Experiments at the CMA: How and when the CMA uses field and online experiments
Published 19 April 2023
1. Executive summary
Consumer psychology, and how it influences consumer decision making, can be central to firms’ anticompetitive or unfair practices. Experiments are therefore increasingly being used by governments and regulatory agencies around the world to understand how business practices may influence consumer behaviour, and evaluate the effectiveness of remedies.
Experiments are primary research methodologies designed to demonstrate causality within their specific circumstances by assigning different interventions (or no intervention) to randomly selected, and therefore statistically similar, groups of participants, then measuring relevant outcomes. Experiments in public policy and regulation often produce evidence that would not otherwise be available, so conducting them can help to strengthen the evidence base for our work and ensure we are maximising our impact for the benefit of consumers.
This document sets out high-level principles as to when the CMA will use experiments, and best practice as to how they can be conducted. For the purposes of this document, experiments cover:
- online experiments, which are conducted with recruited participants in a simulated online environment; and
- field experiments (also known as field trials), which are conducted with consumers or firms who are making decisions in a real-world environment, either online or offline.
These methodologies are increasingly used alongside other evidence by regulators and policymakers around the world to measure the impact of interventions and learn what works. For instance, we provide 4 case studies of how the Financial Conduct Authority, Ofgem, the Danish Competition and Consumer Authority and the Dutch Authority for Consumers & Markets have successfully conducted experiments in Annex 1.
This document is intended to set out high-level principles to inform thinking as to the CMA’s potential new statutory powers to order trialling when exercising new market investigation and digital markets functions. The principles set out in this document will be revisited, assuming the CMA is given these new statutory powers. This document is also intended to inform the CMA’s approach to running experiments on a voluntary or unilateral basis under existing powers.
There are 2 types of research questions that the CMA frequently encounters in projects where experiments are most likely to be useful:
-
Developing and implementing remedies: Experiments can help to isolate and measure the effectiveness of remedies on key outcomes to inform decision making and implementation. For example, a field experiment might look to measure the effectiveness of disclosure on consumer behaviour to inform its potential use as a remedy for misleading sponsored content online.
-
Exploring and providing evidence for theories of harm: Experiments can generate evidence to help us understand potential sources of harm to consumers or competition, including how certain practices might exploit consumers’ behavioural biases. For example, an online experiment might look to measure consumers’ understanding and attitudes towards environmental claims made about products to inform the CMA’s future approach.
When deciding whether to run an experiment, we would first aim to answer 3 questions:
- Would an experiment be valuable? For example, is understanding the link between firms’ practices and consumer behaviour and biases central to the related investigation?
- Would an experiment be feasible? For example, would the evidence generated be sufficiently internally and externally valid for its purpose?
- Would an experiment be proportionate? For example, what would the experiment costs be to the CMA, businesses and consumers?
Answering these questions also helps us to decide which type of experiment to use. Specifically, the 2 factors likely to be most important in determining our approach are:
-
External validity: the extent to which the research replicates target populations and real-life settings, or to which findings can be generalised to other relevant populations or settings beyond. Generally, field experiments are considered to be more externally valid than online experiments, as they involve real consumers making real decisions in the course of normal life. However, well-designed online experiments can be sufficiently externally valid for certain types of research questions (for example, for understanding the role of underlying behavioural mechanisms in decision making) and where we can replicate real decisions consumers are making in online environments. (We discuss some of the factors that can affect external validity in Annex 2.)
-
Feasibility of sample recruitment: whether we can access and recruit a sample that is sufficiently representative of our population of interest. This is particularly relevant for field experiments, where we are dependent on working with firms to recruit a sample of real customers and measure real-world outcomes.
Rigorous experiment design depends heavily on the relevant project context (including the relevant research questions, consumers’ behaviours, and interventions), so each experiment is likely to be different. However, below we discuss some of the principles and best practice we consider in the design process, including: sampling and recruitment; interventions and randomisation; outcomes; analysis approach; cost; and timelines.
For each experiment we run, we would generally seek to detail all design elements and decisions in a Research Protocol document (which we seek to pre-register publicly where appropriate), and conduct a rigorous ethics review. We would also aim to publish all experiment results where appropriate to increase the public impact of results, as the evidence generated could be relevant and valuable information that other organisations could benefit from.
2. Introduction
The Competition and Markets Authority (CMA) works to promote competition for the benefit of consumers. We investigate markets where we believe there are competition or consumer problems, diagnose harm and take action against businesses that use anticompetitive or unfair practices.
Consumer psychology, and how it influences consumer decision making, can be central to firms’ anticompetitive or unfair practices. Behavioural science – the systematic study of human behaviour combining insights from economics, psychology and other social sciences – is increasingly being used by government, competition, and regulatory agencies around the world to understand how businesses might, intentionally or otherwise, exploit consumers’ biases and vulnerabilities for gain. The CMA created a Behavioural Hub in 2019 to provide advice and conduct primary research in support of cases, studies and other projects where behavioural biases play a central role.
This document sets out high-level principles as to when experiments will be used, and best practice as to how they can be conducted. By experiments, we refer in this document to primary research methods that use randomisation and control groups.
The intention of experiments as dealt with in this document is to measure the causal impact of a given practice or intervention on behaviour within the context of the experiment. For the purposes of this document, we consider:
- online experiments, which are conducted with recruited participants in a simulated online environment; and
- field experiments (also known as field trials), which are conducted with consumers or firms who are making decisions in a real-world environment, either online or offline.
For the purposes of this document, we primarily focus on experiments as they might apply to consumers, however, we note that experiments could feasibly be conducted with firms, for example, as the business-to-business customers of other firms.
This document is not intended to specify or determine how the CMA will conduct experiments in the future, but instead aims to put into place principles of good practice so we and others can benefit from these research methodologies. In line with good practice, we also expect to improve and develop these principles as we learn from the experience of running experiments.
Experiments are not always necessary or suitable for a given project. Where they are appropriate, they can support and complement the wide range of evidence from other sources the CMA already uses to inform its work. We will touch on these other evidence sources and research methods as we discuss how and when we might use experiments, but detailed guidance on sources and methods is outside the scope of this document.
The CMA would only use experiments where it is appropriate and helps us to effectively fulfil our functions. For instance, experiments may help us ensure that any interventions are well-designed and effective, or to ensure we properly understand the markets we are analysing. Section 3 provides more detail on the scenarios in which this is most likely to be the case.
We focus in this document on when and how the CMA may run experiments, however, we note that firms may also submit evidence gathered using similar methodologies to the CMA as part of a given case or investigation. This document is not intended to act as guidance for how businesses might conduct research. However, the weight given to such evidence would be assessed against a range of criteria in the context of the given case, including, but not limited to, the principles and best practices described in this document. Parties wishing to conduct and submit evidence resulting from experiments are strongly encouraged to contact the CMA in the early stages of the experiment process to discuss their proposed design.
For the purposes of this document, we do not cover ex-post quasi-experimental approaches, which aim to measure causality without randomisation or control over treatments by using statistical approaches.[footnote 1] However, we highlight the value and relevance of using these methods, particularly in contexts where there are constraints on the possibility, rigour or value of randomisation. We also do not cover experiments or research conducted in physical laboratories, but we observe that they share similarities with online experiments.
This document is intended to inform thinking as to the CMA’s potential future statutory powers to order trialling of remedies in the course of a market investigation, on which the government intends to legislate.[footnote 2] This document is also intended to inform the future approach of the CMA’s Digital Markets Unit (DMU) when conducting trials under the proposed pro-competition regime for digital markets, on which the government intends to introduce legislation.[footnote 3] The principles set out in this document will be revisited, assuming the CMA is given these new statutory powers. This document is also intended to inform the CMA’s approach to running experiments on a voluntary (for example, as part of a consumer enforcement case or market study) or unilateral basis (such as when running online experiments).
The document is structured in line with the steps we go through when conducting experiments: scoping (Section 3); experiment design for online experiments (Section 4a) and field experiments (Section 4b); experiment approval and implementation (Section 5); and dissemination (Section 6).
3. Scoping
3.1 Overview of experiments
Causal mechanisms can form an important part of the CMA’s rationale for regulatory action. Well-designed and executed experiments allow us to infer causality between a specific practice and outcome in a given context by creating a robust counterfactual through randomisation.
Experiments randomly assign people to receive different interventions or no intervention (known as a ‘control group’), then compare outcomes between groups. If there are enough participants, randomisation means we can infer that the groups receiving different interventions are generally similar in both observable (such as demographics and socioeconomic status) and unobservable characteristics (such as personality, preferences or other relevant individual traits that we cannot measure), as well as the external circumstances they experience. This approach means differences in outcomes between groups are statistically highly likely to be caused by the intervention, as opposed to other external factors or differences in characteristics between the groups. These comparisons, in combination with relevant theoretical models and insights from other methodologies, can play an important role in understanding what works and why.[footnote 4] This methodology is also often called a randomised controlled trial.
