. That is why, as we note in detail in our responses to questions on regulator capability and AI sandboxes and testbeds (sections 6.5 and 6.10), we have been focusing on building from the existing strengths of UK regulators by establishing a pilot advisory service for AI innovators through the DRCF, sharing guidance on implementation, and building common regulator capability.
159. Alongside our work to quickly deliver on the centralised risk assessment and regulatory capability and coordination activities, the UK has led the way in convening world leaders at the first ever AI Safety Summit in order to establish an aligned approach to the most pressing risks related to the cutting-edge of AI technology. Countries agreed to the Bletchley Declaration at the AI Safety Summit, recognising the need for international collaboration in understanding the risks and opportunities of frontier AI[footnote 118]. We will deliver a groundbreaking International Report on the Science of AI Safety to promote an evidence-based understanding of advanced AI[footnote 119]. Additionally, the UK, through the AI Safety Institute, will collaborate with other nations, including the US, to enhance our capability to research and evaluate AI risks, underscoring our ability to drive change through international coordination on this critical topic.
160. Our work at the AI Safety Summit is complemented by multilateral engagement in other AI-focused forums, such as the G7 Hiroshima process, G20, UN, GPAI, and Council of Europe. In multilateral engagements, we are working to leverage each forum’s strengths, expertise, and membership to prevent overlap or divergences with other regulatory systems, ensuring they are adding maximum value to global AI governance discussions and the UK’s values and economic priorities. The UK is also pursuing bilateral cooperation with many partners, reflecting our commitment to interoperability and establishing international norms for responsible AI innovation.
6.8. Legal responsibility for AI
L1. What challenges might arise when regulators apply the principles across different AI applications and systems? How could we address these challenges through our proposed AI regulatory framework?
L2.i. Do you agree that the implementation of our principles through existing legal frameworks will fairly and effectively allocate legal responsibility for AI across the life cycle?
L.2.ii. How could it be improved, if at all?
L3. If you work for a business that develops, uses, or sells AI, how do you currently manage AI risk including through the wider supply chain? How could government support effective AI-related risk management?
Summary of questions L1-L3:
161. While respondents praised the benefits of a principles-based approach, nearly half were concerned about potential coordination issues between regulators and consistency across sectors. Some were concerned about confusing interdependencies between the AI regulation framework and existing legislation. Respondents asked for sector-based guidance from regulators, compliance tools, and regulator engagement with industry. Some respondents also pointed to the importance of international alignment and collaboration.
162. A majority of respondents disagreed that the implementation of the principles through existing legal frameworks would fairly and effectively allocate legal responsibility for AI across the life cycle. Just under a third of respondents felt that the government should clarify AI-related liability. However, there was not clear agreement about where liability should sit, with respondents noting a range of potential responsibilities for different actors across the AI life cycle. There was repeated acknowledgement of the complexity of AI value chains and the potential variations in use-cases. Some voiced concerns about gaps in existing legislation, including intellectual property, legal services, and employment law.
163. Around a quarter of respondents to L2.ii stated that new legislation and regulatory powers would be necessary to effectively allocate liability across the life cycle. Respondents stressed the importance of a legally responsible person for AI within organisations, with a few suggestions of an AI equivalent to Data Protection Officers. Some respondents wanted more detail on how the principles will be implemented through existing law, with a few recommending that regulatory guidance would clarify the landscape. A small number of respondents noted that the proposed central functions, including risk assessment, horizon scanning, and monitoring and evaluation, would help assess and adapt the framework to ensure that legal responsibility for new AI-related risks is adequately distributed. A couple of respondents also suggested pre-deployment measures such as licensing and pre-market approvals.
164. Nearly half of organisations that responded to L3 told us that they used risk assessment processes for AI, with many building from sectoral best practice or trade body guidance. Respondents pointed to existing legal frameworks that capture AI-related risks, such as product safety and data protection laws, and stressed that any future AI measures should avoid duplicating or contradicting existing rules. Respondents suggested that it would be useful for businesses to understand the government’s view on AI-related best practices, with some recommending a central guide on using AI safely. Some smaller businesses asked for targeted support to implement the AI principles.
165. Respondents consistently stressed the importance of transparency as a tool for education, awareness, consent, and contestability. Echoing answers to questions Q2 and F1, many respondents mentioned that organisations should be transparent about AI use, outputs, and training data.
Response:
166. We are pleased to note respondents’ broad support for a principles-based approach to AI regulation that can provide proportionate oversight across the many potential applications and uses of AI technologies. We agree with respondents that, as we implement the framework set out in the white paper, it is important to coordinate between regulators, sectors, existing legal frameworks, and the fast-moving international regulatory landscape. That is why we have been working at pace to establish the activities of the central function outlined in the white paper (for a detailed overview see section 5.1.2).
167. We note that there are still questions regarding how to fairly and effectively allocate legal responsibility for AI across the life cycle. We also recognise that many responses endorsed further government intervention to ensure the fair and effective allocation of liability across the AI value chain. Responses stressed the complexity and variability of AI supply chains, with use-cases highlighting expansive ethical and technical questions. We agree that there is no easy answer to the allocation of legal responsibility for AI and we also agree that it is important to get liability and accountability for AI right in order to support innovation and public trust. Building on the commitment to examine foundation models in the white paper, we have focused our initial life cycle accountability work on highly capable general-purpose systems (for details see section 5.2).
168. We are also continuing to analyse how existing legal frameworks allocate accountability and legal responsibility for AI across the life cycle. Our initial analysis suggests that a context-based approach to regulating AI may not adequately address risks arising from highly capable general-purpose systems since a context-based approach does not effectively and fairly allocate accountability to developers of those systems. We are exploring a range of potential obligations targeted at the developers of these systems including those suggested by respondents such as pre-market permits, model licensing, accountability and governance frameworks, transparency measures, and changes to existing legal frameworks. As we continue to iterate the AI regulation framework, we will consider introducing measures to effectively allocate accountability and fairly distribute legal responsibility to those in the life cycle best able to mitigate AI-related risks.
169. We are encouraged by the wide range of risk assessment and management processes that respondents told us they are already using. Our “Emerging processes for frontier AI safety” paper outlines a set of practices to inform the development of organisational AI safety policies[footnote 120]. It provides a snapshot of promising ideas and associated practices in AI safety today. As discussed in response to questions on the cross-sectoral principles (section 6.1), we acknowledge the broad support for measures on transparency and we will continue our work assessing whether and which measures provide the most meaningful transparency for AI end users and actors across the AI life cycle.
6.9. Foundation models and the regulatory framework
F1. What specific challenges will foundation models such as large language models (LLMs) or open-source models pose for regulators trying to determine legal responsibility for AI outcomes?
F2. Do you agree that measuring compute provides a potential tool that could be considered as part of the governance of foundation models?
F3. Are there other approaches to governing foundation models that would be more effective?
Summary of questions F1-F3:
170. While respondents supported the AI regulation framework set out in the white paper, many were concerned that foundation models may warrant a bespoke regulatory approach. Some respondents noted that foundation models are characterised by their technical complexity and stressed their potential to underpin many different applications across multiple sectors. Nearly a quarter of respondents emphasised that foundation models make it difficult to determine legal responsibility for AI outcomes and shared hypothetical use-cases where both upstream and downstream actors are at fault. Respondents stressed that technical opacity, complex supply chains, and information asymmetries prevent sufficient explainability, accountability, and risk assessment for foundation models.
171. Around a fifth of respondents expressed concerns about how foundation models use data, including whether data is of adequate quality, appropriate for downstream applications, compliant with existing law, and sourced ethically. Some stated that it is not clear who is responsible for deciding whether or not data is appropriate to a given application. Respondents stressed that training data currently lacks a clear definition, technical standards, and benchmark measurements.
172. Some respondents noted concerns regarding wider access to AI, including open source, leaking, or malicious use of models. However, a similar number of respondents noted the importance of open source to AI innovation, transparency, and trust.
173. Half of respondents felt compute was an inadequate proxy for governance requirements, with some recommending assessing models by their capabilities and applications instead. Respondents felt that model verification measures, such as audits and evaluations, would be effective, with some suggesting these should be mandatory requirements. A few noted the importance of downstream monitoring or post-market surveillance.
174. About a third of respondents supported governance measures including tools for trustworthy AI such as technical standards and assurance. One respondent suggested a pre-deployment sandbox. A few supported moratoriums, bans, or limits. A small number of respondents suggested that contracts, licences, user agreements, and (cyber) security measures could be used to govern foundation models.
Response:
175. We acknowledge the range of challenges that respondents have raised in regard to foundation models and note the particular attention given to the core characteristics or features of foundation models such as technical opacity and complexity. We also recognise that challenges arise from the fact that foundation models can be broad in their potential applications and, as such, can cut across sectors and impact upon a range of risks. Our analysis shows that many regulators can struggle to enforce existing rules and laws on the developers of highly capable general-purpose AI systems within their current statutory remits in a way that effectively mitigates risk.
176. In response to repeated calls for specific regulatory interventions targeted at foundation models, we have been exploring the impact of foundation models on life cycle accountability for AI. In the AI regulation white paper, we stated that legal responsibility for AI should sit with the actor best able to mitigate any potential risks it poses. Our assessment suggests that, despite their ability to mitigate risks when designing and developing AI, the organisations building highly capable general-purpose systems are currently unlikely to be impacted by existing rules and laws in a way that sufficiently mitigates risk. That is why we are exploring options for targeted, proportionate interventions focusing on these systems and the risks that they present. We have been assessing measures to mitigate risk during the design, training, and development of highly capable general-purpose systems. We have also been exploring options for ensuring effective accountability, including legally mandated obligations, while avoiding cumbersome red-tape.
177. We note respondent views that compute is an imperfect proxy for foundation model capability. As part of our work exploring the right guardrails for highly capable general-purpose systems, we are examining how best to scope any regulatory requirements based on model capabilities, and the risks associated with these, wherever possible. But we recognise that, in some cases, controls might need to be in place before a model’s capability is known. In these cases, limited and careful use of proxies may be necessary to target regulatory requirements to only those systems that pose the most significant potential risks. Our early analysis indicates that initial thresholds could be based on forecasts of capabilities using a combination of two proxies: compute and capability benchmarking. However there might need to be a range of thresholds. For more detail, see section 5.2.
178. To provide greater clarity on best practices for responsible AI innovation – including using data – we published a set of emerging safety processes for frontier AI companies for the AI Safety Summit in 2023[footnote 121]. The document consolidates emerging thinking in AI safety and has been written for AI organisations and those who want to better understand their safety policies. We will update this guide by the end of the year and continue to encourage AI companies to develop best practices (see section 5.2.2 for detail).
179. We acknowledge respondents’ views on both the value and risks of open source AI. Open access can provide wide benefits, including helping to mitigate some of the risks caused by highly capable general-purpose systems. However, open release can also exacerbate the risk of misuse. We believe that all powerful and potentially dangerous systems should be thoroughly risk-assessed before being released. We will continue to monitor and assess the impacts of open model access on risk. We will also carefully consider the impact of any potential measures to regulate open source systems on competition, innovation, and wider risk mitigation.
180. As set out in section 5.2, we will continue our technical policy analysis to refine our thinking on highly capable general-purpose systems in the context of AI regulation and life cycle accountability. We will continue to engage with external experts on a range of challenging topics such as how effective voluntary measures could be at mitigating risks and the right scope of any additional regulatory interventions including proxies and capability thresholds. We will also continue to examine questions related to accountability and liability, including the extent to which existing laws and regulators can “reach” through the value chain to target the developers of highly capable general-purpose systems and the potential impact of open release. We will also engage with regulators to learn from their existing work on this topic. For example, we will continue to engage with the CMA on their work on foundation models.
6.10. AI sandboxes and testbeds
S1. To what extent would the sandbox models described in section 3.3.4 support innovation?
S2. What could government do to maximise the benefit of sandboxes to AI innovators?
S3. What could government do to facilitate participation in an AI regulatory sandbox?
S4. Which industry sectors or classes of product would most benefit from an AI sandbox?
Summary of questions S1-S4:
181. Overall, respondents were strongly supportive of a regulatory sandbox for AI. The highest proportion of respondents agreed that the “multiple sector, multiple regulator” and “single sector, multiple regulator” sandbox models would be most likely to support innovation, stating that the cross-sectoral or cross-regulator basis would help develop effective guidance in response to live issues, harmonise rules, and coordinate implementation of the AI regulation framework. While there was no majority consensus on a specific sector that would most benefit from a sandbox, the largest proportion of question respondents stated that healthcare and medical devices would most benefit from an AI sandbox, followed by financial services and transport.
