Open RAN in High Demand Density Environments Technical Guidance
Updated 6 April 2023
1. Introduction
Although High-Density Demand (HDD) areas represent a small proportion of all network sites, they carry a significant volume of overall network traffic. The unique technical and commercial challenges of these network environments mean that they drive innovation of leading-edge technical features and performance capabilities, which is then deployed more extensively across the network as demand rises, ultimately improving the quality of experience of all customers.
As part of HM Government’s ambition to carry 35% of the UK’s mobile network traffic over open and interoperable RAN architectures by 2030, it is therefore looking at the specific needs and requirements of HDD areas, and how these can be addressed through Open RAN solutions.
This document aims to support this wider programme by developing a set of indicative technical descriptors of HDD areas, together with a snapshot of the typical performance expectations that a mobile network operator may have for equipment used within these environments. The intention is to provide UK telecom equipment vendors with indicative features and performance figures with which to inform their RAN related product development roadmaps relating to UK public 5G networks in HDD areas.
The UK’s Open RAN Principles state that interoperable solutions should demonstrate performance at a minimum, equal to existing RAN solutions. This document supports the journey to develop Open RAN products that are not only highly secure and performant, but also adherent to those principles and will deliver sustainable vendor diversity and network supply chain resilience for 5G and into the future.
HDD areas and their evolution over time
Chapter 2 of this document suggests a set of defining characteristics of HDD scenarios, such as user density (users/km2), traffic density (Gbps/km2) and peak and average DL and UL data transfer rates (Gbps per cell and Mbps per user). It then identifies use cases and the respective individual traffic requirements for dense urban areas, as well as specific locations such as airports, sports venues, rail and subway stations and major public events, and examines traffic profiles and evolution in coming years.
Headline findings from this section include that:
- Although HDD areas are geographically small, they drive significant volumes of mobile traffic. Around 1% of UK geographical areas served by mobile networks could be characterised as HDD. However, these areas drive a high proportion of the nation’s total mobile traffic: an estimated 20% of total mobile traffic and 7% of the highest value macrocells.
- More geographical areas are becoming high-density. By the end of the decade, the area of the UK with traffic density equivalent to today’s HDD areas is set to more than double - with traffic density in today’s HDD areas also doubling in the same period.
- Yearly data traffic from high-density areas is set to rise multiple times. By the end of the decade, yearly data traffic could potentially rise 5 or 10 times - a high-growth scenario could see traffic increase from just under 2 Exabytes today to a potential 26 Exabytes.
Key HDD technical challenge areas
Chapter 3 of this document outlines the challenge areas relating to Open RAN network deployment in HDD environments that have been suggested through our research with multiple sources including MNOs, SIs, vendors and chipset OEMs. These include the following.
Challenge Areas | Main Open RAN challenges in HDD |
---|---|
Fronthaul (O-FH) | Open Front-haul security and performance; Support for 7.2x split for macro layer; O-RU alignment on variations due to options and complexity (O-RU & O-DU need to reflect same category option) |
Chipsets | Lack of diversity in processor suppliers; Power efficiency of solution when using general purpose processors; Restriction of Open RAN component software products (i.e., DU, CU) to specific underlying hardware. |
Massive MIMO (mMIMO) | Capacity and service quality in HDD areas; Performance parity with the traditional RANs for mMIMO products. |
Mobility | Entering and leaving specific handover phases before completion (ping-pong effect) - this will create unnecessary messaging across backhaul reducing available capacity; Consecutive handovers between the same cells; Ensuring load is properly balanced between overlapping network layers to ensure best use of capacity resources. |
Energy Efficiency | Processing demand and energy consumption is highest in HDD areas due to complexity of multiple signal path computations and the number of users. |
Security | Open architecture provides a larger attack surface - in HDD, vulnerabilities can expose a large number of users and a large volume of data. |
Interoperability | Performance of Open RAN has not yet reached parity with traditional single RAN provider solution. In HDD areas consistent data compatibility and support processes for heterogeneous equipment across macro layer and small cell solutions will be needed. |
RAN Intelligent Controller (RIC) | Automation of RAN operations and enhancement of RAN resources usage through use cases. |
Indicative performance expectations
Finally, Chapter 4 develops an indicative set of performance levels that Open RAN equipment may need to meet, if it is to help networks address the areas of challenge set out in the preceding chapter. This looks at areas such as RAN architecture, software features, RU performance, Open fronthaul performance, Open Cloud, DU/CU and RIC.
How this document should be used
The content in this document is drawn from detailed analysis of traditional RAN and Open RAN reference material, and has been informed by the input from key UK and global Telecoms market stakeholders. Our intention in releasing this data is that it will help contribute to, and inform, the cross-industry conversation about the importance of HDD areas, by helping to quantify in numerical terms just what the ‘high-performance’ expectations of equipment used in these areas might be. We would welcome discussion and debate as to the areas in which these figures are - and are not - helpful or accurate.
We recommend you obtain professional advice before acting or refraining from action on any of the contents of this publication, and accept no liability for any loss occasioned to any person acting or refraining from action as a result of any material in this publication. The views expressed are those of the report author and do not necessarily reflect the views or positions of the UK MNOs or others who have provided input for consideration.
2. Defining HDD Areas and Their Evolution Over Time
2.1 HDD definition
The term “High Demand Density” (HDD) is intended to represent areas with the most stretching demands on functionality and performance due to environment, traffic and user concentration, service mix and quality of experience targets. The key parameters for HDD areas and scenarios classification are user density, traffic density, and the average and peak downlink and uplink data transfer rates (per cell and per user). The below table shows these parameters.
Metric | Units | Description |
---|---|---|
User Density | Users/km2 | Number of active users in a specific geographical area at the same time. (Active = data/voice transfer active). Most HDD scenarios occur in situations where a lot of users and devices are present in a specific place or event, putting intense demand on the network. |
Traffic Density | Gbps/km2 | The traffic density measures the ratio between the data transfer rate and the geographical area of a particular event/situation. In HDD scenarios, there are high data volumes per square metre due to the use of social networking and video streaming transfer services (among others) that put high traffic demands on the network. |
Peak and average DL and UL Data Transfer Rates (per cell and per user) | Gbps or Mbps | High peak downlink or uplink transfer data transfer rates can cause network congestion and reduce the user QoE. High average downlink or uplink transfer data transfer rates in a specific event indicates intense network usage across the period. |
2.2 HDD scenario analysis
HDD scenarios occur in dense urban areas as well as in specific locations including airports, sports venues, railway and subway stations and major public event venues.