For the avoidance of any doubt, for the purposes of this document we use the term ‘causality’ to refer specifically to the statistical comparisons within the specific contexts of experiments as described above, and not necessarily to any broader conceptions of causality as they might apply to CMA cases. We also note that there is also a range of other types of evidence that can help to establish causality in a given context, depending on the circumstances at hand. Establishing causality requires a case-by-case evaluation of the context and the potential evidence sources. Figure 1 below depicts a simple experiment.
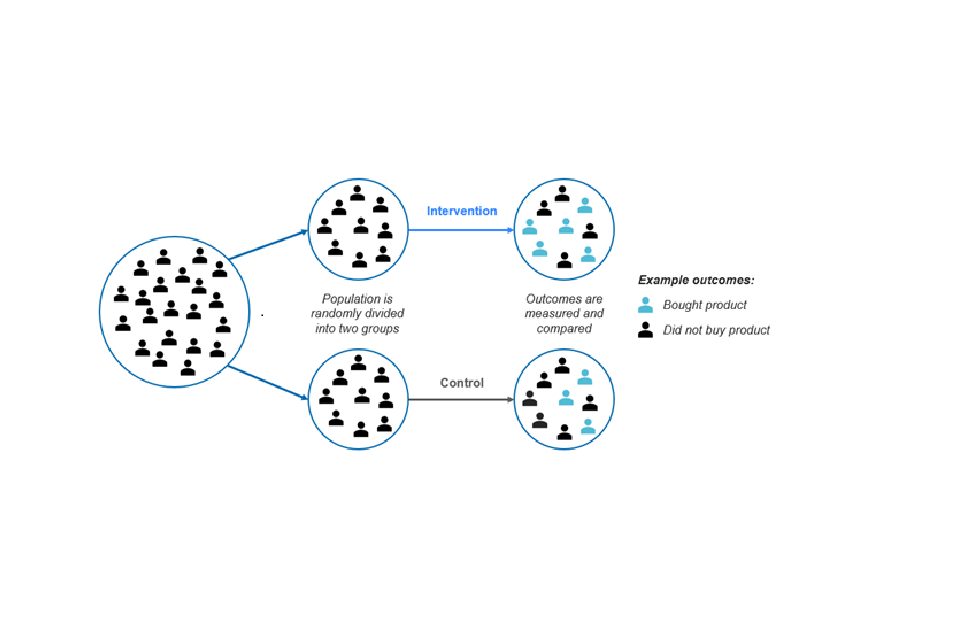
Figure 1: A simple experiment
Both online experiments and field experiments use randomisation to create robust counterfactuals and estimate causal relationships between specific practices and outcomes, however, they differ in several aspects.
- Online experiments involve recruiting people to participate in an online task, where they are randomly assigned to be exposed to interventions, and their decisions, recall or comprehension are measured. Participants’ decisions in online experiments are usually hypothetical, but can also be designed to affect their reward for participating in the experiment. Usefully, online experiments also allow the possibility to ask follow-up questions to participants, for example about their attitudes or how they went about the task.
- Field experiments involve randomly assigning people to a control group, which gets business as usual, and one or more treatment groups, who are exposed to an intervention under investigation. People’s subsequent real-world behaviour and actions are then compared between the groups. We provide more detail on what each of these methodologies involve in Section 4 below.
The box below provides a summary of how experiments have been used by regulators and across public policy, and we provide 4 more detailed case studies in Annex 1.
3.2 Using experiments in regulation and public policy
Experiments are increasingly used by regulators and governments more widely to understand the impact of remedies, interventions and policies. The Financial Conduct Authority (FCA) has run field experiments and online experiments in areas including pensions, mortgages and current accounts, and has published documents detailing how it conducts both field experiments and online experiments based on this experience, on which this document draws substantially.[footnote 5] Ofgem ran 5 field experiments in 2019 testing whether sending disengaged energy customers letters can increase switching to beneficial tariffs,[footnote 6]and the Office of Communications (Ofcom) recently ran a consultation to run field experiments of customer engagement remedies.[footnote 7] The Danish Competition and Consumer Authority (KFST) and the Dutch Authority for Consumers & Markets (ACM) have both run several online experiments.[footnote 8] More widely across public policy, the Behavioural Insights Team[footnote 9] has run over 400 field experiments and online experiments with government departments, regulators, other public bodies and non-governmental organisations over the last 10 years. Similar behavioural policy units have been set up in government departments internationally to run experiments, including in Australia, the Netherlands and Germany.[footnote 10] Companies also increasingly use experimental methodologies to understand consumer behaviour and improve commercial outcomes: for example, many digital companies now conduct extensive A/B testing - randomised experiments run on websites testing the effect of small changes in the user experience - to optimise user experiences and website design.[footnote 11] (See Annex 1 for relevant case studies of online experiments and field experiments used by regulators.)
3.3 Deciding whether to run an experiment
To decide whether an experiment would be appropriate for a given project, we first identify relevant research questions. These can broadly be grouped into 2 categories:
Developing and implementing remedies
Where deemed appropriate, the CMA can implement demand- and supply-side remedies in markets.[footnote 12] Monitoring outcomes after implementing a remedy can be helpful in indicating whether a remedy is having the desired effect. However, it can also be difficult to know if any changes in outcomes are caused by the remedy introduced, or by other factors.
Remedies might also not be effective across all outcomes of interest: for example, the FCA found in a field experiment that removing the option to pay the minimum repayment each month (instead allowing customers to pay in full or choose an amount) did not reduce debt more quickly, despite increasing monthly payments among those in the treatment group.[footnote 13]
Experiments can help to measure the effectiveness of remedies on specific outcomes, independent of other factors. This evidence can help us to determine which remedies should be implemented (before a remedy is rolled out fully, which can take a long time); how long to keep them in place; whether further remedies should be considered; and how we can design more effective remedies in the future. Examples of remedies experiments include testing whether disclosures help people to identify sponsored social media posts; whether text message alerts can help consumers to avoid using their overdrafts; and whether prompt letters can encourage consumers to switch to cheaper energy plans (see Annex 1 for more detail). We expect that most experiments the CMA will run will fall under this category.
Example research questions:
Does this remedy design improve the outcome it is meant to improve?
Which remedy design is most effective?
Are there any unintended consequences of the remedy?
Exploring and providing evidence for theories of harm.
The CMA often seeks to understand the specific ways in which competition and/or consumers may be harmed in a market before we decide to take further action. In such circumstances, experiments can provide evidence to help establish whether firms’ behaviour adversely affects consumer decision making. Furthermore, the rapid rise and dynamic nature of digital markets also presents a challenge to the CMA to keep up with new potential sources of consumer or competition harm. There may be less existing evidence on new potential sources of harm, which can make it difficult to understand the problem and know whether and how to intervene. Experiments can help us to understand the drivers of consumer behaviour in new areas, and how firms’ practices might interplay with behavioural biases to affect market outcomes.
Example research questions:
Are consumers’ decisions in a given market impacted by behavioural biases?
How might practices exploit behavioural biases to the detriment of consumers?
Does a given practice have a significant impact on consumers’ purchase decisions, search behaviour, or willingness-to-pay?
How does a given practice affect consumer understanding and recall of information?
Experiments can, and often do, cover both of these types of research question. For example, where an experiment is testing whether a remedy reduces the effect of a potentially harmful business practice, we might use the descriptive statistics from the business-as-usual control group to explore how harmful the practice currently is. Similarly, if we want to explore a new theory of harm, it could be efficient and valuable to explore early ideas for potential remedies within the same experiment.
Experiments are intended to be complementary to other types of evidence that the CMA relies on. The CMA already uses a wide range of evidence, analysis and research that help us to answer research questions that experiments are not suited to answer.[footnote 14] We can also benefit from using these other evidence sources to inform how experiments are designed through a nuanced understanding of the problem and surrounding context, or to answer the same research question more robustly.
Experiments take time, effort and expertise to run effectively. We would generally consider 3 overarching questions when deciding whether to run an experiment, and if so, which methodology to use: 1. whether an experiment would provide valuable evidence; 2. whether we can feasibly run an experiment; and 3. whether running an experiment is proportionate to the costs to the CMA, businesses and consumers involved (including time).
Below we consider each question in turn, with reference to specific considerations which may inform our answers for each.
Would an experiment provide valuable evidence?
Is understanding the link between firms’ practices and consumer behaviour and biases central to the related investigation? Projects or cases where we want to understand how specific firm practices influence consumer behaviour and biases are likely to be most amenable to experiments. For example, where the CMA is considering a theory of harm where most consumers do not understand a company’s terms or product features, an experiment could be valuable in investigating the level of understanding consumers have, and the role this plays in their subsequent behaviour.
Would the evidence from an experiment appreciably reduce uncertainty? We want to run experiments when we have a hypothesis for whether and how a practice affects consumer behaviour, but there is some uncertainty about its impact. This means the evidence generated, regardless of whether it is significant (ie we find evidence that the intervention impacts outcomes) or a null result (ie we do not find sufficient evidence to conclude that the intervention impacts outcomes), can provide valuable information and learning for a project. If we are already confident about the effectiveness of a remedy, for example, where there is strong existing evidence of effectiveness and it has a clear theory of change, an experiment might be less valuable than for a remedy where there may be limited evidence or we are less confident of the effect it may have on outcomes.