182. Some respondents suggested collaborating with the wider AI ecosystem to maximise the benefit of sandboxes to AI innovators. Many recommended building on the existing strengths of the UK regulatory landscape, such as the DRCF. Linked to this, a few respondents noted that an AI regulatory sandbox presents an opportunity for the UK to demonstrate global leadership in AI regulation and technical standards by sharing findings and best practice internationally.
183. Some respondents recommended making information accessible to maximise the benefit of the sandbox to participants and the wider AI ecosystem. Respondents wanted participation pathways, training, tools, and other resources to be technically and financially accessible. Many respondents noted that accessible guidance and tools would allow organisations to engage with the sandbox. In particular, respondents emphasised the benefits of accessible information for smaller businesses and start-ups who are new to the regulatory process. Respondents advocated for regular reporting on sandbox processes, evidence, findings, and outcomes to encourage “business-as-usual” best practices for AI across the wider ecosystem.
184. Respondents noted the importance of reducing the administrative burden on smaller businesses and start-ups to lower the barrier to entry for those with less organisational resources. Some noted that financial support would help ensure that smaller businesses and start-ups could participate in resource-intensive research and development focused AI sandboxes. Respondents felt that sharing evidence, guidance, and tools would ensure the wider AI ecosystem benefitted from the sandbox. Some suggested access to datasets or product accreditation schemes would incentivise participation in supervised test environment sandboxes.
Response:
185. The response to the consultation – which aligns with independent research commissioned through the Regulators’ Pioneer Fund – has helped to inform the government’s decision to fund a pilot multi-regulator advisory service offered by the DRCF: the AI and Digital Hub. In particular, it has helped to clarify that a new regulatory service is likely to add most value supporting AI innovators from a range of sectors to navigate the multiple regulatory regimes that govern the use of cross-cutting AI products and services, rather than through targeting one specific regulatory remit or regulated sector.
186. The DRCF AI and Digital Hub brings together four of the most critical regulators of AI and digital technologies, including the CMA, ICO, Ofcom, and the Financial Conduct Authority (FCA). Together these regulators are responsible for overseeing some of the most significant regulatory regimes that govern AI products, whether cross-economy (data protection, competition and consumer regulation) or sectoral (financial services, telecommunications and broadcasting).
187. Respondents to the consultation also emphasised the importance of making information and resources relating to the sandbox accessible in order to maximise its benefits. Respondents noted the need to reduce the compliance burden for smaller businesses and start-ups in particular. Again, these considerations are central to the design and operation of the DRCF AI and Digital Hub. In addition to providing tailored support to participating innovators that will be accessed via a simple online application process, the Hub will also publish anonymised case-studies and guidance to support a broader pool of innovators facing similar compliance challenges. Our research has indicated that a repository of use cases such as this will be a particularly effective means of amplifying the outreach and impact of such a pilot.
188. We note that some respondents suggested that additional incentives such as product accreditation or access to data would encourage participation in a sandbox for AI. These additional incentives would best suit a supervised test environment sandbox model. As the DRCF’s AI and Digital Hub pilot phase will focus on providing compliance support, these additional incentives will not be included. However, we are committed to reviewing how the service needs to develop – and what further measures are necessary to support AI and digital innovators – in the light of the pilot findings and further feedback from stakeholders.
Annex A: method and engagement
Consultation method and engagement summary
1. With the publication of the AI regulation white paper on 29 March 2023, we held a formal 12-week public consultation that closed on 21 June 2023. In total, we heard from over 545 different individuals and organisations.
2. Stakeholders were invited to submit evidence in response to 33 questions on the government’s policy proposals for a regulatory framework for AI. Stakeholders were invited to submit evidence through an online survey, email, or post. In total, we received 409 responses in writing. Removing 50 duplicates and blanks left 359 written submissions. See Written submissions below for more detail.
3. We also proactively engaged with 364 individuals through roundtables, technical workshops, bilaterals, and a programme of on-going regulator engagement. Our roundtables sought the views of stakeholders that we might hear from less often with topics including the impact of AI on marginalised communities, public trust, and citizen perspectives. We also held roundtables focused on smaller businesses and the open source community. More detail can be found in the Engagement method and Engagement findings sections below.
Method for analysing written submissions
4. We received written consultation responses from organisations and individuals through an online survey and email. Of the total 409 responses, we received 232 through our online survey and 177 by email.
5. Of the 33 questions, 12 were closed questions with predefined response options on the online survey. We manually coded submissions by email that explicitly responded to these closed questions to follow the Likert-scale structure. The remaining 21 questions invited free text qualitative responses and each response was individually analysed and manually coded. As such, quantitative analysis represents all stakeholders who answered a specific question through email or the online survey. Not all respondents answered every question and we present our findings as an approximate proportion of responses to the question.
6. In accordance with our privacy notice[footnote 122] and online survey privacy agreement, only those individuals and organisations who submitted evidence through our online survey and consented to our privacy agreement will have their names published in the list of respondents (see Annex B).
7. Respondents to the online survey self-selected an organisation type and sector. We manually assigned organisation types and sectors to respondents who submitted written evidence through email. After removing blanks and duplications, we received responses from across 8 organisation types and 18 sectors. Chart M1 shows response numbers by organisation type. The majority of responses came from industry, business, trade unions, and trade associations. This is followed by individuals not representing an organisation and then research groups, universities, and think tanks.
8. Chart M1: AI regulation white paper consultation respondents by organisation type
Department | Total | |
---|---|---|
Industry, business, trade union, association | 132 | |
Individuals | 63 | |
Research organisation, university, think tank | 39 | |
Small or Medium sized Enterprises (SMEs) | 37 | |
Charity, non-profit, social, civic, activist | 28 | |
Other | 24 | |
Regulators | 23 | |
legal services or professional advisory body | 13 |
9. Chart M2: AI regulation white paper consultation respondents by sector
Sector | Total | |
---|---|---|
AI, digital, and technology | 75 | |
Other | 69 | |
Arts and entertainment | 31 | |
Financial services and insurance | 22 | |
Education | 22 | |
Research and development | 22 | |
Healthcare | 20 | |
Legal services | 19 | |
IT | 18 | |
Undiclosed | 14 | |
Public sector | 14 | |
Regulation | 13 | |
Communications | 8 | |
Secondary sectors | 5 | |
Transportation | 4 | |
Real estate | 2 | |
Primary sectors | 1 |
M2 Note: Primary sectors include extraction of raw materials, farming, and fishing. Secondary sectors include utilities, construction, and manufacturing.
10. The sector breakdown in Chart M2 shows that the biggest number of responses came from the AI, digital, and technology industry. This was followed by respondents who selected “other” and then those in the arts and entertainment sector. Further analysis of “other” responses suggests that these responses were often from individuals not representing an organisation and included students.
11. As these demographics indicate, this sample, as with all written consultation samples, may not be representative of public opinion as some groups are over or under represented.
12. In particular, we note that responses received from a number of creative industries stakeholders were either identical or very similar. These responses largely focused on AI and copyright. These responses were analysed and included in the same way as all other responses.
13. 89 emailed pieces of evidence followed the question structure of our online survey. These were analysed alongside responses from the survey to inform quantitative analysis. After removing duplicate responses, we included 66 emailed responses in our analysis.
14. 88 emailed responses provided evidence beyond the scope of our consultation questions or without explicit reference to the questions. We analysed these submissions individually. While our findings from this analysis informs our overall response, we do not include these responses within our quantitative analysis as they do not explicitly answer our consultation questions. Where relevant, we have used insights from these responses to inform our qualitative question summaries. After removing duplicate responses, we included 84 of these in our qualitative analysis.
15. We received 33 duplicate responses that were sent twice through either the online survey or email. We received requests for 4 of these duplications to be deleted on grounds they were incorrect and superseded by a later response. These duplicates were removed from analysis entirely. The remaining 29 duplicates were responses sent by both online survey and email. Where appropriate, we removed either the email or survey response from our quantitative analysis to avoid skewing counts with duplicate submissions. However, in consideration of additional detail given, we analysed both responses to weave any additional insights into our overall qualitative analysis. A further 17 written responses were discounted from analysis entirely on the grounds that they were blank or contained spam. After reviewing and cross-checking responses, we discounted 50 written submissions from the final analysis to avoid overcounting blanks, spam, and duplicate responses. That left 359 submissions of which 209 were received through the online survey and 150 by email.
16. We use illustrative qualitative language such as “many”, “some”, and “a few” to summarise the written responses we received to our consultation. These descriptions are intended to provide an indication of the extent that a particular theme or sentiment was raised by respondents. Not all respondents answered every question. We refer to approximate amounts of respondents to each question, including “a half”, “a quarter”, or “a third”. We use the terms “nearly all” or “most” when a substantial majority of respondents made a particular argument or shared a sentiment. We use the terms “a majority” or “over half” to show when a point was shared by over 50% of respondents. We use “many” when lots of respondents raised a similar point but the theme or sentiment was not shared by over half of respondents. We use “some” to indicate when a theme or sentiment was shared by between a tenth and a fifth of respondents. We use “a few” when a smaller number of respondents made a similar point. We use a “small number” to describe when less than 10 respondents raised a point, specifying if this is “one” or “two” (“a couple”).
Engagement method
17. We held 19 roundtables engaging 278 individuals representing a range of perspectives and organisation types including AI industry, digital, and technology organisations, small businesses and start-ups, companies that use AI, the open source community, trade bodies and unions, legal services, financial services, creative industries, academics, think tanks, research organisations, regulators, government departments, the public sector, charities and advocacy groups, citizens, marginalised communities, and wider civil society.
18. Some roundtables focused on hearing from regulators or stakeholders within a specific sector, including education, transport, financial services, legal services, and health and social care. Others focused on technical elements of the regulatory framework such as methods for AI verification, liability, and tools for trustworthy AI, including technical standards. Some discussions were designed to understand the views of stakeholders we might hear from less often: one explored the impact of AI on marginalised communities, another examined the role of public trust, two further roundtables focused on the perspectives of small businesses and the open source community, and the Minister for AI and Intellectual Property, Viscount Camrose, chaired a citizens roundtable during London Tech Week. Other topics included AI safety, international interoperability, approaches to responsible AI innovation in industry, and the UKRI’s AI Technology Mission.
19. We are grateful to the partners who worked with us to organise roundtables and workshops including CDEI, the Department for Education (DfE), the Department of Health and Social Care (DHSC), the Department for Transport (DfT), the Ministry of Justice (MOJ), UK Research and Innovation (UKRI), the British Computer Society (BCS), Hogan Lovells, Innovate Finance, the Ada Lovelace Institute, the Alan Turing Institute, Open UK, the British Standards Institution (BSI), and the University of Bath ART-AI.
20. Alongside this programme of roundtable discussions and technical workshops, we engaged with 42 stakeholders through external engagements where we presented the AI regulation framework outlined in the white paper. We also held 28 bilaterals and held meetings with 16 regulators as part of our on-going work to support implementation. We include insights from this engagement throughout the consultation response.
Engagement findings
21. In this section, we provide a brief overview of our roundtables and workshops, summarising insights into four areas based on roundtable focus and participation from:
- regulators.
- industry.
- civil society.
- research organisations.
Regulators
22. We held six roundtables with regulators to understand existing capabilities and needs, including how the approach set out in the AI regulation white paper would be implemented into specific sectors including health and social care, justice, education, and transport.
23. Regulators reported varying levels of in-house AI knowledge and capability, with most supporting central measures to enhance technical expertise. Some agreed that a pool of expertise could enhance regulatory capacity, while others suggested that the proposed central function could provide guidance and training materials for regulators.
24. Regulators were broadly supportive of the central function outlined in the white paper, emphasising that they could serve as a useful point of contact for regulators. However, regulators also stressed that the central function should not infringe on the independence or existing statutory remits of regulators, suggesting that any guidance to regulators on the implementation of the principles should not impede, duplicate, or contradict regulators’ current mandates and work.
25. Participants at the roundtables emphasised that regulators need adequate resources, endorsing government investment in technical capability and capacity. Some noted that the government may also need to introduce new regulatory powers in order for the framework to be effective, stating that achieving meaningful transparency and contestability may require the government to mandate disclosure from developers and deployers of AI at set points.
26. Participants raised several challenges to effective regulator oversight specific to AI including unknown and changing functional boundaries, technical obscurity, unpredictable environments, lack of human oversight or input, and highly iterative technological life cycles. Regulators suggested that collaboration between regulators, safety engineers, and AI experts is key to creating robust verification measures that prevent, reduce, and mitigate risks.
27. While regulators stated that the principles provide useful common ground across sectors, they noted that sector-specific analysis would be necessary to identify gaps in the framework. Some noted that sector specific use-cases would help regulators apply the principles in their respective domains.
Industry
28. We heard from a range of industry stakeholders at seven roundtable events with topics ranging from international interoperability, responsible AI in industry, general-purpose AI, and governance and technical standards needs.
