Background information: Fraud and error in the benefit system statistics, 2021 to 2022 estimates
Published 26 May 2022
Applies to England, Scotland and Wales
Purpose of the statistics
Context and purpose of the statistics
This document supports our main publication which contains estimates of the level of fraud and error in the benefit system in Financial Year Ending (FYE) 2021.
We measure fraud and error so we can understand the levels, trends and reasons behind it. This understanding supports decision making on what actions DWP can take to reduce the level of fraud and error in the benefit system. The National Audit Office takes account of the amount of fraud and error when they audit DWP’s accounts each year.
Within DWP these statistics are used to evaluate, develop and support fraud and error policy, strategy and operational decisions, initiatives, options and business plans through understanding the causes of fraud and error.
The fraud and error statistics published in May each year feed into the DWP accounts. The FYE 2022 estimates published in May 2022 feed into the FYE 2022 DWP annual report and accounts.
The statistics are also used within the annual HM Revenue and Customs (HMRC) National Insurance Fund accounts. These are available in the National Insurance Fund Accounts section of the HMRC reports page.
The fraud and error estimates are also used to answer Parliamentary Questions and Freedom of Information requests, and to inform DWP Press Office statements on fraud and error.
Limitations of the statistics
The estimates do not include reviews of every benefit each year. After a pause in reviews for most benefits in FYE 2021 due to the coronavirus (COVID-19) pandemic, we have reviewed a range of benefits for FYE 2022. We were unable to measure Personal Independence Payment for FYE 2022.
This document includes further information on limitations – for example:
- on benefits reviewed and changes this year (sections 1 and 2)
- omissions to the estimates (section 3)
- our sampling approach (section 4)
- how we adjust and calculate estimates for individual benefits (section 5)
- how we bring these together to arrive at estimates of the overall levels of fraud and error (section 6)
Longer time series comparisons may not be possible for some levels of reporting due to methodology changes. Our main publication and accompanying tables indicate when comparisons should not be made.
We are unable to provide information on individual fraud and error cases or provide sub-national estimates of fraud and error as we are unable to break the statistics down to this level.
Comparisons between the statistics
These statistics relate to the levels of fraud and error in the benefit System in Great Britain.
Social Security Scotland report the levels of fraud and error for benefit expenditure devolved to the Scottish Government within their annual report and accounts.
Northern Ireland fraud and error statistics are comparable to the Great Britain statistics within this report, as their approach to collecting the measurement survey data, and calculating the estimates and confidence intervals, is very similar. Northern Ireland fraud and error in the benefit system high level statistics are published within the Department for Communities annual reports.
HM Revenue and Customs produce statistics on error and fraud in Tax Credits.
When comparing different time periods within our publication, we recommend comparing percentage rates of fraud and error rather than monetary amounts. This is because the amount of fraud and error in pounds could go up, even if the percentage rate of fraud and error stays the same (or goes down even), if the amount of benefit we pay out in total goes up compared to the previous year.
Source of the statistics
We take a sample of benefit claims from our administrative systems. DWP’s Performance Measurement team contact the benefit claimants to arrange a review. The outcomes of these reviews are recorded on a bespoke internal database called FREDA. We use data from here to produce our estimates.
We also use other data to inform our estimates – for example:
- Benefit expenditure data (aligning with the Spring Budget published forecasts)
- Benefit recovery data (DWP benefits and Housing Benefit) to allow us to calculate estimates of net loss
- Other DWP data sources and models to improve the robustness of, or categorisations within, our estimates – for example, to allow us to see if claimants who leave benefit as a consequence of the fraud and error review process then return to benefit shortly afterwards, and to understand the knock-on effect of fraud and error on disability benefits on other benefits
Further information on the data we use to produce our estimates is contained within sections 4, 5 and 6 of this report.
Definitions and terminology within the statistics
The main publication presents estimates of Fraud, Claimant Error and Official Error. The definitions for these are as follows:
- Fraud: This includes all cases where the following three conditions apply:
- the conditions for receipt of benefit, or the rate of benefit in payment, are not being met
- the claimant can reasonably be expected to be aware of the effect on entitlement
- benefit stops or reduces as a result of the review
- Claimant Error: The claimant has provided inaccurate or incomplete information, failed to report a change in their circumstances, or failed to provide requested evidence, but there is no fraudulent intent on the claimant’s part
- Official Error: Benefit has been paid incorrectly due to inaction, delay or a mistaken assessment by the DWP, a Local Authority or Her Majesty’s Revenue and Customs to which no one outside of that department has materially contributed, regardless of whether the business unit has processed the information
We report overpayments (where we have paid people too much money), and underpayments (where we have not paid people enough money).
We present these in percentage terms (of expenditure on a benefit) and in monetary terms, in millions of pounds.
We also report the percentage of cases with fraud or an overpayment error, and the percentage of cases with an underpayment error.
Further information about the types of errors we report on, abbreviations commonly used and statistical methodology can be found in the appendices at the end of this document.
Revisions to the statistics
Revisions to our statistics may happen for a number of reasons. When we make methodology changes that impact our estimates, we may revise the estimates for the previous year to allow meaningful comparisons between the two. Further details of any changes will be provided in section 2 of this report. Where we introduce major changes, we may denote a break in our time series and recommend that comparisons are not made back beyond a certain point.
In this year’s publication we are making a revision related to Housing Benefit (HB) expenditure. Previously HB expenditure data included Universal Credit (UC) as a non-passporting benefit. The expenditure data has been adjusted to categorise UC as a passporting benefit because people in receipt of UC who are in supported, sheltered or temporary housing, are treated similarly to those claimants in receipt of other income-related benefits. The need for this change has become apparent as there are now more claimants receiving HB due to being in receipt of UC. We are revising the estimates from FYE 2020 and FYE 2021 for HB as a result of this. We preannounced our plans to make this change in a Statistical Notice.
There are also some minor revisions to ESA and PC figures for these two years, due to a minor change to the extrapolation process and a change to the assigning of error reasons.
See section 2 for more details on these changes.
The National Statistics Code of Practice allows for revisions of figures under controlled circumstances: “Statistics are by their nature subject to error and uncertainty. Initial estimates are often systematically amended to reflect more complete information. Improvements in methodologies and systems can help to make revised series more accurate and more useful”.
Unplanned revisions of figures in reports in this series might be necessary from time to time. Under this Code of Practice, the Department has a responsibility to ensure that any revisions to existing statistics are robust and are freely available, with the same level of supporting information as new statistics.
Status of the statistics
National statistics
National Statistics status means that our statistics meet the highest standards of trustworthiness, quality and public value, and it is our responsibility to maintain compliance with these standards.
The continued designation of these statistics as National Statistics was confirmed in December 2017 following a compliance check by the Office for Statistics Regulation. The statistics last underwent a full assessment against the Code of Practice for Statistics in February 2012. Since the latest review by the Office for Statistics Regulation, we have continued to comply with the Code of Practice for Statistics, and have made the following improvements:
- we conducted a user consultation on the frequency of the publication, the benefits measured and on the breakdowns used within the publication. This has resulted in us changing from a bi-annual to an annual publication, and beginning to measure fraud and error on benefits that have not been measured at all or for a long time
- we have made some methodological changes, resulting in better understood methodologies and assumptions, improved accuracy of the fraud and error statistics, and more consistency across benefits
- we have made a number of changes to improve the relevance and accessibility of our statistics. For example, we have: moved away from using the same wording and charts for all the benefits in our publication to instead focus on the key messages for each benefit, updated the categories of error we report in our publication based on user needs, and made the data from our publication available to analysts within DWP to conduct their own analysis. We have also created an online tool to allow users to explore our fraud and error statistics
- we have produced the documents in HTML format and provided an accessible version of the reference tables
Read further information about National Statistics on the UK Statistics Authority website.
Quality Statement
Quality in statistics is a measure of their ‘fitness for purpose’. The European Statistics System Dimensions of Quality provide a framework in which statisticians can assess the quality of their statistical outputs. These dimensions of quality are of relevance, accuracy and reliability, timeliness, accessibility and clarity, and comparability and coherence.
Our Background Quality Report gives more information on the application of these quality dimensions to our Fraud and Error statistics.
Feedback
We welcome any feedback on our publication. You can contact us at: caxtonhouse.femaenquiries@dwp.gov.uk
Lead Statistician: John Bilverstone
DWP Press Office: 020 3267 5144
Report Benefit Fraud: 0800 854 4400
Useful links
Landing page for the fraud and error statistics.
FYE 2022 estimates, including published tables.
1. Introduction to our measurement system
The main statistical release and supporting tables and charts provide estimates of fraud and error for benefit expenditure administered by the Department for Work and Pensions (DWP). This includes a range of benefits for which we derive estimates using different methods, as detailed below. For further details on which benefits are included in the total fraud and error estimates please see Appendix 2. More information can be found online about the benefit system and how DWP benefits are administered.
The Fraud and Error estimates provide estimates for the amount overpaid or underpaid in total and by benefit, broken down into the types of Fraud, Claimant Error and Official Error, for benefits reviewed this year. For FYE 2022 Universal Credit (UC), Housing Benefit (HB), Employment and Support Allowance (ESA), Pension Credit (PC), State Pension (SP) and Attendance Allowance (AA) had a full Fraud and Error review. The coronavirus pandemic had a significant impact on our measurement of Personal Independence Payment (PIP). For more details see section 2.
Estimates of Fraud and Error for each benefit have been derived using three different methods, depending on the frequency of their review:
Benefits reviewed this year
Fraud, Claimant Error and Official Error (see definitions above) have been measured for FYE 2022 for UC, HB, ESA, PC, SP and AA.
In previous years SP was only measured for Official Error; this year it has had a full review.
The statistical consultation carried out in Summer 2018 included a question on which benefits we should measure in future years. Further information can be found in the consultation.
AA has been measured for the first time in FYE 2022 in response to the user consultation.
Expenditure on measured benefits accounted for 77% of all benefit expenditure in FYE 2022.
Estimates are produced by statistical analysis of data collected through annual survey exercises, in which independent specially trained staff from the Department’s Performance Measurement (PM) team review a randomly selected sample of cases for benefits reviewed this year. See section 4 for more information on the sampling process.
The review process involves the following activity:
-
Previewing the case by collating information from a variety of DWP or Local Authority (LA) systems to develop an initial picture and to identify any discrepancies between information from different sources.
-
Interviewing the claimant (or a nominated individual where the claimant lacks capacity) using a structured and detailed set of questions about the basis of their claim. The interview is completed as a telephone review in the majority of cases. However, where this is not appropriate, there is usually also the option for a completed review form to be returned by post.
-
The interview aims to identify any discrepancies between the claimant’s current circumstances and the circumstances upon which their benefit claim was based.
If a suspicion of Fraud is identified, an investigation is undertaken by a trained Fraud Investigator with the aim of resolving the suspicion.
Benefits were measured with different sample periods, although all were contained within the period October 2020 to November 2021. For more information on the sample period for individual benefits please see Annex 1 of the statistical report.
The following number of benefit claims were sampled and reviewed by the PM team:
Benefit | Sample size | Percentage of claimant population reviewed |
---|---|---|
Universal Credit | 2,995 | 0.07% |
State Pension | 1,499 | 0.01% |
Housing Benefit | 2,738 | 0.10% |
Pension Credit | 1,999 | 0.14% |
Employment and Support Allowance | 1,999 | 0.12% |
Attendance Allowance | 1,610 | 0.12% |
Total | 12,840 | 0.06% |
Overall, approximately 0.06% of all benefit claims in payment were sampled and reviewed by the PM team.