However, specific network requirements vary between these locations. A crowded sports stadium for example, is expected to have a higher average user density than a train station, and a different uplink and downlink usage. The tables below consolidate information from various data sources to show typical values for HDD scenario parameters.
Scenario | Experienced DL data rate | Experienced UL data rate | DL Data traffic capacity | UL Data traffic capacity | Total Data traffic capacity | User density | Activity factor | UE speed (mobility) |
---|---|---|---|---|---|---|---|---|
Urban Macro | 50 Mbps | 25 Mbps | 100 Gbps/km2 | 50 Gbps/km2 | 150 Gbps/km2 | 10k users / km2 | 20 % | Pedestrians and users in vehicles (up to 120 km/h) |
Dense Urban | 300 Mbps | 50 Mbps | 750 Gbps/ km2 | 125 Gbps/ km2 | 875 Gbps/km2 | 25k users/ km2 | 10 % | Pedestrians and users in vehicles (up to 60 km/h) |
Broadband Access in a Crowd | 25 Mbps | 50 Mbps | 3.75 Tbps/km2 | 7.5 Tbps/km2 | 11.25 Tbps/km2 | 500k users/km2 | 30 % | Pedestrians |
A numerical analysis of each identified HDD scenario is presented below. Where available, UK-specific figures are used; otherwise, information from a European operator is used. The figures are on a “per MNO” basis for an MNO with 30% market share in that region.
Scenario | Number of Users | Area | User Density | UK MNO Traffic Volume | UK MNO Traffic Volume (2030) | Event Duration | Traffic Density (2023) | Expected Traffic Density (2030) |
---|---|---|---|---|---|---|---|---|
Airports | 2k | 0.1 km2 (Heathrow Airport) | 20k users/km2 | DL: 5 TB, UL: 1 TB | DL: 57 TB, UL: 11.4 TB | 8 hours | DL: 13.9 Gbps/km2 (1.4 Gbps/airport), UL: 2.8 Gbps/km2 (0.3 Gbps/airport) | DL: 158.3 Gbps/km2 (15.8 Gbps/airport), UL: 31.7 Gbps/km2 (3.2 Gbps/airport) |
Stadiums and Arenas | 17.7k (Stadium Capacity) | 0.036 km2 (Etihad Stadium) | 490k users/km2 | DL: 1.42 TB, UL: 2.64 TB | DL: 16.2 TB, UL: 30.1 TB | 3 hours | DL: 26.4 Gbps/km2 (1.1 Gbps/stadium), UL: 49.0 Gbps/km2 (2.0 Gbps/stadium) | DL: 333.1 Gbps/km2 (12.0 Gbps/stadium), UL: 619.3 Gbps/km2 (22.3 Gbps/stadium) |
Rail and Subway Station | 0.7 k | 0.02 km2 | 35k users/km2 | DL: 11.4 GB, UL: 1.2 GB | DL: 129.5 GB, UL: 14.1 GB | 15 min | DL: 0.2 Gbps/km2 (0.008 Gbps/station), UL: 0.02 Gbps/km2 (0.0009 Gbps/station) | DL: 57.6 Gbps/km2 (1.2 Gbps/station), UL: 6.3 Gbps/km2 (0.1 Gbps/station) |
Public Events | 1.7k | 0.024 km2 (South Bank Centre New Year’s Eve) | 70k users/km2 | DL: 1.2 TB, UL: 2.2 TB | DL: 13.7 TB, UL: 25.1 TB | 7h | DL: 15.9 Gbps/km2 (0.4 Gbps/event), UL: 29.1 Gbps/km2 (0.7 Gbps/event) | DL: 180.9 Gbps/km2 (4.3 Gbps/event), UL: 331.8 Gbps/km2 (8.0 Gbps/event) |
- ‘Public events’ uses European MNO data traffic as a reference;
- ’ UK MNO traffic volume’ is calculated using Ofcom’s Mobile networks and spectrum - Meeting future demand for mobile data high growth scenario (using a 55% of growth until 2030).
- ‘Number of users’ refers to simultaneously attached users.
2.3 Estimated traffic in HDD areas
Global mobile traffic is expected to grow from year to year, and UK traffic is expected to follow the same pattern. Traffic will grow through an increase in devices driven by IoT services and through growth in mobile data traffic per device attributed to three main drivers: improved device capabilities, an increase in data-intensive content and growth in data consumption due to continued improvements in the performance of deployed networks.
Ofcom’s “Mobile networks and spectrum” report (February 2022) modelled three scenarios (high, medium and low) for mobile data traffic growth rates. Noting that growth rates have been generally static or reducing over time, the medium and low growth rates (25% per annum and 40% per annum) are considered more likely bounds for traffic forecasts, compared with the high rate, and so the medium and low rates from this reference are used in this section of analysis.
Figure 1 below shows the estimated traffic growth for HDD areas for each of the two growth scenarios. The key assumptions for the graph are:
- 2022 UK mobile traffic (total in year) for urban areas is estimated at 3852 Petabytes (the Connected Nations 2022 Ofcom Report - 321 petabytes, monthly mobile data traffic in UK urban areas);
- The traffic in HDD areas represents 46% of the urban traffic; based on the Ofcom report, this is the typical proportion of an urban area where traffic density is greater than 0.31 Gbps per km2. (This is the proposed HDD threshold based on consideration of traffic in rail and subway stations as HDD, and corresponds to approximately 140 GB per hour per km2).