29. Some participants were concerned that market imbalances were preventing innovation and competition across the AI ecosystem. In particular, participants argued that more accessible, traceable, and accountable data would promote innovation, noting that smaller companies often have to rely on existing market leaders or lower quality datasets due to the lack of affordable commercial, proprietary datasets. Participants suggested that clear standards for data and more equitable access to higher quality datasets would stimulate AI innovation across the wider ecosystem and prevent incumbent advantages.
30. Participants also noted that some of the potential measures to regulate AI could allow current market leaders to further entrench their advantages and increase existing market imbalances. Participants noted that smaller businesses and the open source community could face a significant compliance burden, with some suggesting that regulatory sandboxes should be used to test the impact of regulation. While some suggested that legal responsibility for AI should be allocated to earlier stages in the life cycle, others warned that placing the legal responsibility for downstream applications on open source developers would severely limit innovation as they would not be able to account for the many potential uses of open source code.
31. There was no consensus on whether licensing requirements for foundation models would effectively encourage responsible AI innovation or, instead, concentrate market power among a few established companies. A few participants noted that practical guidance on implementation and use-cases would support organisations to apply the principles. Some participants noted a licensing framework that only allowed open access to some parts of an AI system’s code could retain some of the benefits of the information sharing and transparency that defines open source.
32. Some participants stated that it is not clear whose job it is to regulate AI, advocating for a new, AI-specific regulator or a clear lead regulator. Participants emphasised the importance of technical expertise to effective regulation.
33. Participants also noted the important role of international interoperability, insurance, technical standards, and transparency in market success for AI.
Civil society and public trust
34. Three roundtables were held with smaller businesses, civil society stakeholders, and special interest groups to discuss public trust and the impact of AI on citizens and marginalised communities.
35. Participants emphasised that fairness and inclusivity were key to realising the benefits of AI for everyone. Participants noted the importance of diversity in regard to the data used to train and build AI, as well as the teams who develop, deploy, and regulate AI. Participants suggested co-creation and collaboration with marginalised communities would ensure that AI could create benefits for everyone.
36. Participants also stressed that organisations using AI not only need to be transparent about when and how AI is used but should also make explanations accessible to different groups. Participants noted that, while AI can offer benefits to marginalised communities, these populations often face a disproportionate negative impact from AI. Participants called for more education on the use of AI on the grounds that there is currently a significant lack of consumer awareness, organisational knowledge, and accessible redress routes.
37. Participants noted that regulators have a key role to play in improving access to contest and seek redress for AI-related harms. Participants emphasised that regulators require adequate funding and resources in order to achieve this. Participants strongly supported a central ombudsman for AI to improve the accessibility of high-quality legal advice on AI. Many noted that legal advice on AI is currently expensive, hard to access, and sometimes given by unregulated providers outside of the legal profession. Participants also noted that the ombudsman would likely receive a large number of small-scale complaints, which they should be adequately equipped to deal with.
38. Participants also advocated for the importance of specific safeguards for young people including potential changes to existing statutory mechanisms such as those for data protection and equality.
Academia, research organisations, and think tanks
39. We held three events to hear from academics, research organisations, and think tanks on AI safety, legal responsibility for AI, and the UKRI’s AI Technology Mission.
40. Participants suggested differentiating the types of risk posed by AI, noting that both immediate and long term risks would need to be factored into any safety measures for AI. Participants felt that sector-specific analysis should inform assessments of AI-related risks. Participants noted that the technical obscurity of AI can make it difficult for organisations and regulators to determine the cause of any harms that arise. Participants emphasised that, in order to prevent harms, pre-deployment measures are key to ensuring that AI is safe for market release.
41. Participants argued that high quality regulation can help AI move quickly and safely from development to market. Participants argued that there was a need for greater technical knowledge across government and regulators, along with better AI skills across the wider ecosystem. Some called for the certification of AI engineers and developers to enhance public confidence, while another promoted the certification of institutional leads responsible for decisions related to AI. There was no consensus on whether a new, central regulator for AI or existing regulators would implement the proposed framework most effectively. However, participants agreed that aligning regulatory guidance and sharing expertise across sectors would build compliance capability. Participants suggested a “mixed economy” of regulation, with statutory requirements to ensure rules worked effectively.
42. Participants noted that AI life cycles are varied and complex. Participants wanted the government to define actors across the AI life cycle and determine corresponding obligations to clarify the landscape. However, there was no agreement on the best way to do this with participants suggesting actors may be defined by their function (as in data protection regulation), market power or benefit (as in digital markets regulation), or proximity to and reasonable foreseeability of risks (as in product safety legislation). While some participants wanted to see more stringent responsibilities for foundation model developers, others warned that too too narrow a focus could mean that other AI-related opportunities might be missed.
Annex B: List of consultation respondents
List of consultation respondents
1. We are grateful to all the individuals and organisations who shared their insights with us over the course of the consultation period.
2. Our AI regulation framework is intended to be collaborative and we will continue to work closely with regulators, academia, civil society, and the public in order to monitor and evaluate the effectiveness of our approach.
3. In accordance with our privacy notice[footnote 123] and online survey privacy agreement, only those individuals and organisations who submitted evidence through our online survey and consented to our privacy agreement there have their names listed below. The list represents the 209 online survey submissions that we analysed after cleaning the data for duplications, blanks, and spam (see Annex A for details). Names are listed as they were given, with personal names removed if an organisation name was available. We provide 207 names here as 2 responses included no name.
4. Further detail on the organisation type and sector of those we received written responses from by email and online survey can be found in the extended method for analysing written responses in Annex A.
Respondents to the online consultation survey
-
Adarga Limited
-
ADS Group
-
Advai Ltd
-
AGENCY: Assuring Citizen Agency in a World with Complex Online Harms
-
Agile Property & Homes Limited
-
AI & Partners
-
AI Centre for Value Based Healthcare
-
Aidan Freeman
-
AIethics.ai
-
Alacriter
-
Aligned AI
-
Alliance for Intellectual Property
-
Altered Ltd
-
Amendolara Holdings Limited
-
Anton
-
Arran McCutcheon
-
ART-AI, University of Bath
-
Arts Council England
-
Association for Computing Machinery Europe Technology Policy Committee
-
Association of British HealthTech Industries
-
Association of Chartered Certified Accountants (ACCA)
-
Association of Financial Mutuals
-
Association of Illustrators
-
Association of Learned and Professional Society Publishers
-
Assuring Autonomy International Programme, University of York
-
Avi Semelr
-
Baringa Partners LLP
-
Barnacle Labs
-
Barry O’Brien
-
Ben Hopkinson
-
BPI British Phonographic Industry
-
Bristows LLP
-
British Copyright Council
-
British Pest Control Association
-
British Security Industry Association
-
Brunel University London Centre for Artificial Intelligence: Social & Digital Innovations
-
BSI Group The Netherlands B.V.
-
BT Group
-
Bud Financial
-
Calvin Karpenko
-
Carlo Attubato
-
Center for AI and Digital Policy Washington, DC. USA
-
Centre for Policy Studies
-
Charlie Bowler
-
Chegg, Inc.
-
Cisco
-
City, University of London
-
Cogstack
-
Colin Hayhurst
-
Congenica Ltd
-
Craig Meulen
-
Creators’ Rights Alliance
-
CTRL-Shift & Collider Health
-
Cyferd
-
CyLon Ventures
-
DACS (Design and Artists Copyright Society)
-
Daniel Marsden
-
Darrell Warner Limited
-
Deborah W.A. Foulkes
-
Deloitte UK
-
Developers Alliance
-
Department for Education (DfE)
-
Direct Line Group
-
DNV
-
Dr. Michael K. Cohen
-
EasyJet Airline Company Ltd.
-
Ed Hagger
-
EKC Group
-
Elliott Andrews
-
Emily Gray
-
Emma Ahmed-Rengers
-
Enzai Technologies Limited
-
Equity
-
Eviden
-
Experian UK&I
-
Falcon Windsor
-
FlyingBinary
-
ForHumanity
-
Freeths LLP
-
Fujitsu
-
Full Fact
-
Geeks Ltd.
-
Getty Images
-
GlaxoSmithKline plc
-
Glenn Donaldson
-
Global Witness
-
Greg Colbourn
-
Greg Mathews
-
Guy Warren
-
Hazy
-
Henry
-
Hollie
-
Hugging Face
-
Iain Darby
-
International Federation of the Phonographic Industry (IFPI)
-
INRO London
-
Institute for the Future of Work
-
Institute of Chartered Accountants in England and Wales (ICAEW)
-
Institute of Innovation and Knowledge Exchange (IKE Institute)
-
Institute of Physics and Engineering in Medicine
-
Institute of Physics and Engineering in Medicine (Clinical and Scientific Computing group)
-
Institution of Occupational Safety and Health
-
International Federation of Journalists
-
Jake Bailey
-
Jake Wilkinson
-
Japan Electronics and Information Technology Industries Association
-
Joe Collman
-
Johnny Luk
-
Johnson & Johnson
-
Jonas Herold-Zanker
-
Joseph Johnston
-
Judith Barker
-
Kainos Software Ltd
-
Kelechi Ejikeme
-
Knowledge Associates Cambridge Ltd.
-
Labour for the Long Term
-
Legal & General Group PLC
-
Leverhulme Centre for the Future of Intelligence
-
Lewis
-
LSE Law, Technology and Society Group
-
Lucy Purdon
-
Luke Richards
-
Lumi Network
-
Market Research Society
-
Marta
-
Martin Gore
-
Mastercard Europe
-
MedTech Europe
-
Megha Barot
-
Michael Fisher
-
Michael Pascu
-
Microsoft
-
Mind Foundry
-
Mukesh Sharma
-
National Physical Laboratory
-
National Taxpayers Union Foundation (NTUF)
-
National Union of Journalists
-
NATS
-
Nebuli Ltd.
-
Newcastle University
-
Newsstand
-
Nicole Hawkesford
-
Office for Standards in Education, Children’s Services and Skills (Ofsted)
-
Office for Statistics Regulation
-
Orbit RRI
-
Paul Dunn
-
Paul Evans
-
Paul Ratcliffe
-
Pearson
-
Phrasee
-
Pippa Robertson
-
Planar AI Limited
-
Policy Connect
-
Professional Publishers Association
-
Professor Julia Black
-
PRS for Music
-
Publishers Association
-
Publishers’ Licensing Services
-
Pupils 2 Parliament
-
Queen Bee Marketing Hive
-
Rebecca Palmer
-
RELX
-
Reset
-
Rohan Vij
-
Royal Photographic Society of Great Britain
-
Salesforce
-
SambaNova Systems inc
-
Samuel Frewin
-
SAP
-
Scale AI
-
ScaleUp Institute
-
Scott Timcke
-
Seldon
-
Sharon Darcy
-
Simon Kirby
-
Skin Analytics Ltd
-
South West Grid for Learning
-
Stability AI
-
Steve Kendall
-
STFC Hartree Centre
-
Surrey Institute for People-Centred Artificial Intelligence
-
Teal Legal Ltd
-
Temple Garden Chambers
-
The Copyright Licensing Agency Ltd
-
The Data Lab Innovation Centre
-
The Institute of Customer Service
-
The Multi-Agency Advice Service (MAAS) AI and Digital Regulations Service for health and social care.
-
The Operational Research Society
-
The Pharmacists’ Defence Association (PDA)
-
The Physiological Society
-
The Publishers Association
-
The Society of Authors
-
The University of Winchester
-
Tom Edward Ashworth
-
TRANSEARCH International
-
Trilateral Research
-
University of Edinburgh
-
University of Edinburgh
-
University of Winchester
-
Valentino Giudice
-
ValidMind
-
W Legal Ltd
-
Wales Safer Communities Network (membership from Police, Fire, Local Authorities, Probation and Third Sector), hosted by WLGA
-
Warwickshire County Council
-
We and AI
-
Workday
-
Writers’ Guild of Great Britain
Annex C: Individual question summaries
The revised cross-sectoral AI principles
1. Do you agree that requiring organisations to make it clear when they are using AI would improve transparency?
1. A majority of respondents agreed that requiring organisations to make it clear when they are using AI would adequately ensure transparency. Respondents who disagreed either felt labelling AI use would be insufficient or disproportionately burdensome.
2. Respondents who argued the measure would be insufficient often stated that regulators lack the relevant powers, funding, and capabilities to adequately ensure transparency. Linked to this, respondents noted issues around enforcement and access to appeal and redress. Some respondents recommended that the government should consider relevant statutory measures and accountability mechanisms. A few respondents suggested that explanations should be targeted to the context and audience.
3. Other respondents were concerned that a blanket requirement for transparency would create a burdensome barrier for lower risk AI applications. One respondent noted that the proposal assumes a single actor in the AI value chain will have adequate visibility across potentially many life cycle stages and applications. A few respondents wanted to see clear thresholds (including “high-risk applications”) and guidance from the government and regulators on transparency requirements.
