Auditing algorithms: the existing landscape, role of regulators and future outlook
Updated 23 September 2022
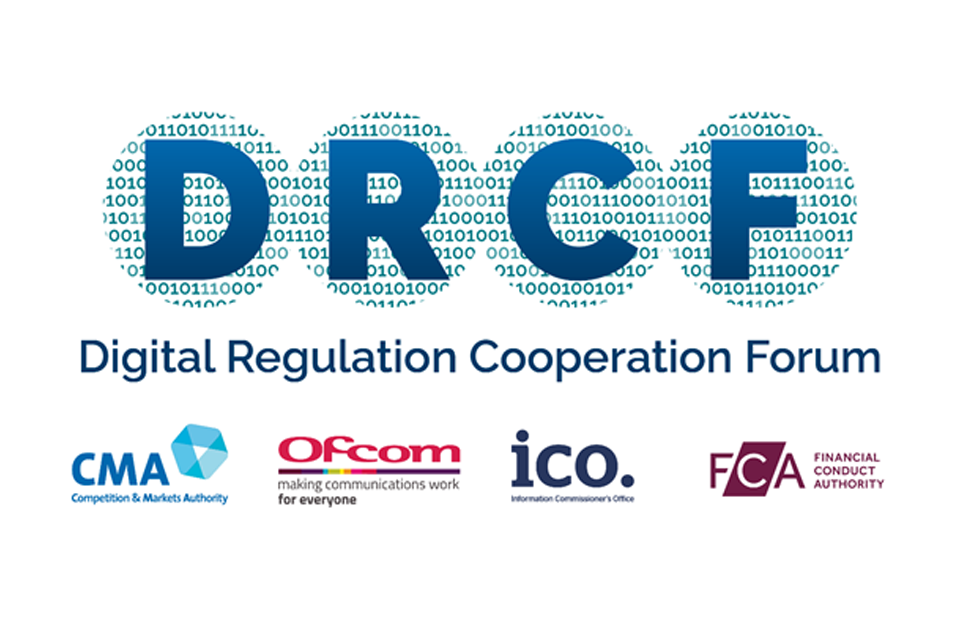
Note: This discussion paper is intended to foster debate and discussion among our stakeholders. It should not be taken as an indication of current or future policy by any of the member regulators of the DRCF.
Executive Summary
Complex algorithms are now widely used within digital products and online services. These algorithms deliver many benefits, such as personalised recommendations that save us time when deciding what film to watch or what food to order. However, their use without due care can lead to individual or societal harms, many of which are outlined in our accompanying publication, ‘The benefits and harms of algorithms: a shared perspective from the 4 digital regulators’. To ensure that the benefits are realised and risks are addressed, we need a way to assess what organisations are doing with algorithms and how algorithmic processing systems operate.
Algorithmic auditing refers to a range of approaches to review algorithmic processing systems. It can take different forms, from checking governance documentation, to testing an algorithm’s outputs, to inspecting its inner workings. Audits can be undertaken by external parties appointed by the organisation, or by regulators, researchers or other parties carrying out an audit of a system on their own initiative. Audits can be done for the purposes of internal assurance, as a route to signalling transparency and establishing trust with users or other parties that are affected, or they can be done to establish whether a system may comply with regulatory requirements. The style and depth of audits will vary depending on the nature and size of risks, the context in which algorithms are deployed and existing regulatory requirements. Algorithmic audit differs from traditional financial audit, which is already well established, professionalised and regulated along clearly defined parameters.
Many regulators [footnote 1], including some members [footnote 2] of the Digital Regulation Cooperation Forum (DRCF) [footnote 3], already have powers to audit algorithmic processing systems in investigations. Some DRCF members have audited such systems as part of formal cases, leading to enforcement action. Beyond the DRCF, other stakeholders with an interest in algorithmic auditing include governments, standards bodies, large technology companies, established and start-up consultancies, researchers in academia and industry, journalists and wider civil society actors. While algorithmic auditing is currently not conducted extensively and the market for audits is relatively immature, an audit ecosystem will likely grow in the coming years.
As regulators we have an important role in answering questions that will shape the landscape of algorithmic audits. What frameworks should underpin algorithmic audits? What standards should algorithmic systems be assessed against? What role should audit play in the context of demonstrating compliance? The DRCF has been considering these and other questions in discussions with stakeholders from industry, academia, civil society and government. This discussion paper summarises the key issues in the current audit landscape and explores the potential shape of the future ecosystem for algorithmic audit, with a specific focus on the role for regulators.
Our engagement revealed several issues in the current audit landscape. Other than in highly regulated sectors such as health and aviation, the algorithm audit landscape lacks specific rules and standards, and auditors can enter the market without any assurance of their quality. It is unclear which standards audits should follow, and an audit without commonly agreed standards cannot provide assurance, only advice. Further, in some sectors, there is inconsistency in what audits focus on. Often auditors are limited by a lack of access to systems, particularly academics and not-for-profit organisations. Our findings suggested this was due to organisations with algorithmic systems being reluctant to co-operate with audits, the risk of auditors being legally sanctioned for scraping information, or clients providing inconsistent documentation. It was also highlighted to us that following audits, there is sometimes insufficient action, particularly because the people affected have no means of receiving redress.
The future landscape will need to address these issues, and regulators can play an important role in developing and shaping solutions. This could include providing guidance on when audits are appropriate – for instance before a system goes live, at regular intervals or following the raising of concerns of harm. Regulators could establish principles for best practice, including what organisations need to disclose about their systems and to whom, and they could assist with the development of audit standards, exploring when they are well placed to set principles or standards themselves and when they may look to be a facilitator. There may be a role for accreditation, through which regulators can approve third-party auditors to certify that systems are being used in ways that are likely to meet certain regulatory standards. Regulators may also have a role in ensuring that auditors have the right to gain sufficient access to systems. Such steps may help assist the development of a third-party auditing market able to complement regulatory activity effectively. Further, to ensure audits have value in driving the improvement of systems, regulators can ensure that action is taken when an audit identifies harms. This could involve using their powers to take regulatory action, providing incentives for parties to come forward with information and making it easier for the public and other parties to report harms. In the event that any such interventions are undertaken, the benefits of introducing new auditing requirements will need to be proportionate and carefully balanced against the regulatory costs to firms.
Regulatory co-operation and collaborative action with industry could help facilitate these improvements, for instance by jointly developing standards and guidance for audits, accreditation for auditors, or providing mechanisms through which auditors, civil society bodies, academics and the public can securely share information with regulators to help create an evidence base for emerging harms. The DRCF has an opportunity to be at the forefront of these developments.
In the next financial year, we intend to undertake further activity to understand regulators’ roles in the field of algorithmic processing. We are now launching a call for input alongside the publication of these 2 papers to inform the future work of the DRCF, and we welcome and encourage all interested parties to engage with us in helping shape our agenda.
Introduction and purpose
Algorithmic processing systems
With 92% of the UK online,[footnote 4] the average citizen can expect to interact with companies’ algorithms across their personal and professional lives, many dozens of times a day. Algorithmic processing systems (algorithms) – broadly defined here as systems that process data in an automated manner [footnote 5] – are now integral to many digital products and online services. These systems, which range from simple statistical models to more advanced machine learning, are increasingly being used in the creation of output (for example, video content) and to make decisions that have a direct bearing on individuals. Algorithmic processing systems have numerous benefits. They can speed up and optimise decision-making that would otherwise be solely undertaken by humans. For example, algorithms can optimise travel routes to make journeys and deliveries quicker and more efficiently. They can provide personalised recommendations for films to watch, articles to read or food to order that are relevant and timesaving. They can be used to detect potentially harmful activity, such as screening for fraudulent transactions in financial services, or flagging potential discrimination in job hiring software.[footnote 6]
However, the proliferation of algorithmic processing systems can lead to individual or societal harms. For example, their use or misuse could result in discriminatory outcomes, compromise individuals’ privacy or their ability to make informed decisions, and could threaten the operational resilience or stability of certain markets. Regulators have been undertaking research to understand these issues better. In our accompanying publication [footnote 7] we outline many of the potential benefits and harms that we consider to be associated with the use of algorithms. The CMA published a report considering the effect of algorithms on outcomes for competition and consumers, [footnote 8] and the FCA and the Bank of England established a public-private forum on artificial intelligence (AI) to better understand the impact of AI on financial services.[footnote 9]
The UK government has also taken a keen interest, and has outlined its intention to develop a national position for governing and regulating AI through the publication of its National AI Strategy in 2021. It has also committed to publish an AI White Paper focusing on governance in 2022.[footnote 10]
Algorithmic audit
The use of algorithmic systems and their outcomes can be opaque, due to a lack of transparency of governance measures and the complex technical nature of some algorithmic systems. Because of this, it is important to develop mechanisms to check and verify that algorithmic systems are meeting regulatory expectations and not producing harms (either unintended and intended).[footnote 11]
Understanding how a system works, the basis on which it makes decisions or recommendations, how it has been developed, and whether it is suitable for the purpose intended is of interest to a number of parties, and different parties will have different assurance needs. Companies developing and deploying algorithmic systems will want to demonstrate that their systems work as claimed and that they do not result in or facilitate the harms described above. Their developers can use an audit as feedback to support continuous monitoring and improvement of their system, while managers could use audit to assess the impact of a system and its suitability for purpose. Regulators will want to verify legal compliance, and end users may wish to know details behind the decisions made about them and the effects on their decision-making. Some other parties who are not directly affected by the system, such as academics or journalists, may also have an interest in scrutinising the system for public interest or research purposes.
It may therefore be helpful for organisations and regulators to have common approaches and techniques to understand how algorithmic systems function. Various terminology exists to describe approaches to the assessment of algorithmic processing systems, such as assurance [footnote 12] and audit. In this discussion paper, ‘audit’ will be used as an umbrella term to describe the assessment of an algorithmic system. This encompasses technical and non-technical measures that range from assessing organisational algorithmic governance policies to the specific data and models being used.
Audit can be undertaken by an internal assurance team in the organisation using the algorithmic system, by external parties such as independent audit providers, or by a regulator or other supervisory authority.
Audit and the regulatory context
DRCF members are increasingly having to assess whether algorithmic systems lead to unlawful outcomes. This may involve ex-post activities, such as investigations or enforcement, and ex-ante activities, such as the use of pre-launch testing environments like sandboxes.
Regulators therefore need to understand how to reliably and consistently assess the impact of algorithmic systems. However, regulators are not just concerned about the system itself. The broader context in which the system is used matters. An organisation may use an algorithmic system as part of a decision-making process which directly affects the circumstances of an individual or society more broadly. An algorithm might determine whether a person can get a mortgage, or a facial recognition technology that screens customers in a supermarket may assess whether a customer is old enough to buy alcohol. The system will be part of a wider ‘decision architecture’ potentially involving human review before a final decision is made. Regulators are concerned with the overall process by which the decision is made – for instance whether the consumer has been treated in a fair and non-discriminatory manner, been provided with transparency information they can understand, and whether appropriate safeguards have been put in place to protect their privacy. The regulator is therefore interested not only in the way a specific algorithm works and how a system produces its decision, but how the system fits within the overall decision architecture, the wider checks and balances provided by the organisation deploying the technology, and the role of human oversight.
The type of audit of interest to a regulator may vary depending on its remit and regulatory approach. A sectoral regulator with supervisory oversight such as the FCA may focus primarily on ensuring the appropriate governance and accountability mechanisms surrounding the outcomes of an algorithmic processing system within, in the FCA’s case, financial services. A cross-sectoral regulator such as the ICO may focus on both the process by which personal data is processed using algorithmic systems, and the outcomes of those systems. Where a regulator undertakes investigation and enforcement activity, inspection of an algorithmic system may form part of the investigatory process. A number of recent investigations have involved algorithmic audit:
- The CMA has been investigating Amazon and Google over concerns they have not done enough to tackle fake and misleading reviews on their sites [footnote 13], including by examining their review moderation and product rating systems.
- In 2021, the ICO and its Australian counterpart investigated Clearview AI Inc’s facial recognition technology due to suspected breaches of UK and Australian data protection laws.[footnote 14]
- The ICO investigated the use of data analytics and personalised microtargeting in political campaigns in 2017.[footnote 15]
- The Australian Competition and Consumer Commission inspected Trivago’s algorithms, revealing that the ranking of hotels was weighted towards those hotels that paid higher commissions to Trivago, rather than those providing the cheapest rates available to consumers.[footnote 16]
Several private and third sector stakeholders are also interested in auditing algorithmic systems, including large technology firms, private sector audit providers, academics, investigative journalists and advocacy groups. Audits carried out by these bodies can provide a degree of assurance that systems are trustworthy and can also uncover potential harmful outcomes from the use of algorithms.
-
Technology companies have begun to develop technical algorithmic auditing tools [footnote 17], including Facebook’s Fairness Flow [footnote 18],IBM’s AI 360 Toolkit [footnote 19], and Google’s Model Cards for Model Reporting [footnote 20] or Fairness Indicators in Tensor Flow [footnote 21].
-
In 2021, the Wall Street Journal investigated TikTok by creating 100 bots to watch videos to understand what TikTok’s algorithms would learn, and therefore what they would recommend.[footnote 22]
-
Advocacy groups such as the Algorithmic Justice League in the US have undertaken audits of facial recognition tools to detect signs of racial and gender biases.[footnote 23]
-
Academic audits have reviewed the impact of algorithms such as the Ribeiro et al (2020) study on the outcomes of recommender systems on YouTube to accessing extreme content that can lead to radicalisation.[footnote 24]
The development of this algorithmic audit ecosystem raises a number of important questions:
-
What constitutes an appropriate framework for carrying out system audits?