Is there limited existing evidence that is sufficiently rigorous and relevant? Experiments are likely to be more valuable in areas where there is limited existing evidence, the evidence is insufficiently rigorous, or the evidence is not sufficiently relevant to the case or specific research question at hand. Existing evidence ideally includes experiments run by regulators in other sectors or countries, academic research, other evidence generated within the project, or evidence generated in previous CMA projects. Whether existing evidence is sufficiently relevant will depend on the nature of the specific topic at hand. For example, if there is existing evidence in a tangential area to a project but its relevance is questionable, there might be value in conducting an experiment more specifically in the area of focus.
Would other research methods be feasible and provide sufficiently rigorous evidence? The value of experiments should be considered alongside, and relative to, other potential research methods. For example, a natural experiment using historical real-world data could generate valuable evidence to assist in establishing causality without the need for an experiment, as it necessarily involves the target consumer population and outcomes, and can create a rigorous counterfactual if certain conditions are met (for example, using propensity score matching, regression discontinuity, difference-in-differences etc).
Can the CMA reasonably take action on the basis of evidence generated? We aim to use experiments as a lever to improve our effectiveness, and therefore to maximise the likelihood of positive outcomes from our projects. Experiments are more likely to be valuable when the results can feasibly inform and influence decision making, depending on the extent of CMA powers, the context of a given project and the related timelines.
Would an experiment be feasible?
Will the evidence generated be sufficiently internally and externally valid for its purpose? Internal validity refers to the extent the results from an experiment support its stated claim about causality within the experiment sample and context. External validity refers to the extent results can be generalised beyond the immediate experiment context: either i) to wider populations (population validity), or ii) in wider settings (ecological validity). Ensuring experiments are sufficiently internally and externally valid is vital to be able to interpret and make use of the evidence generated – it means we can be confident the findings are relevant for their purpose. We provide a more detailed discussion of internal and external validity, and their relevance to the feasibility of running experiments, in Annex 2.
Can we recruit a sufficiently large sample to identify impact? An experiment can often require a large sample of participants (individuals or organisations) to feasibly gather statistically significant results. Experiments are therefore more likely to be feasible where the sample consists of large populations of individual consumers or firms. Where we wish to recruit firms’ existing, new or prospective customers for our sample, this usually requires the ability to work with firms.
Is there sufficient time for the evidence to inform key decisions? Experiments may require several months to set up, implement and monitor outcomes. As with any research strategy, part of running field experiments is that there may be implementation errors, changing circumstances and unexpected outcomes, and we should try to build in flexibility in our planning, processes and timelines to account for these. When planning experiments, care should be taken to ensure there is sufficient time to obtain results for important decision-making milestones. This is particularly important for projects with statutory time limits, such as market studies, market investigation references and merger reviews.[footnote 15]
These questions around feasibility, particularly external validity and sample recruitment, are generally the key factors in determining whether we choose to run an online experiment and/or a field experiment, as the answers are most likely to be different between the methodologies. Field experiments are generally considered to have higher external validity than online experiments, however, online experiments can provide valuable insights into certain types of research questions, and the external validity of any given experiment depends heavily on the specific population and setting at hand. We provide a more detailed discussion of external validity in the context of each methodology in Annex 2. We are also generally only able to conduct field experiments where we can feasibly work with firms, as they involve recruiting customers, delivering interventions and measuring outcomes through firms, whereas we can run certain types of online experiments without working with firms.
Would an experiment be proportionate?
What are the direct experiment costs? Conducting experiments requires a significant investment of time and organisational resources – both by the CMA and eventual partners. The balance of costs will depend on the specific nature of the research. For example, complex field experiments (for example, those involving substantial changes to existing systems or services) can require significant time and resource to design, engage stakeholders and execute effectively in practice. However, if a field experiment involves existing customers, user journeys and data collection mechanisms, the experiment might be relatively inexpensive to run operationally. These costs (both to the CMA and to any partners who would be needed to implement the experiment) should be considered alongside the potential value of the evidence.
Will an experiment not add too much time and delay to the process? Experiments can often take 6, 12 or even 18 months to complete, depending on the complexity and design. If there would be a delay to an investigative process as a result, this would be highly relevant to the decision whether to start an experiment. There is also a risk that experiments may ultimately be inconclusive as to what is the best remedy (for example, if none of the remedies trialled appear to be wholly effective), which we would also weigh against delaying the project.
Is the project strategically important, or likely to inform future projects, for the CMA or other stakeholders? We might place more weight on the value of an experiment over the costs if the findings are likely to be particularly important to CMA strategic priorities, or if there are likely to be a number of related projects in the future that would benefit from the findings.
4. Experiment design
Below we detail some of the practices and principles the CMA may consider when designing and conducting experiments. Our approach to designing experiments will depend heavily on the specific context of each situation, and it is likely to adapt and develop as each experiment progresses and as we run more experiments over time.
4.1 Experiment design: online experiments
Sampling and recruitment
Sample sources: Some of the sources we will seek to recruit our experiment participants through include:
Lists of existing, new or prospective customers from relevant firms:
- Where firms’ customers are the population of interest, recruiting directly from firms’ customer lists is generally likely to result in higher external validity. Samples recruited from customer lists would ideally be directly relevant to the research question, cover the full range of types of customers, and be randomly selected. Using firm customer lists may require information gathering powers as part of an investigation.
Online panel providers:
-
We can also recruit participants through online panel providers, who recruit people willing to take part in incentivised surveys and experiments (usually for market research purposes). Some providers use probability sampling approaches, however, most use non-probability, or ‘convenience’, sampling (for example, advertising for panellists).[footnote 16] These convenience panels allow us to easily recruit a sample that is representative of the consumer population, or large subpopulations, on observable characteristics including location and certain demographics.
-
There is relatively little evidence on the quality of UK online convenience panels, however, the available evidence suggests that surveys of probability samples are significantly more accurate when making population-level estimates compared to nonprobability samples.[footnote 17] As highlighted in our publication detailing good practice for customer surveys,[footnote 18] this may be because members of some online convenience panels might not be fully representative of other consumers on unobserved characteristics: for example, participants may have more experience online than a truly nationally representative sample.[footnote 19]
-
From our experience, there is also considerable variation in the quality of panel providers, particularly with respect to the transparency and rigour of their recruitment methods. Nonetheless, probability samples can be prohibitively expensive to recruit, and nonprobability samples can be fit-for-purpose when answering certain types of research questions.[footnote 20] For example, there is some evidence to suggest convenience samples are more appropriate for estimating experimental treatment effects than for estimating population means.[footnote 21]
-
We will judge whether using a given type of online panel is appropriate in the specific context of the research based on the extent to which we believe sampling biases are likely to affect the accuracy or relevance of the results in the specific context of the research. This involves assessing the information provided by the online panel providers with respect to the internal and external validity considerations set out above and in Annex 2, and the quality of their recruitment methods. For example, evidence from an online convenience panel might provide valuable insights about wider populations if we are looking to identify the presence of a behavioural bias in online consumer decision making relative to a normative standard. It may be less suited if we are looking to prove harm or provide population estimates on the harm caused by an individual firm or understand decision making by specific groups underrepresented in online panels (such as the elderly or non-Western immigrants).[footnote 22]
Screening: We can check that participants do not participate in the experiment multiple times, including by screening for IP address in a manner compliant with data protection regulations and removing those who complete experiments more than once. Many online panels also have systems such as unique identifiers to ensure panel members only complete tasks once. Working with our recruitment partner, we can use quotas to ensure that the resulting sample is representative on key characteristics: for example, stopping recruitment of participants aged 18 to 30 once we have reached the required subsample to meet a representative distribution.
Sample size: We can conduct power calculations to decide the appropriate sample size for an online experiment. We can use power calculations to estimate the minimum number of participants needed to detect a given effect size in an experiment, or the minimum effect size we could detect given a certain number of participants. These calculations assume certain experiment design features such as statistical power, significance levels, and outcome measure baselines. In line with academic common practice, we would generally aim to use a power of 80%[footnote 23] for the main treatment effect (usually between control and the main treatment arms) and conduct significance tests at the 5% confidence level.[footnote 24] The minimum detectable effect size depends heavily on the specific outcome used, and unless we conduct a pilot, the baseline or variance for that outcome is usually difficult to estimate before conducting the experiment. We would therefore also aim to conduct power calculations for a range of possible baselines, or after conducting a pilot, to check robustness.