4. Respondents were concerned that transparency measures may have potential interactions with existing and forthcoming legislation, such as that for data protection and intellectual property.
2. Are there other measures we could require of organisations to improve transparency for AI?
5. There was strong support for a range of transparency measures from respondents. Respondents stressed that transparency was key to building public trust, accountability, and an effective and verifiable regulatory framework.
6. Many respondents endorsed clear reporting obligations on the inputs used to build and train AI. Respondents noted that transparency would be improved through the disclosure of a range of inputs, from data to compute. Echoing responses to question F1 on foundation models, concerns coalesced around whether training data was of sufficient quality, compliant with existing legal frameworks including intellectual property and data protection, and appropriate for downstream uses. A few respondents argued that compute disclosure would improve transparency on the environmental impacts of AI.
7. Many respondents also supported the labelling of AI use and outputs, with many recommending the measure to improve user awareness and organisational accountability. Some respondents suggested that labelling AI generated outputs would help combat AI generated misinformation and promote intellectual property rights. A few respondents wanted to see clearer opt-ins for uses of data and AI, with options for human alternatives.
8. Some respondents endorsed measures that would encourage explanations for AI outcomes and potential impacts. This includes measures for showing users how models produced outputs or answers as well as addressing model limitations and impacts. Similarly, a few respondents noted the importance of organisational and public education through accessible information and targeted awareness raising. A couple of respondents suggested public or organisational registers for (high risk) AI would help improve awareness.
9. While some respondents advocated for reporting on model details, many emphasised that complex technical information would be best disclosed to regulators and independent verifiers rather than the public. Respondents suggested that organisations share technical model details such as weights, parameters, uses, and testing. Respondents stated that impact and risk assessments, as well as governance and marketing decisions, should be available to either regulators or the public, with a few noting potential compromises with trade secrets. Some respondents endorsed independent assurance techniques, such as third-party audits and technical standards.
10. A few respondents suggested clarifying legal rights and responsibilities for AI, with a few of those recommending the introduction of AI legislation and non-compliance measures.
3. Do you agree that current routes to contest or get redress for AI-related harms are adequate?
11. Over half of respondents reported that current routes to contest or seek redress for AI-related harms through existing legal frameworks are not adequate. In particular, respondents flagged that a lack of transparency around when and how AI is used prevents users from being able to identify AI-related harms. Similarly, respondents noted that a lack of transparency around the data used to train AI models complicates data protection and prevents intellectual property rights holders from exercising their legal and moral rights. A few respondents also noted the high costs of individual litigation and advocated for clearer routes for individual and collective action.
4. How could current routes to contest or seek redress for AI-related harms be improved, if at all?
12. Many respondents wanted to see the government clarify legal rights and responsibilities relating to AI, though there was no consensus on how to do this. Many respondents suggested clarifying rights and responsibilities in existing law through mechanisms such as regulatory guidance. There was also a broad appetite for centralisation in different forms with some respondents advocating for the creation of a central redress mechanism such as a central AI regulator, oversight body, coordination function, or lead regulator. Some respondents wanted to see further statutory requirements, such as licensing.
13. Many respondents stressed the importance of meaningful transparency and some emphasised the need for accessible redress routes. Respondents felt that measures to show users when and how AI is being used would help individuals identify when and how harms had occurred. Respondents wanted to see clear – and in some cases mandatory – routes to contest or seek redress for AI-related decisions. Respondents noted issues with expensive litigation, particularly in relation to infringement of intellectual property rights. Respondents felt that increasing transparency for AI systems would make redress more accessible across a broad range of potential harms and, similarly, that clarifying redress routes would improve transparency. Some respondents noted the importance of international agreements to ensure effective routes to contest or seek redress for AI-related harms across borders. Measures such as moratoriums and mandatory kill switches were only raised by a few respondents.
5. Do you agree that, when implemented effectively, the revised cross-sectoral principles will cover the risks posed by AI technologies?
14. A majority of respondents agreed that the principles would cover the risks posed by AI technologies when implemented effectively. Respondents that disagreed tended to cite concerns around enforcement and a lack of statutory backing to the principles or wider issues around regulator readiness, including capacity, capabilities, and coordination.
15. Respondents often noted a need for the framework to be adaptable, context-focused, and supported by monitoring and evaluation, citing the fast pace of technological change.
16. A few respondents felt the terms of the question were unclear and asked for further detail on effective implementation.
6. What, if anything, is missing from the revised principles?
17. Many respondents advocated for the cross-sectoral AI principles to more explicitly include human rights and human flourishing, noting that AI should be used to improve human life. Respondents endorsed different human rights and related values including freedom, pluralism, privacy, equality, inclusion, and accessibility.
18. Some respondents wanted further detail on the implementation of the principles. These respondents often asked for more detail on regulator capacity, noting that the “effective implementation” of the principles would require adequate regulator resource, skills, and powers. A couple of respondents asked for more clarity regarding how regulators and organisations are expected to manage trade-offs, such as explainability and accuracy or transparency and privacy.
19. Linked to this, some respondents wanted further guidance on how the AI principles would interact with and be implemented through existing legislation. Respondents mostly raised concerns in regard to data protection and intellectual property law, though a few respondents asked for a more holistic sense of the government approach to AI in regard to departmental strategies, such as the Ministry of Defence’s AI strategy. Some respondents stated that the principles would be ineffective without statutory backing, with a few emphasising the importance of mandating AI-related accountability mechanisms.
20. Some respondents advocated for the principles to address a range of issues related to operational resilience. These responses suggested measures for adequate security and cyber security, decommissioning processes, protecting competition, ensuring access, and addressing risks associated with over-reliance. A similar number of respondents wanted to see specific principles on data quality and international alignment.
21. A few respondents recommended the inclusion of principles that would clearly correlate with systemic risks and wider societal impacts, sustainability, or education and literacy. In regard to systemic risks, respondents tended to raise concerns about the potential harms that AI technologies can pose to democracy and the rule of law in terms of disinformation and electoral interference.
A statutory duty to regard
7. Do you agree that introducing a statutory duty on regulators to have due regard to the principles would clarify and strengthen regulators’ mandates to implement our principles, while retaining a flexible approach to implementation?
22. Over half of respondents somewhat or strongly agreed that a statutory duty would clarify and strengthen the mandate of regulators to implement the framework. However, many noted caveats that are detailed in Q8.
8. Is there an alternative statutory intervention that would be more effective?
23. Many felt that targeted statutory measures, including expanded regulator powers, would be a more effective statutory intervention. In particular, respondents noted the need for regulators to have appropriate investigatory powers. Some also wanted to see the consequences of breaches more clearly defined. Respondents also suggested specific AI legislation, a new AI regulator, and strict rules about the use of AI in certain contexts as more effective statutory interventions. A couple of respondents mentioned that any AI duties should be on those operating within the market as opposed to on regulators.
24. Some respondents felt the proposed statutory duty is the most effective intervention and should be implemented. However, other respondents couched their support within wider concerns that the framework would not be sufficiently enforceable without some kind of statutory backing. Nearly a quarter of respondents emphasised that regulators would need enhanced resources and capabilities in order to enact a statutory duty effectively. Other respondents felt that the implementation of a duty to regard could disrupt regulation, innovation, and trust if rushed. These respondents recommended that the duty should be reviewed after a period of non-statutory implementation, particularly to observe interactions with existing law and regulatory remits. A few respondents noted that the end goal and timeframes for the AI regulatory framework were not clear, causing uncertainty.
25. There was some support for the government to mandate measures such as third-party audits, certification, and Environmental, Social and Governance (ESG) style supply chain measures, including reporting on training data. A few respondents were supportive of central monitoring to track regulatory compliance and novel technologies that may require an expansion of regulatory scope.
New central functions to support the framework
9. Do you agree that the functions outlined in section 3.3.1 would benefit our AI regulation framework if delivered centrally?
26. Nearly all respondents agreed that central delivery of the proposed functions would benefit the framework, with many arguing centralised activities would allow the government to monitor and iterate the framework. Many suggested that feedback from regulators, industry, academia, civil society, and the general public should be used to measure effectiveness, with some calling for regular review points to assess whether the central function remained fit for purpose. A few respondents were concerned that some of the proposed activities may already be carried out by other organisations and suggested mapping existing work to avoid duplication.
10. What, if anything, is missing from the central functions?
27. While respondents widely supported the proposed central functions, many wanted to see more detail on the delivery of each activity, with some respondents endorsing a stronger emphasis on engagement and partnerships with existing organisations.
28. Responses highlighted the importance of addressing AI-related risks and building public trust in AI technologies. Some respondents suggested that the government should prioritise the proposed risk function, noting the importance of identifying and assessing risks related to AI. Respondents noted that this risk analysis should include ethical risks, such as bias, and systemic risks to society, such as changes to the labour market. A few respondents emphasised that the education and awareness function would be key to building public trust.
29. Respondents noted the importance of regulatory alignment across sectors and international regimes. Some respondents argued that the central functions should include more on interoperability, noting cyber security, disinformation, and copyright infringement as issues that will require international collaboration.
30. Some respondents suggested that some or all of the central functions should have a statutory underpinning or be delivered by an independent body. Respondents also stressed that, to be effective, the central functions should be adequately resourced and given the necessary technical expertise. This was identified as particularly important to the risk mapping, horizon scanning, and monitoring and evaluation functions.
31. Additional activities or functions suggested by respondents included: statutory powers to ensure the safety and security of highly capable AI models; coordination with the devolved administrations; and oversight of AI compliance with existing laws, including intellectual property and data protection frameworks.
11. Do you know of any existing organisations who should deliver one or more of our proposed central functions?
32. Overall, around a quarter of respondents felt that the government should deliver one or more of the central functions. Respondents also highlighted other organisations that could support the central functions, including regulators, technology-focused research institutes and think tanks, private-sector firms, and academic research groups. Many respondents advocated for the regulatory functions to build from the existing strengths of the UK’s regulatory ecosystem. Respondents noted that regulatory coordination initiatives like the Digital Regulation Cooperation Forum (DRCF) could help identify and respond to gaps in regulator remits. Respondents also highlighted that think tanks and research institutes such as the Alan Turing Institute, Ada Lovelace Institute, and Institute for the Future of Work have past or existing activities that may complement those described in the proposed central functions.
12. Are there additional activities that would help businesses confidently innovate and use AI technologies?
33. Many respondents felt the central functions could have further activities to support businesses to apply the principles to everyday practices related to AI. Respondents argued that the government and regulators should support industry with training programs and educational resources. Respondents noted that this support would be especially important for organisations operating across or between sectors.
34. Respondents felt that regulators should develop and regularly update guidance to allow business to innovate confidently. Respondents reported that incoherent and expensive compliance processes could stifle innovation and slow AI adoption.
35. Respondents suggested that the government could improve access to high-quality data, ensure international alignment on AI requirements, and facilitate collaboration between regulators, industry, and academia. Some respondents noted that responsible AI innovation is supported by access to high-quality, diverse, and ethically-sourced data. Respondents suggested that government-sponsored data trusts could help improve access to data. Some respondents saw the government playing a key role in ensuring the international harmonisation of AI regulation, noting that interoperability would promote trade and competition. A few respondents suggested that the government could facilitate collaboration between regulators, industry, and academia to ensure alignment between AI regulation, innovation, and research. A small number of respondents suggested introducing AI legislation rather than central functions to provide greater legal certainty.
12.1. If so, should these activities be delivered by government, regulators, or a different organisation?
36. While respondents identified some activities to support businesses to confidently innovate and use AI technologies that should be led by regulators, a majority of respondents suggested that these activities should be delivered by the government.
13. Are there additional activities that would help individuals and consumers confidently use AI technologies?
37. Respondents prioritised transparency from the cross-sectoral principles, with nearly half arguing that individuals and consumers should be able to identify when and how AI is being used by a service or organisation.
38. Many respondents felt that education and training would build public trust in AI technologies and help accelerate adoption. Respondents emphasised that AI literacy should be improved through education and training that enables consumers to use AI products and services more effectively. Respondents suggested training should cover all stages of the AI life cycle and build understanding of AI benefits as well as AI risks. Respondents stated that, along with the government and regulators, education, consumer, and advocacy organisations should help make knowledge accessible.
39. Some respondents wanted to see clearer routes for consumers to contest or seek redress for AI-related harms. Some emphasised the importance of adequate data protection measures. A few respondents noted that AI specific legislation would provide legal certainty and help foster public trust.
13.1. If so, should these activities be delivered by the government, regulators, or a different organisation?
40. While most respondents recommended that the government, regulators, industry, and civil society work together to help individuals and consumers confidently use AI technologies, nearly half of respondents suggested that activities to improve consumer confidence in AI should be delivered by the government.