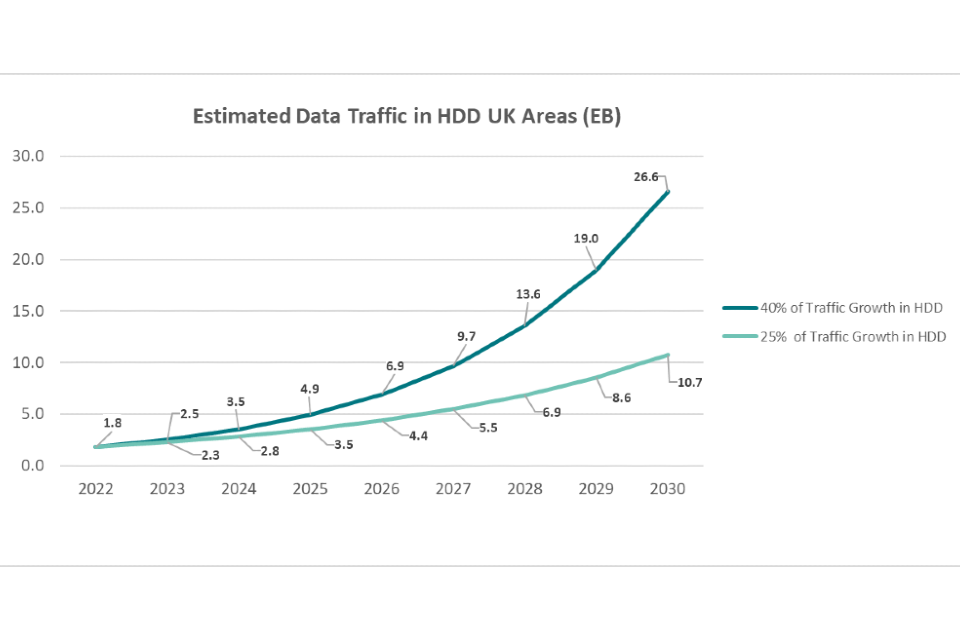
The total traffic captured in HDD areas is estimated to be as follows, (1 ExaByte = 1 000 PetaBytes = 1 000 000 TeraBytes or 1 000 000 000 (billion) GigaBytes)).
Growth scenario assumed | 2025 | 2030 |
---|---|---|
25% | 3.5 EB | 10.7 EB |
40% | 4.9 EB | 26.6 EB |
2.4 UK geography evolution of HDD areas
The proportion of HDD areas is expected to increase in the future, primarily due to the increase in traffic density driven by the number of 5G compliant devices and the emergence of more demanding mobile services.
For the purposes of estimating the growth of HDD areas, Ofcom data on monthly mobile traffic has been used to provide consistent data on area sizes and usage, and support an estimation method from available figures. This data:
- Gives a view of the size of UK urban areas, assuming that an urban area is an area where the monthly data traffic is greater than 1 PB (1024 Terabytes) per 100 km2. It can then be estimated how the size of urban areas will change assuming a 25% traffic growth rate (the areas with traffic greater than 1PB per 100 km2 will increase due to traffic growth).
- Allows the calculation of HDD area as a percentage of the total urban area by examining the area figures for Manchester and London and applying that ratio to the calculation for urban area size (the proportion of the urban area for these locations where there is a total average hourly data traffic of more than 140 GB per km2).
The below figure shows the forecasted evolution of the proportion of UK HDD areas for the years 2023, 2025 and 2030 based on the assumptions above.
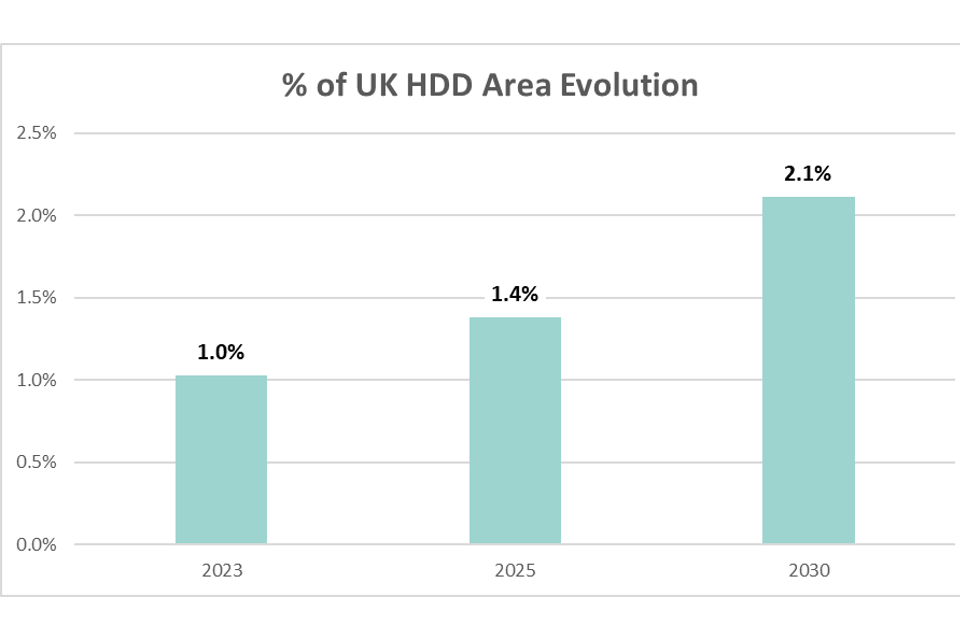
As the graph sets out, HDD areas represent about 1% of the UK landmass today (i.e., 2.496 km2 from a UK total of 243.610 km2). This includes approximately 3000 special locations (such as airports or sport venues). By the end of the decade, the area of the proportion of HDD areas in the UK with traffic density equivalent to today’s HDD areas is set to more than double, to 2.1%. It is important to note however that methods of identifying HDD areas vary - one mobile network operator, for example, suggests that a cluster-based approach to classifying base stations indicates that the above figures could be an under-estimate.
Taking into account the traffic volume estimates in the previous subsection, it is also possible to estimate the traffic density that could be expected in HDD areas, based on the low and medium growth scenarios set out by Ofcom, as follows.
Year | Average traffic density in HDD areas (TB/month/km2) | Demand density (Gbps/km2) |
---|---|---|
2023 | 77 - 83 | 0.36 - 0.38 |
2025 | 91 - 127 | 0.42 - 0.59 |
2030 | 173 - 431 | 0.8 - 2.0 |
Whilst the percentage increases may be small, due to high site density in HDD areas this can represent a significant increase in the number of sites.
2.5 Number of 5G sites in UK HDD areas
HDD areas generate intense traffic demands on the network, with networks needing to carefully plan and monitor sites to optimise the number of base stations and types of base station considering differing size and capacity.
The below figure forecasts the number of 5G sites in a dense urban scenario for the years 2023, 2025 and 2030 based on the area calculation in the previous section and assumptions concerning:
- Adoption of outdoor small cells and mmWave (Ofcom, “Mobile networks and spectrum” (2022));
- Average cell radius considered for the mid-band macro sites, outdoor small cells and mmWave sites.