Incentives: We may use financial incentives as part of online experiments to increase attention and therefore lower variation in how participants respond to questions. Participants will generally receive a flat participation fee, then in some experiments could receive further variable rewards designed to incentivise accurate, unbiased decision making. For example, participants could be rewarded for correct answers with respect to recall, understanding of certain information or optimum decision making within the brief. To ensure value for money of our research and as is common within experimental studies, we may use lotteries, in which some subjects are randomly selected to receive extra performance-based incentives or certain randomly selected actions within the experiment are used to calculate the final incentive payment. Where feasible and appropriate, we may also explore using non-financial incentives (for example, giving participants the product they selected in the task). The criteria for applying incentives will be made clear to participants in advance, though the exact amount to be received may not be known until after the experiment has been completed.
Interventions and randomisation
Intervention designs, experiment instructions and number of interventions: The interventions and remedies we test in experiments may include hypothetical scenarios and mock-ups of existing web pages or choices. To maximise external validity, we would seek to reflect how interventions are, or will be, seen in reality by consumers as much as possible. Where we are testing a new remedy, we would ideally refine and test designs based on behavioural evidence and research to maximise effectiveness. Where experimental interventions diverge from how interventions appear in reality, they would ideally focus on the key elements involved for the research question. For example, if parts of a potential remedy cannot be fully replicated in an online experiment (such as if in practice it would be personalised to individual users’ previous interactions with a service), but a key component of the remedy involves a salient disclosure, the experimental intervention could focus on this component. We would seek to consider any implications of intervention design choices when planning the experiment, and account for them when interpreting results. Where needed to facilitate understanding of the interventions, we would provide clear and concise experiment instructions to participants. We would generally aim to limit the number of interventions tested to increase the statistical power possible from a given sample size. Where relevant, we would also account for multiple hypothesis testing in our sample size calculations and results analysis.
Randomisation strategy: The randomisation strategy would depend on the specific nature of each experiment. We could either use within-subject or between-subject designs depending on the nature of the experiment: in between-subject designs, participants are only exposed to one intervention condition, whereas in within-subject designs, participants are exposed to multiple intervention conditions. Sometimes we may use stratification to control for potential sources of variation in the sample, or to ensure we have a sufficient sample of sub-populations of particular interest by oversampling them. Stratification involves dividing the sample up into subpopulations before they are randomly allocated to intervention groups. Members of each subpopulation are then randomly allocated to treatment groups.
Where we add elements beyond the intervention to the experiment, such as to make the experience more realistic, we would aim to ensure these are consistent or randomised between the control and intervention groups. Similarly, we would aim to randomise the order of different interventions or answer options to minimise ordering or priming effects. In certain circumstances, the methodology may hold similarities to conjoint analysis, where product attributes are varied independently to understand how customers might value them differently.[footnote 25]
Outcomes
Primary and secondary outcome measures: Primary and secondary outcome measures would generally be directly related to the research questions being tested. They could include observable actions or behaviours within the experiment, such as real or hypothetical selections of products or firms to buy from. There is mixed evidence on how well hypothetical choices in survey experiments can replicate actual behaviour,[footnote 26] but this is likely to be dependent on the context and the types of choices presented in a given case. Where questions are hypothetical, we would generally aim to replicate the mindset and incentives of real decision makers as closely as possible in the environment.
Primary and secondary outcomes can also include accuracy of a participant’s recall or comprehension of information. For example, to check whether participants understand a key point of information, we might ask a multiple-choice question that includes a clear, but not obvious, right answer, and wrong answers that represent common misconceptions or indicators of harm.
Exploratory outcome measures: Exploratory outcome measures (ie those related, but not central, to the main research questions) would usually include questions asking participants to report beliefs (what the participants thinks about an intervention or other prompt) or attitudes (how the participants feel towards an intervention or other prompt). These measures can help us to understand and explore why there might be differences in outcomes between interventions or spot whether interventions may affect other related outcomes.[footnote 27]
Question types: Questions would ideally be specific and neutrally framed. Where we choose to use free text responses, we can use a combination of manual qualitative coding and data science techniques to analyse themes in responses. Questions and instructions should be as concise and clear as possible while providing all information necessary to understand the tasks. Following the randomised section of an experiment, we can incorporate general survey questions to build understanding of the issue, as well as to check compliance with and understanding of the experiment instructions.
Attrition: Attrition (where participants drop out before completing an experiment) can cause problems for experimental analysis, both across an experiment and where it differs between treatment groups. Although there is some evidence that attrition in online experiments can be random (Arechar et al., 2018), differential attrition often arises.[footnote 28] We will try to consider and limit any potential causes of differential attrition across control and treatment groups in the experiment design, as it can be difficult to address during analysis. We can reduce the likelihood of general attrition by minimising the time it takes to complete the experiment and by offering appropriate incentives. We can reduce the likelihood of differential attrition by trying to make the duration and incentives similar between treatment arms. However, where we find differential dropout between intervention groups, we can take steps to account for this statistically in the analysis.
Analysis approach
When possible, we would aim to define our analysis approach in advance of conducting the experiments in our Research Protocol document (see Section 5 below).
We would generally conduct balance checks to ensure participants (across the sample, and potentially within treatment groups) are representative of our target population on observable characteristics as much as is feasible. We can also check representativeness on non-demographic characteristics, such as psychometric scales, vulnerabilities, cognitive ability or previous experience in a given context, where we can get this data on our target population. Where a sample is still not sufficiently representative on these characteristics, in certain cases we can also use weighting techniques to rebalance the dataset and account for this imbalance in our analysis.[footnote 29] We can exclude participants who did not complete the task to a satisfactory standard. We might exclude participants who completed the task excessively quickly, who appeared to click through the answers randomly, or who failed instructional manipulation checks, where participants have to select a specific stated combination of answers to proceed.[footnote 30] We would then aim to account for the implications of these exclusions in the interpretation of results.
We would seek to use current best practice statistical techniques for designing and analysing experiments. For most experiments where we have successfully randomised participants, the analysis approach is generally relatively standardised, using simple regressions to statistically model the relationship between the intervention, outcome and characteristics of participants.
Analysis would generally include comparisons between intervention and control groups on primary and secondary outcomes, plus any pre-specified subgroup analysis, for example for specific demographic groups (usually age, gender, ethnicity or education status) or answers to other questions asked during the experiment (for example, psychometric scales, vulnerabilities, numeracy, attention). Analysis will be planned and recorded in advance to prevent post-experiment ‘p-hacking’ (ie adjusting the analysis approach to increase the likelihood of finding a statistically significant result).
4.2 Experiment design: field experiments
Most elements of field experiment design and implementation would depend on the specific experiment and interventions at hand. We detail some guiding principles for these elements below. These principles are in line with the FCA guidance on field trials.[footnote 31]
We also note that there have been a number of developments in more complex and sophisticated causal inference experimental methodologies, such as those utilising machine learning or dynamic randomisation. The design principles in this document focus on simple experiments, however, the underlying principles of causal inference methods will still be relevant in more complex methodologies.
Sampling and recruitment
If we decide that a field experiment would be appropriate, we would generally seek to work with firms to recruit a sample of their existing, new or prospective customers. If we want to understand market-wide effects, we would aim to work with firms that allow us to recruit a representative sample of consumers across the market.
We would aim to avoid customers being able to self-select into experiments where possible, as the customers who opt in might behave differently to those who do not (known as ‘selection bias’). If self-selection is unavoidable and the participation rate is sufficiently high, we would tend to analyse the results on an ‘intention-to-treat’ basis (ie measuring and comparing outcomes for everyone who was offered to participate, rather than only those who opted in).
Depending on the research questions, we would similarly be wary of partnering with a small subset of firms on a voluntary basis, as willing firms might not be representative of the wider industry in important ways (for example, in how they interact with customers, or how their customers behave). If this was our only option, we would investigate whether there are significant observable differences between the firms we work with and other firms in relevant areas, and ensure our findings were sufficiently robust to the potential selection bias. We would also address any potential conflicts of interest working with firms as part of our ethics review process (see Section 5 below for further discussion). 4. Where possible without compromising the robustness of findings, we would aim to balance having a sufficient sample size with the operational impact on firms we are working with and any ethical considerations about participation.
Interventions and randomisation
Interventions would generally be designed by the CMA in line with the relevant project or case. We would ensure the intervention design could be feasibly implemented and optimised for real customers by consulting with the relevant partnering firm and other relevant stakeholders if needed. The extent of engagement with various third parties will depend on the specific case. Interventions would ideally be as close as possible to what is currently seen by consumers, or how consumers would see them if the intervention is applied more widely.
Treatment groups to which people are randomised would generally involve experiencing one intervention, however, they could also contain combinations or sequences of interventions.
There are several different types of field experiments we could use that vary in how treatment groups are assigned to participants:
- Individual-level: Most randomised controlled trials involve randomly allocating individual customers to different treatment groups. This approach maximises statistical power for a given number of participants in the trial.
- Cluster-level: Cluster randomised controlled trials randomise pre-existing groups of individuals (or ‘clusters’) to different treatment groups, such as by location or day of the week, while still measuring outcomes at the individual level. Where there is within-cluster correlation of characteristics or outcomes, this method can have less statistical power than individual-level randomisation. However, the approach can be preferable in certain situations, such as where there are likely to be spillovers between participants (ie participants who are in the control condition come into contact with participants in the treatment group and may therefore be influenced by the intervention). We would aim to explore whether a clustered approach could be feasible when conducting power calculations during the experimental design stage.