14. How can we avoid overlapping, duplicative, or contradictory guidance on AI issued by different regulators?
41. Many respondents suggested the proposed central functions would be the most effective mechanism to avoid overlapping, duplicative, or contradictory guidance. Respondents noted that the central functions would support regulators by identifying cross-sectoral risks, facilitating consistent risk management actions, providing guidance on cross-sectoral issues, and monitoring and evaluating the framework as a whole.
42. While respondents stressed that consistent implementation of the framework across remits would require regulatory coordination, there was no agreement on the best way to achieve this. Some suggested establishing a new AI regulator, a few proposed appointing an existing regulator as the ‘lead regulator’, and others endorsed voluntary regulatory coordination measures, emphasising the role of regulatory fora such as the Digital Regulation Cooperation Forum (DRCF).
43. Some respondents suggested that horizontal cross-sector standards and assurance techniques would encourage consistency across regulatory remits, sectors, and international jurisdictions. Respondents recommended clarifying the specific remits of each regulator in relation to AI to promote coherence across the regulatory landscape. A few argued that introducing AI legislation, including putting the AI principles and regulatory coordination into statute, would prevent regulatory divergence.
Monitoring and evaluation of the framework
15. Do you agree with our overall approach to monitoring and evaluation?
44. Over half of respondents agreed with the overall approach to monitoring and evaluation set out in the AI regulation white paper. Many commended the proposals for a feedback loop and advised that industry, regulators, and civil society should be engaged to help measure the effectiveness of the framework. Respondents broadly supported an iterative approach and some suggested consulting industry as part of a regular evaluation to assess and adapt the framework. A few respondents advocated for findings from framework evaluations to be publicly available.
45. Some respondents stated that there was not enough detail or that the approach to monitoring and evaluation was unclear. To determine the practicality of the approach, respondents requested more information about the format, frequency, and sources of data that will be developed and used. Some of these respondents stressed the importance of identifying issues with the framework in a timely way. Respondents emphasised that AI risks will need to be continuously monitored, noting that more clarity and transparency is needed on how risks will be escalated and addressed.
16. What is the best way to measure the impact of our framework?
46. Many respondents suggested a data driven approach to measuring the impact of the framework would be most effective. Respondents recommended qualitative and quantitative data collection, impact assessments, and key performance indicators (KPIs). Examples of possible KPIs included consumer trust and satisfaction, rate of innovation, time to market, complaints and adverse events, litigation, and compliance costs. A few respondents suggested using economic growth to measure the impact of the framework. A couple wanted to see measurements tailored to specific sectors and suggested that the government engage with regulators to understand how they measure regulatory impacts on their respective industries.
47. Just over a quarter of respondents recommended that the government maintain a close dialogue with industry, civil society, and international partners. Respondents repeatedly stressed the importance of gathering a holistic view on impact with many noting that the government should engage with stakeholders who can offer different perspectives on the framework’s efficacy, including start-ups and small businesses. Respondents felt that broad consultation to gather evidence on public attitudes towards the framework and AI more generally would also be useful.
48. Respondents suggested that international interoperability should be monitored to ensure that the framework allows businesses to trade with and develop products for international markets. Some respondents suggested referencing established indicators and frameworks, such as the United Nations Sustainable Development Goals and the Five Capitals, to inform a set of qualitative and quantitative measures.
17. Do you agree that our approach strikes the right balance between supporting AI innovation; addressing known, prioritised risks; and future-proofing the AI regulation framework?
49. Half of respondents agreed that the approach strikes the right balance between supporting AI innovation; addressing known, prioritised risks; and future-proofing the AI regulation framework. However, some respondents were concerned that the approach would not be able to keep pace with the technological development of AI, stating that adequate future proofing of the framework will depend on retaining flexibility and adaptability when implementing the principles. Respondents wanted greater clarity on the specific areas to be regulated and stressed that regulators need to be proactive in identifying the risk of harm.
50. Over a third of respondents disagreed. Respondents were concerned that the framework does not clearly allocate responsibility for AI outcomes. Some thought that the focus on AI innovation, economic growth, and job creation would prevent a sufficient focus on AI-related risks, such as bias and discrimination.
Regulator capabilities
18. Do you agree that regulators are best placed to apply the principles and the government is best placed to provide oversight and deliver central functions?
51. Nearly all respondents agreed that regulators are best placed to implement the principles and that the government is best placed to provide oversight and deliver the central functions.
52. While respondents noted that regulators’ domain-specific expertise would be key to the effective tailoring of the cross-sectoral principles to sector needs, some also suggested that the government should support regulators to manage AI risks within their remits by building their technical AI skills and expertise.
53. Some respondents argued that the government would need to work closely with regulators to provide effective oversight of the framework and delivery of the central functions. Some also endorsed further collaboration between regulators. A few felt that the government’s oversight of the framework should be open and transparent, advocating for input from industry and civil society.
54. Some respondents were concerned that no current bodies were best placed to support the implementation and oversight of the proposed framework, with a few asking for AI legislation and a new AI regulator.
19. As a regulator, what support would you need in order to apply the principles in a proportionate and pro-innovation way?
55. While regulators that responded to this question supported the proposed framework, just over a quarter argued that the key challenge to proportionate and pro-innovation implementation would be coordination. Regulators saw value in sharing best practices to aid consistency and build existing knowledge into sector-specific approaches. Many suggested that strong mechanisms to share information between regulators and the proposed central functions would help avoid duplicate requirements across multiple regulators.
56. Regulators that responded to this question reported inconsistent AI capabilities, with over a quarter asking for further support in technical expertise and others demonstrating advanced approaches to addressing AI within their remits. Regulators identified common capability gaps including a lack of technical AI knowledge and limited understanding of where and how AI is used by those they regulate. Some suggested that government support in building internal organisational capacity would help them to effectively apply the principles within their existing remits, with some noting that they struggle to compete with the private sector to recruit the right technical expertise and skills. A couple of regulators highlighted how initiatives such as the government-funded Regulators’ Pioneer Fund have already allowed them to develop approaches to responsible AI innovation in their remits. Two regulators reported that the scope of their existing statutory remits and powers in relation to AI is unclear. These regulators asked for further details on how the central function would ensure that regulators used their powers and remits in a coherent way as they apply the principles.
20. Do you agree that a pooled team of AI experts would be the most effective way to address capability gaps and help regulators apply the principles?
57. Over three quarters of respondents agreed that a pooled team of AI experts would be the most effective way to build common capability and address gaps. Respondents felt that a team of pooled AI experts could help regulators to understand AI and address its unique characteristics within their sectors, supporting the consistent application of the principles across remits.
58. While respondents supported increasing regulators’ access to AI expertise, many stressed that a pooled team would need to contain diverse and multi-disciplinary perspectives. Respondents felt the pooled team should bring together technical AI expertise with sector-specific knowledge, industry specialists, and civil society to ensure that regulators are considering a broad range of views in their application of the principles.
59. Some respondents stated that a pool of experts would be insufficient and suggested that in-house regulator capability with sector-specific expertise should be prioritised.
Tools for trustworthy AI
21. Which non-regulatory tools for trustworthy AI would most help organisations to embed the AI regulation principles into existing business processes?
60. There was strong support for the use of technical standards and assurance techniques, with respondents agreeing that both would help organisations to embed the AI principles into existing business processes. Many respondents praised the UK AI Standards Hub and the Centre for Data Ethics and Innovation’s (CDEI) work on AI assurance. While some respondents noted that businesses would have a smaller compliance burden if tools and processes were consistent across sectors, others noted the importance of additional sector-specific tools and processes. Respondents also suggested supplementing technical standards with case studies and examples of good practice.
61. Respondents argued that standardised tools and techniques for identifying and mitigating potential risks related to AI would also support organisations to embed the AI principles. Some identified assurance techniques such as impact and risk assessments, model performance monitoring, model uncertainty evaluations, and red teaming as particularly helpful for identifying AI risks. A few respondents recommended assurance techniques that can be used to detect and prevent issues such as drift to mitigate risks related to data. While commending the role of tools for trustworthy AI, a few respondents also expressed a desire for more stringent regulatory measures, such as statutory requirements for high risk applications of AI or a watchdog for foundation models.
62. Respondents felt that tools and techniques such as fairness metrics, transparency reports, and organisational AI ethics guidelines can support the responsible use of AI while growing public trust in the technology. Respondents expressed the desire for third-party verification of AI models through bias audits, consumer labelling schemes, and external certification against technical standards.
63. A few respondents noted the benefits of international harmonisation across AI governance approaches for both organisations and consumers. Some endorsed interoperable technical standards for AI, commending international standards development organisations (SDOs) such as the International Organisation for Standardisation (ISO) and Institute of Electrical and Electronics Engineers (IEEE). Others noted the strength of a range of international work on AI including that by individual countries, such as the USA’s National Institute of Standards and Technology (NIST) AI Risk Management Framework (RMF) and Singapore’s AI Verify Foundation, along with work on international governance by multilateral bodies such as the Organisation for Economic Co-operation and Development (OECD), United Nation (UN), and G7.
Final thoughts
22. Do you have any other thoughts on our overall approach? Please include any missed opportunities, flaws, and gaps in our framework.
64. Some respondents felt that the AI regulation framework set out in the white paper would benefit from more detailed guidance on AI-related risks. Some wanted to see more stringent measures for severe risks, particularly related to the use of AI in safety-critical contexts. Respondents suggested that the framework would be clearer if the government provided risk categories for certain uses of AI such as law enforcement and places of work. Other respondents stressed that AI can pose or accelerate significant risks related to privacy and data protection breaches, cyberattacks, electoral interference, misinformation, human rights infringements, environmental sustainability, and competition issues. A few respondents were concerned about the potential existential risk posed by AI. Many respondents felt that AI technologies are developing faster than regulatory processes.
65. Respondents argued that the success of the framework relies on sufficient coordination between regulators in order to provide a clear and consistent approach to AI across sectors and markets. Respondents also noted that different sectors face particular AI-related benefits and risks, suggesting that the framework would need to balance the consistency provided by cross-sector requirements with the accuracy of sector-specific approaches. In particular, respondents flagged that any new rules or bodies to regulate AI should build from the existing statutory remits of regulators and relevant regulatory standards. Respondents also noted that regulators would need to be adequately resourced with technical expertise and skills to implement the framework effectively.
66. Respondents consistently emphasised that effective AI regulation relies on international harmonisation. Respondents suggested that the UK should work towards an internationally aligned regulatory ecosystem for AI by developing a gold standard framework and promoting best practice through key multilateral channels such as the OECD, UN, G7, and G20. Respondents noted that divergent or overlapping approaches to regulating AI would cause significant compliance burdens. Respondents argued that international cooperation can support responsible AI innovation in the UK by creating clear and certain rules that allow investments to move across multiple markets. Respondents also suggested establishing bilateral working groups with key strategic partners to share expertise. Some respondents stressed that the UK’s pro-innovation approach should be delivered at pace to remain competitive with a fast moving international landscape.
Legal responsibility for AI
L1. What challenges might arise when regulators apply the principles across different AI applications and systems? How could we address these challenges through our proposed AI regulatory framework?
67. Respondents felt that there were two core challenges for regulators applying the principles across different AI applications and systems: a lack of clear legal responsibility across complicated AI life cycles and issues with coordination across regulators and sectors.
68. Over a quarter of respondents felt it was not clear who would be held liable for AI-related risks. Some respondents raised a further concern about confusing interactions between the framework and existing legislation.
69. While nearly half of respondents were concerned about coordination and consistency across sectors and regulatory remits, some indicated that a solution (and the strength of the framework) lay in a context-based approach. Respondents asked for sector-based guidance from regulators, compliance tools, and regulator engagement with industry.
70. Many respondents suggested introducing statutory requirements or centralising the framework within a single organisational body, but there was no consensus over whether this centralisation should take the form of a lead regulator, central regulator, or coordination function. Some respondents suggested mandating industry transparency or third-party audits.
71. Respondents also raised a lack of international standards and agreements as a challenge, pointing to the importance of international alignment and collaboration.
L2.i. Do you agree that the implementation of our principles through existing legal frameworks will fairly and effectively allocate legal responsibility for AI across the life cycle?
72. While some respondents somewhat agreed that the principles would allocate legal responsibility for AI fairly and effectively through existing legal frameworks, most respondents either disagreed or neither agreed nor disagreed. Many respondents stated that it is not clear how the AI regulation principles would be implemented through existing legal frameworks. Respondents voiced concerns about gaps in existing legislation including intellectual property, legal services, and employment law. Some respondents stated that intellectual property rights needed to be affirmed and clarified to improve legal responsibility for AI. A few respondents noted the need for the AI framework to monitor and adapt as the technology advances and becomes more widely used. One respondent noted that the burden of liability falls at the deployer level and suggested that it would be essential to address information gaps in the AI life cycle to improve the allocation of legal responsibility.