Information about the Performance Measurement Team can be found online.
Benefits reviewed previously
Since 1995, the Department has carried out reviews for various benefits to estimate the level of Fraud and Error in a particular financial year following the same process outlined above. In FYE 2022 around 20% of total expenditure related to benefits reviewed in previous years. Please see Appendix 2 for details of benefits reviewed previously.
Benefits never reviewed
The remaining benefits, which account for around 3% of total benefit expenditure, have never been subject to a specific review. These benefits tend to have relatively low expenditure which means it is not cost effective to undertake a review. For these benefits the estimates are based on assumptions about the likely level of Fraud and Error (for more information please see section 6).
2. Changes to the statistics this year
This section provides detail of changes for the FYE 2022 publication. Any historical changes can be found in previous years’ publications.
Changes to benefits reviewed
The coronavirus outbreak resulted in our normal measurements being suspended or changed in FYE 2021, and UC was the only benefit to be fully measured alongside SP Official Error measurement.
For FYE 2022 we have restarted the measurement of ESA, PC and HB. Also, SP has had a full review for the first time since FYE 2006 and AA has been measured for the first time.
The measurement of PIP has been affected again this year by the Covid-19 pandemic and has not been measured.
Move to telephone reviews
The pandemic has driven a move to complete almost all reviews by telephone rather than face to face home visits. This could potentially impact the estimates at the error reason level.
For example, an increase in abroad errors could be due to reviewing officers identifying a foreign dialling tone when carrying out a telephone review, which previously would not have been identified if the claimant instead chose not to partake in a face-to-face interview. Similarly, when conducting a telephone review, it is more difficult to identify Housing Benefit claimants not in residence at the declared address – previously a visiting officer would be present to identify this fraudulent activity.
We are assuming that there is no impact on total Fraud and Error estimates or at the error type levels (Fraud, Claimant Error and Official Error). Therefore, this change does not affect the comparability of our statistics at these levels.
Housing Benefit coverage
Housing Benefit is split into groups and cases are sampled and reviewed based on these groups. Estimates for Housing Benefit split by age group (working age and pension age), are published alongside the total HB estimates. These two groups are made up of “passported” cases and “non-passported” cases. Passported claims get Housing Benefit because they receive another qualifying income-related benefit: Employment and Support Allowance, Income Support, Jobseeker’s Allowance, Pension Credit, or Universal Credit (for Universal Credit only if they are in supported, sheltered or temporary housing). Non-passported claims get Housing Benefit without one of these qualifying benefits. This means that HB is made up of four groups in total: passported working age, non-passported working age, passported pension age and non-passported pension age. These groups combine to produce the total HB estimates.
Prior to FYE 2020, all four of these groups were measured on a continuous basis. In FYE 2020, only two of the groups were reviewed: non-passported working age and passported pension age. In FYE 2021, none of the groups were reviewed due to the coronavirus pandemic. In FYE 2022, only non-passported working age claims were reviewed. This means that in FYE 2022, the non-passported working age estimates relate to reviews undertaken in FYE 2022, while the passported pension age estimates relate to reviews undertaken in FYE 2020, and the estimates for the remaining groups (passported working age and non-passported pension age) relate to reviews undertaken in FYE 2019. The rates of Fraud and Error found when each group was last reviewed were applied to the FYE 2022 expenditure, to calculate the total HB estimate for FYE 2022.
Change to the Housing Benefit expenditure data used in the estimates
In order to calculate the monetary value of Fraud and Error and the proportion of expenditure overpaid or underpaid we use DWP expenditure figures. Within these figures any case that was getting Housing Benefit and Universal Credit was classed as a non-passported Housing Benefit case. Although not strictly a passporting benefit, those getting Universal Credit and Housing Benefit are treated in a similar way to passported cases (if they are entitled to any Universal Credit their Housing Benefit is paid in full). Due to this we have made the change to the expenditure on Housing Benefit to classify these cases as passported and revised the last year’s figures.
Since there is less opportunity for Fraud and Error to occur on passported cases, this change has the impact of reducing the total Fraud and Error on Housing Benefit.
Due to the roll out of Universal Credit and the jump in Universal Credit claims because of COVID-19, the impact of this change increased over time. In FYE 2020, this change reduces the Housing Benefit total overpayments figure by 0.3 percentage points and underpayments by 0.1 percentage points. In FYE 2021 these figures reduced by 0.5 percentage points and 0.2 percentage points respectively. This means that the trend over the last two years is now decreasing rather than being stable. Prior to FYE 2020, we think the impact on the Housing Benefit total figures would have been negligible and would not impact on the trend.
Adopting this change also resulted in a small change in overpayments at a global (overall) level in FYE 2021. In particular, reducing Fraud overpayments from 3.0% to 2.9%.
Change to the Local Authorities reviewed for Housing Benefit
Some Local Authorities were not reviewed in FYE 2022. This was due to timing issues related to the restarting of reviews, following the pause due to the coronavirus pandemic. Around 10% of Local Authorities were not reviewed. The omission of these Local Authorities had a negligible effect on the HB estimates, so no adjustment has been made to account for this.
Change to use end awards on all Housing Benefit cases to capture the effect of multiple errors more accurately
In FYE 2020, we made the change to add ‘end awards’ to Housing Benefit cases that had a whole award overpayment and an underpayment. ‘End awards’ are the amount of award a claimant is entitled to after Performance Measurement have reviewed their case. We made the change because we couldn’t be sure how much (if any) of the underpayment should be netted off, as the overpayment was capped at the award value and could be much higher (if the award was higher). However, we noticed other scenarios on Housing Benefit where the end award is needed to correctly identify the scale of the error on a case, specifically when a case has multiple errors. If a case has multiple errors, there could be an overlap between the errors that mean either the total impact is less than the impact of all the errors netted off each other or in certain circumstances more. Due to this we now have end awards on all Housing Benefit cases and adjust the error values, where there is a difference between the netted error amount and the award difference.
The impact of making this change is small; regarding FYE 2020 figures, it changes overpayment and underpayment totals by less than £10 million. We have revised the FYE 2020 Housing Benefit figures using this change to allow a valid comparison
Removal of additional extrapolation factors for ESA, PC and HB
Previously, there was an extra extrapolation figure created for Housing Benefit, Pension Credit and Employment and Support Allowance. This figure considered cases that: were referred for a fraud investigation (called High Suspicion Tracking (HST) cases); Official Errors where the evidence DWP held to determine the award amount could not be found (called deemed error cases); and the estimated proportion of the benefit caseload that was not contained within our samples (called the systematic undercount). We have now removed these extra extrapolation scaling factors because:
-
for all benefits we now get estimated outcomes for all HST cases that are outstanding, so no adjustment is needed
-
deemed errors cases are not included in the derivation of the Official Error rate (i.e. when the Official Error rate is calculated we do not include the value of the error in the numerator or that claim’s award in the denominator)
-
the systematic undercount part of this adjustment was based on flows data which has not been updated since 2014/15. Since Universal Credit’s introduction, the flows data on HB and ESA will have drastically changed therefore we no longer feel any adjustment based on this would be appropriate
The change only impact overpayments as the factor was not applied to underpayments.
Making the change to FYE 2020 figures:
-
Housing Benefit overpayments increase by about £30 million
-
Employment Support Allowance overpayments by about £30 million
-
Pension Credit overpayments decrease by less than £10 million
We have revised the FYE 2020 figures for these benefits using this change to allow a valid comparison
Change to how we attribute the amount overpaid or underpaid to error reasons on Housing Benefit, Employment and Support Allowance and Pension Credit, to align with Universal Credit and State Pension
This change has no effect on the amount overpaid or underpaid at a total level or an error type level (i.e. Fraud, Official Error, Claimant Error). We have introduced a new error reason category “Failure to provide evidence/fully engage in the process”. This is applied to cases where the claimant did not fully engage in the process and where we are unsure as to the reason why. These cases had given up their benefit entitlement rather than engage in the benefit review process, therefore we assume that the claim was fraudulent. This change was made last year to Universal Credit and State Pension figures, which were the only benefits reviewed due to the Coronavirus pandemic, and this year’s change is to ensure consistency across benefits.
Changes to the Error Code Framework
Each year we review the error code framework, which maps errors onto our publication reason categories. This year we are introducing some new categories to ensure that SP and AA main causes of loss can be highlighted. Two of these new categories also impact on other benefits as well as SP and AA, these are Hospitalised/Registered Care home and Contributions, which were previously within our “other” publication category.
We have also removed the Non-Dependents deduction category from our breakdown. This is because, although it was possible for this error to occur on benefits that are not HB, it would be classified within Housing Costs so couldn’t be split out. Therefore, for consistency we have moved Non Dependents deduction into Housing Costs on HB.
Making this change does not impact the total Fraud and Error on the benefits, however some of the monetary value may move into different error reasons.
We have revised the FYE 2020 error reason figures (and the FYE 2021 error reason figures for UC) to allow a valid comparison
Change to how we calculate the additional Fraud and Error on State Pension overseas cases
Working for a certain period in the UK means that individuals are entitled to a UK State Pension from State Pension age, even if they subsequently move outside of the UK (before or after reaching State Pension age).
The International Pension Centre collects information on deaths of overseas State Pension claimants, but do not consistently collect any information on other changes of circumstance on these cases. This means that it is only possible to measure Fraud and Error overpayments relating to non-notification, or late notification, of death.
We see very low rates of Fraud and Claimant Error on the GB caseload and therefore it is reasonable to assume that, aside from non or late notification of death, we would find equally low rates for claimants of State Pension living abroad
Two methods are used by DWP to confirm that overseas SP claimants are still alive and entitled to the benefit. These are as follows:
Life Certificates
A life certificate (LC) is a paper-based form that should be completed by the claimant, signed by a witness and then returned by the claimant. If the completed form is not returned after 16 weeks, then the claimant’s benefit is suspended and another LC is sent out, then following a further 16 weeks with no response, the claimant’s benefit is terminated. If the LC is returned by the claimant, then their benefit entitlement continues (subject to any changes in rate due to changes of circumstance reported by the claimant).
The LC exercises are split by country, and by age group where countries have larger caseloads. Countries (and age groups) are not covered more than once in a two-year period.
The number of LCs issued is recorded as is the number of claims terminated. However, for some countries (and age groups) data on the number of cases terminated are incomplete.
LCs were paused due to COVID, from January 2020 and resumed in November 2021, meaning that, for the financial year we are looking at, no life certificate process was running and no cases were terminated unless the Department was directly contacted outside of the LC process. Since restarting, the time between LC exercises for each country has been reduced to 18 months.
Death Exchange
The DWP exchanges death data with Spain, New Zealand, Australia, Republic of Ireland, Germany, Netherlands, Malta and Poland. Most of this data is received monthly. COVID had no impact on the DWP receiving death exchange data. The process for death exchanges begins with these countries requesting lists of claimants living in their country and receiving a UK State Pension. Following this, they send the death data for the DWP to process.
Previous Methodology
In 2006, an estimated amount of SP overpaid was calculated using data from the January 2004 life certification exercise.
Currently all abroad SP claimants that are not residing in countries covered by the death exchange data are part of the LC exercise. However, in January 2004 the death data exchange was not in place and the LC exercise was conducted by selecting a sample of customers using the last number of their NINO. Because the last digit of a NINO can be treated as being allocated randomly, the sample was regarded as having been selected randomly.
