Portfolio of AI assurance techniques
This page provides details about DSIT's portfolio of AI assurance techniques and how to use it.
About the portfolio
The portfolio of AI assurance techniques has been developed by the Responsible Technology Adoption Unit, a directorate within DSIT, initially in collaboration with techUK. The portfolio is useful for anybody involved in designing, developing, deploying or procuring AI-enabled systems, and showcases examples of AI assurance techniques being used in the real-world to support the development of trustworthy AI.
Please note the inclusion of a case study in the portfolio does not represent a government endorsement of the technique or the organisation, rather we are aiming to demonstrate the range of possible options that currently exist.
To learn more about different tools and metrics for AI assurance please refer to OECD’s catalogue of tools and metrics for trustworthy AI, a one-stop-shop for tools and metrics designed to help AI actors develop fair and ethical AI.
We will be developing the portfolio over time, and publishing future iterations with new case studies. If you would like to submit case studies to the portfolio or would like further information please get in touch at ai-assurance@dsit.gov.uk.
Assurance
Building and maintaining trust is crucial to realising the benefits of AI. Organisations designing, developing, and deploying AI need to be able to check that these systems are trustworthy, and communicate this clearly to their customers, service users, or wider society.
AI assurance
AI assurance is about building confidence in AI systems by measuring, evaluating and communicating whether an AI system meets relevant criteria such as:
- regulation
- standards
- ethical guidelines
- organisational values
Assurance can also play an important role in identifying and managing the potential risks associated with AI. To assure AI systems effectively we need a range of assurance techniques for assessing different types of AI systems, across a wide variety of contexts, against a range of relevant criteria.
To learn more about AI assurance, please refer to the roadmap to an AI assurance ecosystem, AI assurance guide, industry temperature check, and co-developed RTA (formerly CDEI) and The Alan Turing Institute introduction to AI assurance e-learning module.
Portfolio of AI assurance techniques
The Portfolio of AI assurance techniques was developed by the Responsible Technology Adoption Unit (RTA), in collaboration with techUK, to showcase examples of AI assurance techniques being used in the real-world.
It includes a variety of case studies from across multiple sectors and a range of technical, procedural and educational approaches , illustrating how a combination of different techniques can be used to promote responsible AI. We have mapped these techniques to the principles set out in the UK government’s white paper on AI regulation, to illustrate the potential role of these techniques in supporting wider AI governance.
To learn more about different tools and metrics for AI assurance, please refer to OECD’s catalogue of tools and metrics for trustworthy AI.
Who the portfolio is for
The portfolio is a helpful resource for anyone involved in designing, developing, deploying or procuring AI-enabled systems.
It will help you understand the benefits of AI assurance for your organisation, if you’re someone who is:
- making decisions about your organisation’s use of AI
- involved in the procurement of AI for your company
Finding case studies of AI assurance
The portfolio allows you to explore a range of examples of AI assurance techniques applied across a variety of sectors. You can search for case studies based on multiple features you might be interested in, including the type of technique and the sector you work within. Each case study is also mapped against the most relevant cross-sector regulatory principles published in the government white paper on AI regulation.
Assurance techniques
There are a range of different assurance techniques that can be used to measure, evaluate, and communicate the trustworthiness of AI systems. Some of these are listed below:
-
Impact assessment: Used to anticipate the effect of a system on environmental, equality, human rights, data protection, or other outcomes.
-
Impact evaluation: Similar to impact assessments, but are conducted after a system has been implemented in a retrospective manner.
-
Bias audit: Assessing the inputs and outputs of algorithmic systems to determine if there is unfair bias in the input data, the outcome of a decision or classification made by the system.
-
Compliance audit: A review of a company’s adherence to internal policies and procedures, or external regulations or legal requirements. Specialised types of compliance audit include system and process audits and regulatory inspection.
-
Certification: A process where an independent body attests that a product, service, organisation or individual has been tested against, and met, objective standards of quality or performance.
-
Conformity assessment: Provides assurance that a product, service or system being supplied meets the expectations specified or claimed, prior to it entering the market. Conformity assessment includes activities such as testing, inspection and certification.
-
Performance testing: Used to assess the performance of a system with respect to predetermined quantitative requirements or benchmarks.
-
Formal verification: Establishes whether a system satisfies some requirements using the formal methods of mathematics.
Using assurance techniques across the AI lifecycle
Check with assurance techniques can be used across each stage of the AI lifecycle.
Technique type | |||||||||
---|---|---|---|---|---|---|---|---|---|
Stage | Impact assessment | Compliance audit | Certification | Bias audit | Conformity assessment | Impact evaluation | Formal verification | Performance testing | Other ongoing testing |
Scoping | Yes | Yes | Yes | ||||||
Data gathering and preparation | Yes | Yes | Yes | Yes | |||||
Modelling and design | Yes | Yes | Yes | Yes | Yes | Yes | Yes | ||
Development | Yes | Yes | Yes | Yes | Yes | Yes | Yes | Yes | |
Deployment | Yes | Yes | Yes | Yes | Yes | Yes | Yes | Yes | |
Live operation and monitoring | Yes | Yes | Yes | Yes | Yes | Yes | Yes | Yes | Yes |
Retirement | Yes | Yes | Yes |
Government background
The National AI Strategy sets out an ambitious plan for how the UK can lead the world as an AI research and innovation powerhouse. Effective AI regulation is key to realising this vision to unlock the economic and societal benefits of AI while also addressing the complex challenges it presents.