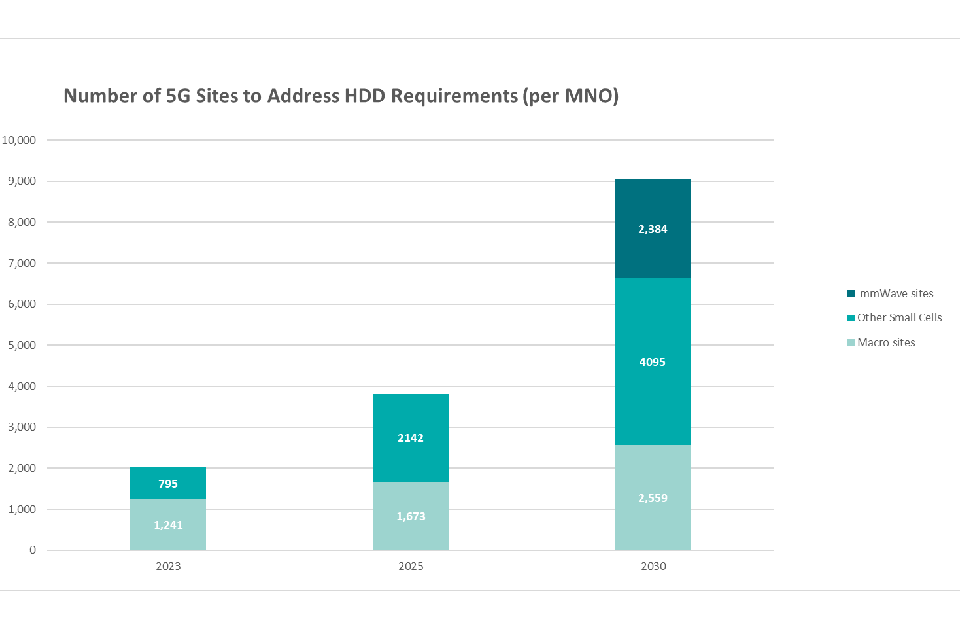
Based on the above assumptions, HDD traffic, and user profile, it is estimated that the following numbers of macro sites and small cells could be required per MNO to provide the HDD coverage footprint.
Year | Average number of HDD macro sites per MNO (estimated) | Average number of HDD small cells per MNO (estimated) |
---|---|---|
2023 | 1241 | 795 |
2030 | 2559 | 4095 (mid-band), 2384 (mmWave band) |
3. Key Technical Challenges for Open RAN in HDD areas
Some key technical challenges to OpenRAN adoption in HDD areas, drawn from stakeholder insight, are summarised below. This list should be seen as indicative and non-exhaustive.
Open Fronthaul
Open RAN deployments introduce the disaggregation of the Baseband Unit (BBU) into O-RU and O-DU connected by the Open Fronthaul (O-FH) interface. MNOs identified the following O-FH challenges for HDD areas:
- Open Fronthaul security and performance;
- Support for 7.2x split for macro layer;
- O-RU alignment on variations due to options and complexity - O-RU and O-DU need to reflect the same category option. Category A O-RUs are simpler with precoding done on the O-DU while category B are more complex with precoding in the O-RU, being recommended for HDD scenarios to reduce O-FH bandwidth and to put a lower processing load on DUs.
Chipsets
MNOs identified the following chipset challenges:
- Lack of diversity in processor suppliers - Intel dominates the O-RAN market as almost all O-RAN deployments to date have used COTS server hardware based on Intel’s FlexRAN architecture with or without hardware acceleration;
- Power efficiency of solution when using general purpose processors;
- Restriction of Open RAN component software products (i.e., DU, CU) to specific underlying hardware. Portability between chipsets and compute stacks would potentially increase DU/CU options for operators and foster competition.
Massive MIMO (mMIMO)
- Massive MIMO refers to antennas with a high number of antenna elements. Together with beamforming, this technology can address performance bottlenecks by managing interference and capacity to support a larger number of users in the call and improve end-user experience in densely populated areas.
- Open RAN systems may need to support mMIMO for good service quality in HDD areas.
- Performance parity with the traditional RANs for mMIMO products has been identified by MNOs as a current challenge.
Mobility
If not properly supported or implemented, mobility can significantly affect network performance. In terms of HDD scenarios there are three relevant mobility use-cases:
- Handover management between gNBs;
- Load-balancing through multiple layers as per MNO policies;
- Inter-layer mobility (between small cells and macro cells), which is highly relevant for HDD since it could offload traffic from the macro cell layer.
Energy Efficiency
- HDD areas are characterised as being highly demanding in terms of energy consumption, mainly due to the high user density and demanding services that the network needs to provide.
- The energy efficiency features to support HDD scenarios are mainly essential after peak traffic load situations, in which some of the active features can be disabled to save energy (eg, mMIMO features can be progressively turned off in a stadium after a match to save energy, and the BS Sleep Mode mechanisms can be turned on in train stations before and after rush hours)
Security
- While security is consistently a priority across all areas of the network, HDD environments are potentially higher priority due to the impact of security incidents being higher in areas of high network traffic.
- Vulnerabilities can expose a large number of users and a large volume of data.
- The disaggregated architecture arguably provides a larger attack surface for security measures to defend.
Interoperability
- Performance: The performance of Open RAN systems with components from a variety of vendors under HDD traffic load is not yet widely believed to have reached parity with traditional RAN vendor provider solutions.
- Network Management: In HDD areas consistent data compatibility and support processes for heterogeneous equipment across macro layer and small cell solutions will be needed.
RAN Intelligent Controller (RIC)
- Development of the RIC, SMO and associated use cases are seen as critical by MNOs to reduce the OPEX associated with network operations. MNOs see this as a differentiator for Open RAN over traditional RAN.
- Use cases to reduce the OPEX through automation of complex features and through automation of RAN operations are seen to be key.
- Further, in HDD areas use cases such as mMIMO optimisation and Traffic Steering can enhance how network resources are utilised.
Use of O-Cloud
O-cloud is the cloud platform needed to host the RAN functions. MNOs see uncertainties in this area as relating to :
- the acceleration architecture that will be required;
- orchestration of the cloud platform over potentially hundreds of sites (a scale beyond what is currently deployed for network purposes) and
- optimisation of cloud performance that does not place an undue burden on infrastructure (e.g. more server capacity per site may be needed if the cloud is not optimised). While several candidates are emerging in the market, there is yet to be a standard performance benchmark.
4. Open RAN Network Elements in HDD: indicative performance expectations
This section presents a high-level indication of the levels of specific performance expectations that may be held by mobile network operators for HDD Open RAN areas, over and above those for other parts of the network. Energy efficiency and security requirements are included since their impact is more pronounced in HDD scenarios, though they are relevant to all areas. These expectations are divided into short-term (1-2 year), mid-term (2-3 year) and longer-term (4-7 year) scenarios.