- Quasi-experimental (non-randomised) designs: Quasi-experimental designs involve applying statistical techniques to natural or non-randomised variation in interventions to create a counterfactual and estimate impact. They are often used to estimate the impact of an intervention retrospectively, for example, by taking advantage of differences in laws, exogenous events, arbitrary eligibility criteria or geographical variation in uptake that are independent of variables that potentially affect outcomes. The CMA already uses some of these analytical techniques in its work. However, they can also be designed in when random treatment allocation might not be possible. For example, where the geographical rollout of an intervention is already set, we might be able to delay its rollout to certain areas and use quasi-experimental techniques to measure the impact.
Accurate treatment allocation and monitoring is crucial to the success of field experiments. In some cases, firms may themselves conduct the randomisation on their systems with CMA oversight. In other cases, the CMA may conduct the randomisation, such as through firms sharing non-personal customer details with us, such as lists of anonymised customer ID numbers. We would then monitor randomisation to ensure the procedure has been implemented as planned (for example, checking that there has been no contamination between intervention groups).
We would generally aim for participants to be unaware they are participating in a study, or which intervention group they are in, as this awareness can threaten the validity of the experiment. However, there may be situations where consumers can or should be aware they are participating in an experiment (for example, if we require consent for people to participate for ethical reasons). This decision would be discussed and agreed during the planning stage and the ethics review process (see Section 5 below).
Outcomes
Primary and secondary outcomes would generally involve observable consumer behaviour, such as purchase decisions or specific browsing behaviour. Ideally these outcomes would use data that is routinely collected by firms, however, we may also seek to create new outcome measures in consultation with firms (for example, running a survey to measure consumer understanding).
Analysis approach
Principles for how we would approach analysing field experiments would generally be similar to our approach for online experiments (see above), including that we would seek to pre-specify our analysis approach in a Research Protocol.
After (and where possible during) a field experiment, we would conduct balance checks on the representativeness of the overall sample with respect to the wider population, and of treatment groups to the control group. These balance checks would use observable consumer characteristics or behaviour where data is available and appropriate to be used. Where we find imbalances, there may be opportunities to adjust the recruitment strategy during implementation, or to account for the imbalances in the analysis approach.
Where field experiments require more complex statistical techniques, we would decide which techniques to use after discussion with internal and external experts as needed. We would seek to think through statistical techniques in advance of the experiment and include them in our Research Protocol.
5. Experiment approval and implementation
5.1 Research Protocol
For both online experiments and field experiments, we would generally seek to draft a Research Protocol that details all elements of experiment design, including any changes and adaptations that are made during implementation. This document would aim to support and facilitate accurate implementation, quality assurance and the potential for replication. We would generally seek input and quality assurance from relevant experts within and outside of the CMA. We note that whether and how we draft the Research Protocol would need to be flexible in line with practical considerations during the experiment, including changes and challenges to timelines and implementation.
When appropriate, we would aim to pre-register a summary of our Research Protocols for experiments (including the interventions, experiment design, outcome measures, and regression specifications) with a public repository like the Open Science Framework.[footnote 32] By documenting the research plan publicly, the practice of pre-registration would enhance the credibility of the research by seeking to minimise the risk that the researchers change their methodology for the specific experiment in response to the data collected. We note that this plan would still need to be flexible where we identify the need to make minor design changes after having published the protocols or started the experiment. Where we are unable to pre-register publicly (for example, for confidentiality reasons in line with the CMA’s guidance on transparency and disclosure[footnote 33], we would generally aim to follow the same procedure and log a finalised document internally in the CMA.
5.2 Ethics review
Before commencing an experiment, we would generally seek to conduct an ethics review. Some of the key criteria we would consider when assessing the extent of ethical risk include:
- Vulnerable individuals: Could a significant proportion of the population in the research be classified as ‘vulnerable’ (for example, older people, people taking out short-term loans)?
- Use of sensitive data: Does the research use sensitive personal data (for example, individuals’ data on spending and saving), special category data, or data that could be used to identify individuals?
- Psychological manipulation: Does the research involve psychological manipulation (for example, deception, emotional manipulation) in order to observe real behaviour or elicit more accurate results?
- Secondary use of data: Do we intend to reuse the data collected in the experiment for purposes other than the primary research?
The ethics review would also consider the awareness and consent of experiment participants, and any relevant conflicts of interest for organisations involved.
Where an experiment faces particularly high ethical risks or there are reasons we might not be able to adequately assess the ethical risks internally, we may consult with external experts.
5.3 Legal compliance, data protection and confidentiality
All of our experiments would comply with the law. We would comply with data protection legislation throughout the process of running experiments. This could include: signing data sharing agreements with relevant partners summarising the data collection, storage, processing and sharing activities between parties; handling data in secure environments; minimising the amount of personal data processed (for example, by requesting that firms share anonymised or pseudonymised customer data for a field experiment as appropriate for the purposes of analysis); and where necessary, deleting individual-level data after an appropriate amount of time following experiment completion. We would expect the data processing in most experiments to require a Data Protection Impact Assessment, which would be signed off by our Data Protection Office.
We would also ensure that all activities carried out during experiments comply with our confidentiality obligations including under Part 9 of the Enterprise Act 2002.
5.4 Experiment implementation (field experiments only)
For field experiments, we would generally seek to detail the activities that need to happen before, during and after the experiment to ensure its successful delivery, either as part of the Research Protocol or in a separate document. This could include: specifying data collection, storage, processing and sharing activities; intervention design and development; any manual implementation requirements (such as setting up randomisation processes); and monitoring.
We would then seek to discuss and agree with each firm the relevant aspects of the experiment that they would be responsible for delivering. We note that these activities are likely to develop over the course of designing and implementing an experiment, so we would need to maintain flexibility over how changes are documented, agreed with firms and implemented.
6. Dissemination
6.1 Analysis Report
For both online experiments and field experiments, we would generally aim to set out our results in an Analysis Report, in line with the pre-specified approach set out in the Research Protocol. The Analysis Report would also include an interpretation of the results in the context of the specific project and how the experiment was implemented in practice, including in relation to the experiment design principle set out above. As for the Research Protocol, whether and how we draft the Analysis Report would need to be flexible in line with the nature and purpose of the experiment at hand.
6.2 Feedback to firms (field experiments only)
Where we have partnered with firms to deliver a field experiment, we would aim to discuss our findings with each firm to help them to understand the implications of results and to help us to confirm our understanding of the outcomes. We would aim to ensure this feedback does not unfairly provide a commercial advantage to these firms.
6.3 Publication
Publishing the results of our experiments, regardless of their statistical significance, increases their impact by contributing to the growing international body of consumer and competition research while avoiding publication bias.[footnote 34] Where appropriate, we would aim to publish experiment results, in line with the Government Social Research guidance,[footnote 35] the Concordat to Support Research Integrity,[footnote 36] and having regard to the CMA’s guidance on transparency and disclosure.[footnote 37] Where experiments are run as part of a case, the results would generally be published in line with the CMA’s transparency requirements for that case. This could also include in reports published on GOV.UK, and where appropriate and supported by academics, in academic journals.
To increase the visibility and public impact of the results, where appropriate, we would also aim to actively disseminate and discuss the results with stakeholders across the CMA, government and international agencies. This could take the form of direct or conference presentations, executive summaries, blogs or other products and activities.
Where experiments are used as evidence for the purpose of exercising the CMA’s statutory functions, there are frameworks which require disclosure to parties so they can understand the basis of the CMA’s decision or can defend a claim. These frameworks may require the disclosure of outputs to parties (for example, the research dataset, aggregated findings and research materials). In the event that a CMA decision is subject to appeal, or litigation commences, additional information may need to be disclosed to enable further scrutiny by the courts.
Where elements of an experiment are confidential or commercially sensitive (for example, firm involvement, firm-specific baseline data or other competitive information), we will handle this information in accordance with the CMA’s statutory obligations to protect confidential information. For further information, please see the CMA’s guidance on transparency and disclosure.[footnote 38]
6.4 Continuous learning
We aim to identify learnings from throughout the experiment process to feed back into future experiments. For example, we may update our internal repository of best practices for experiment design and statistical analysis based on quality assurance from academics, or refine our ways of working with firms to ensure successful outcomes.
7. Annex 1: Case studies
Below we present 4 case studies of experiments conducted by regulators to illustrate the types of research questions and results experiments can help to give. These case studies all test the effectiveness of remedies, but also shed light on theories of harm related to the topic areas.
7.1 Case study 1: Dutch Autoriteit Consument & Markt (ACM) online experiments into price transparency, paid ranking and product information
In 2020, the ACM published Guidelines on the Protection of the Online Consumer highlighting the standards they use for overseeing online deception. The guidelines to businesses include ensuring information is correct, complete and easy to understand, that default settings are favourable to consumers, and to test the effects of online choice architecture. The ACM, in partnership with universities and a research agency, then conducted a series of experiments to understand how price transparency, paid ranking and information about terms and conditions can be improved to increase consumer comprehension.