The LC exercise was treated as a “snapshot” for the week that the life certificates were sent out. All cases where SP had either been stopped because the customer had died prior to the life certificate exercise, or where SP had been suspended, had their amount of benefit they were receiving in that week scaled up to obtain an annual figure. A scaling factor was then applied to bring the number in the LC exercise to the number of SP cases abroad.
The results were then presented as the proportion of expenditure overpaid using expenditure figures for FYE 2004. This rate was then rolled forward and applied to each year’s SP expenditure.
New Methodology
As State Pension has been fully reviewed this year for the first time since FYE 2006, we have recalculated this additional amount of Fraud and Claimant Error on State Pension overseas cases.
We have calculated the amount of Overseas State Pension error by:
-
using the latest number of LC terminations for each country that we have data available in - 2017, 2018 and 2019
-
we have used the average weekly award from the financial year we are measuring (FYE 2022), paid to claimants residing abroad in each country that we have LC data for in 2017, 2018 and 2019
-
we multiplied the number of LCs that were terminated by the average award by 52 for each country, to get a yearly estimate for each country (1)
-
we multiplied the number of LCs that were issued by the average award by 52 for each country, to get a yearly estimate for each country which we then summed to give a final total (2)
-
we obtained the amount of SP expenditure for claimants living abroad in countries served by LCs (3)
-
we calculated an expenditure scaling figure (figure 3 divided by figure 2)
-
we applied this to figure 1 to give a final estimation
Assumptions / Omissions of this analysis
-
for some countries (and age groups) there is no termination information and data are incomplete. As a result, we are assuming, that the rate in these countries is the same as the known rate
-
we are not able to split overpayments due to non-notification (or late notification) of death down into Fraud or Claimant Error. We have therefore applied the proportional split found on GB cases. This means we have classed all overpayments as Claimant Error
-
we have assumed that the rate of Fraud and Claimant error has not been impacted by the Coronavirus pandemic
-
we have assumed that there is no Fraud or Claimant Error on Death Exchange cases
Reporting of three error types for the first time on State Pension
This year there are three error types reported for the first time on State Pension:
-
Home Responsibilities protection – we have continually measured Official Error on State Pension, however this did not involve contact with customers as part of the benefit review. When contacting claimants this year as part of the full review we have identified an area of Official Error that we were not capturing in previous years. This related to Home Responsibilities Protection. For people reaching State Pension age before 6 April 2010, Home Responsibilities Protection (HRP) reduced the number of qualifying years needed for a basic State Pension where someone stayed at home to care for children for whom they received Child Benefit or a person who was sick or disabled. For people reaching SP age since 6 April 2010, previously recorded periods of HRP were converted into National Insurance credits
-
State 2nd Pension – this enabled low and moderate earners, some carers and long term disabled or ill to build up a more generous additional pension by treating them as if they had earnings at the low earnings threshold if they were entitled to certain benefits. Official Errors have been identified where customers have been in receipt of qualifying benefits but the “State 2nd Pension liability” has not been recorded, resulting in an underpayment
-
Uprating Errors – these are tiny individual errors caused by how the Pension Strategy Computer System (PSCS) uprates the Graduated Retirement Benefit component of State Pension. These errors, in most cases less than 2p per week, have been part of the uprating process for a number of decades but we are including these for the first time in response to a recommendation from the National Audit Office. There are a large volume of these errors with a small impact on the monetary value. These errors are included in the monetary value of Fraud and Error but excluded from the proportion of cases with an error (‘incorrectness’). We have taken a de minimis approach to these Official Errors (removal of all errors below 10p), which means they have been excluded from our reporting on the number of incorrect cases, given this would create a misleading impression of the number of pensions with an incorrect award. These errors affected 16.6% (14.6%, 18.5%) of cases for overpayments and 23.3% (21.1%, 25.5%) of cases for underpayments
The exclusion of Uprating Errors from the incorrectness figures this year is something that has only been done for State Pension. We will be reviewing whether we should take a de minimis approach (removal of cases with a low monetary value) across other benefits in our assessment of incorrectness for future publications.
3. Interpretation of the results
Care is required when interpreting the results presented in the main report:
-
the estimates are based on a random sample of the total benefit caseload and are therefore subject to statistical uncertainties. This uncertainty is quantified by the estimation of 95% confidence intervals surrounding the estimate. These 95% confidence intervals show the range within which we would expect the true value of Fraud and Error to lie
-
when comparing two estimates, users should take into account the confidence intervals surrounding each of the estimates. The calculation to determine whether the results are significantly different from each other is complicated and takes into account the width of the confidence intervals. We perform this robust calculation in our methodology and state in the report whether any differences between years are significant or not
-
unless specifically stated within the commentary in the publication or in the accompanying tables, none of the changes over time for benefits reviewed this year are statistically significant at a 95% level of confidence
As well as sampling variation, there are many factors that may also impact on the reported levels of Fraud and Error and the time series presented.
-
these estimates are subject to statistical sampling uncertainties. All estimates are based on reviews of random samples drawn from the benefit caseloads. In any survey sampling exercise, the estimates derived from the sample may differ from what we would see if we examined the whole caseload. Further uncertainties occur due to the assumptions that have had to be made to account for incomplete or imperfect data or using older measurements
-
a proportion of expenditure for benefits reviewed this year cannot be captured by the sampling process. This is mainly because of the delay between sample selection and the interview of the claimant, and also the time taken to process new benefit claims, which excludes the newest cases from the review. The estimates in the tables in this release have been extrapolated to account for the newest benefit claims which are missed in the benefit reviews and cover all expenditure
-
the estimates do not encompass all Fraud and Error. This is because Fraud is, by its nature, a covert activity, and some suspicions of Fraud on the sample cases cannot be proven. For example, cash in hand earnings are harder to detect than those that get paid via PAYE. Complex official error can also be difficult to identify. More information on omissions can be found later in this section
-
some incorrect payments may be unavoidable. The measurement methodology will treat a case as incorrect, even where the claimant has promptly reported a change and there is only a short processing delay
Verification easements due to coronavirus
A number of operational easements and changes to benefit administration were introduced as a consequence of the coronavirus pandemic. Some of these changes were necessary to comply with public health restrictions, and others were introduced to meet departmental aims to protect vulnerable customers and ensure benefit claim processing was as timely as possible.
Many easements related to verification of evidence required to complete a benefit claim. Examples include verification of identity, of household circumstances, and of tenancy agreements.
Last year we carried out analysis as detailed in Appendix 3 of the FYE 2021 main publication.
to understand whether we needed to make any adjustments to the rates of Fraud and Error from when various benefits were last reviewed, in order to more accurately reflect the current rates of Fraud and Error.
From rerunning the analysis on the three benefits we did not measure this year (BSP, CA, JSA), we estimate that no more than £1m would have been added to each benefit’s MVFE.
Impact of the £20 uplift on Universal Credit
The UC standard allowance was temporarily increased by £20 a week to support households through the COVID-19 pandemic. This was withdrawn during FYE 2022, such that UC awards with an assessment period ending after 5th October 2021 no longer received the uplift. The UC sample overrepresented claims that received the uplift during FYE 2022, due to the time lag between the sampling period and the financial year. We estimate that the overpayment rate for FYE 2022 would have still been 14.7% had the £20 uplift not applied to any case in the sample. Therefore, the overrepresentation of claims receiving the UC uplift had a negligible influence on the overpayment rate.
Impact of Earnings Taper Rate and Work Allowance Changes on Universal Credit
A deduction is made to account for any earned income when calculating the UC payment. The deduction depends on the amount of earned income, the Earnings Taper, and the Work Allowance. The Earnings Taper is the rate at which the UC payment reduces as earnings increase. The Work Allowance is the amount some households are allowed to earn before the UC payment is affected.
The Earnings Taper rate and Work Allowance thresholds changed during FYE 2022. For any assessment period ending on or after 24th November 2021: the Earnings Taper rate reduced to 55% (from 63%); the Work Allowance thresholds increased by £42. These changes act to decrease the earnings deduction and increase the UC payment as a result.
The UC sample for FYE 2022 underrepresented claims that were subject to the updated Taper Rate and Work Allowance, due to the time lag between the sampling period and the financial year. We estimate that the overpayment rate for FYE 2022 would have been 0.1 percentage points lower had the new Taper Rate and Work Allowance applied to all cases in the sample. The underrepresentation of these claims is therefore considered to have had a minimal impact and no adjustment has been made.
Impact of SEISS Payments
The UK government paid grants to self-employed people impacted by the COVID-19 pandemic under the Self-Employed Income Support Scheme (SEISS). SEISS payments could be claimed up to and including 30th September 2021.
Universal Credit
The UC sample for FYE 2022 overrepresents claims that could have received a SEISS payment, as it does not align completely with the financial year. It is estimated that the overall overpayment rate may be overstated by 0.1 percentage points as a result. No adjustment has been made due to the small impact.
Housing Benefit
The HB sample for FYE 2022 also overrepresents claims that could have received a SEISS payment, due to not aligning completely with the financial year. There is a negligible impact on the overall overpayment and underpayment rates, so no adjustment has been made.
PIP Award Reviews
There was a specific change made as a direct result of the coronavirus pandemic with regard to when PIP claims in payment are reviewed. Some PIP claims are subject to reviews through an ‘Award Review’ (AR). The change resulted in claims that were due an AR from April 2020 having their AR paused for four months.
We investigated the potential impact on Fraud and Error of this change, by taking the extension to ARs into consideration. This was estimated using existing published data on PIP, using numbers of ARs delayed, the length of the pause, and likely changes in the rate of benefit received after the AR.
The latest published data showed that around 32,000 ARs had been cleared each month from February 2021 to January 2022 (the most recent 12 month period from data published on Stat-Xplore). We assumed that this many cases had been delayed from each month beginning in April 2020.
Data on the outcomes of ARs could be used to determine the proportions of these cases that had their awards changed (i.e., increased, decreased or disallowed). AR outcomes from the period February 2020 to January 2022 (all months available on Stat-Xplore) were used on a rolling 12 month basis, to indicate monthly proportions.
The associated monetary changes to PIP awards could be determined by using information on PIP awards before and after the AR (derived from published table 6C and PIP benefit rates. Changes to award rates from the whole period of the published data (June 2016 to January 2022) were used, and these were not noticeably different from those for more recent ARs.
All of this information was then combined, including the number of ARs that had been delayed within FYE 2022, in order to arrive at the initial estimates of £130m overpayments and £60m underpayments on PIP in FYE 2022.
The overpayment estimates were presented as three scenarios, with different possible reductions to take account of adjustments we usually apply for PIP, where claimants may not be reasonably expected to know to report an improvement in their functional needs.
We also noted that claimants may ask for the AR outcome to be reviewed (a Mandatory Reconsideration), and they may also appeal the outcome of that. However, we have not been able to adjust the overpayment estimates in the main publication appendix to account for this, as there is no published data on the rates of these in relation to outcomes of ARs. Over 13% of all PIP initial assessment decisions in FYE 2021 had their award changed after either a Mandatory Reconsideration or an appeal. The true overpayment estimate from our modelling would likely be lower by at least this amount – perhaps more if Mandatory Reconsiderations and appeals are more likely for claimants whose awards are decreased or disallowed (than for claimants whose awards are increased or maintained at an AR).
The estimates for this can be found in appendix 3 of the main publication.
Omissions from the estimates
The Fraud and Error estimates do not capture every possible element of Fraud and Error. Some cases are not reviewed due to the constraints of our sampling or reviewing regimes (or it is impractical to do so from a cost or resource perspective), some cases are out of scope of our measurement process, and some elements are very difficult for us to detect during our benefit reviews. The time period that our reviews relate to means that any operational or policy changes in the second half of the financial year are usually not covered by our measurements.