4.1 RAN Architecture
The various deployment models for Open RAN solutions balance multiple factors such as fibre availability, compute requirements, pooling gains, energy consumption, and latency. Multiple deployment models must be supported for HDD areas due to these variables.
For example, while it is beneficial to keep the O-RU at site level, other functions can be placed in a more centralised location (O-DU and/or O-CU), as long as it would not negatively impact the services. Centralising network elements at edge or regional level can enable other benefits beyond pooling capacity such as air conditioning energy consumption savings.
Single MNO
Requirement | Description | Relevance | Target Date |
---|---|---|---|
Single MNO | RU on site + vDU / vCU | Top | Short term |
Single MNO | RU + vDU on site + vCU centralised | Top | Short term |
Single MNO | RU on site + vDU / vCU centralised | Top | Short term |
Small Cell (single MNO)
Requirement | Description | Relevance | Target Date |
---|---|---|---|
Single MNO | RU + vDU / vCU Edge Cloud | High | Mid-term |
Single MNO | RU + vDU on site + vCU Edge/Regional Cloud | High | Mid-term |
Single MNO | RU+PHY on site + vDU / vCU Edge/Regional Cloud (Split 6) | Medium | Mid-term |
4.2 RAN Software Features
RAN software features refer to the key capabilities for a functioning mobile network and for the optimal utilisation of available infrastructure and resources. This section covers mobility, throughput, capacity, self-organising networks (SON), and energy efficiency.
Mobility
Radio network mobility expectations include radio link switching of a mobile user, inter and intra site handover management based on user location and criteria for managing data traffic demand.
Requirement | Description | Relevance | Target Date |
---|---|---|---|
Inter DU Mobility | Inter DU mobility / Inter DU mobility for EN-DC | Top | Short-term |
Handover | Intra / Inter | Top | Short-term |
Carrier Aggregation | Minimum 3 CCs (incl. 4x4), inter/intra site | Top | Short-term |
Carrier Aggregation | Intra gNB Inter DU CA | High | Mid-term |
Throughput
To achieve required throughput for users, mMIMO 32T32R and 64T64R are “top” expectations. To enable a more cost-effective deployment, MNOs may also pair 4T4R MIMO 5G O-RU with older antennas on sites that allow 5G set up using 4G frequencies as this does not involve replacing all hardware.
MIMO
Description | Relevance | Target Date | Obvs | Performance |
---|---|---|---|---|
4T4R | Top | Short-term | Low band (e.g. 700MHz) | ~200Mbps DL max throughput, 5 bps/Hz, BW 10MHz, 700MHz |
4T4R | High | Mid-term | Mid band (1.5GHz or 2.3GHz) | ~450Mbps DL max throughput, 5.6bps/Hz, BW 20MHz, 1.5GHz |
DL Multi-user | Top | Short-term | N.A. | N.A. |
UL Multi-user | Top | Short-term | N.A. | N.A. |
While the best performance could come from mMIMO RUs and antennas, there are significant constraints and MNOs may choose to maintain older antennas for 700MHz, 800MHz, 900MHz, 1.8GHz, 2.1GHz and 2.6GHz. As a consequence, only 2T2R (or 4T4R depending on the installed antenna) would be used for low bands and 4T4R used for mid-bands.
Massive MIMO
Description | Relevance | Target Date | Obvs | Performance |
---|---|---|---|---|
32T32R | Top | Short-term | Macro layer 3.4-3.8GHz, 1.5GHz, 2.3GHz, and 700MHz* | Proposed for busier rural areas, sub-urban or urban: ~4.7Gbps DL max throughput, 5.8bps/Hz, BW 100MHz, 3.5GHz, 8DL layers |
32T32R | High | Mid-term | Macro layer 2.6GHz | as above |
64T64R | Top | Short-term | Macro layer 3.4-3.8GHz, 1.5GHz, 2.3GHz, and 700MHz* | Proposed for dense urban areas and HDD scenarios: ~4.7Gbps DL max throughput, 5.8bps/Hz, BW 100MHz, 3.5GHz, 8DL layers |
64T64R | High | Mid-term | Macro layer 2.6GHz | as above |
*2021 auctioned by Ofcom
Although the expectation for 32T32R is shown, 64T64R could be the preferred option due to its long-term viability for urban areas rather than just for dense urban and HDD.
In HDD areas, higher modulation schemes are considered important to enhance throughput by up to 20%.
Modulation
Description | Relevance | Target Date | Obvs | Performance |
---|---|---|---|---|
1024QAM downlink modulation scheme | Top | Short-term | It corresponds to features usually released as licensed | Up to 20% gain from using 1024QAM instead of 256QAM modulation scheme |
256QAM uplink modulation scheme | Top | Short-term | As above | Up to 20% gain from using 256QAM instead of 64QAM modulation scheme. |
Capacity
The need to manage cell capacity, and ensure efficient utilisation of asset beamforming capability, is seen as fundamental by network operators. The use of specific and targeted beams to devices for managing Signal to Interference and Noise Ratio (SINR) is also seen as leading to improved user experience.
Requirement | Description | Relevance | Target Date |
---|---|---|---|
Beamforming | BeamSet Format (Radiating elements with the same signal at the same wavelength and phase, combine to form a single antenna, increasing the data rate) | High | Short-term |
Quality of Service
Traffic prioritisation mechanisms supporting QoS Class Identifier (QCI) and 5G QoS Identifier (5QI) designed to meet Guaranteed Bit Rate (GBR) (e.g., voice traffic) and non-GBR (e.g., video streaming) traffic requirements are considered important across RAN areas. For HDD scenarios, network slicing is expected to be a relevant feature to guarantee contracted SLAs for defined services under network congestion and high load scenarios.
Requirement | Description | Relevance | Target Date |
---|---|---|---|
Network Slicing | Slicing (5QIs/QCIs and types of services/slices configuration) | Top | Short-term |
Self-Organising Networks (SON)
SON features in RAN functions are relevant to HDD scenarios for automating processes across planning, configuration management, optimisation and healing on mobile radio access networks. Automated mechanisms for neighbour management and managing Physical Cell identity are expected to be considered especially important in HDD areas due to the level of change that is likely to occur amongst a large concentration of cells, and the need to eliminate human errors in configuration that could impact the network performance. The development of these features may also align with MNO strategies to reduce OPEX.