-
Against what standards should systems be reviewed and how should standards vary depending on the regulatory environment?
-
What role should audit play in the context of demonstrating compliance with regulatory requirements?
-
How transparent should organisations be and to whom (for example, consumers and others impacted by algorithmic systems)?
-
Which type of audit is appropriate in different sectors and contexts?
-
Who should be responsible for developing algorithmic audit standards?
-
Under what circumstances should algorithmic audits be mandatory?
Regulators have a central role to play in addressing each of these questions. However, the answers may differ to some degree across regulators and different sectors, meaning that organisations deploying algorithmic systems may be required to comply with requirements from multiple regulators. It will be important for any associated regulatory environment to be clear and predictable in order to encourage innovation and market development. The approaches to audit taken by regulators will need to complement each other and provide sufficient clarity around the expectations of organisations.[footnote 25]
Audit and the role of the DRCF
The DRCF provides a forum through which 4 regulators – the CMA, FCA, ICO and Ofcom – can co-operate to develop answers to these questions and consider the role for regulators to play in the audit ecosystem. In recent months the DRCF has explored how algorithmic processing systems are currently audited and is considering how the audit landscape may evolve. This has included a round of engagement with parties involved in the algorithmic audit ecosystem, including industry and academia.
The purpose of this paper is to summarise the key issues from our discussions and initial stakeholder engagement to date, and to establish an initial DRCF position on the algorithmic auditing landscape. We set out a number of initial hypotheses that we will test through a call for input, and invite feedback from stakeholders interested in this area. This will feed into the work conducted in our next workplan, where we will undertake further research into the algorithmic audit ecosystem.
The remainder of this paper is structured as follows:
-
Section 2 sets out some core background to understand the key issues associated with algorithmic audit and in particular the role regulators could play.
-
Section 3 discusses the different types of audit and the potential outcomes from an audit.
-
Section 4 considers the existing landscape for audit, covering the parties involved in the audit ecosystem and the limitations and issues identified with the current landscape.
-
Section 5 discusses the potential shape of a future landscape for algorithmic audit, including the potential role of a market for third party audits.
-
Section 6 presents some hypotheses the DRCF has developed in the course of researching this discussion paper, and invites stakeholder feedback.
The role for audit in algorithmic governance
Audit forms a crucial element of the algorithmic governance landscape. As algorithmic systems can be complex and require specialised knowledge to understand, there are asymmetries of information between the parties involved.[footnote 26] Developers of a system are likely to have more information about the performance and workings of a system than the users of a system. This occurs both internally within an organisation where there may be different teams developing a system from those deploying or managing it, as well as between 2 parties in the context of a system user procuring a system developed by a third party. Organisations deploying a system usually have more information than their end users such as consumers or subscribers, or other parties that may not directly use the system but could ultimately be affected by it. The organisations deploying a system also usually have more information than regulators responsible for ensuring the use of the system is compliant with the law. Asymmetries of information between organisations and regulators are common in many situations. When algorithmic systems are used, the asymmetry can be substantially exacerbated because of the inherent complexity of the systems.[footnote 27]
Algorithmic audit seeks to help solve this informational asymmetry by ensuring that a system is reviewed by a party with suitable specialist knowledge who can convey information about the impact of the system to other parties. Audit is not necessarily the only solution to an information asymmetry problem. To close the information gap, explicit mandatory transparency requirements could help, or the use of a pre-authorisation system review to highly specified standards to ensure only systems with certain characteristics can enter a market.
Audit for transparency and trust in algorithmic systems
Given the information asymmetry currently associated with algorithmic processing, auditing may be important for building trust among different groups of stakeholders. It may have a part to play in internal assurance to give senior management confidence that a system designed in-house or procured externally follows the organisation’s processes and delivers what it is intended to do. It could also provide external ‘signalling’ of system quality to an organisation’s end users, particularly when the system is assessed and given a level of assurance of quality by a third party that is credible to the users. For example, a supermarket chain may use facial recognition technology to assess the age of a customer when purchasing age-restricted products. Supermarket management would require assurance that the system was accurate in identifying customers that required an age check and did not make discriminatory decisions that could lead the supermarket to be in breach of the Equality Act 2010.[footnote 28] The supermarket customers may be sceptical or uncomfortable with this new technology and require assurance that its use would not lead to them being treated unfairly.
Effective algorithmic auditing provides different stakeholder groups with a better understanding of the nature and impact of algorithmic systems. This could be a first-hand understanding through undertaking algorithmic audits of a system or an organisation’s governance measures, or from the assurance of a third-party auditor verifying that a system functions as intended. Where users are able to exercise choice between products that involve algorithmic systems, this allows market forces to exert pressure on organisations deploying them to maintain or improve the quality and usefulness of their systems for their users.
Audit for compliance with regulatory requirements
As well as providing a general signal of the trustworthiness of algorithmic systems, audit can play a role in ensuring that they are used in ways that are likely to comply with regulatory requirements.
Regulators can use their legal powers to inspect algorithms in certain cases. See box 1 below for a non-exhaustive list of the legal powers of the 4 DRCF agencies.
Box 1: Examples of the DRCF regulators’ legal powers to audit algorithms
The CMA can use its statutory information gathering powers under competition law and consumer protection legislation to request information, including data and code, from businesses. For example, the CMA can use its information gathering powers when it launches an antitrust investigation, a market study or market investigation, or a merger inquiry. In addition, the CMA can compel individuals to attend interviews under the Competition Act 1998 and the Enterprise Act 2002.
The FCA has broad information gathering powers under the Financial Services and Markets Act 2000. These could be deployed to require firms to provide information about data and code. The FCA also has powers to commission a “skilled person” report, which might be used in the context of algorithmic auditing. In addition, the FCA has broad powers to conduct investigations, including compelled interviews and on-site visits.
The UK General Data Protection Regulation and the Data Protection Act 2018 gives the ICO a range of powers under which it can conduct off-site checks, on-site tests and interviews, and engage in the recovery and analysis of evidence. The depth of the analysis will depend on the context of the case. Data Protection Impact Assessments must be submitted to the ICO before the start of any personal data processing where the risk of a severe impact on data subjects is high and cannot be mitigated. This requirement may change in the near future on account of the UK government’s ongoing work to reform the UK’s data protection regime.[footnote 29]
Ofcom is preparing to take on new functions under the Online Safety Bill, which is currently being considered by Parliament.[footnote 30] The new regime will require “user-to-user services” (for example, social media platforms) and “search services” to carry out risk assessments and take steps to mitigate and manage identified risks of particular types of illegal and harmful content. Some service providers will also be required to publish transparency reports. Ofcom’s information gathering and investigative powers, including a specific power to carry out audits and powers to obtain skilled person’s reports, alongside its own research and that of third parties, would enable Ofcom to scrutinise the governance of platforms’ algorithms as appropriate.
In practice, some DRCF regulators have used their existing powers outlined above for algorithm audit in a number of ways. The CMA has undertaken several algorithm audits, including through the use of digital mystery shoppers to understand how consumers use digital comparison tools when making a purchase through website or app,[footnote 31] or in assessing whether websites are manipulating the presentation of online reviews of their products and services.[footnote 32] The ICO’s Assurance team has built an internal AI-specific Assurance toolkit, and it is undertaking a series of ‘friendly audits’ to test and improve it, while also supporting innovative companies committed to data protection compliance.
The ICO is also adopting additional approaches alongside legal enforcement to help organisations to achieve regulatory compliance, such as through its Regulatory Sandbox. Through the Sandbox, the ICO provided advice and support to Onfido as they developed their facial recognition technology. The purpose of this advice and support was to help Onfido measure and mitigate algorithmic bias in a manner compliant with data protection law.[footnote 33] The ICO has also developed its external AI Risk Toolkit that organisations can voluntarily use to assess compliance throughout the AI lifecycle. It has also created an Accountability Framework,[footnote 34] in addition to guidance on Explaining Decisions Made with AI and Guidance on AI and Data Protection,[footnote 35] to assist businesses with compliance.
Other regulators currently outside the DRCF are also considering the implications of AI. The Medicines and Healthcare products Regulatory Agency (MHRA) is in the process of updating its regulations as they apply to software and AI as a medical device. This is likely to include clarificatory guidance, standards and processes around pre-market requirements and a post-market surveillance system to capture adverse incidents.[footnote 36] The MHRA has also worked together with other regulatory organisations in the health sector to launch a multi-agency advice service. The service offers a single platform for advice and guidance on requirements across the remits of the regulatory agencies involved.[footnote 37]
In addition, the National Health Service’s AI Lab is trialling an algorithmic impact assessment for data access in the proposed National Medical Imaging Platform and the National Covid-19 Chest Imaging Database designed by The Ada Lovelace Institute.[footnote 38]
The role of standards
Standards will play an important role in the audit of algorithmic systems. Standards can include:
-
Standards for algorithmic processing: for instance, ensuring that algorithms are built using training datasets that are representative of the population being analysed in order to minimise the risk of biased outputs;
-
Standards for auditing algorithms: for instance, setting out how an auditor should inspect an algorithm for biased outcomes (for example, by running fresh data through a model and comparing how the results vary by demographic of the data subject); or
-
Standards for performance against which algorithmic system outputs can be benchmarked: for instance, setting out the criteria that would determine whether outputs were transparent, explainable or biased.[footnote 39]
The DRCF members are interested in all 3 of these types of standards, although there is some variation in the emphasis on the different types between our organisations. Regulators have an interest in ensuring that the benchmarks against which systems are tested are meaningful in a regulatory context, for instance ensuring that a ‘bias audit’ gives relevant information as to whether or not a system is complying with legal and regulatory requirements to avoid discrimination and treat users in a fair way. Algorithmic processing and benchmarking standards can help achieve this aim.
We also have an interest in establishing trust in the audit market, so that organisations and people can be sure that audits have credibility. This could involve professionalising the audit system, for example through standards for algorithmic audit, accreditation or chartering of audit bodies.
Some standards could be regulator-led. As mentioned above, the ICO has produced an AI Auditing Framework that encompasses tools for its own investigation teams to use when assessing organisations’ compliance, detailed guidance on AI and data protection for those organisations themselves, and an AI and data protection toolkit providing practical support to organisations auditing the compliance of their own AI systems. Regulators could build on this with further guidance and toolkits so other regulatory concerns beyond data protection can be addressed.
Others could be the product of industry or third-sector initiatives. International standards bodies such as the Institute of Electrical and Electronics Engineers (IEEE) Standards Association, the International Organisation for Standardization (ISO) and the International Electrotechnical Commission (IEC) are also beginning to develop standards for algorithmic processing. These international bodies are made up of experts, typically from industry and academia, who use these forums as means to achieve consensus on best practices for product design, organisational processes and measuring system performance.[footnote 40] Although work into developing standards for algorithmic processing is relatively nascent, the use of technical standards is already common practice in other contexts such as product safety and sustainability reporting.
Typically, industry-led technical standards are voluntary; however, there are often pull factors for companies to comply, such as technical standards translating regulatory requirements into product or process design. Indeed, under World Trade Organisation (WTO) rules, member states are obliged to use internationally agreed technical standards in domestic regulations, unless the standards are “ineffective or inappropriate” for achieving specific policy measures.[footnote 41] Where regulators designate standards, these standards can be used to provide a presumption of conformity with the essential requirements of a regulation. More study is needed to better understand where standards ought to be created by regulators and where they could be formulated by industry bodies.
The role of certification
Where appropriate standards are in place, it may be possible to create a system of certification. Approved auditors would be able to certify that organisations’ systems are being used in a way that is likely to meet certain regulatory standards or industry best practices. There may be a role for regulators in determining who is able to carry out certifying functions, and how certification interacts with the regulatory process.
At present, given the nascency of the algorithmic audit market, it can be challenging for a regulator to be certain that an auditor will undertake an algorithmic audit in exactly the way expected. If a regulator does delegate some responsibility for monitoring and assessment to a third party, it loses a degree of control over the process of oversight. This is a weaker form of regulation than where a regulator directly takes responsibility for auditing systems. The decision of a regulator to accept a form of delegated oversight must therefore trade the cost of a weaker form of regulation against the benefit of the potential for more comprehensive oversight over a larger range of systems than would be allowed by the regulator’s own capacity. Third party audit may be better used as a complement to existing regulatory assessment, rather than substituting for audit that would have otherwise been undertaken by a regulator directly.
Certification may indicate assurance from a third party that can help provide confidence to a regulator that its standards are being followed, although this would not be a guarantee of compliance unless a system was established where a regulator agreed to trust verification of compliance to an approved auditor. In some cases, the regulator may compel a firm to provide the governance documentation of the systems audited for the purpose of verifying compliance.