For most omissions from our estimates we make adjustments or apply assumptions to those cases. For some omissions we assume that the levels of Fraud and Error for those cases are the same as for the cases that we do review, and for other omissions we apply specific assumptions where we expect the levels of Fraud and Error to be different.
This section of this document details the omissions from the estimates as far as possible. The examples that follow are not an exhaustive list but are an attempt at providing further details on known omissions in the estimates.
There are a number of groups of cases that we are unable to review or which we do not review. Some of the main examples of these are as follows:
-
from a sampling perspective, we do not review cases in some geographically remote local authorities (Orkney Islands and the Shetland Islands). We assume that the rates of Fraud and Error in these areas we do not sample from are the same as for the rest of Great Britain
-
we are unable to review short duration cases (of just a few weeks in duration) due to the time lags involved in accessing data on the benefit caseloads, drawing the samples and preparing these for reviewing. For these cases, we assume the rates of Fraud and Error are the same as in the rest of the benefit caseloads. We do, however, also make an adjustment using “new cases factors” to try to ensure that the results are representative across the entire distribution of lengths of benefit claims (see section 5 for further details)
New and short-term cases
It can take time for new cases to be available for sampling, meaning they are potentially under-represented in the sample. Analysis was undertaken to quantify the impact of these potential exclusions.
New cases make up a small proportion of cases for most benefits. The table below shows the yearly average percentage that are less than three months old at a given time.
Benefit | Average number of cases less than three months old |
---|---|
AA | 3.7% |
ESA | 1.9% |
PC | 1.5% |
HB | 1.0% |
UC | 9.2% |
SP | 2.1% |
Sensitivity analysis was carried out across all benefits to estimate the impact of excluding new cases if the error rate were doubled, halved or remained the same.
The age of a case when it becomes available for sampling differs by benefit, e.g. AA claims must be at least 6 weeks old and HB claims at least 10 weeks. Based on these timescales, analyses were undertaken using an exclusion period of either 6 weeks or 3 months.
Overall, this analysis showed that because short term cases make up such a small percentage of total cases at any given time and many are available to be sampled later in the period, the impact on final published figures for all benefits is negligible.
Results showed that the impact of excluding new cases for all benefits rounds to 0.0 percentage points except for UC overpayments. For UC overpayments the scenario of doubling the error rate increases the impact by 0.2 percentage points and halving the error rate decreases it by 0.1 percentage points. The latter is the scenario we would consider more likely due to new claims having had recent checks and less time has passed for changes in circumstances, meaning we would expect a lower propensity for Fraud and Error.
PIP short-term cases
Cases that are less than 92 days old are excluded from sampling for PIP. Cases that are excluded at the start of the sampling year will become available for sampling once they are older than 92 days.
A simulation of the sampling process was performed and repeated multiple times to investigate the impact of this exclusion. Typically, around 2.7% of cases are excluded, with a negligible proportion of excluded cases reviewed later once they become available for sampling.
The results of a sensitivity analysis on this basis are included below. It would be expected that the rate of Fraud and Error in these cases would typically be lower than the full population of claimants since they have recently been assessed. There is little impact on the published statistics and no adjustment is required.
Estimated impact of excluding short-term cases on the PIP overpayment rate and global overpayment rate
Level of overpayment in excluded group | Estimated change in overpayment | Impact on PIP overpayment estimate | Impact on global overpayment estimate |
---|---|---|---|
Double the published rate (0.6%) | + £1.1m | + 0.0 p.p | + 0.0 p.p |
Half the published rate (0.15%) | - £0.6m | - 0.0 p .p | - 0.0 p .p |
Estimated impact of excluding short-term cases on the PIP underpayment rate and global underpayment rate
Level of underpayment in excluded group | Estimated change in underpayment | Impact on PIP underpayment estimate | Impact on global underpayment estimate |
---|---|---|---|
Double the published rate (0.6%) | + £15.5m | + 0.1 p.p | + 0.0 p.p |
Half the published rate (0.15%) | - £7.8m | - 0.1 p.p | - 0.0 p.p |
Unclaimed Benefits
Eligible claimants that have not made a benefit claim are not considered in these figures. Statistics on benefit take-up can be found online.
Disallowed claims
Claims which do not receive an award are not included in our sample. These claims may have been disallowed in error, resulting in a possible underpayment.
Using data on the number of disallowed claims and appeal success rates, sensitivity analysis was carried out to investigate the impact of various assumed error rates for disallowed cases.
A summary across benefits for various error rate scenarios is shown below. The worst-case scenario is not plausible and is included to illustrate that even assuming an unrealistic level of error, the adjusted estimate would still fall firmly within the published confidence intervals.
Results show that there is likely to be little to no impact on our estimate of underpayments as a result of not sampling disallowed claims.
Attendance Allowance
Scenario | Estimated change in underpayment | Impact on AA underpayment estimate | Impact on global underpayment estimate |
---|---|---|---|
Extreme worst case: appeal success rate applied to all disallowed claims | + £30.1m | +0.6 p.p | + 0.0 p.p |
Disallowed cases have the same error rate as measured cases | + £16.9m | + 0.3 p.p | + 0.0 p.p |
Twice as many appeals are received and the success rate is the same | + £1.6m | + 0.0 p.p | + 0.0 p.p |
Employment Support Allowance
Scenario | Estimated change in underpayment | Impact on ESA underpayment estimate | Impact on global underpayment estimate |
---|---|---|---|
Extreme worst case: appeal success rate applied to all disallowed claims | + £34m | + 0.3 p.p | + 0.0 p.p |
Disallowed cases have the same error rate as measured cases | + £5.6m | + 0.0 p.p | + 0.0 p.p |
Twice as many cases are eligible to be awarded on appeal as actually are | + £0.7m | + 0.0 p.p | + 0.0 p.p |
Personal Independence Payment
Scenario | Estimated change in underpayment | Impact on PIP underpayment estimate | Impact on global underpayment estimate |
---|---|---|---|
Extreme worst case: All disallowed cases are appealed with the same success rate | + £142.8m | + 1.1 p.p | + 0.1 p.p |
Disallowed cases have the same error rate as measured cases | + £22.1m | + 0.2 p.p | + 0.0 p.p |
Note: PIP worst case analysis is slightly different due to its high appeal rate
Pension Credit
Scenario | Estimated change in underpayment | Impact on PC underpayment estimate | Impact on global underpayment estimate |
---|---|---|---|
Extreme worst case: appeal success rate applied to all disallowed claims | + £7.4m | + 0.1 p.p | + 0.0 p.p |
Disallowed cases have the same error rate as measured cases | + £3.2m | + 0.1 p.p | + 0.0 p.p |
Twice as many cases are eligible to be awarded on reconsideration as actually are | + £0.8m | + 0.0 p.p | + 0.0 p.p |
Universal Credit
Appeals information was not available for UC therefore the sensitivity analysis could not be carried out fully as in the above tables.
Using numbers of disallowed cases, analysis shows that even if the error rate in disallowed cases was double that found in the sample, the total difference in the UC rate would be 0.0 percentage points.
State Pension
Recent data was not available on the number of disallowed State Pension claims, however, given the data available it is expected to be very low. For the most recent year for which data was available (December 2020 to November 2021), applying sensitivity analysis again resulted in estimated additional underpayments of below 0.0%.
Housing Benefit
Applications for housing benefit are processed by local authorities, not centrally by DWP as with the other benefits reviewed, and it was not possible to obtain numbers of disallowed cases.
Since analyses of other benefits has shown negligible impact in excluding disallowed claims, the assumption is made that the impact on Housing Benefit is also negligible.
In conclusion, no adjustments are required to the estimates to account for the exclusion of disallowed cases from the sample.
Nil payment claims
A case is considered to be nil-payment if there is a claim in place but the total award being paid is zero. These cases are not included when the sample is selected. Some benefits do not allow a nil-award, meaning all active claimants are receiving some payment.
Some cases receive a nil-award | No cases receive a nil-award |
---|---|
ESA | AA |
PC | HB |
UC | SP |
PIP |
Note:
-
for AA, whilst no cases are classed as having a nil-payment, it is possible for cases to be suspended, for example if the claimant is in hospital. However, these are included in the sample
-
for a very small proportion of the caseload (0.2%), the combination of award rates (daily living and mobility) is reported as nil-nil. Investigations suggest that award rates may be temporarily shown as nil for a short period whilst a claim review is in process, after which the new award rate is set. These cases will be monitored
Nil-payment claims are a potential source of underpayment that is not included in the sample.
Employment Support Allowance
For the year up until August 2021, the most recent year for which data is available, the number of nil-payment claims ranged from 5.7% to 6.1%, with 6.0% being the average. Simulating the sampling process shows around 6.8% of claims that would otherwise be sampled are missed due to this. The potential impact of a different error rate in this group is an increase of 0.1% if the error rate is the same or half that of the measured rate. The worst-case scenario of this group having double the measured error rate would increase the rate by 0.3%.
Pension Credit
Only a very small percentage of Pension Credit claimants are in nil-payment at any given time. For the most recent year of data available this number was always below one fifth of one percent. Simulating the sampling process shows that over 99.8% of cases sampled would be the same even if the nil-payment cases were included in the group available for sampling. The potential impact of a different error rate in this group thus rounds to zero, even in a worst-case scenario of doubling the error rate in the excluded cases.
Universal Credit
For Universal Credit the information available is the number of cases that are not receiving payment at a given point in time. This number is published monthly.
If we assume that the proportion of cases that have an underpayment is the same as it is in our sample, 7.1%, and that the average amount underpaid is the same as in our sample, then the additional underpayment would be around £70 million. This is around 0.2 % of UC expenditure and within the published error bands.
Impact of exclusions from the PIP sample population
The monthly samples are taken from live PIP claims in advance of the scheduling of the benefit reviews. Any benefit record relating to a claimant who meets specific exclusion criteria (e.g. terminally ill, reviewed in the last three months) will not be reviewed. We assume the rates of Fraud and Error for these cases are the same as the rest of the PIP caseload. The potential impact of each excluded group are summarised below.
Terminally Ill cases
Terminally ill claimants make up a very small percentage of PIP claimants, typically around 1.1%. Sensitivity analysis was carried out to test the impact of their exclusion if the Fraud and Error rate on these cases were to differ by as much as double that of the sampled population.
Estimated impact of excluding terminally ill cases on the PIP overpayment rate
Level of overpayment in excluded group | Estimated change in overpayment | Impact on PIP overpayment estimate | Impact on global overpayment estimate |
---|---|---|---|
Double the published rate (0.6%) | + £0.4m | 0.0 p.p | 0.0 p.p |
Half the published rate (0.15%) | - £0.2m | 0.0 p .p | 0.0 p .p |
Estimated impact of excluding terminally ill cases on the PIP underpayment rate
Level of overpayment in excluded group | Estimated change in underpayment | Impact on PIP underpayment estimate | Impact on global underpayment estimate |
---|---|---|---|
Double the published rate (0.6%) | + £5.7m | 0.0 p.p | 0.0 p.p |
Half the published rate (0.15%) | - £2.8m | 0.0 p .p | 0.0 p .p |
The small proportion means the total estimated level of Fraud and Error would differ by less than 0.05 percentage points in the above scenarios, therefore no adjustment has been made to the statistics because of this exclusion.
Scheduled reviews
PIP awards are reviewed regularly. The period between reviews is set on an individual basis and ranges from 9 months to 10 years, with the majority of claimants having a short-term award of 0-2 years. If a claim has had a planned award review in the last 92 days, has a review ongoing or a review due in the next six weeks then it is not eligible to be sampled.