Requirement | Description | Relevance | Target Date |
---|---|---|---|
ANR | Automatic definition of neighbour relations for Intra Frequency, Inter Frequency, and Inter RAT | High | Mid-term |
Energy Efficiency
Energy consumption is estimated to represent between 20% to 40% of network OPEX and is highest when traffic load is at its highest. Within this, the RAN is estimated to consume around 80% of the total network energy of which air cooling systems consume 30-66% of base station site energy, so energy-efficient radio network products can make a considerable difference.
The operator expectations set out below could be achieved through strategies such as partially turning off the mMIMO array, or even disconnecting some cells based on utilisation or forecasted utilisation by AI/ML - this could reduce the RAN power consumption when traffic load is low to around 30% of its typical consumption. On the hardware side, improving the power amplifier (PA) energy consumption (estimated to be 55% of the total mMIMO RU energy consumption at 100% load) could also yield savings.
Requirement | Description | Relevance | Target Date | Performance |
---|---|---|---|---|
ON/OFF DL MIMO adaptation | Automatic deactivation of transmit branches and elements to reconfigure cell to simpler MIMO configurations during low traffic | High | Mid-term | Up to 20% savings when in lower loads by disabling a portion of the mMIMO array |
Enhanced Radio Deep Sleep | RRUs can be automatically switched off to enable energy savings, configured and controlled by RIC and SMO, working with mMIMO and FDD/TDD | High | Mid-term | No energy savings information was found concerning this feature |
mMIMO DFE shutdown | Part of digital front end and beamforming can be automatically shut down based on traffic load, without impacting on the mMIMO antenna components or reliability | High | Mid-term | as above |
Base station Sleep mode mechanisms | SW support for automatic deactivation of number of PAs in mMIMO, based on traffic, and LTE carriers in low traffic periods | High | Mid-term | as above |
Battery Consumption Saving | Additional enhancements are required to address issues in Rel-16, (idle/inactive-mode power consumption in NR SA deployments) | High | Mid-term | as above |
Battery Consumption Saving | Building blocks to enable small data transmission in INACTIVE state for NR | High | Mid-term | as above |
Others
This encompasses miscellaneous RAN features that are not part of the previous subsections but are nonetheless important for Open RAN solutions in high traffic density scenarios.
Requirement | Description | Relevance | Target Date |
---|---|---|---|
Counters | RAN Software features counters | Top | Short-term |
Counters | O-RU power consumption metrics | High | Mid-term |
Positioning | Support of feature around user positioning: RAN positioning based on Cell ID & timing advance, E-CID positioning | High | Mid-term |
RAN Sharing | eNB/gNB supporting MOCN and MORAN configuration | High | Mid-term |
As networks evolve with increasing levels of automated operations, counters and data on features and performance are seen as critical (e.g., to optimise network performance, pre-empt congestion and detect congested areas). Positioning capability is also viewed as highly relevant as well as the potential of Open RAN solutions in network sharing deployments to address TCO challenges by sharing infrastructure.
4.3 RU
The following analysis sets out indicative operator expectations for current Open RAN RU performance in HDD areas.
Spectrum Allocation
The following tables outline six specific scenarios:
- RU#1 – High Band TDD (Small Cell)
- RU#2 – Wide Band / High Band TDD / mMIMO (WB-HBT-MM-04)
- RU#3 – Wide Band/ High Band TDD/ mMIMO (WB-HBT-MM-02)
- RU#4 – mmWave TDD/mMIMO (mmW-MM-01)
- RU#5 – Single Band / Mid Band TDD / RRU (SB-MBT-RU-02)
- RU#6 – mmWave TDD/m
Description | RU#1 | RU#2 | RU#3 | RU#4 | RU#5 | RU#6 |
---|---|---|---|---|---|---|
Frequency Requirements (MHz) | 3400 - 3800 | 3400 - 3800 | 3400 - 3800 | 700+800+900 | 2600 | 26000 |
IBW (MHz) | 200 | 400 | 400 | 95MHz | 50 | 1000 |
OccBW (MHz) | 200 | 200 (min) / 300 (targeted) | 300 (min) / 400 (targeted) | 95MHz | 50 | >=800 |
Antenna Design
Description | RU#1 | RU#2 | RU#3 | RU#4 | RU#5 | RU#6 |
---|---|---|---|---|---|---|
AAU Number of simultaneous TX user beams/layers per carrier | N.A. | 16DL/8UL | 16DL/8UL | N.A. | N.A. | N.A. |
Beam-steering capability | N.A. | Digital Beamforming | Digital Beamforming | N.A. | N.A. | N.A. |
AAU Antenna Elements | N.A. | 192 | 384 | N.A. | N.A. | N.A. |
Tx/Rx | 4T4R | 64T/64R | 64T/64R | 2T4R | 4T4R | 4T/4R |
Radiated Signal
Description | RU#1 | RU#2 | RU#3 | RU#4 | RU#5 | RU#6 |
---|---|---|---|---|---|---|
EIRP | N.A. | 76dBm | 79dBm | N.A. | N.A. | N.A. |
RF Power (W) | 4*5 | 320 (minimum) | 320 (minimum) | 2*160 | 4*80 | 4*5 |
Modulation
Description | RU#1 | RU#2 | RU#3 | RU#4 | RU#5 | RU#6 |
---|---|---|---|---|---|---|
Downlink modulation Mode | 256QAM, 1024QAM | 256QAM, 1024QAM | 256QAM, 1024QAM | 256QAM, 1024QAM | 256QAM, 1024QAM | 256QAM, 1024QAM |
Uplink modulation Mode | 64QAM, 256QAM | 64QAM, 256QAM | 64QAM, 256QAM | 64QAM, 256QAM | 64QAM, 256QAM | 256QAM |
Energy Efficiency
Description | RU#1 | RU#2 | RU#3 | RU#4 | RU#5 | RU#6 |
---|---|---|---|---|---|---|
Power Consumption @100% Load | 0.12kVA | <0.9kVA | <0.9kVA | 0.48kVA | 0.48kVA | 0.12kVA |
Power Consumption @0% load | <30% of power consumption @100% load | <30% of power consumption @100% load | <30% of power consumption @100% load | <30% of power consumption @100% load | <30% of power consumption @100% load | <30% of power consumption @100% load |
4.4 Open Fronthaul
This section refers to Open Fronthaul (O-FH) expectations, encompassing:
- Supported profiles, referring to supported beamforming methods and compression;
- Management and synchronisation plane requirements;
- Physical interfaces;
- Fronthaul Gateway, which can support L1 processing offload;
- And supported split option 7.2x.
