Using artificial intelligence (AI) to help radiologists with CT scans
Learn how ACE helped radiologists within the NHS find a solution to diagnose and improve patient outcomes using artificial intelligence.
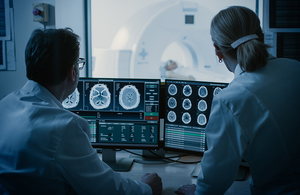
Image from Shutterstock.
Introduction
We worked with the NHS to explore how artificial intelligence (AI) could help radiologists quickly compare and assess computerised tomography (CT) scans to enable quicker diagnoses and improve patient outcomes.
The challenge
Radiologists at George Eliot Hospital NHS Trust, which serves more than 300,000 people across Warwickshire, Leicestershire and Coventry, perform around 60 scans each day.
Many of these are related to cancer, and in most cases a comparison with a previous scan is necessary to assess lesion growth or shape changes. This manual alignment and comparison is labour intensive, but no suitable automation tools exist.
Automating this process and improving alignment and overlay of scans would enable slight changes in volume or new lesions to be picked up more quickly. This includes a report guiding radiologists to review or further evaluate particular regions.
Increased accuracy would improve patient safety and outcomes by allowing faster diagnoses, and successful automation would also save radiologists’ time.
The approach
Roke was the supplier selected from the Accelerated Capability Environment’s (ACE) Vivace industry and academia community for this commission and delivered a proof of concept (POC) in just 12 weeks.
The POC uses AI to automatically overlay images, compensating for factors including movement and breathing as well as body composition changes frequently found in patients. The tool calculates potential anomalies in three-dimensions, allowing volume changes in lesions, or new lesions, to be quickly identified.
It was trained on anonymised CT scan data from 100 patients supplied by the hospital which also included machine data on body part positioning and the angle of scan.
Roke developed the graphic user interface (GUI) visualisation tool by breaking the problem down into 3 key areas covering alignment, tissue sectioning, and anomaly detection, which Roke termed the ‘Holy Grail’.
The impact
As part of validation testing, lesions were successfully detected in 7 out of 9 patients, and in 10 out of 17 images.
Roke also developed a masked data deep learning approach, trained on human body composition, which fills in what it thinks should make up any given area. This can then be compared to the actual scan, with the difference between prediction and reality helping identify a lesion and score the degree of anomaly.