L.2.ii. How could it be improved, if at all?
73. Many respondents felt that the framework needed to further clarify liability across the AI life cycle. In particular, respondents repeatedly noted the need for a legally responsible person for AI and some suggested a model similar to Data Protection Officers.
74. Over a quarter of respondents stated that new AI legislation or regulator powers would be necessary to effectively allocate liability across the life cycle. Some named specific measures that would need statutory underpinning, with a few advocating for licensing and pre-approvals and a couple suggesting a moratorium on the most advanced AI.
75. Others felt that it would be best to clarify legal responsibility for AI according to existing frameworks. Respondents wanted clarity on how the principles would be applied with or through existing law, with some suggesting that regulatory guidance would provide greater certainty.
76. Respondents also suggested that non-statutory measures such as enhancing technical regulator capability, domestic and international standards, and assurance techniques would help fairly and effectively allocate legal responsibility across the AI life cycle.
77. Others noted that the proposed central functions, including risk assessment, horizon scanning, and monitoring and evaluation, would be key to ensuring that legal responsibility for AI was fairly and effectively distributed across the life cycle as AI capabilities advance and become increasingly used.
L3. If you are a business that develops, uses, or sells AI, how do you currently manage AI risk including through the wider supply chain? How could government support effective AI-related risk management?
78. Nearly half of respondents to this question told us that they had implemented risk assessment processes for AI within their organisation. Many used existing best practice processes and guidance from their sector or trade bodies such as techUK. Some felt that the proliferation of different organisational risk assessment processes reflected the absence of overarching guidance and best practice from the government. Of these respondents, many suggested that it would be useful for businesses to understand the government’s view on AI-related best practices, with some recommending a central guide on using AI safely.
79. Many respondents noted their compliance with existing legal frameworks that capture AI-related risks, such as product safety and personal data protections. Respondents highlighted that any future AI measures should avoid duplicating or contradicting existing rules and laws.
80. Respondents consistently stressed the importance of transparency, with some highlighting information sharing tools like model cards. Similarly to Q2, some respondents suggested that labelling AI use would be beneficial to users, particularly in regard to building literacy around potentially malicious AI generated content, such as deepfakes and disinformation. A few respondents argued that AI labelling can help shape expectations of a service and should be a consumer protection. Echoing answers to F1, respondents also mentioned that services should be transparent about the data used to train AI models so users can understand how tools and services work as well as their limitations.
81. Responses showed that the size of an organisation shaped the capacity to assess AI-related risks. While larger organisations mentioned that they engage with customers and suppliers to shape and share best practices, some smaller businesses asked for further support to assess AI-related risk and implement the AI principles effectively.
Foundation models and the regulatory framework
F1. What specific challenges will foundation models such as large language models (LLMs) or open-source models pose for regulators trying to determine legal responsibility for AI outcomes?
82. While respondents supported the AI regulation framework set out in the white paper, many were concerned that foundation models may warrant a bespoke regulatory approach. In particular, respondents noted that foundation models are characterised by their technical complexity and stressed their potential to underpin many different applications across multiple sectors. Nearly a quarter of respondents emphasised that foundation models make it difficult to determine legal responsibility for AI outcomes, with some sharing hypothetical use-cases where both upstream and downstream actors are at fault. Respondents stressed that technical opacity, complex supply chains, and information asymmetries prevent sufficient explainability, accountability, and risk assessment for foundation models.
83. Many respondents were concerned about the quality of the data used to train foundation models and whether training data is appropriate for all downstream model applications. Respondents stated that it was not clear whether data used to train foundation models complies with existing laws, such as those for data protection and intellectual property. Respondents noted that definitions and standards for training data were lacking. Respondents felt that data use could be improved through better information sharing measures, benchmark measurements and standards, and the clear allocation of responsibility to a specific actor or person for whether or not data is appropriate to a given application.
84. Some respondents emphasised the complexity of foundation model supply chains and argued that information asymmetries between upstream developers (with technical oversight) and downstream deployers (with application oversight) not only muddies legal responsibility for AI outcomes but also prevents sufficient risk monitoring and mitigation. While some respondents noted the concentrated market power of foundation model developers and suggested these actors were best positioned to mitigate related risks, others argued that developers would have limited sight of the risks linked to specific downstream applications. Many raised concerns about the lack of measures to rigorously judge the appropriateness of a foundation model to a given application.
85. A few respondents noted concerns regarding wider access to AI, including open source, leaking, or malicious use. However, a similar number of respondents noted the importance of open source to AI innovation, transparency, and trust.
F2. Do you agree that measuring compute provides a potential tool that could be considered as part of the governance of foundation models?
86. Half of respondents felt compute was an inadequate proxy for governance requirements, with many arguing that the fast pace of technological change would mean compute-related thresholds would be quickly outdated. However, nearly half somewhat agreed that measuring compute would be useful for foundation model governance, suggesting that it could be used to assess whether a particular AI model should follow certain requirements when used with other governance measures. A few respondents noted that measuring compute would be one way to capture the environmental impact of different AI models.
F3. Are there other approaches to governing foundation models that would be more effective?
87. There was wide support for governance measures and tools for trustworthy AI, with respondents advocating for the use of organisational governance, technical standards, and assurance techniques dedicated to foundation models.
88. Some respondents recommended assessing foundation model capabilities and applications rather than compute. Respondents felt that model verification measures, such as audits and evaluations, would be effective, with some suggesting these should be mandatory requirements. Some respondents noted the importance of downstream monitoring or post-market surveillance. One respondent suggested a pre-deployment sandbox.
89. A small number of respondents wanted to see statutory requirements on foundation models. A few endorsed moratoriums, bans, or limits on foundation models and uses. Others suggested using contracts, licences, and user agreements, with respondents also noting the importance of both physical and cyber security measures.
AI sandboxes and testbeds
S1. Which of the sandbox models described in section 3.3.4 would be most likely to support innovation?
90. While a large majority of respondents were strongly supportive of sandboxes in general, the “multiple sector, multiple regulator” (MSMR) and “single sector, multiple regulator” (SSMR) models were seen to most likely support innovation.
91. Over a third of respondents felt the MSMR model would support innovation, noting that the cross-sectoral basis would enable regulators to develop effective guidance in response to live issues, harmonise rules, coordinate implementation, ensure applicability to safety critical sectors, and identify complementary policy levers. Respondents suggested that a MSMR sandbox should tackle issues related to the implementation of the AI principles, including identifying and addressing any gaps in the framework, overlap with existing regulation, coordination challenges between sectors and regulators, and any blockers to effective implementation of the regulatory framework, such as regulator capacity. Respondents also stressed that the sandbox should be flexible and adaptable in order to future proof against new technological developments.
92. An equal number of respondents endorsed the SSMR model. Respondents noted that the SSMR and “multiple sector, single regulator” (MSSR) models would be easier to launch due to their more streamlined coordination across a single sector or regulator. For this reason, respondents felt that these models might drive the most immediate value. Some suggested that an initial single sector or single regulator sandbox could be adapted into a MSMR model as work progressed in order to capture the benefits of both models.
S2. What could the government do to maximise the benefit of sandboxes to AI innovators?
93. Some respondents argued that the sandbox should be developed and delivered in collaboration with businesses, regulators, consumer groups, and academics and other experts. Respondents suggested building on the existing strengths of the UK regulatory landscape, such as facilitating cross-sector learnings through the Digital Regulation Cooperation Forum (DRCF).
94. Respondents stated that the sandbox should develop guidance, share information and tools, and provide support to AI innovators. In particular, respondents said that information about opportunities for involvement should be shared and noted that sharing outcomes would encourage wider participation. Respondents wanted the sandbox to be open and transparent, with many advocating for sandbox processes, regulatory assessments and reports, decision processes, evidence reviews, and subsequent findings to be made available to the public. Respondents suggested that regular reports and guidance from the sandbox would inform innovators and future regulation by creating “business-as-usual” processes. Respondents felt that measures should be taken to make the sandbox as accessible as possible, with a few advocating for dedicated pathways and training for smaller businesses.
95. Respondents felt that the sandbox should be used to inform and develop technical standards and assurance techniques that can be widely used. A few mentioned that this would help promote best practice across industry. Others noted that, to be most beneficial, the sandbox should be well aligned with wider regulation for AI. Respondents also noted that a sandbox presents an opportunity for the UK to demonstrate global leadership in AI regulation and technical standards by sharing findings and best practices internationally.
96. Respondents noted that the sandbox could support innovation by providing market advantages, such as product certification, to maximise the benefits to AI innovators. Other financial incentives suggested by respondents included innovation grants, tax credits, and free or funded participation in supervised test environment sandboxes. A few stakeholders agreed that funding would help start-ups and smaller businesses with less organisational resources to participate in research and development focused sandboxes. Respondents suggested that the sandbox could collaborate with UK and international investment companies to build opportunities for participating companies.
S3. What could the government do to facilitate participation in an AI regulatory sandbox?
97. Some respondents suggested that grants, subsidies, and tax credits would encourage participation by smaller businesses and start-ups in resource-intensive, research and development focused sandbox models such as supervised test environments.
98. Respondents endorsed a range of incentives to facilitate participation in different sandbox models including access to standardised and anonymised datasets, and accreditation schemes that would show alignment with regulatory requirements and help gain market access. There was some support for innovation competitions that would help select participants.
99. Similarly to S2, respondents agreed that collaboration and consultation with a range of stakeholders would help facilitate broad participation. Respondents suggested research centres, accelerator programmes, and university partnerships. There was support for a diverse group of stakeholders to be involved in the early stages of sandbox development, especially to identify regulatory areas with high risk. There was some support for harmonised evaluation frameworks across sectors to reduce regulatory burden and encourage wider interest from prospective stakeholders. One respondent proposed a dedicated online platform that would provide access to relevant guidance and provide a portal for submitting and tracking applications along with a community forum.
100. There was broad support for a simple application process with clear guidelines, templates, and information on eligibility and legal requirements. Respondents expressed support for clear entry and exit criteria, noting the importance of reducing the administrative burden on smaller businesses and start-ups to lower the barrier to entry.
S4. Which industry sectors or classes of product would most benefit from an AI sandbox?
101. While there was no overall consensus on a specific sector or class of product that would most benefit from an AI sandbox, respondents identified two “safety-critical” sectors with a high-degree of potential risk: healthcare and transport. Respondents noted that these sectors are characterised by an inability for real-world testing and would benefit from an AI sandbox. Respondents noted the potential to enhance healthcare outcomes, patient safety, and compliance with patient privacy guidelines by fostering innovation in areas such as diagnostic tools, personalised medicine, drug discovery, and medical devices. Other respondents noted the rise of autonomous vehicles and intelligent transportation systems along with significant enthusiasm from industry to test the regulatory framework.
102. Some respondents suggested that financial services and insurance would benefit from an AI sandbox due to heavy investment from the sector in automation and AI. Respondents also noted that financial services and insurance are also overseen by multiple regulators, including the Information Commissioner’s Office (ICO), Prudential Regulation Authority (PRA), Financial Conduct Authority (FCA), and The Pensions Regulator (TPR). Respondents noted that financial services could leverage an AI sandbox to explore AI-based applications for risk assessment, fraud detection, algorithmic trading, and customer service.
103. It was noted by one respondent that the nuclear sector is currently already benefiting from an AI sandbox. The Office for Nuclear Regulation (ONR) and the Environment Agency (EA) have taken the learnings from their own regulatory sandbox to develop the concept of an international AI sandbox for the nuclear sector.
Annex D: Summary of impact assessment evidence
This annex provides a summary of the written evidence we received in response to our consultation on the AI regulation impact assessment[footnote 124]. We asked eight questions including seven open or semi-open questions that received a range of written reflections. We asked:
- Do you agree that the rationale for intervention comprehensively covers and evidences current and future harms?
- Do you agree that increased trust is a significant driver of demand for AI systems?
- Do you have any additional evidence to support the following estimates and assumptions across the framework?
- Do you agree with the estimates associated with the central functions?
- Are you aware of any alternative metrics to measure the policy objectives?
- Do you believe that some AI systems would be prohibited in Options 1 and 2, due to increased regulatory scrutiny?
- Do you agree with our assessment of each policy option against the objectives?
- Do you have any additional evidence that proves or disproves our analysis in the impact assessment?
In total we received 64 written responses on the impact assessment consultation from organisations and individuals. The method of our analysis is captured in Annex A and a summary of responses to these questions follows below.
Question 1: Do you agree that the rationale for intervention comprehensively covers and evidences current and future harms?