Price transparency
Experiment 1
- Experiment design: The study used an online experiment where 1,208 participants had to do research either for booking a holiday or purchasing a new mobile plan on a fictitious website. They randomly assigned participants to see one of 3 ways of presenting additional fees that were not presented as part of the price: i) using a clickable i-symbol next to the price; ii) using clickable text such as “excluding booking fees”; or iii) presenting the extra costs directly next to the price.
- Findings: Only 2% of participants clicked on i-symbols to access the total price of products, and participants were more likely to report they were certain there were additional costs when fees were presented next to the price than when behind an i-symbol (25% vs. 9% when booking a holiday; 25% vs. 7% when purchasing a new mobile plan). The study also found providing additional costs up front did not affect reported purchase intentions or level of trust in the seller.
Experiment 2
- Experiment design: The study involved an online experiment in which 1,012 participants were asked to book a holiday home on a fictive website. Participants’ behaviour on this website was monitored to see which icons were clicked or which holiday homes were viewed. Following the simulated purchase, participants received a questionnaire. Participants were divided into 2 experimental groups, the first group received a version of the website in which prices were shown without additional costs (e.g. tourist tax or mandatory bedding, cleaning costs). These costs were only visible when clicking an “i-symbol” and were shown later in the booking process. The second group received a version of the website in which mandatory additional costs were included in the price.
- Findings: Results show that showing incomplete price information has a negative impact on the experience of participants on the website. Additional costs were only clicked by 15% of participants when using an “i-symbol”. The price was perceived as less clear, comparing holiday homes was more difficult and decision making was hindered. This finding was supported by the time spent on the fictive website: untransparent prices led to an increase of 20% in time spend to complete the booking compared to the transparent price website. Trust in the website was lower, and more participants felt they were misled. Untransparent prices did not lead to different choice outcome in this experiment, but participants did report lower satisfaction with their choice.
The ACM’s Behavioral Insights Team then collaborated with 3 holiday house providers to evaluate effects of transparent prices in live websites. Results confirmed the experiences with the fictive website. Customers preferred seeing complete prices from the start of the booking process. This led to more satisfaction with the booking process and fewer questions at the customer support desk. In some cases, a slight decrease in conversion was seen when prices were transparent. The ACM suggested this may be caused by a lack of level playing field; websites showing additional prices later in the booking process may initially seem cheaper when they in fact are not. Nonetheless, all 3 holiday house providers decided on the basis of these experiments to show prices including mandatory additional costs.
Paid ranking
- Experiment design: The ACM conducted a natural experiment with an undisclosed online platform. In 2019, the platform started to add a disclosure label about sponsored search result rankings of those suppliers that paid extra for a better position. For a period of time, the label was only present on the website, and not in the app. As the ranking mechanism was identical between the website and app, this setting created a natural experiment on the effects of the label.
- Findings: ACM found that the introduction of the label had no statistically significant effect on the extent to which consumers buy at suppliers that sponsor their position. For the subset of inexperienced consumers (defined as buying no more than once in our dataset), ACM found that consumers buy less from suppliers sponsoring their position, although the effect is economically small (a relative decrease of 2.6 per cent). The ACM suggested a number of potential explanations as to why the effect of the transparency on purchases at sponsoring suppliers was so small: i) consumers may not have noticed the label, ii) consumers did not understand the label, and/or iii) consumers did not consider the results’ sponsored nature relevant for their order decision.
Experiment 4
- Experiment design: Following the natural experiment, the ACM conducted a study involving a survey followed by an online experiment, both of which involved showing participants a recreated online platform displaying businesses offering services. In the survey, participants were asked to make a purchase on the website, then to complete a questionnaire. In the online experiment, participants were randomly presented with 5 different versions of the “sponsored” tag: i) “sponsored” (the control group); ii) “paid position” and using an eye-catching colour; iii) “paid position”, eye-catching colour and moving the tag to a more salient position; iv) “paid position”, eye-catching colour, salient position and a clickable i-symbol (provides more information about the meaning of “paid position”); or v) “paid position”, salient position and an explanation of “paid position” at the top of the page. They were then asked a series of questions.
- Findings: Participants were more likely to correctly describe what the tag meant when they used “paid position” as opposed to “sponsored” (50-55% vs. 32%), and to report that they took the tag into account in their decision (31-35% vs. 14%). Participants were also more likely to remember the tag when they used an eye-catching colour and moved the tag to a salient position (31% vs 9%).
Information about terms and conditions
Experiment 5
- Experiment design: The study involved an online experiment where 2,208 participants were asked to imagine they were going to purchase a laptop or a sweater from a fictitious online store. They were then randomly assigned to see one of 4 different versions of a payment page varying how terms and conditions are presented: i) a checkbox confirming they have read the terms and conditions, with the option to click and read them (the control group); ii) a summary of the terms and conditions using visual symbols; iii) an urgency notification that this was the last chance to read the terms and conditions before the purchase; or iv) both a visual symbol summary and an urgency notification. They were then asked a series of questions.
- Findings: Participants were more likely to give the correct answer to questions around comprehension of the terms and conditions in the 3 treatment conditions vs. the control group (35-47% vs. 32%). They were also more than 3 times more likely to view the terms and conditions when prompted with an urgency notification than in the control group (30% vs. 9%).
7.2 Case study 2: Danish Konkurrence- og Forbrugerstyrelsen (KFST) online experiments into paid advertisement disclosures
Despite disclosure of commercial intent being mandatory by law on social media in the EU, commercial content (for example by paid influencers) can be difficult to discern from other types of social content. The KFST conducted a behavioural study with 2 online experiments to explore consumers’ ability recognise commercial content on social media.
Experiment 1: Children’s understanding of video-based commercial content
- Study design: 1,463 children aged 6-12 participated in an online experiment where they were asked to watch 4 types of videos in a random order: i) natural influencer commercials; ii) unboxing videos; iii) non-commercial influencer videos; and iv) traditional TV-like commercials. Half of the children were randomly selected to see content labelled as prescribed by current guidelines, while the other half saw content labelled with a more salient commercial disclosure (designed using a co-creation exercise with children and tested in a qualitative study). After seeing each type of video, children were immediately asked to indicate whether they thought the video they had just seen was an advertisement, then presented with different statements about why the video had been made and asked which ones they agreed with. At the end of the experiment, children were entered into a lottery content where they could win a prize of their choice. They could choose from 6 items (presented in a random order), of which half were advertised in the videos seen in the experiment and half were similar items not seen in the videos.
- Findings: The more salient commercial disclosure increased the likelihood children correctly identified natural influencer commercials and unboxing videos (from 62% to 70% and 73% to 77% respectively). This effect was primarily driven by younger children from age 6-10, helping them to perform at a level similar to older children. The more salient disclosure also helped children aged 6-8 to identify commercial intent for both natural influencer commercials and unboxing videos. However, children were still more likely to prefer items they have just seen in a natural influencer commercial or unboxing video, regardless of whether or not they saw the more salient disclosure.
Experiment 2: Adults’ awareness of the commercial intent of posts on Instagram
- Experiment design: 1,410 adults participated in a 2-part online experiment. In the first part, participants were presented with an Instagram feed with 6 posts from known Danish influencers, of which one post was an advertisement. Participants were randomly assigned to receive one of 7 versions of the news feed that varied in how the sponsored post’s commercial intent was disclosed through wording (“paid partnership” vs. “advertisement”), placement (above or below the image), whether wording was highlighted, and whether the post was surrounded by a yellow frame. Participants were then asked whether any of the posts they had just seen had commercial intent. In the second part, participants saw a feed of 20 Instagram posts, of which 6 were disclosed as having commercial intent. Again, they randomly received one of 7 variations of news feeds that combined different disclosure styles from the first part of the experiment, and then were asked to identify the commercial posts.
- Findings: In the first part, 52% of Instagram users and 36% of non-users reported having seen “paid” content. Adding a yellow frame increased the share of participants noting commercial intent (from 49% to 65%), as did highlighting the wording, but only when placed below the post (from 44% to 62%). Placement or wording did not have an impact. In the second part, standardised disclosure styles across the 6 commercial posts increased the average number correctly identified by participants (from 4.3 to 4.7 out of 6), and the likelihood Instagram users were able to correctly identify all 6 (from 51% to 62%).
7.3 Case study 3: Ofgem field experiments testing switching remedies
In 2016, the CMA conducted an Energy Market Investigation where we identified weak customer response as having an adverse effect on competition, driven by limited awareness of the ability to switch, barriers to accessing and assessing information and barriers to switching. The CMA therefore recommended a series of switching remedies to Ofgem, including maintaining a database of disengaged consumers and providing consumers with details of cheaper tariffs on the market. The CMA also recommended Ofgem should carry out a series of field experiments to test these remedies’ effectiveness.