Category | Requirement | Description | Relevance | Target Date |
---|---|---|---|---|
O-FH profile | NR-TDD-FR1-CAT-B-mMIMO-RTWeights-BFP | NR TDD FR1 with Massive MIMO, config Cat B, with weight-based beamforming and Block Floating Point compression | Top | Short-term |
O-FH profile | NR-TDD-FR1-CAT-B-mMIMO-RTWeights-ModComp | NR TDD FR1 with Massive MIMO, config Cat B, with weight-based beamforming and modulation compression | Top | Short-term |
A fronthaul gateway can support the precoding function, compression/decompression, provide synchronisation of DU and RU, L1 low PHY, Digital Front End (DFE), and beamforming processing offload with dedicated cards. Processing offload is viewed as highly relevant to HDD scenarios to reduce CPU demand on the server.
Category | Requirement | Description | Relevance | Target Date |
---|---|---|---|---|
Fronthaul Gateway | Support of O-RAN compliant 7.2x interface with O-DU | FHGW for connectivity of legacy O-RUs. Also relevant for HDD since it allows L1 processing offload with dedicated acceleration cards | Top | Short-term |
Beamforming | Support of Predefined-Beam Beamforming | n/a | Top | Short-term |
Beamforming | Support of Weight-based Dynamic Beamforming | n/a | High | Mid-term |
Supported Splits | Split option 7.2x | Support functional splits option 7.2x. Being targeted at HDD scenarios, it should support category B O-RUs for mMIMO and beamforming capabilities | Top | Short-term |
The SyncE method of synchronisation is considered beneficial in HDD scenarios since GPS/GNSS synchronisation is not always possible in deep urban areas due to surrounding buildings.
Category | Requirement | Description | Relevance | Target Date |
---|---|---|---|---|
Synchronisation | SyncE (FH&BH) | Synchronous Ethernet ITU-T Rec. G.8261 | Top | Short-term |
4.5 O-Cloud
Expectations for O-Cloud relate to the virtualisation of DU and CU network functions over cloud infrastructure. They include the following specific considerations.
In HDD scenarios, L1 acceleration cards are considered fundamental to offload L1 processing from the CPU, enabling a more robust infrastructure to address high loads.
O-CLOUD INFRA
Requirement | Description | Relevance | Target Date |
---|---|---|---|
Drivers | Accelerator Driver – eASIC / Network Driver | High | Mid-term |
eASIC | Accelerator Driver – eASI, Accelerator Driver – GPU Operator, Hardware Accelerator Abstraction Layer (AAL) | High | Mid-term |
GPU operator | Accelerator Driver – eASI, Accelerator Driver – GPU Operator, Hardware Accelerator Abstraction Layer (AAL) | High | Mid-term |
AAL | Accelerator Driver – eASI, Accelerator Driver – GPU Operator, Hardware Accelerator Abstraction Layer (AAL), Look-aside acceleration mode / Inline acceleration model2025 | High | Mid-term |
Management | HW Acceleration Management | High | Mid-term |
O-Cloud Infra / Platform | Scale-In / Scale-Out | High | Mid-term |
The O-Cloud infrastructure is expected to be fully integrated with the SMO through the O2 interface to leverage automation and scalability for HDD scenarios. For instance, at off-peak loads the infrastructure can be scaled-down (scale-up processes must be considered as well) for energy consumption savings.
Requirement | Description | Relevance | Target Date |
---|---|---|---|
Integration with SMO via O2 interface | O-Cloud Monitoring Service / O-Cloud Provisioning Service / O-Cloud Software Management / O-Cloud Fault Management / O-Cloud HTTP support / O-Cloud Infrastructure Lifecycle Management / O-Cloud Deployment Lifecycle Management | High | Mid-term |
Management Interfaces | Scalability, O2 interface support / O-Cloud Infrastructure Lifecycle Management / O-Cloud Deployment Lifecycle Management | High | Mid-term |
In HDD scenarios, mMIMO solutions are recommended. These are power intensive, and therefore energy efficiency features become imperative. For instance, these features can dynamically decrease the size of the mMIMO array or turn off a specified number of antenna elements. It therefore is crucial to understand the benefit realisation of these features and to monitor network energy consumption.
Requirement | Description | Relevance | Target Date |
---|---|---|---|
Management Energy Efficiency KPI & Monitoring | Cost & Energy / Green management / Energy consumption KPIs at hardware level / Energy consumption KPIs at workload level / Energy consumption KPIs at hardware level / Electrical Power / Power Saving Features | High | Mid-term |
Specific services may require tight SLAs and may be deployed over a network in an HDD scenario. To ensure that those SLAs are satisfied, network slicing is considered a priority for HDD scenarios.
Requirement | Description | Relevance | Target Date |
---|---|---|---|
RAN Sharing / Slicing | RAN Sharing / Slicing | High | Mid-term |
Due to the high number of users and traffic in HDD areas, security plays a fundamental role, and so related features are considered high priority.
Requirement | Description | Relevance | Target Date |
---|---|---|---|
Security | O-Cloud Zero Trust Architecture (ZTA) | Top | Short-term |
Security | Container host OS security | Top | Short-term |
Security | Secure and trusted code/security testing | Top | Short-term |
Security | Image Security | Top | Short-term |
Security | Security Deployment | Top | Short-term |
Security | Secure Storage of Data | Top | Short-term |
Security | Secure Communications between services and functions | Top | Short-term |
Security | Secure Configuration of the Cloud Infra Assets | Top | Short-term |
Security | Secure Administration | Top | Short-term |
Security | Trusted Registry | Top | Short-term |
Security | Interface Security | Top | Short-term |
Security | Artifact build & management | Top | Short-term |
Security | Secure SMO and O-Cloud Communication | Top | Short-term |
Security | Security handshake between SMO and O-Cloud | Top | Short-term |
Security | Hardening | Top | Short-term |
Security | Local Identification and authentication | Top | Short-term |
Security | Remote Identification and authentication | Top | Short-term |
Security | Passwords | Top | Short-term |
Security | RBAC (Role Based Access Control) | Top | Short-term |
Security | IP Security | Top | Short-term |
Security | Container Register Security | Top | Short-term |
Security | Centralised Authentication System (CAS) | Top | Short-term |
4.6 DU/CU
The following set of expectations relate to the virtualisation of DU/CU over a bare metal server, and covers several specifications, namely:
- Inputs and outputs (e.g., PCIe slots, highly relevant for L1 processing offload which has relevant benefits for HDD);
- Synchronisation through chaining, which can be beneficial in high cell density areas;
- Network function scalability;
- Energy efficiency and monitoring.