Even where there are no regulatory obligations requiring standards and certification, voluntary standards and certification tend to be driven by market incentives. Sustainability certification, such as Fairtrade, has been used by organisations to demonstrate that they follow ethical business practices. There is potential for a similar market-led certification scheme to emerge in the field of ethical algorithmic processing and auditing.[footnote 42]
Limitations of audit
Audit can be a part of a broader governance ecosystem that enables good practice and effective oversight. An important role of audit is to identify problems, however there are many reasons that audits will not surface every problem in a system, especially due to limitations in the conditions for testing. It could be very challenging for a technical audit (see below), for instance, to replicate the exact conditions under which an algorithm is deployed. In particular for machine learning algorithms the process is data driven and probabilistic in nature, so only statistical performance metrics can be given, which may provide limited insights. Hence the only way to know the exact output in all circumstances is to test with all possible inputs, which is obviously not possible in a real world system, which means that there is always a risk of unforeseen issues arising. Further, there can be feedback loops under which algorithms adapt their behaviour based on how other algorithms (or humans) respond to their actions. This can make it impossible to know what the outputs will be when simulating the algorithm output in an isolated test environment. In this case, audit may need to be accompanied by other methods for mitigating harms, such as impact and risk assessments that can allow organisations to address uncertainty and less observable potential future risks.
Assurance from an audit generally reports on a system at a specific point in time, so there may need to be a limit on the time from which an audit certificate is accepted as being valid. Issues may arise with dynamic algorithmic systems after being deployed and after previously being audited. Many machine learning algorithms are updated periodically with new data after deployment, and in some cases, models may be updated on a weekly or even daily basis. This model re-training is necessary to maintain performance as real-world conditions change. However, after retraining there is no guarantee that previous performance metrics are still valid, and it is possible that new biases or other issues may be introduced. A further difficulty can arise due to personalisation. Some models are now re-trained on a user’s device with local data, so different users will then have models that behave in diverging ways. In addition, any certification will only be applicable to the use of an algorithm for specific use cases, as other use contexts will engender different risks.
Key takeaway: Algorithmic audit is already in extensive use and regulators have important powers to this end; however the audit market is nascent and lacks standards against which to audit. Technical issues and the real-time nature of algorithms also raise questions regarding what conditions, and for how long, audit results can be valid. Rather than there being a single approach to audit, the nature of an appropriate audit will vary on a case-by-case basis, depending on the business model, the consumers or citizens concerned, the context of how the algorithm has been developed and deployed, and the regulatory environment relevant to its use.
Regulators may need to develop a range of approaches, depending on the particular use case and the associated level of risk in the context the algorithm is being used. The approach used may vary across regulators and even within the remit of a regulator depending on the use. There will be variety across algorithmic systems which may necessitate different approaches, for instance the type of approach used for a gaming app algorithm may be radically different from that used for medical devices.
Level of audit / what audit can involve
Algorithms can be audited at a number of different levels. One level of audit covers simply reviewing a system’s governance frameworks and documentation. Deeper levels of audit involve testing a system’s outputs or assessing the design and technical nature of a system.
The appropriate form of audit will depend on the context in which the system is used. A deep technical audit of a system may be time consuming and resource intensive. This may be relevant in a use case involving medical screening where individuals’ health outcomes are at stake, but unnecessary in most contexts.
The type of audit possible may also be constrained by the degree of access to the system. Regulators may be able to obtain greater access to a system than a third party private auditor or an academic.
Our broad typology includes 3 distinct types of audit: governance, empirical and technical.
Governance audit | Empirical audit | Technical audit | |
---|---|---|---|
Description | Assessing whether the correct governance policies have been followed. | Measuring the effect of an algorithm using inputs and/or outputs. | Looking “under the bonnet” of an algorithm at data, source code and/or method. |
Methods | Impact assessment, compliance audit (including transparency audit), conformity assessment. | Scraping audit, mystery shopper audit. | Code audit, performance testing, formal verification. |
Example | The draft EU AI Act has mandated conformity assessments for high-risk applications of AI. | Propublica undertook an investigation into the use of the recidivism algorithms by COMPAS through comparing predicted rates of reoffending with those that materialised over a two-year period. | Internal code peer reviewing has become a common practice in Google’s workflow development. |
Sources[footnote 43]
Governance frameworks & documentation
Governance audits broadly assess whether an organisation deploying an algorithmic system has the appropriate policies governing its use and has followed good practice in the procedures and documentation surrounding the system’s design and implementation. This could include a review of how transparent or explainable a system is – whether the purpose of the system and the basis on which recommendations or decisions are made are well understood by the party deploying the system and the system’s users or others affected by it. It could also involve monitoring the level, efficiency and effectiveness of human oversight, through considering the extent to which a human overseer accepts or scrutinises recommendations from a system, or demonstrates confirmation or automation bias. This may require access to further data and an understanding of the context in which it was used in order to interpret the results effectively. Such audits could also help organisations developing algorithmic systems by auditing their quality management systems or risk management frameworks.
These audits can be undertaken internally by an organisation checking its governance measures against best practice guidance or through employing external expertise. Utilising external governance auditors could be beneficial, as domain experts will know what to look for and the right questions, which may be lacking within an organisation.
Governance and accountability form an important part of compliance in some regulatory contexts. Where the outputs of an algorithmic processing system have impacts on individuals, the system will be subject to regulatory expectations such as around ensuring consumers or citizens are treated fairly, not discriminated against, and have their rights to privacy respected. The fact that these outputs have been generated by an algorithmic system rather than a human does not affect the requirement to comply with regulatory expectations.
Examples of governance audits include:
-
A financial services firm making decisions on whether to offer or decline a consumer’s application for credit or insurance makes use of an algorithmic system to make recommendations or even automate the decision-making process. However, it is still subject to the governance and accountability requirements of the FCA’s Senior Managers Certification Regime.
-
In response to the CMA’s investigation into Google’s Privacy Sandbox, Google offered a commitment to appoint a monitoring trustee whose role would include conducting a governance audit. The monitoring trustee would check internal processes around the development and implementation of Google’s proposed technologies. In particular, this governance audit would seek descriptions of data separation mechanisms and of the process through which Google records how criteria agreed with the CMA were assessed in key design decisions for relevant products.[footnote 44]
-
In a data protection context, it is important for organisations to have clear policies to identify processing that needs a data protection impact assessment (DPIA). Where a DPIA identifies a high risk that cannot be mitigated, organisations must have a process for reporting this to the ICO.[footnote 45] The ICO’s auditing framework includes a component for governance and accountability, [footnote 46] including leadership engagement and oversight, management and reporting structures and documentation and audit trails. It recommends that there is meaningful human input in order to mitigate the risk of automation bias in a machine-led decision process that involves the processing of personal data.
The ICO also includes a governance framework in its guidance on explaining AI in practice. This includes prioritising explanations, collecting and pre-processing personal data, building the system in a way that can extract information appropriately, translating the system’s results in to useable and easily understandable reasons, preparing implementers to deploy the system and presenting the explanation to the affected individual.[footnote 47]
Empirical audit
An empirical audit is designed to measure the effects of using an algorithmic system, by assessing the system inputs and outputs. Where access to the system is possible, for instance through an API or third-party sandbox, this could involve the auditing party testing the outputs that are generated by the system when using a testing dataset. This can test the system against certain hypotheses, such as whether a facial recognition system is less accurate when used on faces from particular groups.[footnote 48]
Where access is not possible, there are other ‘black box’ approaches that observe only the inputs and outputs, such as a ‘mystery shopping’ approach where accounts are created with specifically curated characteristics and tested on a live system. This is an approach often used by researchers and journalists investigating algorithmic bias.
A widely cited example of an empirical audit is the investigatory work by the news organisation Propublica, which claimed to find racial bias in an algorithmic tool used to predict reoffending rates amongst those arrested in the US. In the UK, the Advertising Standards Authority created digital avatars to understand whether children were being served online ads for gambling.[footnote 50]
An empirical audit is outcomes-focused and does not review the working of the system itself other than its performance in achieving particular outputs. In a regulatory context, the outputs can be aligned with regulatory standards, such as avoiding unequal outcomes for people with legally protected characteristics, or monitoring whether organisations are using algorithms to self-preference. For example, as part of the European Commission investigation into Google’s comparison-shopping service for self-preferencing their rankings, the Commission simulated switching search result ranking in order to see if the number of clicks changed.[footnote 51]
The development of Google’s Privacy Sandbox involves real-world experiments where other market participants test Google Chrome’s APIs and observe the outcomes. As provided for by Google’s Commitments, the company will work with the CMA to test the effectiveness of the APIs against criteria agreed with the regulator and, for example, measure their impact on publisher’s revenues. This aspect of the implementation of the Commitments amounts essentially to Google and the CMA undertaking an empirical audit, in addition to a governance audit, on the Privacy Sandbox.
An empirical audit can assess whether there are problems with the outputs from an algorithmic system, but does not generally reveal why those problems exist or how they can be resolved. Empirical audits could lead to further, deeper audits, and could therefore be considered a ‘first step’ by regulators before undertaking a technical audit.
Technical audit
A technical audit allows for a ‘look under the hood’ to find out where there may be issues in a system. A technical audit allows for exploration of a system’s inner machinations, to see if there are problems with the data, source code, or methods.
It could include:
-
Reviewing system inputs – for instance testing whether data is balanced and of high quality.
-
Assessing the development of the model – investigating the optimisation criteria that were used to train the algorithm (such as the loss function) and performance metrics used to evaluate the algorithm during its development. It could also include how the model was built, trained and tested, potentially including interviews with the developers to understand the workings of the system.
-
Reviewing measures used to control risk – such as technical controls for bias mitigation.
-
‘Stress testing’ the model through test data sets and carrying out simulations.
-
Reviewing the code – for instance undertaking static code analysis to screen for formatting errors, or detailed manual review by coding experts.
The CMA undertook a technical audit in its investigation of Google’s Privacy Sandbox. An initial step in Google testing its APIs involved functional tests to ensure each API functioned as intended – this complemented empirical tests to ensure the technologies did not entail new privacy or competition harms. In addition, Ofcom carries out soft and technical audits, and spot checks of the user interfaces as part of its accreditation scheme for digital comparison tools for the communications sector (phone, broadband and pay-TV).
Technical audits are often difficult to undertake, given the complex nature of systems and the challenges in gaining sufficient access to systems.
Consolidating approaches to audit
In some cases, as with the CMA’s investigation into Google’s Privacy Sandbox, all 3 approaches to audit could be relevant when reviewing a system for a particular purpose.
Consider another example in which an algorithmic system is used as part of a process for moderating ‘hate speech’ on a platform. A governance audit could review the organisation’s content moderation policy, including its definition of hate speech and whether this aligns with relevant legal definitions. It could review how the system fits into the overall ‘decision architecture’: for instance, whether the system auto-moderates certain types of content or flags certain content for human review. The audit could assess whether there is appropriate human oversight and determine whether the risk of system error is appropriately managed through human review. An empirical audit could involve a ‘sock puppet’ approach where auditors create simulated users and input certain classifications of harmful, harmless or ambiguous content and assess whether the system outputs align with what would be expected in order to remain compliant. A technical audit could review the data on which the model has been trained, the optimisation criteria used to train the algorithm and relevant performance metrics.
Outcome of audit
The main output of an algorithmic audit is likely to be a report detailing the audit methodology and the findings of the assessment. When undertaken by a regulator, the outputs may be included in a regulatory decision taken as part of enforcement action, for instance a decision that establishes an infringement of the Competition Act 1998.
There may also be ongoing tools for monitoring, such as reports or dashboards that update periodically to ensure ongoing performance management and compliance. The report may be made available to different stakeholders, for example it could be:
-
retained within the organisation for use by internal management only;
-
shared with shareholders or partners such as consultants hired to advise an organisation on change;
-
shared with regulators to help demonstrate compliance; or
-
made available to the public, making the system subject to public accountability through the means of academic or journalistic critique, and the choices of consumers and users of the system.
In some cases, a mixed approach is possible, with summaries available to the public and the detail restricted to the organisation or a regulator. For the audit outputs to be as effective as possible in driving improvement and establishing trust, it is important for the report to be as transparent as possible. Consumers or those affected by algorithmic systems who have a better understanding of these systems can then take informed decisions about how or when they engage with different products and services. Enabling meaningful engagement and understanding means that audits need to be communicated at the appropriate level of detail and complexity.
Where the outcome of an audit shows that the system has met a benchmark of quality set out under specific standards and has demonstrated compliance with legal frameworks, the audit provides a level of assurance for the deployer of the system and a way to signal quality to users or potential users.
Where the outcome reveals potential or actual issues within the systems, it enhances the understanding of associated failures and risks for both the developers and users of the system. In response to such findings of potential risks and failures, organisations may employ targeted mitigation strategies. If an organisation does not act to improve its systems following the discovery of issues in an audit, exposure of the report to public scrutiny allows the public, advocacy groups, academics or journalists to challenge and apply pressure to the organisation. Where regulators have access to the findings from an audit, a regulator can, where its remit allows, follow up with further investigation or enforcement activity, enabling access to redress for parties that are harmed by the outcomes of an algorithmic system.
Existing landscape
Parties involved in the audit landscape
Governments
In addition to the activities of regulators described above, governments have begun to propose legislation to address some of the risks of harms associated with the misuse or unintended consequences of algorithms.[footnote 52] These include the proposed Digital Markets regime[footnote 53] and the Online Safety Bill in the UK,[footnote 54] the amendment to the Acts against Restraints of Competition in Germany,[footnote 55] the Cyberspace Administration of China’s draft regulations for algorithmic systems,[footnote 56] the European Commission’s draft Artificial Intelligence Act, Digital Services Act, and Digital Markets Act, as well as data protection laws in several countries.