Experiment 1: Disengaged customer database remedy
- Experiment design: 2,400 customers from 2 large energy suppliers who had not switched for more than 3 years were randomly assigned to one of 3 intervention groups: i) no letter (the control group); ii) marketing letters from other suppliers; or iii) a best offer letter from Ofgem. They then measured the likelihood that customers initiated a switch after receiving the letter to the end of the assessment period (around 3 months).
- Findings: Both the marketing letters and the best offer letter increased the likelihood that customers requested switching in the assessment period (from 7% to 13% and 12% respectively), which on average was estimated to save customers around £131 per year.
Experiment 2: Cheaper Market Offers Letter remedy
- Experiment design: 137,876 customers from 2 large energy suppliers were randomly assigned to one of 3 intervention groups: i) no letter (the control group); ii) an Ofgem-branded letter displaying 3 cheaper, personalised tariffs offered by rival suppliers (a Cheaper Market Offers Letter, or CMOL); or iii) a current supplier-branded CMOL.
- Findings: Receiving either an Ofgem- or supplier-branded CMOL increased the likelihood that customers switched, and supplier-branded CMOLs were more effective than Ofgem-branded (3.4% vs. 2.4% vs. 1%). CMOLs were also more effective for customers who had switched less frequently, suggesting they have the potential to engage more ‘sticky’ customers, and customers who received a CMOL and switched saved on average £50 more than customers who did not receive a CMOL and switched.
7.4 Case study 4: Financial Conduct Authority (FCA) field experiment testing an intervention encouraging pawnbroking customers to claim surplus money
In 2018, the FCA identified that pawnbroking customers were not always collecting the ‘surplus’ money owed to them. Surplus money is generated when a customer defaults on their loan and their pawned item is sold at auction for more than what is owed by the customer. Although there is a process for notifying customers when a surplus is generated, collection rates are low. In partnership with one of the largest pawnbroking firms in the UK, the FCA mapped out the typical consumer journey, conducted staff and customer interviews and combined these insights with analytical data work to design an intervention, which was subsequently tested in a field experiment.
- Experiment design: 569 customers that had incurred a surplus from an item being sold at auction were randomly allocated to receive, or not to receive, a behaviourally-designed reminder letter 14 days post-auction. The letter communicated that the customer was owed money in a simple, visually-direct way, and was sent in a blue envelope to attract attention.
- Findings: Receiving the letter increased the likelihood that customers collected their surplus from 11% to 22%. They did not find evidence, however, that the reminder letter had a greater impact on repeat customers or for higher surplus amounts.
The FCA has run a number of field experiments to date, the results of which can be found in their Occasional Papers series.
8. Annex 2: Internal and external validity
Internal validity refers to the extent the results from an experiment support its stated claim about causality within the experiment sample and context. The internal validity of experiments is threatened if the result could be explained by something other than the interventions: for example, if there are unobservable systematic differences between the participants in each group that can explain differences in outcomes, or if outcome measures are not reliable or consistent. Online experiments and field experiments both have high internal validity if designed and executed well, as the purpose of randomisation is to ensure there are no significant, systematic differences between intervention groups (either on observable or unobservable characteristics). This means differences in outcomes between groups can be reasonably attributed to differences in the interventions.
External validity refers to the extent results can be generalised beyond the experiment context: either i) to wider populations (population validity), or ii) in wider settings in the real world (ecological validity).
- Population validity: It is not always practical, possible or ethical to conduct experiments on the full population of interest, so it is common to select a representative sample to take part and then apply the results to populations of interest beyond the sample. This could mean the population from which a sample was directly drawn; the population a sample was intended to represent; or tangentially-related populations. Experiments have high external validity when the experiment sample is representative of the population of interest – ideally where we can randomly select our sample from this population. However, this is not always possible for a number of practical reasons. For example, we might only be able to conduct experiments with customers from a subset of firms in a market but wish to extrapolate the results to a larger set of firms. Sometimes we may not know much about our population of interest (which could be “all consumers”) and so a nationally representative sample may be suitable. The external validity of these experiments needs to be judged on a case-by-case basis, including how it is weighed against practical considerations.
- Ecological validity: We might also want to apply the results to different decision-making settings or situations in the real world. For example, an online experiment might test hypothetical consumer decision making which is intended to simulate some kind of real decisions they make. The closer to reality the experimental design is, the more externally valid it will be, so we ideally want to replicate as closely as possible how an intervention will be seen, and how consumers would behave, in the real world.
8.1 Field experiments vs. online experiments
Field experiments are generally held to be more externally valid than online experiments, both with respect to generalising to other populations and settings, as they involve real consumers making real decisions in the course of normal life. There are some important factors that are likely to affect online experiments’ generalisability:
- Depending on the recruitment method, participants who are willing to take part in an experiment might not be representative of consumers from a target population. While the sample may be representative in terms of its demographic profile, it may be biased in different ways: for example, evidence suggests that online panellists spend more time on the internet and engage more actively than other consumers in searching for better deals online.[footnote 39]
- As participants know their behaviour is being observed in an experiment by researchers, they may behave differently to how they would in real-life (the ‘Hawthorne effect’), such as paying more or less attention to information[footnote 40], or acting more or less pro-socially or ethically than they otherwise would.[footnote 41]
- Some determinants of real consumer decision making can be difficult to replicate online or, more generally, in a laboratory setting. For example, online experiments are less likely to be able to replicate choices where consumers are influenced by others or are under stressful or emotional conditions, or where choices are high stakes, involve high levels of personalisation, include large choice sets, are repeated, or are made over a long time horizon.[footnote 42]
There are also a number of factors that can make the findings of an online experiment sufficiently externally valid for certain purposes:
- In real life, consumers increasingly make decisions in an online environment, which can be closely replicated in an online experiment.
- Online experiments can provide a particularly good understanding of underlying behavioural mechanisms for 3 reasons:
- There is evidence that, although there is some variation, behavioural biases are experienced relatively consistently across different populations[footnote 43] and over time.[footnote 44] This means external validity is likely to be higher for online experiments seeking to understand underlying behavioural mechanisms.
- Online experiments give us more control over important contextual factors than field experiments, which helps us to better isolate and understand general principles driving behaviour and decision making. For example, if we are interested in the effectiveness of information provision in increasing consumer understanding, we can control for the amount of time someone spent reading the information.[footnote 45] If we are interested in the effectiveness of information provision in transient media, for example, television or radio, we can restrict the time participants can attend the information.[footnote 46])
- As this approach means decisions might be abstracted from the real world, Kessler and Vesterlund (2015) suggest emphasising experiments’ qualitative external validity (i.e. the direction of an effect), rather than their quantitative external validity (i.e. the magnitude of an effect).[footnote 47]) This means, for example, if we are comparing different remedy designs, should more confident that the most effective remedy in an online experiment would also be most effective in the real world, than we would be about the size of that effect.
- Camerer (2011) suggests that studies generally tend to find behaviours or outcomes are consistent between field experiments and laboratory experiments that are designed with generalisability in mind.[footnote 48] He suggests that there are few studies that are deliberately designed to generalise from the laboratory to the field, but only finds one of these that shows reliable evidence of poor generalisation when experiment features are closely matched. He also identifies a wider range of experiments that use different samples to compare behaviour in field experiments and laboratory experiments designed to have similar features to the field, and finds over 20 examples with good comparability and only 2 with poor comparability.[footnote 49]
- There are ways we can increase online experiments’ external validity. For example, to address the effect of being observed, we could emphasise to participants the anonymity of results, or replicate limited attention by asking the participants to complete cognitively demanding tasks, such as memory, arithmetic or logic tasks.
We would consider the balance of all of these factors when judging the external validity of an online experiment for a given population or setting, and in the context of the relevant research questions.