A simulation of the sampling process was performed and repeated multiple times to investigate the impact of this exclusion.
Cases with an upcoming review would be expected to have a higher propensity for Fraud or Error due to the length of time since their last review. By the same reasoning cases that have had a review completed recently would be expected to have a lower propensity for Fraud or Error.
The split of review outcomes is published as part of the official statistics for PIP, and were used in estimating the impact of excluding cases with scheduled reviews. It was assumed that an award that is increased after a planned review would have an underpayment had we reviewed it, and cases that were decreased or disallowed would have an overpayment.
Estimated impact of excluding cases with a review that is due, ongoing, or recently completed using review outcomes to estimate Fraud and Error rates
Zero error in recently reviewed cases
Estimated difference (£) | Impact on PIP estimate (percentage point change) | Impact on global estimate | |
---|---|---|---|
Overpayment | + £26.9 m | + 0.2 p.p. | + 0.0 p.p. |
Underpayment | + £94.4 m | + 0.7 p.p. | + 0.0 p.p. |
Half the sampled rate of error in recently reviewed cases
Estimated difference (£) | Impact on PIP estimate (percentage point change) | Impact on global estimate | |
---|---|---|---|
Overpayment | + £71.4 m | + 0.5 p.p. | + 0.0 p.p. |
Underpayment | + £139.1 m | + 1.0 p.p. | + 0.1 p.p. |
The total estimated level of Fraud and Error would differ by a maximum of 1.0 percentage points in the above scenarios, with a maximum of 0.1 percentage point impact on the global estimate. Therefore, no adjustment has been made to the statistics because of this exclusion.
Get your State Pension
Get Your State Pension is a relatively new system on which claims to SP are recorded. No cases were selected in the SP sample from the new Get Your State Pension system as we are as yet unable to access the data on it for sampling purposes. The numbers of cases on this new system are very small in comparison to the caseload as a whole (approximately 3%). An assumption has been made that the Fraud and Error present on these cases is the same as those in the legacy recording system from which our sample is drawn.
Time Lags
The time lags involved in the Fraud and Error measurement process mean that further omissions are possible. Any policy or operational changes in the second half of the financial year of the annual publication will not usually be covered by the reviews feeding into the publication, as the reviews tend to finish in the September of that financial year (although the timings of reviews have been affected by the coronavirus pandemic). In addition, some cases do not have a categorisation by the time the estimates are put together, often due to an ongoing fraud investigation. “Estimated outcomes” are generated for these cases for the purposes of the statistics, made by the review officer estimating the most likely outcome of the case, or based on the results from the reviews of similar cases that have been completed.
For all benefits we carried out additional work to better understand any implications of major policy/operational changes within the financial year. The conclusion was that we felt the sample period was representative of the financial year. See section 5 for further details.
Work Capability Assessment
Measurement of ESA was first included in the FYE 2014 estimates, and followed the existing methodology for JSA, IS and PC, reviewing the financial side of a claimant’s circumstances. However, the measurement of ESA does not include a review of the Work Capability Assessment; this is also the case for the Work Capability Assessment for claimants on UC.
Other elements out of scope
Some elements are out of scope of our measurement. Benefit advances is one of these (see separate section lower down). Other examples include:
-
we only review the benefit that has been selected for a review, and do not assess any consequential impacts on other benefits. However, in certain circumstances, for some benefits, there may be a knock-on impact on other benefits. An example of this is how changes in entitlement to DLA or PIP affects disability and carer premiums on income-related benefits (specifically IS, PC and HB), as well as CA. We account for this in our estimates by using DWP’s Policy Simulation Model to assess the impact. The Policy Simulation Model is the main micro-simulation model used by DWP to analyse policy changes, and is based on the annual Family Resources Survey
-
the accuracy of third party deductions is not measured (i.e. whether the deduction is at the correct amount and is still appropriate). Third party deductions can take place to cover arrears for things like housing charges, fuel and water bills, Council Tax and child maintenance. The rate of benefit is not impacted by any third party deductions, and the amount of any Fraud or Error is based on the “gross” amount of benefit in pay
-
for UC, we do not assess whether the Department follows correct “labour market” procedures and takes any necessary follow up action for non-compliance by claimants (i.e. considers whether a sanction should apply if a claimant fails to apply for a job or leaves a job voluntarily). However, if a sanction decision has already been made when we review a case, then we do assess whether the impact this has on the benefit award is correct
-
for UC, we only measure income in the assessment period we are checking. Self-employed people must report their income on a monthly basis. If they receive income that removes their entitlement to UC in one month, and this is above the surplus earnings threshold, then any extra income is carried forward into the next month (the surplus earnings threshold is defined as £2,500 above the amount that removes their entitlement to UC in that month). If a self-employed claimant incurs a loss of any amount within an assessment period, this loss is also rolled forward to the next assessment period. When reviewing the benefit, any rolled forward income or loss is assumed to be correct
There are also elements of fraudulent activity that are very hard for us to detect during the benefit reviews which underpin the Fraud and Error estimates. For example, earnings from the hidden economy that are not declared by claimants, or the impact of cyber-crime on benefit expenditure (e.g., due to fraudulently made claims). The Department does identify cyber-crime through its Integrated Risk and Intelligence Service, which is also used to help identify a range of fraudulent activities. However, these can be very difficult to identify within the measurement process for our Fraud and Error statistics.
Benefit Advances
One of the largest current omissions from our estimates is benefit advances, which are out of scope of our measurement. The benefit review process for the Fraud and Error statistics examines cases where benefit is in payment. A benefit advance is not a benefit payment and is not included in our measurement process. Claimants who progress to receive payment of a benefit will be included within the scope of our measurement, but we will only review the existing benefit payment. This will not examine Fraud or Error that may have existed in any prior benefit advance payment. Claimants who only receive a benefit advance, but do not go on to receive a subsequent benefit payment, will not be included within the measurement. Advances are available for a number of benefits but, for FYE 2022, advances for UC constituted the vast majority of expenditure on benefit advances.
UC supports those who are on a low income or out of work. It includes a monthly payment to help with living costs. If a claim is made to UC but the claimant is unable to manage financially until their first payment, they may be able to get a UC Advance, which is then deducted a bit at a time from future payments of the benefit.
The latest published figures on the levels of potentially fraudulent UC advances are from a National Audit Office (NAO) report released in March 2020. The data in the report is now over two years out of date, and the Department has done a lot of work to reduce the volume and amount of advances Fraud within that time. Therefore, we feel that the estimate contained within the report is not representative of the current situation.
Note: a small (but not insignificant) proportion of expenditure on benefit advances is included in the published expenditure forecasts from Spring Budget, which are used in our estimates. This does not affect the rates of Fraud and Error we report for various benefits – these are based on the reviews of benefit cases currently in payment that we carry out. However, part of the monetary estimates of Fraud and Error that we report will effectively relate to advances. For example, around £40m of the Universal Credit monetary estimates of Fraud and Error is related to advances rather than the benefit itself.
Rounding policy
In the publication and tables, the following rounding conventions have been applied:
- percentages are rounded to the nearest 0.1%
- expenditure values are rounded to the nearest £100 million
- headline monetary estimates are rounded to the nearest £10 million
- monetary estimates for error reasons are rounded to the nearest £1 million
Incorrectness is rounded to the nearest 1% in the publication and expressed in the format “n in 100 cases”. The tables present the same values as a percentage rounded to the nearest 0.1%.
Individual figures have been rounded independently, so the sum of component items do not necessarily equal the totals shown.
4. Sampling and Data Collection
The Fraud and Error statistics are determined using a sample of benefit records, since is it not possible to review every benefit record. The sample of benefit records provide data from which inferences are made about the Fraud and Error levels in the whole benefit claimant population.
The number of benefit records to be reviewed is determined by a sample size calculation. The sample size calculation is used to ensure that a sufficient number of benefit records are sampled, which allows meaningful changes in the levels of Fraud and Error to be detected for the whole benefit claimant population.
Benefit records are selected on a monthly basis from data extracts of the administrative systems. The population from which the samples are drawn are the benefit records that are in payment in a particular assessment period, where there is evidence of a payment relating to the previous month. This is known as the liveload.
The monthly samples are taken from the liveload in advance of the scheduling of the benefit reviews, to give time for the sample to be checked and for background information to be gathered on each benefit record sampled. Any benefit record relating to a claimant who has been previously sampled in the last 6 months, or meets specific exclusion criteria (e.g. terminally ill) will not be reviewed.
We use Simple Random Sampling to select samples of benefit records for each benefit that is reviewed in the current year. Benefit records are sampled randomly to ensure an equal chance of being selected for the sample.
The sampling methodology is used to attempt to minimise selection bias in the sample and aims to select a sample that is representative of the entire benefit claimant case population.
The benefits sampled for this year and the methodologies applied are as follows:
Simple random sample:
-
Employment and Support Allowance
-
Pension Credit
-
Universal Credit
-
State Pension
-
Attendance Allowance
Housing benefit methodology was simple random sampling stratified by PSU and four different client groups:
-
Working Age in receipt of IS, JSA, ESA, PC or UC
-
Working Age not in receipt of IS, JSA, ESA, PC or UC
-
Pensioners in receipt of IS, JSA, ESA, PC or UC
-
Pensioners not in receipt of IS, JSA, ESA, PC or UC
Note: only one client group was reviewed for HB in FYE 2022. See section 2 for more details
Abandoned Cases
Of the benefit records sampled, there are some that cannot be reviewed and have to be y excluded from the sample, according to pre-defined criteria for abandonment. Typical examples of benefit records that fall into this category are:
-
the claimant has a change of circumstances that ends their award before the interview can take place
-
the claimant has had a benefit reviewed within the last six months
-
if the claimant or their partner is terminally ill
When such cases occur in the sample, they are replaced by another case, from a reserve list. However, for a small number of abandoned cases replacement is not possible for practical reasons.
Abandoned Cases FYE 2022
It is the decision of the Performance Measurement (PM) team, during the preview stage of a case, if a case should be abandoned.
The following table shows the main reason for cases abandoned for each benefit.
Abandonment Reason / Benefit | AA | ESA | HB | PC | SP | UC | Total |
---|---|---|---|---|---|---|---|
Benefit not in payment/ceased or suspended | 7 | 42 | 630 | 51 | 0 | 350 | 1,080 |
Sensitive issues | 69 | 51 | 8 | 64 | 11 | 7 | 210 |
Incorrectly sampled | 42 | 0 | 24 | 0 | 0 | 0 | 66 |
Operational Issues | 6 | 23 | 25 | 8 | 1 | 3 | 66 |
Claimant in Hospital | 2 | 17 | 6 | 16 | 0 | 9 | 50 |
Miscellaneous | 42 | 15 | 22 | 30 | 87 | 62 | 258 |
Total Cases Abandoned for Each Benefit | 168 | 148 | 715 | 169 | 99 | 431 | 1,730 |
Total Sample Cases for Each Benefit | 1,610 | 1,999 | 2,738 | 1,999 | 1,499 | 2,995 | 12,840 |
Abandonment Rate for Each Benefit | 10% | 7% | 26% | 8% | 7% | 14% | 13% |
The five named reasons in the table account for just around 85% of all abandonments. The Miscellaneous category is made up of a number of different reasons which only have a small number of cases for each.