Some recent regulatory measures include specific provisions focused on algorithmic auditing. For instance, the draft EU AI Act mandates conformity assessments for high-risk applications of AI. Typically, this is an internal governance audit carried out to ensure that the governance of AI is compliant with the regulation; however, in specific high-risk cases external audits by notified bodies[footnote 57] are required. In the United States, senators have proposed draft legislation for an Algorithmic Accountability Act 2022, which would require impact assessments when companies are using automated systems to make critical decisions.[footnote 58] At a sub-national level, New York City passed a bill in November 2021 which requires hiring vendors to conduct annual bias audits of their AI systems.[footnote 59] Finally, the Canadian government has mandated Algorithmic Impact Assessments for federal government institutions[footnote 60] although stakeholders have noted that adoption has been limited.
There are clear indications that government interest in algorithmic auditing will continue to grow. In the UK alone, recent announcements and publications include the Centre for Data Ethics and Innovation’s AI Assurance roadmap, a UK government White Paper on governing and regulating AI, a pilot for an AI Standards Hub for coordinating UK engagement in AI standardisation globally,[footnote 61] and the Central Digital and Data Office’s Algorithmic Transparency Standard which seeks to help public sector organisations provide clear information about the algorithmic tools they use and why they are using them.[footnote 62] In 2020, the UK’s National Audit Office also worked with other public audit organisations in Norway, the Netherlands, Finland and Germany to produce a white paper and audit catalogue on how to audit machine learning models used in government agencies.[footnote 63]
Standards bodies
The IEEE has been working with experts from academia, industry, civil society, policy and government on its Global Initiative on Ethics of Autonomous and Intelligent Systems to produce a document, Ethically Aligned Design.[footnote 64] The aim is to push forward the discussion on establishing ethical and social implementations of AI and inspire the creation of standards and associated certification programs. Alongside several other related workstreams, the IEEE also leads The Ethics Certification Program for Autonomous and Intelligent Systems (ECPAIS) to create specifications for certification to address transparency, accountability and bias in autonomous and intelligent systems.[footnote 65]
ISO and the IEC have a Joint Technical Committee that is developing a number of standards, including trustworthiness in AI, bias in AI systems and AI-aided decisions making, and ethical and societal concerns.[footnote 66] Many of the standards have the potential to be used in the audit of algorithmic systems. There are numerous other groups that determine technical standards and specifications both broadly and narrowly, including open source communities that determine technical specifications for things such as programming languages, technical libraries and operating systems.
These efforts have been ongoing for several years, with implementation likely to take place over the next few years.
In financial services, the international standards-setting organisation IOSCO set out non-legally binding guidance related to how regulators may best address conduct risks associated with the development, testing and deployment of artificial intelligence and machine learning.[footnote 67]
Industry
Industry has begun to play an active role in developing internal and external auditing capabilities. Internally, some companies have set up their own oversight mechanisms in reaction to public pressure for scrutiny over their algorithms, such as Twitter’s algorithmic bias bounty challenge.[footnote 68] Several companies have also collaborated to develop codes of conduct for ethical AI through the Partnership on AI. From our stakeholder conversations, it emerged that some companies are carrying out internal audits or governance initiatives as part of their due diligence. For example, model risk management (MRM), including model audits, are standard practice in areas of the economy that have traditionally used data and statistical methods, such as financial services.
Current approaches to MRM, however, are designed for static models. These approaches do not take into account difficulties associated with data-driven machine learning models, particularly those that are updated following deployment.[footnote 69] It is also challenging to externally assess the impact of these and other internal audit activities given their limited external transparency and accountability. Accordingly, it is likely that improvement will need to take place for current measures to be sufficient to meet any potential future regulatory requirements for audit.
Externally, companies have begun offering algorithmic auditing services. Large traditional consulting companies have expanded their offering to include assessment and auditing services for algorithms. For example, one consultancy states that they offer a range of services including expertise in algorithms, risk management and coding skills, and helping organisations to understand how they use algorithms and address governance and oversight. Another created a tool for clients to identify and mitigate unfair bias in AI systems, as part of its broader suite of AI testing services. Recent years have seen the emergence of algorithm auditing start-ups and third-party small and medium-sized enterprises, which often offer services in relation to specific issues such as transparency or explainability of algorithmic processing systems. Audits conducted by start-ups can potentially target a wide variety of issues, and can also be used to perform due diligence on a system. The CDEI has collated a number of examples of these firms in its AI Assurance Guide.[footnote 70]
The third-party algorithm auditing sector is likely to grow significantly in the near future – not least because organisations are seeking more reassurance around the use of AI. Further, the proposed European Commission AI regulation will require that organisations using high-risk systems should undertake ex-ante conformity assessments before placing a system on the market. A limited number of these systems will require external audits, which will drive the development of the sector. However, there is currently a lack of agreed standards for such audits, at least across some sectors and areas of application.[footnote 71] Audits can range from suggestions for best practice to in-depth forensic explorations of an algorithmic system. As will be discussed in this section, addressing this lack of conformity is a priority.
Researchers in academia and industry
Researchers have undertaken various forms of algorithm ‘audits’ of companies’ systems, with many focusing on algorithmic bias and discrimination, and amplification of content or information. By one recent meta-analysis’ estimate, the number of English-language algorithm audit studies conducted by academics stands at 62.[footnote 72] Examples include work by academics at Northeastern University, who examined recruitment algorithms used by a company, Pymetrics.[footnote 73] Most audits led by researchers are undertaken without access to a company’s data and algorithms, i.e. they are empirical assessments based on the outputs of the algorithm.
Some researchers have also produced tools, such as the Aequitas bias and fairness toolkit,[footnote 74] and a method to detect discrimination in AI and machine learning systems created by researchers at Oxford that has been adopted by Amazon in its bias toolkit.[footnote 75] Researchers have also created frameworks intended to help companies audit their algorithms internally, such as the AI Now Institute’s Algorithmic Impact Assessment framework,[footnote 76] or those produced by industry researchers including Deborah Raji, Timnit Gebru and Margaret Mitchell.[footnote 77] Although highly valuable, these frameworks and toolkits that are deployed by companies internally often do not allow for external scrutiny of an organisation’s algorithmic processing systems. There is therefore a question to be raised about how much these tools support external accountability, where researchers can play an important role. Mechanisms to strengthen researcher access to data and algorithmic systems will need to be considered, to enable researcher participation in auditing and assessment efforts.
Journalists, civil society and whistleblowers
Journalists have played an important role in surfacing algorithmic practices. In 2019 the Wall Street Journal alleged that Amazon had changed its search algorithm to more prominently feature listings that are more profitable for Amazon. According to the Wall Street Journal, instead of showing customers mainly the most relevant and best-selling listings when they search, the change allegedly benefited Amazon’s own private label products on its platform at the expense of competing products on Amazon Marketplace.[footnote 78]
Other activities focus on enhancing transparency, supporting greater scrutiny, assessment and understanding of algorithms. The Markup is a nonprofit newsroom employing quantitative journalists to collect and analyse evidence on how technology is being used. Their investigations have found several instances of detrimental impacts of algorithms.[footnote 79] They have also created a ‘citizen browser’, a desktop app that allows a panel of paid users to share with The Markup what content they see on their Facebook accounts.[footnote 80]
Civil society organisations have taken a range of approaches to assessing algorithms and holding those using them accountable. Some are bringing legal cases before the courts to challenges harmful practices by companies using algorithms. Foxglove, a digital rights non-profit, pursues legal challenges such as the cases brought against the Home Office and government over the use of a visa algorithm and an A-Level grading algorithm,[footnote 82] respectively. In addition, the Irish Council for Civil Liberties has taken legal action against adtech companies over violations of the GDPR,[footnote 83] while 2 not-for-profit organisations in the Netherlands have brought privacy litigation against Facebook.[footnote 84]
Others are collecting data to uncover harms, such as Who Targets Me. This is a non-profit group that has created a downloadable browser extension to build a crowdsourced global database of political adverts placed on social media. The aim is to tackle a lack of transparency around personalisation in political advertising.[footnote 85] Finally, some organisations are producing frameworks and related research, such as the Doteveryone consequences scanning framework,[footnote 86] [footnote 87] and the Ada Lovelace Institute’s work on accountability of algorithmic decision-making systems.[footnote 88]
Finally, whistleblowers play an important role in uncovering detrimental practices, often at high risk to themselves. One of the most recent examples of this is the role Frances Haugen, a former employee at Facebook, played in exposing alleged inaction on harms caused by its recommender systems.[footnote 89] Another example is the ex-Google employee Guillaume Chaslot, who blew the whistle in 2018 on alleged biases in YouTube’s recommender algorithm.[footnote 90]
Issues with the existing landscape
This nascent audit ecosystem provides a promising foundation; however, work is still needed to ensure that algorithmic processing systems are properly scrutinised. To identify issues with the current landscape and potential solutions, we spoke to stakeholders with expertise in the use of the algorithmic processing systems across academia, industry, the public sector, and civil society. This included 14 interviews with experts: academics from the UK, United States and Canada; start-ups operating in the UK, US and EU; civil society bodies operating in the UK and EU, and representatives of government departments and regulators in the UK. We also hosted a workshop in partnership with the Ada Lovelace Institute, which brought together a broader group of representatives from academia and civil society. We held a further workshop for industry representatives to explore our initial ideas in more detail. Stakeholders were chosen based on their experiences and perspectives on auditing algorithms and knowledge of the landscape.
Key takeaway: Where a market for algorithm auditing services exists, it is at an early stage of development. Efforts to proactively surface and identify new issues in algorithmic systems through auditing have been particularly slow to emerge. This is where there may be a role for the public sector in incentivising an ex-ante approach.
The following sections summarise specific issues raised by stakeholders we spoke to.
Lack of effective governance in the ecosystem
Stakeholders in the AI auditing industry were concerned about the quality of audits in what they perceived as a currently largely unregulated market for auditing.[footnote 91] They were concerned that this risked becoming a “wild west” patchwork where entrants were able to enter the market for algorithm auditing without any assurance of quality. Indeed, some investigative journalistic publications such as The Markup have established themselves because of a perceived lack of accountability and regulation around algorithms. Several stakeholders noted that regulators are unlikely to have the capacity to investigate every instance of alleged harm, and that journalists, academics and other third parties play an important part in scrutinising algorithmic systems.
In our engagement with stakeholders we also observed that there is a lack of clarity about standards that auditors should be auditing against. As mentioned before, regulators such as the ICO have been developing toolkits to provide guidance.[footnote 92] Despite such work, some stakeholders we spoke to felt existing standards were often vague and based on general principles, with very few distilled down into anything that can be tested against. This lack of clarity around what good auditing and outcomes look like could act as a disincentive for organisations to pursue internal or external algorithmic auditing. We found that the development of sectoral or domain-specific principles and testable criteria could help to address this lack of clarity. For example, such developments are happening in the health sector.[footnote 93] [footnote 94] However, establishing such guidance may prove difficult for horizontal regulators who work across sectors and could prove to be less effective than test criteria developed by companies themselves. As such, members of the DRCF may wish to undertake further work to explore which types of regulatory measures are appropriate in which contexts.
Standards bodies are likely to fill some of the gaps left by regulators, yet there are concerns around a lack of transparency over how they are developed and by whom.[footnote 95] Standards making is often portrayed as a “neutral” process led by experts within the specific technical area, yet academic research has revealed that these processes are political.[footnote 96] Indeed, given that many technical standards for algorithms will involve value-based choices, some academics have been critical of relying too heavily on standards bodies, which often lack civil society representation and democratic oversight.[footnote 97] Moreover, from a geopolitical perspective, the EU, China, and the US have all explicitly stated a desire to take a leading international role in the development of technical standards for algorithms.[footnote 98] More research is needed to understand how this may impact the governance of audit in the UK.
Insufficient access to systems
From speaking to stakeholders from academia, civil society and industry we observed that the quality of audit may be compromised if it is not possible for appropriate auditors to obtain sufficient access to algorithmic systems.
In a July 2020 blog post, the then-CEO of TikTok stated that ‘all companies should disclose their algorithms, moderation policies and data flows to regulators’, and outlined some steps that TikTok had taken to do so.[footnote 99] [footnote 100]. In some cases, extensive transparency and granular explanations should only be shared with those that need to understand them (i.e. auditors or regulators) given that they could increase the risk of unwanted manipulation of the algorithms (i.e. ‘gaming’)[footnote 101] or, in the case of pricing algorithms, facilitate collusion by market participants.[footnote 102] Academics are an important part of the algorithm audit landscape and have the opportunity and incentive to find new problems and unintended harms if organisations are themselves incentivised to be more transparent and share data. Such access for academics would, however, need to be balanced with any legitimate privacy and commercial concerns relating to the organisations being audited. A possible technological solution could rely on a third-party sandbox in which algorithms can be shared by their owners and analysed in a privacy-preserving manner by appropriate external parties. There are technologies in active development that could further support such privacy-preserving analysis.[footnote 103] [footnote 104] Nonetheless, introducing these sandboxes may still prove resource intensive and require long lead times to implementation. Furthermore, academics and other auditors will often need access to domain experts who can explain the context in which algorithms have been developed and how they function.