Summary of responses:
More than half of respondents disagreed that the rationale for intervention comprehensively covers evidence of current and future harms. Nearly half of respondents stated that not all risks are adequately addressed. Many of these respondents argued that the rationale does not account for unexpected harms or existential and systemic risks. One respondent argued that the rationale does not consider the impact of AI on human rights. Another respondent suggested that there should be mandatory requirements for the ethical collection of data and another advocated for pre-deployment measures to mitigate AI risks.
Over a quarter of respondents suggested analysing risks and opportunities for each sector. These respondents often argued that the potential harms and benefits in different industries are not accounted for, such as the impact of AI on jobs.
Some respondents advocated for the government to build the evidence on current and future harms as well as potential interventions. Many of these respondents emphasised the importance of including diverse perspectives and the public voice when conducting research and regulating AI.
A few respondents noted that the government and regulators should adopt a flexible approach that monitors and can adapt to technological developments.
A few respondents stated that excessive regulation and government intervention will stifle innovation instead of encouraging it. These respondents argued that there needs to be a balance between mitigating risks and enabling the benefits of AI.
One respondent stated that there should be an independent regulator for AI.
Question 2: Do you agree that increased trust is a significant driver of demand for AI systems?
Summary of responses:
Over half of respondents agreed that trust is a significant driver of demand for AI systems. However, around a quarter disagreed and some remained unsure.
Over a third of respondents gave a written answer that could provide further insight outside of agreeing or disagreeing. Of these, many respondents stressed that transparency, education, and governance measures (such as regulation and technical standards) increase trust. These ideas were reflected in both respondents who agreed and disagreed in trust driving demand for AI.
Respondents also argued that trust in AI could be reduced by concerns about bias or safety. Some of these respondents highlighted that unfair or untransparent bias in AI systems not only reduces trust but impacts already marginalised communities the most. Some respondents argued that prioritising innovation over trust in a regulatory approach would reduce trust.
Of the respondents that disagreed that trust was a driver of AI uptake and provided further written responses, two main themes emerged. First, that demand for AI is driven by economic and financial incentives and, second, that it is driven by technological developments. For example, one respondent highlighted that AI could increase productivity and thus the profitability of companies. Respondents also highlighted technological developments as a driver for AI demand, with two respondents stating that companies’ “fear of missing out” in new technologies could drive their demand for AI systems.
Respondents that disagreed often suggested that increasing AI demand and adoption comes at the cost of safeguarding the public and risk mitigation.
Question 3: Do you have any additional evidence to support the following estimates and assumptions across the framework?
Summary of responses:
Respondents reacted to each statement differently. There was a mixed amount of agreement across all statements. In written feedback, some respondents suggested that our estimates and assumptions depend on complex factors or that it is not possible to provide estimates about AI due to too many uncertainties.
For the first estimation, that 431,671 businesses will be impacted by adopting/consuming AI less than the estimated 3,170 businesses supplying/producing AI, disagreeing respondents found that it understates the number of businesses that will likely be affected by AI, that the number can rapidly change as it is easy to integrate AI into a product or service, that the division between AI adopters and producers is somewhat artificial, and that consumers should also be considered.
For the second statement, saying that those who adopt/consume AI products and services will face lower costs than those who produce and/or supply AI solutions products and services, there was some disagreement and one response that agreed. Those who disagreed with the statement argued that consumers of AI will have lower costs than producers of AI since consumers and users more widely can face (increasing) costs of using AI applications. On the other hand, one respondent mentioned that cost savings will apply to users without a deep understanding of the technology and producers will face high salary costs because of a small pool of labour talent able to operate advanced AI systems.
Concerning the third estimate of familiarisation costs (here referring to the cost of businesses upskilling employees in new regulation) landing in the range of £2.7 million to £33.7 million, a couple of respondents that disagreed stated that familiarisation costs could vary from business to business. These respondents argued the current range was understating the full costs and recommended considering other costs. Some suggested that consumers need to be trained on residual risk and how to overcome automation bias. Others mentioned that the independent audit of AI systems will create many new highly-trained jobs.
Finally, on the fourth estimation that compliance costs (here reflecting the cost of businesses adjusting business elements to comply with new standards) will land in the range of £107 million to £6.7 billion, there was further disagreement. Some respondents said that compliance costs should be as low as possible, but there was no agreement on how best to achieve this. Other respondents stated that companies will not comply and that compliance would necessitate new business activities.
Question 4: Do you agree with the estimates associated with the central functions?
Summary of responses:
A slight majority of respondents somewhat disagreed with the estimates outlined in the AI regulation impact assessment, suggesting that central function estimates are too high. Some respondents mentioned that the central function could deploy AI and use automation to harness efficiency and drive down cost estimates. Two respondents also highlighted that the central function could employ techniques such as peer-to-peer learning and networks to drive down cost estimates.
On the other hand, some respondents indicated that central function estimates are too low. Some respondents believe that the current estimates are too low because they do not account for costs associated with late upskilling of central function employees. One respondent suggested that the increasing demand for AI from the commercial sector would raise costs further, and create challenges in the central function accessing AI solutions due to inflationary cost pressure. Some respondents suggested that the expanding scale and capabilities of AI would require a larger central function to regulate the technology, arguing current costs are likely to be conservative estimates.
A few respondents did agree that the estimates are accurate. However, many noted that it would be a challenge to pin a specific number to the estimates associated with the central function, and suggested that a lack of clarity in defining terms made it difficult to assess accuracy of the estimates.
Question 5: Are you aware of any alternative metrics to measure the policy objectives?
Summary of responses:
More than a third of respondents suggested alternative metrics that could be used to measure the policy objectives. Some suggestions included tracking the number of models being audited for bias and fairness; the number of AI-related incidents being reported and investigated; and metrics related to the framework’s operation such as the number of regulators publishing guidance, the nature of guidance and associated outcomes for organisations that have adopted it, or sentiment indicators from stakeholders. Other suggestions included tracking public trust and acceptance of AI systems.
Almost a quarter of respondents suggested existing frameworks and models. A couple of respondents suggested that effective assessment and regulation of harm would be key to measuring the policy objectives.
Question 6: Do you believe that some AI systems would be prohibited in options 1 and 2 due to increased regulatory scrutiny?
Summary of responses:
Over half of respondents agreed that some AI systems would be prohibited in options 1 and 2 due to increased regulatory scrutiny. Around a quarter of respondents disagreed and just under a third were unsure.
Of respondents that expanded on their thoughts, a third suggested that some AI systems present a threat to society and should be prohibited. These respondents emphasised that prohibition would reduce AI risks and saw prohibition as a positive impact. Some suggested that a lack of any prohibition would represent a failure of the regulatory framework.
Some stakeholders suggested that some AI systems would be prohibited. However, a similar amount suggested that the regulatory scrutiny under options 1 and 2 would not be sufficient enough to prohibit AI systems. These two sets of responses reflected conflicting understanding around the intensity of the proposed regulations, as opposed to inherent views on how regulation might impact the sector. A few indicated that the impact assessment was unable to provide enough evidence around which AI systems might be prohibited.
Question 7: Do you agree with our assessment of each policy option against the objectives?
Summary of responses:
Just over a third of respondents either strongly or somewhat agreed with the assessment of each policy option against objectives, with most responding that they somewhat agree. A similar amount either strongly or somewhat disagreed, with most of these responding that they only somewhat disagreed. Around a quarter of respondents neither agreed nor disagreed, or indicated they were unsure.
Question 8: Do you have any additional evidence that proves or disproves our analysis in the impact assessment?
Summary of responses:
Almost half of written responses suggested that the AI regulation impact assessment insufficiently estimated the impacts of AI. These respondents indicated that the impacts of AI are much larger and more harmful than is implied by the AI regulation impact assessment and white paper.
Just under a third indicated that the government should act quickly to regulate emerging AI technologies. These respondents emphasised that timely action should be a key focus for AI regulation given the quickly advancing capabilities of the technology.
Some respondents indicated that there was too great a degree of uncertainty to make accurate assessments. These respondents thought that any estimation would be inaccurate due to the nature of AI and the many uncertainties around future developments.
Some respondents suggested that regulators should harmonise their approach to AI, emphasising that the use of these technologies across sectors requires coordinated and consistent regulation.
-
United Kingdom Artificial Intelligence Market, US International Trade Administration, 2023. ↩
-
Frontier AI: capabilities and risks, Department for Science, Innovation and Technology, 2023. ↩
-
New advisory service to help businesses launch AI and digital innovations, Department for Science, Innovation and Technology, 2023. ↩
-
To support the government’s planning and policy development, and given the material uncertainties that exist, the Government Office for Science has prepared a foresight report outlining possible scenarios that may arise in the context of AI development, proliferation and impact in 2030. See: Future risks of frontier AI (Annex A), Government Office for Science, 2023. A full report on the scenarios will be published shortly (this report will not be a statement of government policy). ↩
-
Prime Minister’s speech on AI: 26 October 2023, Prime Minister’s Office, 10 Downing Street, 2023. ↩
-
International Science Partnerships Fund, UKRI, 2023. ↩
-
How should AI systems behave, and who should decide?, OpenAI, 2023. ↩
-
Safety and Security Risks of Generative Artificial Intelligence to 2025, Government Office for Science, 2023. ↩
-
We provide further detail on this area as part of our description of the cross-sectoral safety, security and robustness principle in the AI regulation white paper. See: AI regulation: a pro-innovation approach, Department for Science, Innovation and Technology, 2023. ↩
-
Frontier AI: capabilities and risks, Department for Science, Innovation and Technology, 2023. ↩
-
Large dedicated AI companies make a major contribution to the UK economy, with GVA (gross value added) per employee estimated to be £400,000, more than double that of comparable estimates of large dedicated firms in other sectors. See: AI Sector Study 2022, Department for Science, Innovation and Technology, 2023. ↩
-
The Global AI Index Tortoise Media, 2023. ↩
-
AI regulation: a pro-innovation approach, Department for Science, Innovation and Technology, 2023. ↩
-
AI regulation: a pro-innovation approach – policy proposals, Department for Science, Innovation and Technology, 2023. ↩
-
Frontier AI: capabilities and risks, Department for Science, Innovation, and Technology, 2023. ↩
-
Race towards ‘autonomous’ AI agents grips Silicon Valley, Anna Tong and Jeffrey Dastin, 2023; Introducing superalignment, Jan Leike and Ilya Sutskever (OpenAI), 2023; AI could be one of humanity’s most useful inventions, Google Deepmind, n.d.. ↩
-
Employment Outlook 2023: artificial intelligence and jobs, OECD, 2023. ↩
-
Generative AI and the UK labour market, KPMG, 2023; The economic potential of generative AI: the next productivity frontier, McKinsey, 2023; What drives UK firms to adopt AI and robotics, and what are the consequences for jobs?, Institute for the Future of Work, 2023. ↩
-
ChatGPT is the fastest growing app in the history of web applications, Cindy Gordon, 2023. ↩
-
Using AI to monitor trackside Britain’s wildlife, Zoological Society London, 2023. ↩
-
A foundation model for generalizable disease detection from retinal images, Esma Aïmeur et al., 2023. ↩
-
Synthetic lies: understanding AI-generated misinformation and evaluating algorithmic and human solutions, Jiawei Zhou et al., 2023; Fake news, disinformation and misinformation in social media: a review, Yukon Zhou et al., 2023; AI could create a perfect storm of climate misinformation, Victor Galaz et al., 2023. ↩