-
Quasi-experimental approaches include natural experiments (where an external event results in random-like assignment of subjects to treatment), propensity score matching (comparing individuals that match on observable variables) and regression discontinuity (comparing participants who fall just above and below an artificial threshold that determines whether they receive an intervention). ↩
-
For more detail, see the BEIS consultation document and government response for “Reforming Competition and Consumer Policy”. In its response, the government said it would be ‘creating more flexible and versatile remedies in market investigations’ by ‘enabling the CMA to require businesses to conduct trials to determine the final format of certain remedies’. On 17 November 2022, the Chancellor said in his Autumn Statement that the government is ‘bringing forward the Digital Markets, Competition and Consumer Bill to provide new powers to the ‘Digital Markets Unit’ (DMU) in the CMA to foster more competitive digital markets; make changes to the competition framework that will include streamlined decision making and updating merger and fine thresholds; and protect consumers in fast-moving markets by tackling ‘subscription traps’ and fake reviews online’. ↩
-
For more detail, see the BEIS and DCMS consultation document and government response for “A new pro-competition regime for digital markets”. The government response said that the DMU ‘will be able to … require that firms carry out field trials (including A/B testing) to evaluate the impact of new innovations or processes if necessary’; and that ‘[t]he DMU will be able to take a flexible approach to imposing pro-competitive interventions, … [including] trialling and iterating new remedies’. As noted above, the government is bringing forward the Digital Markets, Competition and Consumer Bill to provide new powers to the CMA to oversee digital markets. ↩
-
For a discussion of some of the limitations of randomised controlled trials and why they should be used in combination with theoretical models and other methodologies, see Deaton, A., & Cartwright, N. (2018). Understanding and misunderstanding randomized controlled trials. Social Science & Medicine, 210, 2-21. Though note that we do not endorse all their views. ↩
-
See the FCA’s reports: When and how we use field trials, 2018 and Occasional Paper 51: Using online experiments for behaviourally-informed consumer policy. ↩
-
See Ofgem’s Collective Switch trials for more details. ↩
-
See Ofcom’s consultation: Trialling consumer remedies for more details. ↩
-
See the Danish KFST online experiments into paid advertisement disclosures and the Dutch ACM’s online experiments into price transparency, paid ranking and product information. We also present a summary of these experiments as case studies in Annex 1. ↩
-
The Behavioural Insights Team are a social purpose company, originally founded in the Cabinet Office in 2010, who generate and apply behavioural insights to public policy. ↩
-
See the World Bank report on Behavioural Science Around the World for profiles of 10 countries. ↩
-
For example, Uber’s Experimentation Platform claims to run over 1,000 experiments at any one time, and Google ran over 700,000 experiments just in search in 2021. ↩
-
See the UK Competition Network (CMA and FCA) report on demand-side remedies for more detail. ↩
-
Although many people chose higher direct debit amounts, this was offset by lower subsequent manual payments and lower likelihood of setting up a payment at all. See the FCA’s report here for more details. ↩
-
For example, the CMA analyses firm data using data science techniques; conducts legal and economic analyses using historical cases and market data; runs and commissions market research surveys and qualitative research; conducts web scraping; and reviews existing academic evidence, as needed for different cases. These approaches might help to answer questions such as why a practice is effective; how it is experienced by consumers; how effective it is in specific vulnerable populations; how effects might change in a dynamic environment; what the legal implications are of previous case law; or how economic pressures might impact effectiveness. ↩
-
The statutory deadlines for different types of CMA investigations are as follows: market studies must provide notice of a proposed decision for a market investigation reference within 6 months, and publish the final report within 12 months from commencement; market investigation references must be completed within 18 months (extendable by up to 6 months for special reasons); merger reviews must complete Phase 1 within 40 working days, Phase 2 within 24 weeks (extendable by up to 8 weeks for special reasons) and make an order or accept undertakings to give effect to Phase 2 remedies within 12 weeks. For more information, see Market Studies and Market Investigations: Supplemental Guidance on the CMA’s Approach and A Quick Guide to UK Merger Assessment. ↩
-
Probability-based approaches to recruitment take additional steps to include those unable to participate online and other underrepresented groups compared to convenience panels. For example, see NatCen’s probability-based panel. ↩
-
For a review of the available evidence, see Cornesse, C., Blom, A. G., Dutwin, D., Krosnick, J. A., De Leeuw, E. D., Legleye, S., Pasek, J., Pennay, D., Phillips, B., Sakshaug, J., Struminskaya, B. & Wenz, A. (2020). A review of conceptual approaches and empirical evidence on probability and nonprobability sample survey research. Journal of Survey Statistics and Methodology, 8(1), 4-36. Also see the American Association of Public Opinion Research’s 2010 report on online panels, which concludes that “there currently is no generally accepted theoretical basis from which to claim that survey results using samples from nonprobability online panels are projectable to the general population”. ↩
-
See CMA. (2018). Good practice in the design and presentation of customer survey evidence in merger cases. ↩
-
Hargittai, E., & Shaw, A. (2020). Comparing internet experiences and prosociality in amazon mechanical turk and population-based survey samples. Socius, 6, 2378023119889834. ↩
-
For example, see the discussion of justifications for nonprobability approaches in Cornesse, C. et al. (2020). A review of conceptual approaches and empirical evidence on probability and nonprobability sample survey research. Journal of Survey Statistics and Methodology, 8(1), 4-36. ↩
-
Coppock, A., Leeper, T. J., & Mullinix, K. J. (2018). Generalizability of heterogeneous treatment effect estimates across samples. Proceedings of the National Academy of Sciences, 115(49), 12441-12446. The authors found population treatment effects from online convenience sample survey experiments on Amazon Mechanical Turk closely mirrored those from nationally representative samples. ↩
-
See Scherpenzeel, A. C. (2018). How representative are online panels? Problems of coverage and selection and possible solutions. In Social and behavioral research and the internet (pp. 105-132). Routledge. ↩
-
Ie there is an 80% probability that we correctly find the intervention was effective when there was an effect. ↩
-
Ie there is a 5% probability that we find the intervention was effective when in fact there was no effect. ↩
-
See our mergers customer survey design and presentation good practice for more details. ↩
-
See for example Hainmueller, J., Hangartner, D., & Yamamoto, T. (2015). Validating vignette and conjoint survey experiments against real-world behavior. Proceedings of the National Academy of Sciences, 112(8), 2395-2400. ↩
-
For a discussion of the intention-behaviour and attitude-behaviour gaps, see Sheeran, P. (2002). Intention—behavior relations: a conceptual and empirical review. European Review of Social Psychology, 12(1), 1-36. ↩
-
Arechar, A. A., Gächter, S., & Molleman, L. (2018). Conducting interactive experiments online. Experimental Economics, 21(1), 99-131 ↩
-
For examples of potential solutions where online panels are not fully representative, see Scherpenzeel, A. C. (2018). How representative are online panels? Problems of coverage and selection and possible solutions. In Social and behavioral research and the internet (pp. 105-132). Routledge. ↩
-
See Oppenheimer, D. M., Meyvis, T., & Davidenko, N. (2009). Instructional manipulation checks: Detecting satisficing to increase statistical power. Journal of experimental social psychology, 45(4), 867-872. ↩
-
FCA. (2018). When and how we use field trials. ↩
-
See the Open Science Framework website. ↩
-
See Transparency and Disclosure: Statement of the CMA’s policy and approach (CMA6. January 2014). ↩
-
Publication bias describes the problem in academia where research is more likely to be published if it gives a significant result. See Section 2 in FCA Occasional Paper 23 for a discussion of publication bias as relevant for regulation. ↩
-
See Publishing research and analysis in government GSR Publication Protocol for more detail. ↩
-
See Guidance to implement the Concordat to Support Research Integrity within Government for more detail. ↩
-
See Transparency and Disclosure: Statement of the CMA’s policy and approach (CMA6. January 2014). ↩
-
See Transparency and Disclosure: Statement of the CMA’s policy and approach (CMA6. January 2014). ↩
-
For a discussion of the representativeness of Amazon’s Mechanical Turk recruitment platform for political research as an example, see Berinsky, A. J., Huber, G. A., & Lenz, G. S. (2012). Evaluating online labor markets for experimental research: Amazon.com’s Mechanical Turk. Political analysis, 20(3), 351-368. ↩
-
For a discussion of the impact of incentives in experiments, see Camerer, C. F., & Hogarth, R. M. (1999). The effects of financial incentives in experiments: A review and capital-labor-production framework. Journal of risk and uncertainty, 19(1), 7-42; and Smith, V. L., & Walker, J. M. (1993). Monetary rewards and decision cost in experimental economics. Economic Inquiry, 31(2), 245-261. ↩
-
For example, see Andreoni, J., & Bernheim, B. D. (2009). Social image and the 50–50 norm: A theoretical and experimental analysis of audience effects. Econometrica, 77(5), 1607-1636. ↩
-
For a discussion of choice set and time horizon restrictions, see Levitt, S. D., & List, J. A. (2007). What do laboratory experiments measuring social preferences reveal about the real world?. Journal of Economic perspectives, 21(2), 153-174. ↩
-
Stango, V., & Zinman, J. (2020). We are all behavioral, more or less: A taxonomy of consumer decision making (No. w28138). National Bureau of Economic Research. ↩
-
Stango, V., & Zinman, J. (2020). Behavioral biases are temporally stable (No. w27860). National Bureau of Economic Research. ↩
-
See Falk, A., & Heckman, J. J. (2009). Lab experiments are a major source of knowledge in the social sciences. science, 326(5952), 535-538. ↩
-
See KFST (2021). Consumers benefit from simplified information disclosure ↩
-
Kessler, J., & Vesterlund, L. (2015). The external validity of laboratory experiments: The misleading emphasis on quantitative effects (Vol. 18, pp. 392-405). Oxford, UK: Oxford University Press. We note that their discussion is in the context of lab experiments, which hold many parallels with online experiments. ↩
-
See Camerer, C. (2011). The promise and success of lab-field generalizability in experimental economics: A critical reply to Levitt and List. ↩
-
Camerer, C. (2011). The promise and success of lab-field generalizability in experimental economics: A critical reply to Levitt and List. ↩