Academics and others also noted that a significant barrier to their success in finding new and unintended harms was their perceived lack of legal protection or certainty for their investigative methods. Some participants noted that they can face legal action based on what they saw as an unclear legal stance on web scraping and risks around creating fake users of systems to understand how they operate and uncover harms.[footnote 105] For example, researchers from New York University who studied political disinformation on Facebook faced legal threats in 2020[footnote 106] and subsequently had their accounts disabled in 2021 for scraping data.[footnote 107] It was also noted that a lack of clarity around the UK GDPR as it applies to researchers create further problems for academics. The UK GDPR already contains research provisions[footnote 108] and the ICO has drafted guidance on this issue.[footnote 109] The ICO has also produced guidance that sets out what stakeholders can or cannot do with publicly accessible data.[footnote 110] Providing greater legal certainty around when academics and others can legitimately access to technology companies’ data could ameliorate these concerns.
Our engagement with stakeholders also revealed a concern that some organisations use ‘trade secrets’ as a way to block access to systems. Organisations may be more willing to provide access to systems to auditors where the audit is not made public and for the benefit of the organisation only; for external audit that may be provided to the regulator or the public, they may offer only limited access. Nevertheless, where enforcement action is taken against an organisation, regulators have full access to systems. In order to allow proportionate regulatory oversight, in its Online Platform and Digital Advertising Market Study the CMA proposed a principle around making high-level “objective functions” of algorithms transparent.[footnote 111]
Finally, a lack of access to effective documentation by the audit client makes audit more challenging to conduct. Some clients will use open source code downloaded from the internet, add their data to it and fail to effectively document where the source code comes from. This could prove to be problematic if the code has been developed in a different jurisdiction such as the US, as it will not have been developed for the UK regulatory context, complicating auditing activity. Relevant documentation might include communications and internal documents about the business context, objectives, design, architectural diagrams, training (including relevant function(s) that has been maximised during an algorithm’s training stage), key performance indicators, and monitoring of algorithmic systems.[footnote 112] The guidance produced by the ICO and The Alan Turing Institute outlines the policies, procedures and documentation that firms could provide to regulators.[footnote 113]
Insufficient avenues to seek redress
We highlight in our accompanying benefits and harms of algorithms paper that transparency is an important means to accessing redress. [footnote 114] Auditing can indicate to individuals that they have been harmed, for example from a biased CV screening algorithm. It can provide them with evidence that they could use to seek redress. However, there is an apparent lack of clear mechanisms for the public or civil society to challenge outputs or decisions made with algorithms or to seek redress. Regulatory cooperation will be important to ensure that people can seek redress without having to navigate separate regulatory systems themselves. We also noted that stakeholders considered it important for regulators to commit to actions in response to audits that surface problems.
In the EU, civil society bodies have also called for the European Commission’s AI Act to include provisions for individual and collective redress. They have also recommended the creation of a mechanism for public interest organisations to lodge a complaint with national supervisory authorities.[footnote 115]
Under the UK’s data protection framework, individuals have rights in relation to how their personal data is used. These include the right to: access (a subject access request), rectify, erase, restrict or object. Where individuals are subject to automated decision-making with legal or similarly significant effects, they have additional rights, which include the right to obtain meaningful information about the logic involved and to contest the decision. However, some have argued that data protection law only provides remedies for unfair outcomes for individuals and lacks them for groups or communities. This makes it harder to detect the systemic-level impacts arising from automated systems.[footnote 116]
Inconsistency of current audits
Some stakeholders from academia and industry felt that the current focus on governance and documentation was insufficient. In some cases, they thought that it will be necessary to develop statistical tests for technical audits to evaluate the concerns that we as regulators and society have with algorithmic systems. Those who develop these tests will need to be upfront about which harms the tests assess and which they do not, and the relevant regulator can then evaluate whether the test is effective for the regulator’s purposes. Regulators may also signal the categories of harms or aspects of AI they find particularly relevant to their remits. For example, in a blog, the US Federal Trade Commission stressed the importance of being transparent, explaining decisions to consumers, ensuring that inputs are accurate, and that inputs, processes, and outcomes are fair. Where there are concerns about specific harms, the Ada Lovelace Institute and Datakind UK have proposed a ‘bias audit’, to test for given hypothetical harms using a systematic analysis of inputs and outputs.[footnote 119]
In practice, conceptual and practical difficulties have emerged when attempting to ensure consistency between audits. One difficulty is the question of how to operationalise the concept of “fairness”. There is no agreed upon definition of what constitutes fairness,[footnote 120] with this differing depending on sector, application and context of use. Typically, fairness falls into 2 categories:
procedural fairness that focuses on fair treatment of individuals; and outcome fairness which is concerned with what decisions are made.[footnote 121] Even within these 2 broad categories, there are numerous statistical definitions of fairness that are often mutually exclusive.[footnote 122] Therefore, determining which idea (or ideas) of fairness is appropriate within a particular context, as well as determining suitable measurements for bias within the applicable context, are necessary for deciding whether the findings of an audit are problematic.[footnote 123] Take the earlier example of the Propublica investigation into algorithmic predictions of criminal reoffending; Northpoint, the company that developed the algorithmic system, claimed that harmful bias was not present because they used a different conception of fairness.[footnote 124]
There is also the practical question of what is being considered within an audit. A widely publicised example was an audit by ORCAA, the AI auditing firm set up by Weapons of Math Destruction author, Cathy O’Neil. Her organisation was contracted by HireVue to conduct a review of its facial analysis technology, which it used in job interviews to judge candidates’ suitability. In its report of the audit, the company claimed that the audit did not find bias in HireVue’s candidate assessments. However, it has been reported that the parties had agreed that the audit focus only on a specific use case, and it therefore did not examine assessments using facial analysis.[footnote 125] This type of practice could lead organisations to “ethics wash” through making misleading or superficial claims about the scrutiny their systems have been subjected to.[footnote 126]
Despite these drawbacks, technical audits can help support greater public transparency of algorithmic processing systems. Pymetrics, a company offering a candidate screening service, conducted a joint audit with researchers at Northeastern University in the United States. The aim of the audit was to determine whether Pymetrics’ algorithms discriminate against candidates based on race or gender. The audit found that although the company’s algorithms were not completely free of bias, they did implement the fairness guarantees that they claimed to, and also satisfied the US recruitment industry’s ‘four-fifths’ hiring guideline.[footnote 127] The auditors also put in place mechanisms to ensure the independence of the audit, and ensured they were able to report publicly about the audit.[footnote 128]
These examples demonstrate that the methods used and interpretation of the results of audits can vary widely and may need sector-specific guidance or standards to ensure good outcomes. The examples also demonstrate that there may be a need for bespoke approaches to auditing algorithmic systems depending on the context in which they are deployed.
Other issues
Another concern we heard among industry stakeholders related to the relationship between the auditor and any potential rivals of the audited organisation, and whether there are any existing commercial relationships. Strong legal protections around the use of data in the context of an audit would be required to address this.
Finally, the costs of audit and any other related regulatory activity must be carefully considered. Audits may have high financial costs associated with them, which may mean that larger organisations are better able to adapt and absorb the additional costs than smaller organisations. Any perceived burden of regulation or audit could also impact the incentives on firms to innovate. We are highly mindful of these potential consequences, and we strive to ensure our regulatory activities are proportionate and encourage innovation for both small and large organisations.
Potential future landscape
Members of the DRCF include cross-sectoral regulators such as the CMA and the ICO, as well as sector regulators such as Ofcom and the FCA. As the DRCF we are exploring topics of common interest across our combined remits, but as individual regulators our regulatory responses and approaches to auditing may necessarily differ in line with the different contexts we oversee and our overarching regulatory approaches. Some regulators may favour a hands-on approach to auditing companies or introducing specific requirements, while others may choose to rely more heavily on industry self-governance. For instance, the FCA is technology-neutral and holds firms accountable for fair consumer outcomes irrespective of the technologies and processes they deploy.
Where they are required in the future, the type and depth of audits will likely vary depending on the nature and size of the potential harms, the ability to specify clear ex-ante rules, and the costs of auditing. For instance, there may be stronger requirements around effective assurance of algorithmic systems where they are involved in sensitive applications and the stakes are particularly high for people, such as in healthcare, recruitment or education. In some contexts, regulators could consider requiring higher risk systems to undergo a third-party audit. In other contexts, regulators may wish to require the results of internal or external audit be made available to the regulator before the system is put on the market, as occurs in the regulation of some medical devices.[footnote 129] Some regulators might consider that access to algorithmic systems for regulators or a self-regulatory body may only be necessary where specific concerns arise through ex-post enforcement. This could be the case in areas judged to be lower risk or where regulatory requirements and expectations are already established and strong.
Overall, regulatory agencies including the DRCF members are likely to make individual choices based on contextual factors, working with each other and with central government where appropriate to determine appropriate approaches to auditing organisations’ algorithmic systems. It will be important for us to ensure that compliance costs are proportionate, and implementation is as straightforward as possible, so that the introduction of new auditing requirements can feasibly be met by organisations. This would help facilitate the trustworthy use of algorithms and provide the regulatory clarity needed to stimulate innovation. Likewise, it will be important that regulators coordinate and where possible minimise additional burdens to industry beyond those necessary to ensure good outcomes for society.
This is not to say that regulators will be the only important actor within the future audit landscape. Our stakeholder engagement suggested that industry-led initiatives, as well as regulator-industry collaboration, could be important for the development of an effective algorithmic auditing ecosystem. The remainder of this section considers the potential role that regulators and industry could play in the future auditing landscape.
Role for regulators
Regulators will likely play an important role in the future audit landscape to ensure that the application of algorithmic processing systems is trustworthy and legally compliant. However, some of the stakeholders we engaged with suggested that regulators would not have the capacity to assess or audit algorithms themselves at the scale required to address harms of various degrees across sectors. Instead, in their view possible roles for regulators included: stating when audits should happen to ensure that parties are more likely to comply with the law; establishing standards and best practices to reflect our views on how audits may encourage legal compliance; acting as an enabler for better audits; ensuring action is taken to address harms identified in an audit where appropriate; and identifying and tackling misleading claims and practices.
Stating when audits should happen
Depending on the potential risk of harm of an algorithmic system, regulators could issue guidance on when an audit should take place. Audit requirements could be internal to an organisation, undertaken by a regulator, or provided through an external organisation – a topic that will be specifically addressed in this section. on external audit markets of third party providers. There are likely to be 3 primary ways regulators could state an audit should happen (as appropriate):
-
Before or as algorithmic systems go live.
-
At regular intervals: given the dynamic and changing nature of algorithmic systems, it may be necessary to monitor them through regular audits on an ongoing basis.[footnote 130]
-
After there are specific concerns raised about harms with respect to an individual organisation, for example in enforcement cases where agencies seek to establish whether there has been a breach of the law, or where a major error occurs.
The CMA has been investigating Google’s Privacy Sandbox proposals to remove third party cookies and other functionalities from its Chrome browser, based on competition concerns in digital advertising markets. Google has made commitments to address these concerns, including a monitoring arrangement to ensure ongoing compliance with these commitments.[footnote 131] In addition, pre-validation of algorithmic systems (which involves the processing of personal data) is already required to a certain extent under the UK GDPR’s requirements relating to Data Protection Impact Assessments, which must be submitted to the ICO if processing of personal data is likely to result in a high risk to individuals.[footnote 132] [footnote 133] Other approaches that regulators could promote include the use of bug bounties[footnote 134] or other rewards to incentivise individuals to proactively detect problems and share their findings with regulators.
Establishing standards and best practice
Our engagement with stakeholders suggested a need for clear standards and best practices on which audits could be based, and clear criteria against which algorithms could be tested. This is echoed in the AI Assurance Roadmap produced by the Centre for Data Ethics and Innovation.[footnote 135] Regulators can play a key role in establishing this guidance. Further regulatory guidance could make auditing more accessible through creating clear criteria and providing guidance on documentation and the level of required transparency. This could make it easier for companies, regulators, and external auditors to inspect algorithmic systems.
Such guidance could be created by regulatory bodies, either by themselves or in collaboration with other public-sector groups and standard-setting bodies. Guidance could also be general or could exist only for specific use cases where high-risk outcomes have been identified. Finally, guidance could be prescriptive where appropriate, for example in relation to a specific sector, or outcome-focused, with the private market left to create the tests to demonstrate that systems are producing those outcomes in the latter approach.
There are advantages and disadvantages to each approach. For instance, the private market could be more agile than regulators in innovating the tests that could demonstrate that the processes and outcomes desired by regulators have been achieved. On the other hand, the tests developed by the private market may not adequately assess for the outcomes desired by regulators. Further, outcome-based regulation may fail to provide the clarity needed to industry, potentially impacting innovation. It is likely that any regulatory measures introduced will be a hybrid of the approaches described above. As an example, broader outcome-based guidance could be complemented by context and use-case specific guidance that is more prescriptive where higher levels of risk are perceived.
In deciding which measures are appropriate, digital regulators could also draw on other sector regulation for inspiration. For instance, where the impacts of harms to individuals and society are potentially very high, financial regulation offers examples, including the notion of embedding compliance staff within large companies. In addition, under Section 166 of the Financial Services and Markets Act 2000, the FCA can require a company to pay for an approved person to do an independent assessment (or a ‘skilled persons reports’) on a specified matter. This model could be applied for digital organisations.