-
Benefit not in Payment/ceased or suspended – This is the largest cause of abandonment, with the vast majority of these cases relating to HB and UC. These claims are no longer in payment either because the claimant is no longer entitled to the benefit or because they are now claiming another benefit. There is a time lag between the sample being selected and the benefit review taking place. or HB claims this lag is more than six weeks. This time lag accounts for 23% of HB cases being abandoned
-
Sensitive Issues – This reason affects all benefits reviewed. Reasons include the death of the claimant or a close relative, or that we are made aware of terminal illness
-
Incorrectly sampled - This is where circumstances have changed and the claimant is no longer included in the client group being sampled (for HB) or there has been interaction with the Department in the three months prior to the benefit review (for AA)
-
Operational Issues – This relates to reviews being difficult or impossible to complete, due to unforeseen circumstances. An example of this is discovering a claimant is not able to manage their own affairs, but no named contact is held for the claimant or there is nobody available to provide assistance to the claimant, in order for the review to be completed
-
Claimant in Hospital – This reason is used when the claimant is in hospital during the review process, but also when the discharge date is not known
-
Miscellaneous – This is a separate category that covers all remaining categories of abandonment. There are over 60 different categories for abandonment used by PM, with many in this category having less than 5 cases abandoned
Most of these reasons are unavoidable due to the time lag between sample provision and benefit previews and reviews commencing. For example, we are not able to predict if a person may fall ill or stop claiming a benefit.
We are currently looking at improvements to our approach towards abandoned cases and how they are dealt with for our sampling process.
Official Error Checking
Cases are checked for official errors within a specified sample week. For UC this is the assessment period of one month prior to the last payment (or the date payment was due if no payment is made).
Specially trained DWP benefit review officers carry out the checks. The claimant’s case papers and DWP computer systems are checked to determine whether the claimant is receiving the correct amount of benefit according to their presented circumstances. This identifies any errors made by DWP officials in processing the claim and helps prepare for the next stage: a telephone review of circumstances with the claimant.
Claimant Error and Fraud Reviews
For all benefits, benefit review officers normally check for Claimant Error (CE) or Fraud by comparing the evidence obtained from the review to that held by the Department. The claimant may not be interviewed if:
-
the case is already under an ongoing fraud investigation
-
a suspicion of Fraud arises while trying to secure an interview
When such cases occur in a sample, the outcome of the fraud investigation is used to determine the review outcome.
Where the claimant reports a change of circumstances that results in entitlement to that benefit ending, an outcome of Causal Link would be considered without the claimant being interviewed. See section 5 for information on Causal Link errors.
Types of errors excluded from our estimates
Some failures by DWP staff to follow procedures are not counted as official errors; where the failure does not have a financial impact on the benefit award or where the office have failed to take action which could have prevented a claimant error from occurring. These are called procedural errors.
Accounting errors are errors where, despite an error in the claimant’s benefit, an overpayment (or underpayment) of the benefit undergoing a check could be offset against any corresponding underpayment (or overpayment) on the same benefit or in the case of State Pension and Pension Credit each other. For State Pension and Pension Credit these errors are excluded from the monetary estimates but are included in incorrectness estimates. For errors where the offset is on the same benefit they are excluded completely.
Deemed errors are official errors where evidence that was available to the department when the award of benefit was made has been misplaced or is not available. This means that the official error check is not complete. These errors are not counted as official errors, since we cannot be sure whether there would have been a financial impact on the claimant. Any case that has a deemed error raised against it is also excluded from the calculation of the official error rate (but may still be counted in the calculation of Claimant Error and Fraud)
Recording Information
Case details relating to the Fraud and Error reviews are recorded on internal bespoke ‘Fraud and Error’ software (the system is known as FREDA), to create a centrally held data source. This can then be matched against our original sample population to produce a complete picture of Fraud and Error against review cases across our sample.
5. Measurement Calculation Methodology
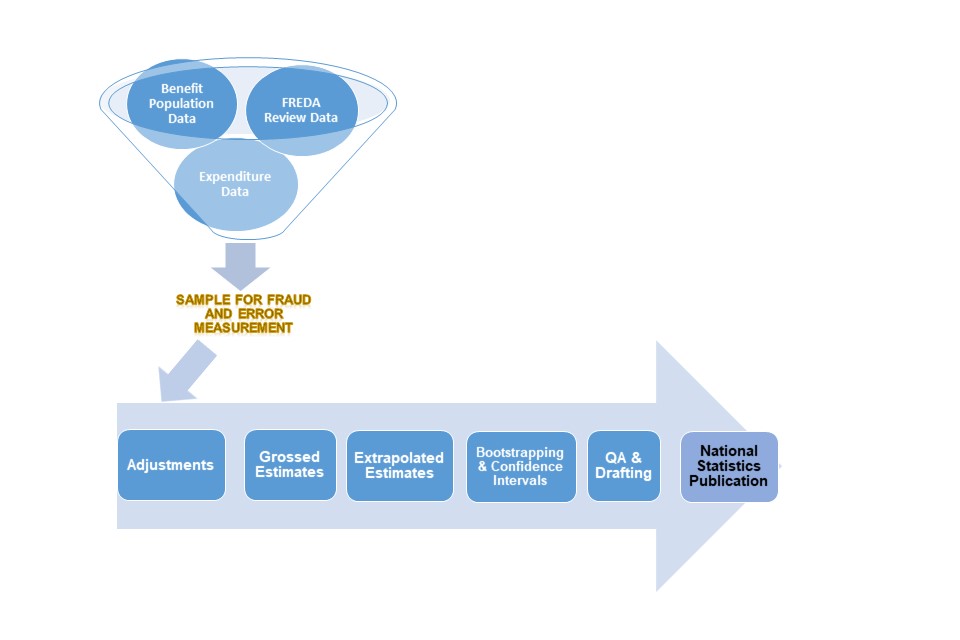
Fraud and error measurement relies on three data sources:
-
Raw Sample held on ‘FREDA’ (the database on which the review outcomes are recorded), is used to identify the Monetary Value of Fraud and Error (MVFE) for individual cases, categorise its cause and quantify it as a proportion of the sample
-
Benefit Population data to estimate the extent of Fraud and Error across the whole claimant caseload from the sample data
-
Expenditure data to estimate the total MVFE to the department
Estimates are categorised into overpayments (OP) and underpayments (UP) and one of three incorrectness types: Claimant Fraud (CF), Claimant Error (CE) or Official Error (OE). Further sub-categories of error reasons are used to provide more details about the nature of the Fraud or Error. Details on error classifications can be found in the glossary at Appendix 3.
Detailed below are the main calculation steps that the Fraud & Error Measurement and Analysis (FEMA) team carry out to produce the final Fraud and Error estimates.
Causal Link
Cases where there is a change to the claimant’s award as a result of the review activity or, after initial contact, the claimant subsequently fails to engage in the review process, are categorised as Claimant Fraud with causal link. Action is taken to suspend their payment and subsequently terminate their claim.
Examples of behaviours that can trigger cases to be categorised as Causal Link include:
-
the claimant receives notification of the review and subsequently contacts the department to report an immediate change e.g. living with a partner, starting work, self-employment or capital changes. Then supporting evidence needed to verify the change is not provided, resulting in claim suspension and termination
-
the claimant completes the review but subsequently declares that a change has happened shortly following the period of the review
-
the claimant receives notification of the review and does not engage in the review process or contacts the department with a request to withdraw their claim
-
the claimant completes a review and a change is declared, however supporting evidence needed to verify the change is not returned, resulting in claim suspension and termination
For UC there are cases where the claimant fails to engage in the review process, but there is supporting evidence that a change is not due to the review. These are categorised as ‘mitigating circumstances’. For these cases, information is available on our systems to indicate why the person may not have engaged. In most cases, they have moved into paid work following the Assessment Period under review.
For all benefits post-review, every Causal Link error is categorised as either high suspicion or low suspicion. This categorisation is used in the netting and capping procedure to help attribute losses to the error reasons we are most confident about. Any losses attributed to low suspicion Causal Link after netting and capping will have their error reason changed to “Failure to provide evidence/fully engage in the process”.
Examples of high suspicion Causal Link errors include:
-
shortly after review the claimant terminates their claim (rather than send in evidence)
-
the claimant told us at the review of a change of circumstances, but we cannot confirm that the change occurred prior to (or in) the assessment period checked
-
post-review, the claimant made a change of circumstances that cannot be confirmed as starting after the assessment period checked
-
when asked to send in more information or to clarify further queries on evidence sent in, the claimant stops engaging
The main reason that we would class a case as low suspicion is where the claimant fails to send in evidence but we have no prior suspicions of fraudulent intent.
Adjustments
A series of adjustments are made to the sample data, to allow for various characteristics of the benefits and how their data is collected and recorded. The following table highlights which adjustments apply to each of the benefits reviewed in FYE 2022:
CA | ESA | PC | HB | UC | AA | |
---|---|---|---|---|---|---|
Netting and Capping | Y | Y | Y | Y | Y | Y |
Estimated Outcomes | Y | Y | Y | Y | Y | Y |
New Cases Factor | N | Y | Y | Y | N | N |
Cannot Review Cases | N | N | N | Y | N | N |
Underlying Entitlement | Y | Y | Y | Y | Y | Y |
Reasonably Expected to know | N | N | N | N | N | Y |
Y=Adjustment applies, N=Adjustment does not apply
Netting and Capping
Where a case has more than one error, these errors can be “netted off” against one another to produce a total value. For example, if a case is found to have two different OEs, one leading to an UP and one leading to an OP, then these are “netted off” to produce a single OP or UP. This is done to better represent the total monetary loss to the public purse (via OPs) or to the claimant (via UPs).
The monetary loss on each case is the difference between the case award paid at the review/assessment period, and the correct award calculated following the review – the “award difference”.
A case may have OPs of more than one ‘type’ which sum to a total greater than the award difference. To ensure that the total OP does not exceed the total award difference, we ‘cap’ the OP amount using a hierarchy order of actual CF, Causal Link (high suspicion) CF, Causal Link (low suspicion) CF, CE then OE. This capping process means that a small proportion of CE and OE found during the survey is not included in the estimates, and therefore the final estimates may actually be under-reporting CE and OE in the benefit system.
Estimated outcomes
For all benefits reviewed this year, there were a number of cases where the review process had not been completed at the time of the analysis and production of results, often because fraud investigations are still to be completed. Predictions for the final outcomes for these cases have been made in the analysis using either the review officer (RO) estimation of the most likely outcome, or the results from the reviews of similar cases that had been completed.
New Cases Factors
New Cases Factors are an adjustment applied to help ensure that the durations on the sample accurately reflect the duration on benefit within the population.
As a result of the time required to collect the information needed to review a case, as well as other operational considerations, there is an unavoidable delay between sample selection and case review. This delay means that fewer low duration claims will be represented in the sample of cases, which artificially introduces a bias around claim durations at the point of interview.
Cannot Review
Cases that cannot be reviewed, primarily due to the claimant not engaging in the review process resulting in their benefit claim being suspended and later terminated, are initially recorded as Fraud.
Not all of these cases will be Fraud so additional checks are conducted at a later date to determine if the individuals have reclaimed benefit or a suspicion of Fraud recorded on the case at the initial preview. The outcome of these checks will result in these cases being re-categorised for reporting purposes. 2.0% of sampled cases in FYE 2022 did not have an effective review.
There are three different categories that are applied to cannot review cases for reporting purposes:
-
Not Fraud - If the individual reclaims benefit within 4 months, with the same circumstances and at a similar rate they were receiving prior to review, then the Fraud is removed
-
Fraud remains - If an individual does not reclaim benefit and there was a suspicion of Fraud raised at the preview stage of the review then the case remains as Fraud
-
Inconclusive – If the individual does not reclaim benefit and there was no suspicion of Fraud at the preview stage of the review then the case is categorised as inconclusive as there is no evidence to suggest the case is Fraud or not
Inconclusive cases are excluded from the estimates and reported separately in footnotes in the publication and supporting tables.