-
Dual use of artificial-intelligence-powered drug discovery, Fabio Urbina et al., 2022. ↩
-
AI regulation: a pro-innovation approach, Department for Science, Innovation and Technology, 2023. ↩
-
AI Foundation Models: initial review, CMA, 2023. ↩
-
How do we ensure fairness in AI?, ICO, 2023. ↩
-
Software and AI as a Medical Device Change Programme – Roadmap, MHRA, updated 2023 [2021]. ↩
-
The government has written to the Office of Communications (Ofcom); Information Commissioner’s Office (ICO); Financial Conduct Authority (FCA); Competition and Markets Authority (CMA); Equality and Human Rights Commission (EHRC); Medicines and Healthcare products Regulatory Agency (MHRA); Office for Standards in Education, Children’s Services and Skills (Ofsted); Legal Services Board (LSB); Office for Nuclear Regulation (ONR); Office of Qualifications and Examinations Regulation (Ofqual); Health and Safety Executive (HSE); Bank of England; and Office of Gas and Electricity Markets (Ofgem). The Office for Product Safety and Standards (OPSS), which sits within the Department for Business and Trade, has also been asked to produce an update. Regulators will be best placed to determine the form and substance of their update and we encourage all regulators that consider AI to be relevant to their work to publish their approaches. As we continue to implement the framework and assess regulator readiness, our prioritisation of regulators may change to reflect evolving factors such as our risk analysis. We will also work with other regulators and encourage the publication of action plans to drive transparency across the wider ecosystem. ↩
-
Response to Professor Dame Angela McLean’s Pro-Innovation Regulation of Technologies Review: Cross Cutting, HM Treasury, 2023. ↩
-
£250 million to secure the UK’s world-leading position in technologies of tomorrow, UKRI, 2023. ↩
-
Members of the DRCF include the CMA, ICO, FCA, and Ofcom. See: New advisory service to help businesses launch AI and digital innovations, Department for Science, Innovation and Technology, 2023. ↩
-
The AI Standards Hub, AI Standards Hub, 2022. ↩
-
Portfolio of AI Assurance Techniques, Department for Science, Innovation and Technology, 2023. ↩
-
Public attitudes to data and AI: Tracker survey (Wave 3), Department for Science, Innovation and Technology, 2023. ↩
-
We have previously categorised these as societal harms; misuse risks; and loss of control. See: Frontier AI: capabilities and risks, Department for Science, Innovation and Technology, 2023. ↩
-
Bridging Responsible AI Divides, BRAID UK, 2024. ↩
-
AI Skills for Business Guidance: Feedback Consultation Call from The Alan Turing Institute, Innovate UK, 2023. ↩
-
A recent study by the Institute for the Future of Work shows that the net impact on skills and job creation for UK firms that have adopted AI and robotics technologies is positive. However, these positive impacts on jobs and job quality are associated with the levels of readiness within a firm. See: What drives UK firms to adopt AI and robotics, and what are the consequences for jobs?, Institute for the Future of Work, 2023. ↩
-
The impact of AI on UK jobs and training, Department for Education, 2023. ↩
-
Apprenticeships are for people aged 16 and over who are not in full time education. See: Find an apprenticeship, Department for Education, n.d.. ↩
-
Skills Bootcamps are for adults aged 19 and over. See: Find a skills bootcamp, Department for Education, 2024 [2022]. ↩
-
Lifelong Learning Entitlement overview, Department for Education, 2024. ↩
-
A world-class education system: The Advanced British Standard, Department for Education, 2023. ↩
-
Creative Industries Sector Vision, Department for Culture, Media and Sport, 2023. ↩
-
Frontier AI: capabilities and risks, Department for Science, Innovation and Technology, 2023. ↩
-
Algorithmic discrimination in the credit domain: what do we know about it?, Ana Cristina Bicharra Garcia et al., 2023. ↩
-
Ethics and discrimination in artificial intelligence-enabled recruitment practices, Zhisheng Chen, 2023. ↩
-
Fairness Innovation Challenge, Department for Science, Innovation and Technology; Innovate UK, 2023. ↩
-
Guidance on AI and data protection, ICO, 2023. ↩
-
Covenant for Using Artificial Intelligence (AI) in Policing, National Police Chiefs’ Council, n.d.. ↩
-
Frontier AI: capabilities and risks, Department for Science, Innovation and Technology, 2023. ↩
-
Misinformation in action: Fake news exposure is linked to lower trust in media, higher trust in government when your side is in power, Katherine Ognyanova et al., 2020. ↩
-
Emerging Processes for Frontier AI Safety, Department for Science, Innovation and Technology, 2023. ↩
-
AI Foundation Models: initial review, CMA, 2023. ↩
-
Government AI Readiness Index 2023, Oxford Insights, 2023 ↩
-
Chancellor to cut admin workloads to free up frontline staff, HM Treasury; Home Office, 2023. ↩
-
£21 million to roll out artificial intelligence across the NHS, Department of Health and Social Care, 2023. ↩
-
Generative artificial intelligence in education call for evidence, Department for Education, 2023. ↩
-
Generative AI Framework for HMG, Cabinet Office and Central Digital and Data Office, 2024. ↩
-
Artificial Intelligence, National Cyber Security Centre, n.d.. ↩
-
Frontier AI: capabilities and risks, Department for Science, Innovation and Technology, 2023. ↩
-
Dual use of artificial-intelligence-powered drug discovery, Fabio Urbina et al., 2022. ↩
-
Frontier AI: capabilities and risks, Department for Science, Innovation and Technology, 2023. ↩
-
UK Biological Security Strategy, Cabinet Office, 2023 ↩
-
National vision for engineering biology, Department for Science, Innovation and Technology, 2023. ↩
-
Frontier AI: capabilities and risks, Department for Science, Innovation and Technology, 2023. ↩
-
Practices for Governing Agentic AI Systems, Yonadav Shavit et al., 2023. ↩
-
Frontier AI: capabilities and risks, Department for Science, Innovation and Technology, 2023. ↩
-
Race towards ‘autonomous’ AI agents grips Silicon Valley, Anna Tong and Jeffrey Dastin, 2023; Introducing superalignment, Jan Leike and Ilya Sutskever (OpenAI), 2023; AI could be one of humanity’s most useful inventions, Google Deepmind, n.d.. ↩
-
Future Risks of Frontier AI, Government Office for Science, 2023. ↩
-
Prime Minister’s speech on AI: 26 October 2023, Prime Minister’s Office, 10 Downing Street, 2023. ↩
-
Pause Giant AI Experiments: An Open Letter, Future of Life Institute, 2023. ↩
-
AI regulation: a pro-innovation approach, Department for Science, Innovation and Technology, 2023. ↩
-
We note, for instance, the enforcement action of the ICO who have used data protection law to hold organisations using AI systems that process personal data to account for breaches of data protection law. The CMA’s initial review of foundation models notes that accountability for obligations under competition and consumer law applies across the AI life cycle to both developers and deployers. See: AI Foundation Models: initial review, CMA, 2023. Similarly, the Medicines and Medical Devices Act 2021 gives the MHRA enforcement powers sufficient to hold manufacturers of medical devices accountable, including the power to require that unsafe devices are removed from the market. In addition, enforcement of serious non-compliance can, where appropriate, result in criminal prosecution through the courts. ↩
-
The same model may be deployed directly by the developer and also integrated into an almost limitless variety of systems, products and tools that will fall under the remit of multiple regulators. ↩
-
The law may allocate liability to “Quantum Talent Technologies” in this scenario if the actor has established an “agency” relationship according to equality law or was privately contractually obligated to abide by equality law. The law may also attribute liability along the supply chain in negligence if there is a duty of care that has been breached causing foreseeable damage. However, some laws only apply to actors based in the UK. In this scenario, data protection law would apply, allowing the ICO to take enforcement action for any failure by a relevant data controller (such as “Count Your Pennies Ltd”) to process personal data fairly and lawfully. ↩
-
Equality Act 2010: guidance, Government Equalities Office and Equality and Human Rights Commission, 2015 [2013]. ↩
-
Company Policies, AI Safety Summit, 2023. ↩
-
Emerging Processes for Frontier AI Safety, Department for Science, Innovation and Technology, 2023. ↩
-
Responsible capability scaling is an emerging framework to manage risks associated with highly capable AI and guide decision-making about AI development and deployment. See: Responsible Capability Scaling in Emerging Processes for Frontier AI Safety. Department for Science, Innovation and Technology, 2023. ↩
-
International expertise to drive International AI Safety Report, Department for Science, Innovation and Technology, 2024. ↩
-
To support the government’s planning and policy development, and given the material uncertainties that exist, the Government Office for Science has prepared a foresight report outlining possible scenarios that may arise in the context of AI development, proliferation and impact in 2030. See: Future risks of frontier AI (Annex A), Government Office for Science, 2023. A full report on the scenarios will be published shortly (this report will not be a statement of government policy). ↩
-
AI regulation: a pro-innovation approach, Department for Science, Innovation and Technology, 2023. ↩
-
UK International Technology Strategy, Foreign, Commonwealth & Development Office, 2023. ↩
-
Prime Minister’s speech on AI: 26 October 2023, Prime Minister’s Office, 10 Downing Street, 2023. ↩
-
The Bletchley Declaration by Countries Attending the AI Safety Summit, 1-2 November 2023, Department for Science, Innovation, and Technology; Foreign, Commonwealth and Development Office; Prime Minister’s Office, 10 Downing Street, 2023. ↩
-
World leaders, top AI companies set out plan for safety testing of frontier as first global AI Safety Summit concludes, Prime Minister’s Office, 10 Downing Street; Department for Science, Innovation and Technology, 2023. ↩
-
International expertise to drive International AI Safety Report, Department for Science, Innovation and Technology, 2024. ↩
-
G7 Leaders’ Statement on the Hiroshima AI Process, Ministry of Foreign Affairs Government of Japan, 2023. ↩
-
G20 New Delhi Leaders’ Declaration, Ministry of External Affairs Government of India, 2023 ↩
-
The 17 goals, United Nations, 2023. ↩
-
GPAI New Delhi Ministerial Declaration, Global Partnership on AI, 2023. ↩
-
OECD AI Principles overview, OECD, 2024. ↩
-
CDEI portfolio of AI assurance techniques, Centre for Data Ethics and Innovation; Department for Science, Innovation and Technology, 2023. ↩
-
Catalogue of tools and metrics for trustworthy AI, OECD, n.d.. ↩
-
Recommendation on the Ethics of Artificial Intelligence, UNESCO, 2023. ↩
-
Governing AI for Humanity, United Nations, 2023. ↩
-
The AI Standards Hub, AI Standards Hub, 2022. ↩
-
UK unites with global partners to accelerate development using AI, Foreign, Commonwealth & Development Office, 2023. ↩
-
International Science Partnerships Fund, UKRI, 2023. ↩
-
The Atlantic Declaration, Prime Minister’s Office, 10 Downing Street, Foreign, Commonwealth & Development Office, Department for Business and Trade, 2023.US ↩
-
The Hiroshima Accord: An enhanced UK-Japan global strategic partnership, Prime Minister’s Office, 10 Downing Street, 2023. ↩
-
The Downing Street Accord: A United Kingdom-Republic of Korea Global Strategic Partnership, Prime Minister’s Office, 10 Downing Street, 2023. ↩
-
Joint Declaration by the Prime Ministers of the Republic of Singapore and the United Kingdom of Great Britain and Northern Ireland on a Strategic Partnership, Prime Minister’s Office, 10 Downing Street, 2023. ↩
-
Guidance on AI and data protection, ICO, 2023. ↩
-
Developed by the Department for Science, Innovation and Technology (DSIT) and Central Digital and Data Office (CDDO) for the public sector. ↩
-
Emerging Processes for Frontier AI Safety, Department for Science, Innovation and Technology, 2023.databases. ↩
-
AI Foundation Models: initial review, CMA, 2023; Generative AI & Advertising: Decoding AI Regulation, ASA, 2023; What generative AI means for the communications sector, Ofcom, 2023. ↩
-
How do we ensure fairness in AI?, ICO, 2023; Software and Artificial Intelligence (AI) as a Medical Device, MHRA, updated 2023 [2021]. ↩
-
Response to Professor Dame Angela McLean’s Pro-Innovation Regulation of Technologies Review: Cross Cutting, HM Treasury, 2023. ↩
-
AI regulation: a pro-innovation approach, Department for Science, Innovation and Technology, 2023. ↩
-
CDEI portfolio of AI assurance techniques, Centre for Data Ethics and Innovation; Department for Science, Innovation and Technology, 2023. ↩
-
Fairness Innovation Challenge, Department for Science, Innovation and Technology; InnovateUK, 2023. ↩
-
Emerging Processes for Frontier AI Safety, Department for Science, Innovation and Technology, 2023. ↩
-
For an overview of DSIT’s latest research on public attitudes to data and AI, see: Public attitudes to data and AI: Tracker survey (Wave 3), Department for Science, Innovation, and Technology, 2023 ↩
-
The ATRS is the Algorithmic Transparency Recording Standard. For more detail see section 5.1. ↩
-
Frontier AI: capabilities and risks, Department for Science, Innovation, and Technology, 2023. ↩
-
The Bletchley Declaration by Countries Attending the AI Safety Summit, 1-2 November 2023, Department for Science, Innovation, and Technology; Foreign, Commonwealth and Development Office; Prime Minister’s Office, 10 Downing Street, 2023. ↩
-
International expertise to drive International AI Safety Report, Department for Science, Innovation and Technology, 2024. ↩
-
Emerging Processes for Frontier AI Safety, Department for Science, Innovation and Technology, 2023. ↩
-
Emerging Processes for Frontier AI Safety, Department for Science, Innovation and Technology, 2023. ↩
-
Office for Artificial Intelligence – information collection and analysis: privacy notice, Department for Science, Innovation and Technology, 2023. ↩
-
Office for Artificial Intelligence – information collection and analysis: privacy notice, Department for Science, Innovation and Technology, 2023. ↩
-
UK Artificial Intelligence Regulation Impact Assessment, Department for Science, Innovation and Technology, 2023. ↩