In its recent AI regulation white paper the UK government describes its pro-innovation, proportionate, and adaptable approach to AI regulation that supports responsible innovation across sectors. The white paper outlines five cross-cutting principles for AI regulation: safety, security and robustness; appropriate transparency and explainability; fairness; accountability and governance; and contestability and redress. Due to the unique challenges and opportunities raised by AI in particular contexts the UK will leverage the expertise of existing regulators, who are expected to interpret and implement the principles in their domain and outline what compliance with the principles looks like across different use cases. In addition, the white paper sets out the integral role of tools for trustworthy AI, such as assurance techniques and technical standards, to support the implementation of these regulatory principles in practice, boost international interoperability, and enable the development and deployment of responsible AI.
The RTA has conducted extensive research to investigate current uptake and adoption of tools for trustworthy AI, the findings of which are published in its industry temperature check. This report highlights industry appetite for more resources and repositories showcasing what assurance techniques exist, and how these can be applied in practice across different sectors.
Wider ecosystem
The UK government is already supporting the development and use of tools for trustworthy AI, through publishing a roadmap to an effective AI assurance ecosystem in the UK, having established the UK AI Standards Hub to champion the use of international standards, and now through the publication of the portfolio of AI assurance techniques.
UK AI Standards Hub
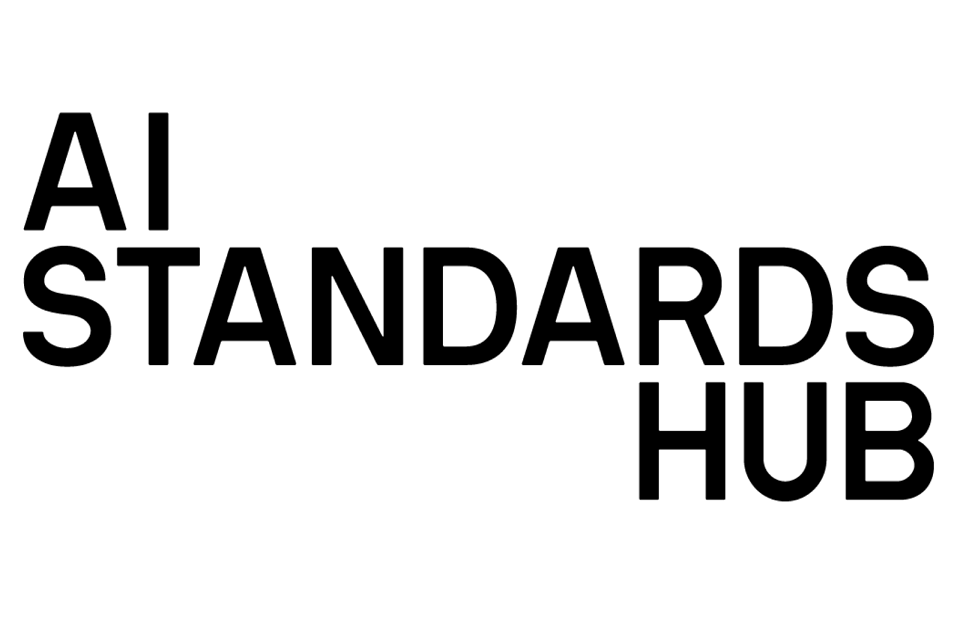
The AI Standards Hub is a joint initiative led by The Alan Turing Institute in partnership with the British Standards Institution (BSI), the National Physical Laboratory (NPL), and supported by government. The hub’s mission is to advance trustworthy and responsible AI with a focus on the role that standards can play as governance tools and innovation mechanisms. The AI Standards Hub aims to help stakeholders navigate and actively participate in international AI standardisation efforts and champion the use of international standards for AI. Dedicated to knowledge sharing, community and capacity building, and strategic research, the hub seeks to bring together industry, government, regulators, consumers, civil society and academia with a view to:
- shaping debates about AI standardisation and promoting the development of standards that are sound, coherent, and effective
- informing and strengthening AI governance practices domestically and internationally,
- increasing multi-stakeholder involvement in AI standards development
- facilitating the assessment and use of relevant published standards
To learn more, visit the AI Standards Hub website.
OECD AI catalogue of tools and metrics for trustworthy AI
The catalogue of tools and metrics for trustworthy AI is a one-stop-shop for tools and metrics designed to help AI actors develop and use AI systems that respect human rights and are fair, transparent, explainable, robust, secure and safe. The catalogue gives access to the latest tools and metrics in a user-friendly way but also to use cases that illustrate how those tools and metrics have been used in different contexts. Through the catalogue, AI practitioners from all over the world can share and compare tools and metrics and build upon each other’s efforts to implement trustworthy AI.
The OECD catalogue features relevant UK initiatives and works in close collaboration with the AI Standards Hub, showcasing relevant international standards for trustworthy AI. The OECD catalogue will also feature the case studies included in this portfolio.
To learn more, visit The OECD catalogue of tools and metrics for trustworthy AI.
Open Data Institute (ODI) data assurance programme
Data assurance is a set of processes that increase confidence that data will meet a specific need, and that organisations collecting, accessing, using and sharing data are doing so in trustworthy ways. Data assurance is vital for organisations to build trust, manage risks and maximise opportunities. But how can organisations assess, build and demonstrate trustworthiness with data? Through its data assurance work, the ODI is working with partners and collaborators to explore this important and rapidly developing area in managing global data infrastructure. ODI believe the adoption of data assurance practices, products and services will reassure organisations and individuals who want to share or reuse data, and support better data governance practices, fostering trust and sustainable behaviour change.
To learn more, visit the ODI website.