Benefit-specific adjustments
This section contains details of any benefit-specific sampling issues, or things that we only do for certain benefits when we calculate our estimates. This section only usually includes details for benefits which we have reviewed this year.
Universal Credit
Zero payment cases: Universal Credit cases still live but with zero entitlement are not included in our sample for benefit reviews. However, when calculating the levels of incorrectness on Universal Credit we scale the final figure to account for these cases.
State Pension
State Pension has been reviewed fully this year for Claimant Error and Fraud as well as Official Error, for the first time since FYE 2006. We do not review overseas cases as part of the SP review. We assume that the rate of Official Error in the overseas cases (which constitute around 4% of total expenditure on SP) is the same as for cases resident in Great Britain. Overseas Fraud and Claimant Error is calculated differently for SP – see section 2 for details.
Personal Independence Payment
PIP has not been reviewed this year, but some information on the benefit is relevant as it links to information contained within appendix 3 of the main publication.
For disability benefits, there are some changes which the claimant should report (for example, hospitalisation). However, many changes are gradual improvements or deteriorations in their medical needs, and it is difficult for some claimants to know at what point these needs have changed sufficiently to affect their benefit entitlement.
PIP legislation states that when a case is reassessed and their benefit is reduced, the Department will only seek to recover an overpayment when it is reasonable for the claimant to have known they should have reported the change. In other cases, the benefit will be treated as correct up to the point of reassessment.
It was identified that during PIP reviews there appeared to be variance in the application of “Reasonably expected to know” decisions, resulting in such cases not always having overpayments reported.
In Spring 2017, PM staff completed a joint exercise with PIP Operational staff to reconsider all of the information available to identify improvements to the review process to ensure the measurement of PIP was sufficiently robust.
Accordingly, error cases have been excluded from the headline overpayment estimates where the claimant could not reasonably have been expected to know they should have reported it.
Attendance Allowance
AA is subject to the same methodology above on PIP regarding “Reasonably expected to know” cases.
Grossing
Grossing is the term used to describe the creation of population estimates from the sample data; sample results are scaled up to be representative of the whole population.
Example of a simple grossing factor ‘G’, if we were to sample 100 cases from a population of 1,000:
G = N ÷ n
= 1000 ÷ 100
= 10
Where ‘N’ is the population or sampling frame from which the cases are selected and ‘n’ is the sample size taken.
The above grossing factor shows that, in this example (sampling 100 cases from a population of 1,000), then each case would have a grossing factor of 10 (i.e. each sample case represents 10 cases from the population). Hence if a case was shown to be in error, this would represent 10 errors once grossed.
The UC caseload and expenditure is on a significant upward trend, with new case types being introduced as rollout continues. Since UC is replacing other benefits, as UC caseload increases, their caseload will decrease. As a result, grossing for all benefits are calculated on a monthly basis. This ensures that an error identified at the start of the year is grossed up by less than an error identified at the end of the year if the caseload is increasing (or vice versa if it is decreasing).
Grossing factors are different for each benefit due to the sample, population and adjustments made. For ESA and PC, there are two different broad grossing factors, one for official error and one for Fraud and Claimant Error. The official error grossing factor is used on the sample which covers all cases where an official error check was done. The Fraud/Claimant Error grossing factor is used on the sample which covers all cases where a full check was done – i.e. Official Error check and claimant review. For all other benefits there is only one grossing factor as every case has had both an Official Error check and a Fraud/Claimant Error check.
Percentage overpaid and underpaid
The grossing factors are then applied to the sample data to calculate values for the grossed awards, the grossed overpayments and grossed underpayments i.e. these are scaled up proportionally to what we would expect to find in the population. In turn, the resulting grossed values are used to calculate the total (global) annual percentage overpaid and underpaid.
Extrapolation
The grossed results provide a core estimate of levels of Fraud and Error. Extrapolation aligns the monetary amount with the benefit expenditure, which is particularly important given the sample period and the financial year do not fully align.
Monetary Value of Fraud and Error (MVFE)
To then calculate the MVFE across the benefits, we apply the OP or UP percentage rates to the total annual expenditure for each benefit. This means that the MVFE is affected by the increases and decreases in expenditure, even if the OP and UP percentages are stable. We see the impacts of this in our estimates for benefits not reviewed in the current year, where we use the same rate of Fraud and Error from previous years but apply it to the expenditure on the benefit in the current year (which will have changed from the year before).
Although expressing Fraud and Error in monetary terms (i.e. MVFE terms) might be helpful for a reader to contextualize the figures, we recommend making comparisons on a year-on-year basis based on the percentage rates of Fraud and Error. This is particularly important for benefits where the expenditure changes a lot each year (such as we are seeing for UC at the moment), as comparisons of monetary amounts can be misleading. For example, on a benefit with growing expenditure, it can be possible for the monetary amount of Fraud and Error to increase, even if the percentage rate of Fraud and Error has actually gone down.
Central Estimates and Confidence Intervals
The central estimates produced following extrapolation are based on reviews of random samples and hence are subject to variability. Therefore, confidence intervals are provided with the central estimates to quantify the uncertainty associated with these estimates.
The central estimates and confidence intervals are incorporated into the Global (overall) Estimates of Fraud and Error. These combine all separate DWP benefits to calculate an overarching set of Fraud, Claimant Error and Official Error rates for overpayments and underpayments. See section 6 on Measurement of Total Overpayment and Underpayments for more detail.
Incorrectness
The main published figures include Fraud and Error as a percentage of expenditure, and the associated MVFE. We also calculate figures on incorrectness. Incorrectness estimates are published as part of the tables accompanying the publication and are referenced in the main publication.
A case can have both a Fraud/overpayment error and an underpayment error on it. Therefore, it would appear in both the proportion of cases with a Fraud or an overpayment error as well as the proportion of case with an underpayment error. This means that these two incorrectness proportions cannot be summed.
This year we have taken a de minimis approach to incorrectness on State Pension, removing all errors that are 10p or less. For more information then please see “Reporting of three error types for the first time on State Pension” in Section 2 of this document.
6. Measurement of Total Overpayment and Underpayments
The Fraud and Error estimates need to include all expenditure on benefits by DWP. Some benefits have been reviewed for Fraud and Error in the current year, and some have been reviewed in previous years. We also need to include estimates for benefits which have never been reviewed. A full list of which benefits are in scope for each release of the Fraud and Error in the Benefit system estimates is included within Appendix 2 of this document.
We also have an estimate of Interdependencies, the knock on effect of Disability Living Allowance (DLA) Fraud and Error on other benefits, where receipt of DLA or PIP is a qualifying condition. This is only included within the overpayments calculation and not the underpayments.
Methodology for Benefits never reviewed
Taken together, all the benefits reviewed this year or in previous years account for approximately 97% of the total benefit expenditure. Benefits that have never been reviewed account for 3% of the total.
Estimates for benefits never reviewed are created using a common model. Examination of the qualification conditions and administrative structure suggests that benefits belong in “families” of similar benefits. For example, DLA and Industrial Injuries Disablement Benefit (IIDB) are very similar benefits that belong to the same family.
All benefits never reviewed that sit in the same family as a benefit reviewed this year or previously are assigned the same rate of Fraud and Error, in percentage terms, as the benefit reviewed. The benefits never reviewed that are in families that do not contain a benefit reviewed this year or previously are assigned the average rate of Fraud and Error, in percentage terms, of all benefits that have ever been reviewed.
For each of the benefits that have never been reviewed, we apply the estimated percentage of Fraud and Error to the expenditure on that benefit to get an estimate of the monetary value of Fraud and Error.
Central Estimate and Confidence Intervals
The percentage estimate (i.e. the overall rate of Fraud and Error) is the sum of the monetary value of Fraud and Error for all benefits reviewed this year, those reviewed in previous years, those never reviewed and interdependencies, divided by the overall expenditure. This is done independently for Fraud, Claimant Error, Official Error and the overall Fraud and Error.
The central estimate is the estimate obtained from the sample data. It provides our best guess of the unknown value that we are trying to measure.
Confidence intervals are calculated for the percentage estimates, to quantify the statistical uncertainty associated with the central estimate. Some adjustments are made to the individual benefits before this is calculated for overall Fraud and Error:
-
confidence intervals for benefits reviewed previously are deliberately widened
-
confidence intervals may be widened further if it is believed that non-sample error could impact the accuracy of the estimates
-
confidence intervals are widened for the benefits never reviewed, whereby the standard error is assumed to be 40% of the central estimate, to reflect greater uncertainty given the less robust method of estimation for these benefits
The uncertainty surrounding a central estimate is associated with both the variance of the outcome within the sample and the size of the sample from which it is calculated. A 95% confidence interval is used to indicate the level of uncertainty. It shows the range of values within which we would expect the true value of the estimate to lie. A wider range for the confidence interval implies greater uncertainty in the estimate.
Confidence intervals are calculated using a statistical technique called Bootstrapping. It is used to approximate the sampling distribution for the central estimate. The sampling distribution describes the range of possible values, for the central estimate, that could occur if different random samples had been used.
Bootstrapping is a computationally intensive technique that simulates resampling. A computer program is used to take 4,000 resamples with replacement, of equal size, from the initial sample data. The percentage rate of Fraud and Error is calculated for each of the resamples. These estimates are ordered from smallest to largest and this gives the approximated sampling distribution.
The 95% confidence intervals are obtained from the Bootstrapping results, by taking the 100th estimate (2.5th percentile) and the 3,900th estimate (97.5th percentile). We also check the median estimate (50th percentile) against our actual central estimate to ensure that no bias exists.
Calculation of Net Loss estimate
Recoveries refer to money recovered in the same financial year as the overpayment estimates, regardless of the period the debt is from. They include debt recovered by both the Department and Local Authorities (who administer Housing Benefit payments). The recovery data for Housing Benefit covers the period October 2020 – September 2021, due to a time lag on the data being available.
Net loss is calculated as a monetary amount by subtracting the value of the recoveries from the value of the overpayments. The percentage estimate is then calculated by dividing the monetary net loss by the expenditure. Net loss can only be calculated at the overall Fraud and Error level, because error classification differs between overpayments and recoveries.
As the recoveries are actual values rather than estimates, the calculation of net loss does not affect the uncertainty around the overpayment estimates. The confidence intervals for net loss are calculated by subtracting the value of the recoveries from the upper and lower confidence limits. Percentage confidence intervals are obtained by dividing by the expenditure.
Net loss is calculated overall and individually for benefits reviewed this year and previously reviewed benefits. Net loss is also calculated for the group of benefits never reviewed combined, by subtracting all recoveries relating to these benefits from the total overpayments.
Some recoveries have no associated overpayments for the same period, as these benefits are no longer administered by the Department. This is because the debt relates to expenditure from previous years. In addition, some recoveries the Department makes are not included in our net loss estimate as they do not relate to our Fraud and Error reporting. For example, recoveries of tax credits and of benefit advances (which are outside the scope of our measurement; see section 3 for more details).
The overall net loss estimate includes the benefits reviewed this year, benefits reviewed in previous years, benefits never reviewed and recoveries for which there is no overpayment.
7. Future Reporting
The future coverage and scope of the national statistics “Fraud and Error in the Benefit System” is kept under review and users are kept informed of our plans via our Publication Strategy document.