Act as an enabler for better audits
At present, there may be obstacles to conducting governance and technical audits effectively, as this typically requires the full cooperation and agreement of the organisation being audited. Some experts we spoke to from academia and industry stressed that regulators could facilitate better audits through introducing specific algorithmic access obligations; whether these access obligations can be implemented will vary across regulators and depend on their respective remits.
Providing greater access obligations for research or public interest purposes and/or by certified bodies could lessen current information asymmetries, improve public trust, and lead to more effective enforcement. It would be important to carefully consider the costs and benefits of any mandated access to organisations’ systems, beyond access for regulators where appropriate, and to consider alternative approaches.
Some regulators may wish to explore avenues for enabling scrutiny of algorithmic systems without unduly burdening industry. One possibility might be to only provide access to certain elements of an algorithmic system. Organisations that are the target of audits may be concerned about their intellectual property. However, empirical audits which assess the inputs and/ or outputs of a system (depending on the harm being investigated) may not require access to the ‘black box’, which may alleviate this concern. Likewise, access to the algorithmic system itself may not be required if the auditor is undertaking a governance audit focused more on the organisational measures in place around the algorithmic system.
Another possibility might be to control who has access to different elements of the algorithmic system. Access could be given to different extents to different parties, for example where access is required, an auditor certified by an appropriate body could undertake the audit. Auditors could be required to operate under a non-disclosure agreement for the data they inspect, but not for any other aspect of the audit to ensure the audit’s transparency and accountability. The DRCF could consider precedents from other audited sectors in developing its governance mechanisms.
However, where parts of an algorithmic system are built on elements from several different providers, identifying where in the supply chain the auditing should take place could be challenging. This challenge could be addressed through contracts between organisations in the supply chain that clarify at which stage auditing takes place, and who the responsible parties are for ensuring the timely completion of such audits. The feasibility of this solution for open source code will require further consideration.
Alternatively, some regulators may want to expand the use of regulatory sandbox environments in the future, to test algorithmic systems and check for harms in a controlled environment. Regulators could also collect data from organisations, for example on the safety and bias of algorithmic systems. In some cases, they may wish to analyse the data themselves to determine whether they are satisfied that the systems are sufficiently safe and free from bias. If appropriate, regulators could share sufficiently anonymised data with select third parties such as researchers to enable further investigation and reporting.
Another possible step for some regulators could be to issue case studies and guidance on how existing regulatory principles or rules apply where algorithms are deployed. As an example, the CMA’s research paper on algorithms and competition and consumer harms includes a number of direct harms to consumers that, if they are likely to harm UK consumers’ economic interests, are likely to fall foul of the general duty on traders not to trade unfairly by acting contrary to the requirements of professional diligence.[footnote 136] In addition, other DRCF members may want to consider building on the ICO’s DPIAs and providing guidance on how DPIAs could be complemented to address the impacts in other regulatory areas, such as consumer protection or competition.
Ensure action is taken to address harms identified in an audit
When a significant problem or breach of the law is identified through an audit, regulators could, subject to their powers, prohibit organisations from using the system until the organisation has addressed and mitigated the harm. This approach would vary widely depending on the nature of the harm and legal breach identified, and the nature of the impact on consumers and citizens of disrupting the use of the algorithmic system. For instance, regulators could work together to establish certain red lines where algorithmic systems cannot be used based on their perceived risk to the public, building on the right to restrict processing of personal data under the UK GDPR. Regulators could also share insights gained from audits on how algorithmic systems can create harm, and how this can be mitigated. This can help inform algorithmic design from the outset, or allow companies to gain a better understanding of how they should audit their own algorithmic systems.
Some stakeholders we spoke to from civil society warned that any remedies created by regulators or the parties involved following an audit need to be carefully considered. If they are badly designed, they could fail to address the underlying problem and result in negative unintended consequences.
In order to incentivise organisations to come forward to the regulator when they identify problems in their algorithmic systems, regulators could choose to adopt a form of leniency system. Such a system would provide incentives for organisations to disclose harmful outcomes to regulators, for example less strict enforcement or reduced fines.
The public may also benefit from a way of reporting suspected harms from algorithmic systems, alongside the journalists, academics and civil society actors that already make their concerns known. This reporting could include an incident reporting database that would allow regulators to prioritise audits.[footnote 137] It could also comprise some form of popular petition or super complaint mechanism through which the public could trigger a review by a regulator, subject to sensible constraints. That being said, without parallel transparency requirements on firms across regulators, it may be difficult to evidence differing treatment or other algorithmic harms. This could lead to regulators being provided with poor quality information that they cannot act on effectively.
It may also be beneficial to include the public in the design of algorithmic systems themselves, rather than only being involved once the system has been deployed. We heard that some consumers want much more control over the algorithmic systems they are subject to, in part to be able to enforce their rights.
Identifying and tackling misleading claims
Regulators have an important role in receiving, understanding, and responding to complaints surrounding misleading claims made about and practices involving algorithmic systems. Many AI systems that predict social or other human outcomes have been found to be flawed and inaccurate, leading to harmful consequences for those impacted.[footnote 138] For example, Dressel and Farid (2018) found that the controversial commercial risk assessment software COMPAS used to predict recidivism was no more accurate than a simple linear classifier using a fraction of the number of features.[footnote 139] The British Medical Journal has also expressed concern around risks to patient safety from misleading studies claiming that AI is as good as, or better than, human experts at interpreting medical images.[footnote 140]
Role of self-governance by industry
Self-governance by industry is likely to make up another important element of the future landscape. Organisations using algorithmic systems could introduce internal mechanisms for checking the processes of their systems. These could be internally developed frameworks or based on regulator guidance.
A healthy AI ecosystem incentivises and rewards effective self-governance. In many other fields, industry has developed effective incentive systems to understand and check internal practices. Sustainability reporting provides a clear example of this. As of 2020, 96% of the world’s top 250 organisations produced sustainability reports on the environmental, social and corporate governance (ESG) elements of their businesses.[footnote 141] This reporting is typically guided by industry-led standards, which businesses can benchmark themselves against to demonstrate to investors and consumers that they are a socially beneficial enterprise.
In the field of algorithmic processing, industry bodies and third sector organisations working together with academia and industry would be well-placed to develop technical standards and tools to support algorithm audits. Efforts to develop such schemes are beginning to emerge. For example, the non-profit Swiss Digital Initiative has created a Digital Trust Label, which creates criteria that independent third-party auditors can use to assess organisations’ systems for security, data protection, reliability, and fair user interaction.[footnote 142] There is significant potential for industry to develop its own transparency and self-reporting mechanisms, based on an internal assessment of organisational practices.
Industry self-governance could have many benefits including greater dynamism over how auditing is undertaken and lower costs from regulatory burdens. However, it is likely that self-governance is only appropriate for a sub-section of algorithmic systems, specifically lower-risk systems. Using the above example of sustainability reporting, it has taken over 20 years for this to become a common practice, indicating that a similar industry-led ecosystem may be slow to emerge.
Role of external audit markets
Collaborative external audit markets are a potential third way between a complete regulator- and industry-led approach to algorithmic auditing. An effective external auditing market could complement or possibly substitute for formal regulatory activity in some areas, while still providing assurance that industry is acting in a trustworthy way through using market incentives. Stimulating an external audit market could lessen the burden on regulators through lessening requirements for producing guidance and checking practices. Trusted audits could also help organisations to signal the quality of their algorithmic systems and demonstrate compliance with legislation. If introduced properly, external audit markets for algorithmic systems could prove beneficial for all parties interested in algorithmic audit.
External audit markets
Stimulating an effective external audit market for algorithms would involve regulator and industry cooperation in defining guidance and standards. External audit markets are already present in several other fields, such as for medical devices and cybersecurity. These markets differ in terms of the extent to which they are regulator, market, or collaboratively led. For instance, it has become common practice for the company sustainability reports described above to be externally audited; an initiative that has almost exclusively been driven by industry. Here, we are interested in collaborative external audit markets that are both regulator and industry driven.
Regulators can work with standards bodies to develop specific criteria against which algorithms can be audited. One example of an existing external audit market is the UK’s Cyber Essentials Scheme; for this project, the National Cyber Security Centre worked alongside the IASME Consortium to develop a cybersecurity standard for small businesses. Organisations can choose whether to undertake a self-assessment or a third-party technical verification, with certification provided to match the level of assessment.[footnote 143] Any IASME Consortium approved body can act as the third party-party auditor, which has created a market of firms capable of offering certification services. Although the Cyber Essentials Scheme is voluntary, in other cases audit could be a requirement, as is the case for a range of products that require a “CE” marking in the EU, signifying the product conforms to legal requirements.[footnote 144]
For algorithmic processing, a nascent market of third-party auditors is beginning to emerge, both from traditional professional services firms and SMEs. However, this market is predominantly industry-led and is currently constrained by a lack of common standards or regulator-supported certification, meaning that audits may not necessarily provide sufficient assurance over the trustworthiness of a system or build adequate trust between parties. Regulator-backed certification could be promoted, which would provide consumers with more information when making a decision between products, and may allow space for consumer-led challenges to a specific organisation’s certification. Helping grow these capabilities would support the DRCF in achieving its ambitions of addressing the unique challenges posed by regulating online platforms.
Potential challenges with creating an effective external audit market
Some stakeholders were sceptical that a market for algorithmic auditing could arise that would effectively surface problems. For example, a third-party auditing company that is paid by the target of the audit could lack the incentives to find fault with the target. This type of regulatory capture is one of the key issues with the credit ratings agency market which helped facilitate the 2007-2008 financial crash.[footnote 145]
Some experts we spoke to were concerned that algorithm audits could become dominated by the large technology companies and the ‘Big Four’ financial auditing firms,[footnote 146] potentially threatening market competition. There were also concerns about the effect on competition and the risk of a concentrated market developing due to a shortage of relevant skills. Employees from large technology companies could become auditors and supply auditing services to their previous employers. These ex-employees could face pressure to produce favourable results. Further, barriers to entry for smaller auditing firms in the absence of regulatory requirements and standards for audit could mean that clients seek out those large technology companies with ‘brand recognition’ for their auditing needs.
What regulators could do to encourage a well-functioning market
We heard from some experts across industry, academia and government that one of the key initiatives that regulators could usefully undertake is the development of clear guidance for external auditing. This guidance could range from introducing common language for risk management, to developing standards for benchmarking, to creating standardised tools and methodologies for third-party audit. The view was shared that translating regulatory concerns into measurable criteria and supporting the creation of practical tools could help ensure a competitive, well-functioning market for audit develops.
Some stakeholders felt that there may be an important role for regulators to support the development of an external auditing market by removing barriers to effective audits. For instance, there may be settings where regulators want to ensure that third-party auditors have access to information and organisations’ systems to be able to successfully undertake audits. For voluntary audits to be effective, regulators could support sufficient access to the aspect of the algorithm being audited, be that the data, model, inputs and outputs, or wider governance documents. For mandatory audits, it is important that problematic findings or a lack of cooperation are backed up with consequences. Some of the academics we engaged with also held the view that certification and accreditation would need to be accompanied by sufficient public transparency regarding how audits themselves were carried out, and their results, for trustworthiness to be achieved.
Even if access is sufficient, a number of steps need to be taken to overcome the potential risk of poor quality audits. Regulators may wish to encourage professionalisation and accreditation of auditors to ensure that baseline capabilities are present. Furthermore, regulators are likely to have a role in providing guidance as to their expectations of auditors and auditing firms. Our stakeholders were divided over what degree of liability was appropriate for auditors, with some arguing that weak protections would discourage audit, while others felt that imposing liability on auditors was important in order to engender trust in audit firms. Finally, there is the risk of auditors being captured by the target company. Licensing or accrediting auditors and putting in place ways to fine auditors or prohibit them from operating could help overcome this issue. However, some stakeholders from academia and civil society warned that regulators themselves would also need to avoid regulatory capture when faced with lobbying from well-resourced large technology companies whilst developing any regulatory regime for algorithm audit.
Regulators could therefore consider creating or supporting standards that set expectations for the independence of an audit, minimum transparency requirements, and the scope of an audit. Some regulators could also consider requiring organisations using algorithmic systems to publish a ‘notice of use’, to make consumers and affected parties aware of their use. In addition, regulators could encourage organisations to procure audits of their systems in order to promote better outcomes both for their businesses and those impacted by those systems. Finally, some regulators could explore creating a confidential database through which auditors could share the results of audits with regulators. A database of this kind may help build an evidence base for enforcement investigations. Some regulators could also explore encouraging and incentivising auditing providers to seek out new issues and harms, rather than focussing solely on existing harms. ## Conclusion and next steps
Conclusion
AI and other algorithmic processing systems benefit society in many important ways and in many instances work well. To check that algorithmic systems are operating in their intended way and without unknown, harmful consequences, organisations can conduct audits, either independently or through using external third-party providers. This might cover governance, technical and empirical audits. Done well, those who review their own systems internally through self-governance can benefit from greater dynamism over how auditing is undertaken and lower costs from regulatory burdens.
There have been instances where concerns about algorithms have not emerged until other actors inspected these systems. Regulators, academics, third-party auditing companies, civil society bodies and journalists may approach auditing in different ways, and, depending on the context, may all have a part to play in ensuring algorithmic processing systems are subject to adequate scrutiny.