Requirement | Description | Relevance | Target Date |
---|---|---|---|
Motherboard | General Purpose Processor for: Full processing in the CPU, FEC/LDPC offload in look-Aside Architecture / L2 processing in CPU, Full L1 offload in-line architecture | Top | Short-term |
External ports | PCIe slots to install acceleration cards | Top | Short-term |
Security | IPSec – Midhaul / IPSec – Backhaul | Top | Short-term |
Synchronisation | Sync chaining | High | Mid-term |
Connectivity | Multiple O-DUs (O-CUs shall support connectivity to multiple O-DUs) | High | Mid-term |
Scalability | Scale out / Scale in support to enable dynamic dimensioning of NFs | Top | Short-term |
Energy Efficiency | Energy efficiency counters/KPI (CNF) | High | Mid-term |
Power Consumption | Power metering | High | Mid-term |
The following table presents expectations relevant to O-CU. Inter-CU mobility is highly relevant to HDD as the cell radius in these areas are small, and cell handovers are therefore more likely to span different CUs.
Requirement | Description | Relevance | Target Date |
---|---|---|---|
Feature | Inter CU Mobility | High | Mid-term |
At the vDU level the following points are seen as highly relevant for HDD:
- Offloading L1 processing, to improve performance and energy efficiency;
- As the cell radius is small in HDD areas, cell handovers are more likely to span different DUs;
- HDD areas are often dense urban which can restrict GPS/GNSS synchronisation due to weak signal reception. SyncE/G.8262 is critical as this protocol circumvents this limitation.
Requirement | Description | Relevance | Target Date |
---|---|---|---|
Hardware Acceleration | Hardware Acceleration card | Top | Short-term |
Hardware Acceleration | Hardware Acceleration architecture | Top | Short-term |
Features | Inter O-DU mobility | High | Short-term |
Features | Inter O-DU mobility for EN-DC | Top | Short-term |
Features | Intra gNB inter O-DU CA | High | Mid-term |
Synchronisation | SyncE /G.8262 | Top | Short-term |
Synchronisation | Holdover mechanisms | High | Mid-term |
4.7 RIC and SMO
RIC and SMO are seen as major differentiators for Open RAN compared to traditional RAN, as they can enable unprecedented levels of automation in the MNO network. In HDD areas, automation is seen as enhancing network performance, security, and efficiency even further, unlocking new methods for load management, security processes automation and security control maintenance among others.
Requirement | Description | Relevance | Target Date |
---|---|---|---|
Open Interfaces | E2, A1, O1, O2 | Top: E2, A1, O2 ; High: O1 | Short-term |
Scalability | Support E2 connections with a single instantiation aligned with the scalability of E2 endpoint nodes | High | Mid-term |
Performance | Allow configuration and enforcement of latency targets as required by the xAPP |
The top priority RIC use cases in the short-term are:
- QoE Optimisation
- QoS Based Resource Optimisation
- Massive MIMO Optimisation
- Energy Efficiency
- RRC enhancements
Non-RT RIC
Non-RT RIC has a special focus on the implementation of rApps and their training through AI/ML algorithms.
Requirement | Description | Relevance | Target Date |
---|---|---|---|
Open APIs | R1 interface | Top | Short-term |
rApps | rApp implementation / rApp security | Top | Short-term |
Open Interface Testing | A1 Testing | High | Mid-term |
Near-RT RIC
Requirement | Description | Relevance | Target Date |
---|---|---|---|
Open APIs | Open APIs approaches, E2 related API, A1 related API, Management API, SDL API, Enablement API | Top | Short-term |
Interface Functions | E2 Security | Top | Short-term |
Platform Functions | xApp Subscription Management, Shared Data Layer, xApp Repository Function | High | Mid-term |
xApps | xApp implementation / Life-Cycle Management of xApp / xAPPs Security/ xApps Authentication | Top | Short-term |
Open Interface Testing | E2 Testing | High | Mid-term |
Top-priority SMO enablers for HDD
Requirement | Description | Relevance | Target Date |
---|---|---|---|
SMO slicing requirements | NFMF: NF automatic config. functions and Service Based exposure | High | Short-term |
SMO slicing requirements | Creation of O-RAN network slice subnet instances and define slice priority order | High | Short-term |
SMO slicing requirements | RAN slice data model (RAN-NSSMF), RAN slice subnet (RAN-NSSMF) - QoS, RAN slice data model (RAN-NSSMF) | High | Short-term |
SMO design | Energy efficiency / Auto healing of SMO | Top | Short-term |
Fault Management | Automatic Fault Detection, Resolution Recommendations, Integrated Analytics Engine, Reporting system | Top | Short-term |
Performance Management / analytics | Performance monitoring, Data analysis, Thresholds definition, Energy efficiency etc | Top | Short-term |
Performance Management / analytics | Notifications and reports, Suggestion Tool | High | Mid-term |
O-Cloud | General O-Cloud Management support | Top | Short-term |
O-Cloud | O2 interface supports SMO in managing cloud infra and deployment operations with infra that hosts the O-RAN functions in the network (bare metal, public or private cloud). Also supports the orchestration of the infra where O-RAN functions are hosted, providing resource management (e.g., inventory, monitoring, provisioning) and deployment of the O-RAN NFs, providing logical services for managing the lifecycle of deployments that use cloud resources | Top | Short-term |
Automation | PaaS Automation, CNF Automation, PNF Automation, Hardware Acceleration Automation, etc | Top | Short-term |
Automation | Deployment Automation, Fault Management Automation | High | Mid-term |
E2E Orchestration | API exposure to E2E orchestration, Orchestration functions and capability | High | Mid-term |
5. Glossary
ANR – Automatic Neighbour Relation
CNF – Containerised Network Functions
CU – Centralised Unit
DL – Downlink
DU – Distributed Unit
HDD – High Demand Density
MIMO – Multiple Input Multiple Output
mMIMO – Massive Multiple Input Multiple Output
MNO – Mobile Network Operator
N.A. – Non Available
O-CU – Open Centralised Unit
O-DU – Open Distributed Unit
O-FH – Open Fronthaul
O-RU – Open Radio Unit
OSS – Operational Support Systems
PCI – Physical Cell Identity
QoS – Quality of Service
QCI – QoS Class Identifier
RAN – Radio Access Network
RIC – RAN Intelligent Controller
RU – Radio Unit
SA – Standalone
SON – Self-Organising Network
NSA – Non-Standalone
SMO – Service Management and Orchestration
UL – Uplink