Appendix 1: Glossary of abbreviations
Abbreviation | Full name |
---|---|
AA | Attendance Allowance |
CA | Carer’s Allowance |
CE | Claimant Error |
CF | Claimant Fraud |
DLA | Disability Living Allowance |
DWP | Department for Work and Pensions |
ESA | Employment and Support Allowance |
FEMA | Fraud and Error Measurement and Analysis |
FYE | Financial Year Ending |
HB | Housing Benefit |
HMRC | Her Majesty’s Revenue and Customs |
IB | Incapacity Benefit |
IS | Income Support |
JSA | Jobseeker’s Allowance |
LA | Local Authority |
MVFE | Monetary Value of Fraud and Error |
OE | Official Error |
OP | Overpayment |
PC | Pension Credit |
PIP | Personal Independence Payment |
PM | Performance Measurement team |
SP | State Pension |
UC | Universal Credit |
UP | Underpayment |
Appendix 2: List of benefits included in fraud and error estimates
Benefits reviewed this year
- Universal Credit
- State Pension
- Housing Benefit
- Employment and Support Allowance
- Pension Credit
- Attendance Allowance
Benefits reviewed previously
- Personal Independence Payment (last reviewed FYE 2020)
- Carer’s Allowance (last reviewed FYE 2020)
- Housing Benefit:
- passported pension age (last reviewed FYE 2020)
- passported working age and non-passported pension age (last reviewed FYE 2019)
- Jobseeker’s Allowance (last reviewed FYE 2019)
- Income Support (last reviewed FYE 2015)
- Incapacity Benefit (last reviewed FYE 2011)
- Disability Living Allowance (last reviewed FYE 2005)
Benefits never reviewed
- Maternity Allowance (proxy measure: Employment and Support Allowance)
- Severe Disablement Allowance (proxy measure: Employment and Support Allowance)
- Financial Assistance Scheme (proxy measure: State Pension)
- Industrial Death Benefit (proxy measure: State Pension)
- Winter Fuel Payments (proxy measure: State Pension)
- State Pension Transfers (proxy measure: State Pension)
- Cold Weather Payments (proxy measure: Jobseeker’s Allowance)
- Widow’s Benefit / Bereavement Benefit (proxy measure: Jobseeker’s Allowance)
- Industrial Disablement Benefit (proxy measure: Disability Living Allowance)
- Armed Forces Independence Payment (proxy measure: Disability Living Allowance)
- Christmas Bonus (proxy measure: General)
- Statutory Sick Pay (proxy measure: General)
- Statutory Maternity Pay (proxy measure: General)
Note: the General proxy is determined by all benefits which have ever been reviewed: Income Support, Jobseeker’s Allowance, Pension Credit, Housing Benefit, Disability Living Allowance, State Pension, Carer’s Allowance, Incapacity Benefit, Employment and Support Allowance, Universal Credit, Attendance Allowance and Personal Independence Payment.
Appendix 3: Further information on types of errors reported
The definitions of the key terms of Fraud, Claimant Error and Official Error are included at the start of this document. This section includes additional information on how we classify errors, including a detailed list of the types of errors we report for benefits reviewed in the current year.
Note that our methodology states that all errors (Fraud, Claimant Error and Official Error) found on a case are recorded separately and the full values of each error are recorded in isolation of one another. This can lead to the sum of the error values being higher than the benefit award. In such cases a capping calculation is performed (using a Fraud, Claimant Error, Official Error hierarchy) to ensure that the sum of the errors does not exceed the award, so that the monetary value of Fraud and Error is not over-reported. This can lead to some of the originally captured Fraud, Claimant Error and Official Error raw sample values being reduced during the calculation of the estimates.
In addition, it should be noted that an error which is initially categorised as claimant error, will instead be categorised as official error where the error has clearly been caused by an official of the Department/LA, and the ESA/CA/HB business unit (or, for PC, the pension centre) is in possession, from whatever source, of the true facts, regardless of whether the information has been processed by the business unit.
A Glossary of the current error types for overpayments and underpayments is given below
-
Abroad – claimant left Great Britain after claim began, did not notify DWP before leaving and is confirmed to be abroad for a period that exceeds any allowable absence limit. State Pension abroad errors are different in that being abroad does not remove their entitlement to the benefit, however the uprating of State Pension differs depending on what country you reside in
-
Award Determination – where a Case Manager from the DWP makes an incorrect award of PIP or AA based on the declared functional needs of the claimant. This includes failing to consider the qualifying period
-
Capital – concealed or incorrect declaration of the amount of savings in bank or building society accounts, cash, ISA/PEPs, premium bonds, other property interests or shares that exceed the minimum value for capital limits
Capital official errors include incorrect calculation by DWP staff of the value of declared savings, money and other financial assets available to the claimant, or failure to correctly adjust tariff levels and amend the benefit entitlement due.
-
Childcare Costs – Childcare costs incorrectly included or excluded or an incorrect declaration of the amount of childcare costs for the childcare element of UC
-
Conditions of entitlement – undeclared change in personal circumstances of claimant or their partner that would end entitlement to a benefit. Examples are being in full-time education, long-term hospitalisation, imprisonment, death and assuming a false identity
Includes staff failing to act on information received raising doubt on basic entitlement to benefit. For Universal Credit this includes the agent accepting the claimant commitment on behalf of a claimant who has the capacity required to accept their claimant commitment.
-
Control Activities – failure of staff to conduct actions at the due time which otherwise may have changed the level of benefit payable. For example, not conducting routine interviews where claimant non-participation can result in a benefit sanction or late notification of benefit disallowances
-
Earnings/Employment – concealment or under-declaration of full or part-time work undertaken during the claim by the claimant or their partner. This work can be for an employer or self-employment. Staff failing to correctly calculate the amount of monthly benefit due for claimants who have declared any paid work they or their partner have undertaken during the claim
-
Elements/Premiums/Components – Elements (UC only) – The award of UC is made up of a number of different Elements. Some of these are treated in this report in a similar way to Premiums in Legacy benefits, for example Carer Element, Disabled Child Element and Work Capability Elements. Child Care Costs and Housing Costs (also Elements in their own right) are recorded separately
Premiums: DWP can pay additional amounts in means-tested benefits when other benefits are also being paid, for example Disability or Carer’s allowances. This often introduces additional criteria for staff to consider before deciding the qualification for the extra amounts.
Components (SP only): The award of SP is made up of a number of different Components (for example Graduated Pension, State Second Pension, Additional Pension etc). This often introduces additional criteria for staff to consider before deciding the qualification for the additional amounts.
Elements and Premiums: Claimants also fail to report when the other benefit commences, or the rate payable changes or ceases, leading to an overpayment or underpayment of the benefit premiums.
-
Failure to provide evidence/Fully engage in the process – where the reason for the error is unclear. We are confident that the case is fraudulent, as the claimant has forgone their right to benefit, but with the evidence we have available we cannot be certain as to why
-
Functional Needs – where the claimant has failed to declare a change in their ability to carry out any of the activities on which PIP or AA is considered, or misrepresented their abilities when making their claim (whether intentionally or not)
-
Household composition – failing to disclose changes in household composition, for example a non-dependant leaving. In older claims where Tax Credits are not being claimed, claiming incorrectly for number of children (or their specific circumstances) which increases the value of DWP benefits payable, or claiming for adult partners who leave the household
Includes incorrect action by staff taken in respect of other people the claimant declared living with them. This includes a partner or dependent children incorrectly omitted or included in the assessment (with due regard to entitlement to any disability premium or benefit reductions due to long-term hospitalisation).
- Housing costs – DWP provides financial assistance with the costs of mortgages, ground rent and service charges. Claimants fail to disclose payment of or changes to housing costs, for example service charges, or fail to declare sale of property, or a change of address which would end the extra amounts payable. For Universal Credit this includes determining the housing cost element that replaces housing benefit, this can include calculation of the rent amount, correctly incorporating rent free weeks and size criteria
DWP can also inaccurately calculate the mortgage interest, ground rent or service charges, or incorrectly calculate awards of extra benefit to help with mortgage payments due to wrong start dates or by ignoring mortgage protection policies
- Hospital/Registered Care Home
Attendance Allowance (AA) is not normally payable for any period or periods of more than 28 days during which a customer is being maintained free of charge whilst undergoing treatment as an in-patient in a hospital or similar institution, or is resident in a care home where the Local Authority (LA) meets the costs of any of the qualifying services.
For UC couple cases, a person who is living away from their partner ceases to be treated as a member of a couple and part of the same benefit unit where they are absent from the household, or expected to be absent from the household for 6 months or more.
- Income - Occupational and Personal Pensions – concealed or incorrect declaration of income received from a non-state pension, obtained through contributions paid in past employment schemes, annuities or personal investments
DWP staff failing to take into account the correct amount of non-state pensions declared by the claimant.
- Income – Other benefits – concealed or under-declaration of income received from another benefit or tax credit.
DWP IT systems or staff have failed to take into account the correct value of other social security benefits currently paid to the claimant or partner, including benefits paid by a foreign state.
- Income - Other – concealed or under-declaration of income coming into the household from sources such as HMRC Tax Credits, sick pay from work, spousal maintenance, partner’s student income, unemployment or similar insurance policy payments
Failure by staff to correctly identify or record other money coming into the household, such as HMRC Tax Credits, Child Benefit, sick pay from work, spousal maintenance, partner’s student income, unemployment or similar insurance policy payments.
-
Labour Market Issues – lack of evidence of staff enforcement of jobseekers fulfilling legal requirements. These include actively seeking and being available for work, non-compliance with or absence of a valid Jobseeker’s Agreement, not imposing sanctions when employment is refused and not following up job submissions
-
Living Together – where a claimant declares to be single but has failed to declare they actually live with another person and maintain a joint household
-
Other – this covers a range of different cases not covered in the categories above or below
-
Passporting – relevant to Housing Benefit only. Working age claimants are required to inform the Local Authority paying Housing Benefit of any changes in their circumstances, including when DWP benefits end. Failure to do so can result in HB continuing to be paid
It includes communication failures between different IT systems that notify the termination of a claimant’s means-tested DWP benefit to the Local Authority, impacting the HB award, or LA staff failing to act on the information received.
-
Residency – errors relating to Housing Benefit claimants only, where DWP confirms that the claimant no longer lives at the address being paid for. For Universal Credit, where the claimant no longer lives at the address for which they are being paid Housing Costs Element, the error would appear in Housing Costs
-
Tax Credits – errors where the amount or existence of tax credits results in an incorrect award of a DWP benefit
Appendix 4: Glossary of statistical terms
Key statistical terms used in this report are explained below.
-
95% Confidence Interval: The range of values within which we can be 95% sure that the true value we are trying to estimate lies. It is used as a measure of the statistical uncertainty in an estimate
-
Estimate: An estimate is an indication of the value of an unknown quantity based on observed data. It provides information about unknown values in the population that we are trying to measure
-
Population: A population is any entire collection of items from which we may collect data. It is the entire group that we are interested in, which we wish to describe or to draw conclusions about (generally benefit claimants or expenditure in the context of this report). There are two different types of population referred to in sampling:
-
The Target Population – consists of the group of population units from whom we would like to collect data (e.g. all people claiming a benefit).
-
The Survey Population – consists of the group of population units from whom we can collect data (e.g. all claimants with sufficient case details on our datasets). The Survey Population is sometimes referred to as the ‘Sampling Frame’.
-
-
Sample: A group selected (randomly in the context of this report) from a larger group (known as the ‘population’). Through analysing the sample, we aim to draw valid conclusions about the larger group
ISBN 978-1-78659-423-5