The stakeholders we engaged with pointed to a number of issues in the current landscape of algorithmic auditing. First, they suggested that there is lack of effective governance in the auditing ecosystem, including a lack of clarity around the standards that auditors should be auditing against and around what good auditing and outcomes look like. Second, they told us that it was difficult for some auditors, such as academics or civil society bodies, to access algorithmic systems to scrutinise them effectively. Third, they highlighted that there were insufficient avenues for those impacted by algorithmic processing to seek redress, and that it was important for regulators to ensure action is taken to remedy harms that have been surfaced by audits.
Regulators seek to ensure that markets work well for consumers and that people are protected from harmful content, products and services. This entails a level of scrutiny that minimises risks of harms, whilst also encouraging companies to innovate and deploy systems that will benefit society. Any role for regulators in auditing must be proportionate to the harms involved and the value audits might bring.
There is a potential role for regulators including DRCF members in supporting the healthy development of the auditing landscape, which is likely to need to bring together different tools and approaches to surface harms and enable responsible innovation. For some regulators this might include stating when audits should happen; establishing standards and best practices; acting as an enabler for better audits; ensuring action is taken to address harms identified in an audit; and identifying and tackling misleading claims about what algorithmic systems can do. Industry will likely have an important role to play as the landscape develops, in some cases through self-governance to complement any regulator activity, and potentially through working together with regulators to ensure that the external audit ecosystem can deliver effective, relevant audits where these are required.
Next steps
During our first year operating as the Digital Regulation Cooperation Forum, we have engaged with other regulators and with stakeholders from government, academia, industry, and civil society. The aim of this engagement has been to set the foundations of our cooperation and what we can do to support a healthy ecosystem for algorithmic processing.
As one of our next steps we will widen our engagement through a Call for Input on a set of hypotheses related to the potential role for regulators in the algorithmic audit landscape. We do not anticipate a common approach across all regulators and contexts. Nonetheless, considering these hypotheses will help us test ideas that may be applicable in some settings, identify further areas of common interest for the future, and focus some of our collective work. We are interested to explore and receive input on the below hypotheses (see Call for input section for more information on our questions and how to send us your views).
Hypothesis | Potential benefits | Potential drawbacks |
---|---|---|
H1: There may be a role for some regulators to clarify how external audit could support the regulatory process, for example, as a means for those developing and deploying algorithms to demonstrate compliance with regulation, under conditions approved by the regulator. | Organisations gain greater certainty and clarity over how to demonstrate compliance with regulations, and greater competition in the audit market is stimulated by higher demand from those organisations. | May reduce firms’ flexibility to devise and adopt innovative approaches to audit. Further, regulators cannot always determine compliance (for example, where this is left to the courts). Thus, guidance can only make parties more likely to comply with the law. |
H2: There may be a role for some regulators in producing guidance on how third parties should conduct audits and how they should communicate their results to demonstrate compliance with our respective regimes. | Guidance that helps third parties understand what types of audit are more likely to be appropriate for demonstrating compliance could also address the requirements of multiple regimes, saving costs for audited organisations. Such guidance could also lower the barrier to entry to the algorithm auditing market by creating a level playing field. | Regulators need flexibility to be able to adapt guidance on types of auditing that are deemed ‘sufficient’, to adapt to the emergence of new harmful practices as the use of algorithms evolves. In addition, guidance may be too high level and therefore risk being misinterpreted, without sufficiently demonstrative examples. |
H3: There may be a role for some regulators in assisting standards-setting authorities to convert regulatory requirements into testable criteria for audit. | Third-party auditors, whether from industry, academia, or civil society, understand how they can support regulatory compliance. Creating testable criteria also lowers barriers to entry to auditing companies. | It may not be possible or appropriate to reduce some regulatory requirements to testable criteria. |
H4: Some regulators may have a role to provide mechanisms through which internal and external auditors, the public and civil society bodies can securely share information with regulators to create an evidence base for emerging harms. Such mechanisms could include a confidential database for voluntary information sharing with regulators. | Such mechanisms could form an important complement to formal regulation in terms of oversight of algorithms and their impacts on individuals and society. When appropriate, the information gathered by regulators could lead to the launching of more formal investigations or other actions. Regulators could also share insights from audits to the benefit of sectors understanding how algorithms can create harms. | Information may be poor quality or opaque, thus reducing the insights that may be gathered from it. |
H5: There may be a role for some regulators in accrediting organisations to carry out audits, and in some cases these organisations may certify that systems are being used in an appropriate way (for example, through a bias audit) in order to demonstrate compliance with the law to a regulator. | Accreditation of auditors reduces the need for regulatory audits and the associated costs to organisations. Greater numbers of accredited auditors can improve the trust and use of algorithmic systems. | Accreditation of auditors without attendant requirements about how transparent audits need to be, to appropriate parties or the public, risks undermining accountability for the impacts of the algorithmic system used. |
H6: For some regulators there may be a further role to play in expanding the use of regulatory sandboxes (where a regulator has power to do so) to test algorithmic systems in a controlled environment. | Where regulatory sandboxes are joined up, organisations developing and deploying algorithmic systems can test their systems and understand whether they align with regulatory requirements before they are deployed. This saves them time and limits costs of regulation in the longer term. | If regulatory sandboxes are not joined up, organisations may have to approach multiple regulators to test their systems’ compliance. |
Call for Input Questions
We would welcome views from stakeholders on the following questions:
- What are the advantages and disadvantages of each of these hypotheses?
- Which of these hypotheses would you prefer the DRCF tested and explored further?
- Are there any other actions that the DRCF should consider undertaking in the algorithmic auditing space?
The call for input will last until Wednesday 8th June and a summary of responses published in due course. Stakeholders can submit views via email at drcf.algorithms@cma.gov.uk.
-
Throughout this paper we have referred to ‘regulators’ in the general sense of regulatory institutions that act independently from Government in order to oversee activity within their mandate. We acknowledge that the differences in powers and mandates across regulatory institutions means that the DRCF approach cannot speak for all regulators. ↩
-
Ofcom is currently preparing to take on new functions under the Online Safety Bill. Further detail is set out below in Box 1. ↩
-
The DRCF was established in July 2020 to support regulatory coordination in digital markets, and cooperation on areas of mutual importance, given the unique challenges posed by regulation of online platforms. The DRCF is a non-statutory body – it is not a decision-making body and does not provide formal advice or direction to members. The DRCF members are currently the Competition and Markets Authority (CMA), Financial Conduct Authority (FCA), Information Commissioner’s Office (ICO) and Ofcom. ↩
-
ONS (2021) ‘Internet users, UK: 2020’. 6 April. ↩
-
The definition followed in this paper aligns with the common implementation-focused used of the term rather than the formal mathematical definition. See Tsamados, A et al (2022) ‘The ethics of algorithms: key problems and solutions’, AI & Society, Vol. 37, pp.215-230. ↩
-
See Kleinberg, J, Ludwig, J, Mullainathan, S, & Sunstein, CR (2020), ‘Algorithms as discrimination detectors’. In Proceedings of the National Academy of Sciences, Vol. 117, No. 48. 28 July. ↩
-
DRCF (2022) ‘The benefits and harms of algorithms: a shared perspective from the 4 digital regulators’. ↩
-
Competition and Markets Authority (2021) ‘Algorithms: How they can reduce competition and harm consumers,’ 19 January. ↩
-
Bank of England and Financial Conduct Authority (2022) ‘The AI Public-Private Forum: Final report.’ ↩
-
Office for AI, DCMS & BEIS (2021) ‘National AI Strategy’. 22 September. ↩
-
CDEI (2021) ‘Roadmap to an effective AI assurance ecosystem’. ↩
-
CDEI (2021) ‘Roadmap to an effective AI assurance ecosystem’. ↩
-
CMA (2021) ‘CMA to investigate Amazon and Google over fake reviews.’ [Press release] 25 June. ↩
-
ICO (2021) ‘ICO issues provisional view to fine Clearview AI Inc over £17 million’. 29 November. ↩
-
ICO (2017) ‘Investigation into the use of data analytics in political campaigns: A report to Parliament (PDF, 2.2 MB)’. 6 November. ↩
-
ACCC (2020) ‘Trivago misled consumers about hotel room rates.’ [Press release] 21 January. ↩
-
These and other possible regulatory technology tools have been built by companies and researchers. Regulators could choose to use them in their enforcement and monitoring activities – see Parties involved in the audit landscape section. ↩
-
Facebook (2021) ‘How we’re using Fairness Flow to help build AI that works better for everyone’, 31 March. ↩
-
IBM (2021) ‘The AI 360 Toolkit: AI models explained’, 4 February. ↩
-
See Google (2020) ‘Introducing the Model Card Toolkit for Easier Model Transparency Reporting’, Google AI Blog. ↩
-
See Google (2020) ‘Responsible AI with TensorFlow’, TensorFlow blog. ↩
-
Wall Street Journal (2021) ‘Investigation: How TikTok’s Algorithm Figures Out Your Deepest Desires’, 21 July. ↩
-
Ribeiro, Manoel Horta, et al. “Auditing radicalization pathways on YouTube.” Proceedings of the 2020 conference on fairness, accountability, and transparency. 2020. ↩
-
The FCA has an outcomes-focused, technology neutral approach to regulation and sets clear expectations around accountability for FSMA authorised firms through the Senior Managers and Certification Regime. Accountability for the outcomes of algorithmic decisions remains with the Senior Manager accountable for the relevant business activity whatever technology is deployed. For instance, where firms use ‘robo advisory’ services, the Senior Manager accountable for advice would be accountable for the advice given and the end outcomes for consumers. Senior Managers should ensure that there are adequate systems and controls around the use of an algorithm. ↩
-
Rebalancing information asymmetries is a fundamental concept associated with audit and assurance in general, particularly within financial audit. These asymmetries are exacerbated by the use of complex AI systems in decisions making. ↩
-
Financial services firms should follow the FCA’s guidance for firms on the fair treatment of vulnerable customers to ensure their needs are met. ↩
-
The FCA’s vulnerability guidance also requires compliance with the Equality Act 2010. ↩
-
DCMS (2021) ‘Data: a new direction’. 10 September. ↩
-
UK Parliament (2022) Online Safety Bill. 17th March. ↩
-
GfK (2017) ‘CMA Digital Comparison Tools (DCT) Mystery Shopping Research: Technical Report (PDF, 6.6 MB)’. September. ↩
-
CMA (2021) ‘CMA to investigate Amazon and Google over fake reviews’ [Press release] 25 June. ↩
-
ICO (2020) ‘Regulatory Sandbox Final Report: Onfido (PDF, 291 KB).’ September. ↩
-
ICO (no date) ‘Accountability Framework, ICO’. ↩
-
ICO (no date) ‘Explaining decisions made with AI, ICO’. ↩
-
MHRA (2021) ‘Software and AI as a Medical Device Change Programme’. 16 September. ↩
-
NHSx (no date) ‘The multi-agency advice service (MAAS)’. ↩
-
Ada Lovelace Institute (2022) ‘Pioneering framework for assessing the impact of medical AI set to be trialled by NHS in world-first pilot’ [Press release] 8 February. ↩
-
Note that algorithmic processing standards could in many cases be used to benchmark system outputs. ↩
-
CDEI (2021) ‘The role of technical standards in AI assurance’ in AI Assurance Guide. ↩
-
World Trade Organisation (no date) ‘Technical barriers to trade’. ↩
-
Matus, KJM & Veale, M (2021) ‘Certification systems for machine learning: Lessons from sustainability’, Regulation and Governance, Vol. 16, No. 1, pp.177-196. 9 June. ↩
-
Sandvig, C, Hamilton, K, Karahalios, K & Langbort C (2014) ‘Auditing Algorithms: Research Methods for Detecting Discrimination on Internet Platforms (PDF, 82.8 KB)’. Paper presented to “Data and Discrimination: Converting Critical Concerns into Productive Inquiry,” a preconference at the 64th Annual Meeting of the International Communication Association. May 22, 2014; Seattle, WA, USA; CDEI (no date) ‘Techniques for assuring AI systems’ in AI Assurance Guide.; Larson, J, Mattu, S, Kirchner, L & Angwin, J (2016) ‘How We Analyzed the COMPAS Recidivism Algorithm’. ProPublica. 23 May; Sadowski, C, Söderberg, E, Church, L, Sipko, M & Bacchelli, A (2018) ‘Modern Code Review: A Case Study at Google’, Proceedings of 40th International Conference on Software Engineering: Software Engineering in Practice Track, May 27-June 3. ↩
-
CMA (2021) ‘Notice of intention to accept modified commitments offered by Google in relation to its Privacy Sandbox Proposals [Case number 50972] (PDF, 2.0 MB)’. 26 November. ↩
-
ICO (no date) ‘Risks and data protection impact assessments (DPIAs)’. ↩
-
Under the UK GDPR, organisations must put in place appropriate technical and organisational measures to meet the requirements of accountability in the legislation. ↩
-
ICO & The Alan Turing Institute (no date) ’Part 2: Explaining AI in Practice’. ↩
-
In the US, NIST undertakes accuracy assessments of facial recognition systems: see [here] (https://www.nist.gov/speech-testimony/facial-recognition-technology-frt-0) ↩
-
ASA (2019) ‘Harnessing new technology to tackle irresponsible gambling ads targeted at children’. 4 April. ↩
-
CMA (2021) ‘Algorithms: how they can reduce competition and harm consumers’. ↩
-
Proposed legislation is in addition to existing laws that algorithms are subject to, such as the Consumer Protection from Unfair Trading Regulations 2008. Where a trader applies an algorithm in a misleading or otherwise unfair manner, that practice may infringe the CPRs 2008. ↩
-
DCMS & BEIS (2021) ‘A new pro-competition regime for digital markets’. 9 August. ↩
-
UK Parliament (2022) Online Safety Bill. 17th March. ↩
-
Bundeskartellamt (2021) ‘Amendment of the German Act against Restraints of Competition.’ [Press release] 19 January. ↩
-
Cyberspace Administration of China (国家互联网信息办公室) (2021) ‘Notice of the Cyberspace Administration of China on Public Solicitation of Comments on the Provisions on the Administration of Internet Information Service Algorithms Recommendation’. 31 December. ↩
-
European Commission (no date) ‘Notified bodies’. ↩
-
Metcalf, J, Smith, B & Moss, E (2022) ’A New Proposed Law Could Actually Hold Big Tech Accountable for Its Algorithms’. 9 February. ↩
-
Lee, NT & Lai, S (2021) ‘Why New York City is cracking down on AI in hiring’. Brookings Institute. 20 December. ↩
-
Government of Canada (no date) ‘Algorithmic Impact Assessment Tool.’ ↩
-
UK Government (2021) ‘[National AI Strategy](https://www.gov.uk/government/publications/national-ai-strategy’, 22 September. ↩
-
CDDO (2021) ‘Algorithmic Transparency Standard’. 29 November. ↩
-
Supreme Audit Institutions of Finland, Germany, the Netherlands, Norway and the UK (2020) ‘Auditing machine learning algorithms’. 24 November. ↩
-
IEEE (2018) ‘Ethically Aligned Design – Version II (PDF, 4.8 MB)’. ↩
-
IEEE (2021) ‘The Ethics Certification Program for Autonomous and Intelligent Systems (ECPAIS)’. ↩
-
British Standards Institute (2019) ‘BSI White Paper – Overview of standardization landscape in artificial intelligence’. ↩
-
The Board of the International Organization of Securities Commissions (2020) ‘The use of artificial intelligence and machine learning by market intermediaries and asset managers: Consultation Report’. June. ↩
-
Chowdhury, R & Williams, J (2021) ‘Introducing Twitter’s first algorithmic bias bounty challenge’. 30 July. ↩
-
Bank of England and FCA (2021) ‘Minutes of the third meeting of the Artificial Intelligence Public-Private Forum (PDF, 612 KB)’. 15 June. ↩
-
CDEI (no date) ‘Case studies’. AI Assurance Guide. ↩
-
Johnson, K (2021) ‘What algorithm auditing startups need to succeed.’ VentureBeat. 30 January. ↩
-
Bandy, J (2021) ‘Problematic Machine Behaviour: A Systematic Literature Review of Algorithm Audits (PDF, 504 KB).’ Forthcoming, Proceedings of the ACM (PACM) Human-Computer Interaction, CSCW ’21. ↩
-
Wilson, C, Ghosh, A, Jiang, S, Mislove, A, Baker, L, Szary, J, Trindel, K, Polli, F (2021) ‘Building and Auditing Fair Algorithms: A Case Study in Candidate Screening (PDF, 543 KB).’ In ACM Conference on Fairness, Accountability and Transparency (FAccT ’21), March 1-10, 2021, Virtual Event, Canada. ACM, New York, NY, USA, 12 pages. ↩
-
Saleiro, P, Kuester, B, Hinkson, L, London, J, Stevens, A, Anisfield, A, Rodolfa, KT, Ghani, R (2018) ‘Aequitas: A Bias and Fairness Audit Toolkit (PDF, 787 KB).’ Arxiv. ↩
-
Oxford Internet Institute (2021) ’AI modelling tool developed by Oxford Academics incorporated into Amazon anti-bias software’ [Press release] 21 April. ↩
-
Reisman, D, Schultz, J, Crawford, K, Whittaker M (2018) ‘Algorithmic Impact Assessments: A Practical Framework for Public Agency Accountability (PDF, 204 KB)’. AI Now Institute, New York University. ↩
-
Raji, D, Smart, A, White, N, Mitchell, M, Gebru, T, Hutchinson, B, Smith-Loud, J, Theron, D, Barnes, P (2020) ‘Closing the AI Accountability Gap: Defining an End-to-End Framework for Internal Algorithmic Auditing (PDF, 607 KB).’ In Conference on Fairness, Accountability, and Transparency (FAT* ’20), January 27-30, 2020, Barcelona, Spain. ACM, New York, NY, USA, 12 pages. ↩
-
Wall Street Journal (2019), ‘Amazon Changed Search Algorithm in Ways That Boosted Its Own Products’, 16 September. ↩
-
The Markup (no date) ‘Investigations’. ↩
-
Mattu, S, Yin, L, Waller, A, Keegan, J (2021) ‘How We Built a Facebook Inspector’, The Markup, 5 January. ↩
-
Foxglove (2020) ‘We put a stop to the A Level grading algorithm!’ 17 August. ↩
-
Taylor, C (2021) ‘Irish Council for Civil Liberties files privacy lawsuit over online ads.’ The Irish Times, 16 June. ↩
-
Lomas, N (2021) ‘Dutch court will hear another Facebook privacy lawsuit.’ Tech Crunch, 2 July. ↩
-
See Who Targets Me ↩
-
Doteveryone (no date) ‘Consequence Scanning – an agile practice for responsible innovators’. ↩
-
The Open Data Institute has taken on Doteveryone’s resources including the consequence scanning framework. See ODI (2020) ‘The ODI to take on Doteveryone’s TechTransformed resource’, 28 May. ↩
-
Ada Lovelace Institute (2021) ’Accountability of algorithmic decision-making systems’. ↩
-
Horwitz, J (2021) ‘The Facebook Whistleblower, Frances Haugen, Says She Wants to Fix the Company, Not Harm It’, Wall Street Journal, 3 October. ↩
-
Lewis, P (2018) ‘’Fiction is outperforming reality’: how YouTube’s algorithm distorts truth’, The Guardian, 2 February. ↩
-
Auditing companies will remain accountable to existing regulatory regimes, such as misleading claims under consumer law. ↩
-
ICO (2021) ‘Blog: New toolkit launched to help organisations using AI to process personal data understand the associated risks and ways of complying with data protection law.’ 20 July. ↩
-
Medicines & Healthcare product Regulatory Agency (2021) ‘Software and AI as a Medical Device Change Programme.’ 16 September. ↩
-
ITU & WHO (no date) ‘Benchmarking health AI models’. ↩
-
Büthe, T & Mattli, W (2013) ‘The New Global Rulers: The Privatization of Regulation in the World Economy’. Princeton University Press. ↩
-
Veale, M & Borgesius, FZ (2021) ‘Demystifying the Draft EU Artificial Intelligence Act’ Computer Law Review International. 6 July. ↩
-
Financial Times (2022) ‘EU to outline tech standards plan to counter China influence’. 2 February. ↩
-
TikTok (2020), ‘Fair competition and transparency benefits us all’29 July. ↩
-
Where companies fail to disclose such information, and that failure affects a consumer’s transactional decision, for example their decision simply to use that platform over another, may lead to that trade infringing the Consumer Protection from Unfair Trading Regulations 2008. ↩
-
Tsamados, A et al (2021) ‘The ethics of algorithms: key problems and solutions’, AI and Society. 20 February. ↩
-
CMA (2021) ‘Algorithms: How they can reduce competition and harm consumers’ ↩
-
Royal Society (2019), Protecting privacy in practice: The current use, development and limits of Privacy Enhancing Technologies in data analysis. ↩
-
CDEI (2021), Privacy enhancing technologies: adoption guide. ↩
-
Web scraping is likely to trigger a requirement to complete a Data Protection Impact Assessment under the GDPR. ↩
-
Sellars, A (2020) ‘Facebook’s threat to the NYU Ad Observatory is an attack on ethical research’. 29 October. ↩
-
Bond, S (2021) ‘NYU Researchers Were Studying Disinformation On Facebook. The Company Cut Them Off.’ 4 August. ↩
-
The research provisions make reference to 3 types of research activity: (1) archiving purposes in the public interest; (2) scientific or historical research purposes; and (3) statistical purposes. Scientific or historical research can be understood broadly and includes research carried out in traditional academic settings, as well as academic research in arts, social sciences and humanities disciplines. It can also include research carried out in commercial settings, and technological development. ↩
-
ICO (2022) ‘ICO consultation on draft guidance for the research provisions within the UK GDPR and DPA 2018’. 16 February. ↩
-
ICO (no date) ‘What common issues might come up in practice?, ICO’. ↩
-
CMA (2020) ‘Online Platforms and Digital Advertising Market Study, Appendix U: supporting evidence for the code of conduct’ (PDF, 1,363 KB) – paras. 160-165. ↩
-
CMA (2021) ‘Algorithms: How they can reduce competition and harm consumers’. ↩
-
ICO and Alan Turing Institute, Explaining decisions made with Artificial Intelligence. ↩
-
DRCF (2022) ‘The benefits and harms of algorithms: a shared perspective from the 4 digital regulators’ ↩
-
EDRi (2021) ‘An EU Artificial Intelligence Act for Fundamental Rights: A Civil Society Statement’ (PDF, 297KB). 30 November. ↩
-
Institute for the Future of Work (2020), ’Mind the gap: How to fill the equality and AI accountability gap in an automated world’. 26 October. ↩
-
Ada Lovelace Institute & DataKind UK (2020), ‘Examining the Black Box: Tools for Assessing Algorithmic Systems’. ↩
-
Binns, R (2018) ‘Fairness in Machine Learning: Lessons from Political Philosophy’ in Proceedings of the 1st Conference on Fairness, Accountability and Transparency, PMLR 81:149-159. ↩
-
CDEI (2020) ‘Review into bias in algorithmic decision-making (PDF, 9.9 MB)’. November. ↩
-
Mehrabi, N et al (2022) ‘A Survey on Bias and Fairness in Machine Learning’. Arxiv. ↩
-
In addition, there are often more statistical definitions of fairness within these broad categories, some of which can be mutually exclusive. ↩
-
Hellman, D (2020) ‘Measuring Algorithmic Fairness’, Virginia Law Review, Vol. 106, No. 4, pp.811-866. ↩
-
Engler, A (2021) ‘Independent auditors are struggling to hold AI companies accountable’. Fast Company, 26 January. ↩
-
Floridi, L (2021) ‘Translating Principles into Practices of Digital Ethics: Five Risks of Being Unethical.’ Ethics Governance, and Policies in Artificial Intelligence, pp. 81-90. ↩
-
The Four-Fifths Rule is a guideline promoted by the Equal Opportunity Employment Commission in the United States. The rule states that if a hiring process selects a particular group at a rate that is less than 80 percent of the group with the highest selection rate, then the process has an adverse impact. ↩
-
Engler, A (2021) ‘Auditing employment algorithms for discrimination.’ Brookings Institute Report. 12 March. ↩
-
MHRA (2020) ‘Medical devices: conformity assessment and the UKCA mark’. 31 December. ↩
-
See ‘Ongoing algorithmic monitoring’ in CMA (2021) ‘Algorithms: How they can reduce competition and harm consumers’. 19 January. ↩
-
CMA (2021) ‘CMA secures improved commitments on Google’s Privacy Sandbox’. 26 November. ↩
-
ICO (no date) ‘Data protection impact assessments’. ↩
-
The majority of organisations using AI systems processing personal data will need to submit a Data Protection Impact Assessment. ↩
-
Bug bounties are used in cybersecurity to identify security flaws. They provide financial rewards to members of the public to identify and notify companies of vulnerabilities they find in their code. As mentioned in section 4.1.3, in 2021 Twitter introduced its algorithmic bias bounty challenge to get help from the machine learning community to identify bias in its saliency algorithm (also known as its image cropping algorithm). See ‘Introducing Twitter’s first algorithmic bias bounty challenge’. ↩
-
CDEI (2021) ‘The roadmap to an effective AI assurance ecosystem – extended version’. 8 December. ↩
-
CMA (2021) ‘Algorithms: how they can reduce competition and harm consumers’. ↩
-
Raji, D (2021) ‘Radical Proposal: Third-Party Auditor Access for AI Accountability’. Stanford University Human-Centred Artificial Intelligence. 20 October. ↩
-
Narayanan, A (2021) ‘How to recognize AI snake oil (PDF, 1,141 KB)’. ↩
-
Dressel, J & Farid, H (2018) ‘The accuracy, fairness, and limits of predicting recidivism’. Science Advances, Vol. 4, Issue 1. ↩
-
BMJ (2020) ‘Concerns over “exaggerated” study claims of AI outperforming doctors’. 25 March. ↩
-
KPMG (2020) ‘The time has come: The KPMG Survey of Sustainability Reporting 2020 (PDF, 11.2 MB)’. December. ↩
-
Swiss Digital Initiative (no date) ‘The Digital Trust Label’. ↩
-
National Cyber Security Centre (no date) ‘About Cyber Essentials’. ↩
-
European Commission (no date) ‘CE marking’. ↩
-
Clark, J & Hadfield, G (2019) ‘Regulatory Markets for AI Safety (PDF, 774 KB)’. Arxiv. ↩
-
The ‘Big Four’ refers to the leading global accounting firms: Deloitte, PwC, Ernst & Young and KPMG. ↩