Intellectual property and investment in Artificial Intelligence
Published 4 July 2022
Executive summary
This study commissioned by the UK Intellectual Property Office (UKIPO) investigates important factors influencing decision-making on UK investment in Artificial Intelligence (AI). In particular, it analyses the extent to which this is influenced by the UK intellectual property (IP) framework currently in place.
Definitions of investment, market applications and AI applications were established. This provides a scope for the study and a rationale for sampling views across a range of relevant actors in the sector.
We gathered information from different sources. We first reviewed the literature on AI and AI investment. We then conducted a series of face-to-face stakeholder interviews, facilitated by a prepared set of questions, during the period from November 2021 to January 2022. We also benefited from discussions with several members of the UKIPO team and other parties already engaged in related UKIPO studies on IP and AI.
The conclusions from the literature survey are summarised below.
-
the UK is number three in the world as a destination for private investment in AI (behind United States of America (USA) and China) and number one in Europe in 2020 (Zhang, et al., 2021);
-
UK-based applicants and inventors rank sixth in terms of patenting activity measured by the number of AI related patent families (UKIPO, 2019)
-
London is the main hub in Europe for AI development activity
-
the UK has a large pool of AI and software talent, with important research institutes focused on AI research and development (R&D)
-
the UK shares the advantage of being naturally English-speaking with the much larger USA ecosystem
-
the UK is good at developing AI solutions but is lacking in investment and AI company growth beyond the start-up stage. The stakeholder interviews provide some elements of response on this
The face-to-face interviews provide additional conclusions, summarised below.
-
the UK IP Framework is not a fundamental consideration for investment by the participants in this study
-
other factors dominate investment decisions: the availability of AI-skilled people; the reputation of technology hubs and universities; government grants and access to early-stage funding. Overall, the relative strength of these factors encourages AI investment in the UK
-
AI companies have an internationally dispersed workforce from the start-up phase onwards. This is determined by the need to find AI skills anywhere in the world. Opportunities for home working on software development arise for international graduates returning to their home countries
-
the UK IP framework is broadly regarded as trusted and reliable and not of concern in investment decisions, perceived as a background hygiene factor. However, it was noted that early-stage AI start-ups lacked experience of using the UK IP framework
-
investors typically require patents to support investment decisions. Patents provide third-party opinions on the uniqueness of the technology and a ‘saleable asset insurance’ in the event that the company ceases trading
-
technology sector participants had a generally perceived misconception that ‘core AI software is not patentable’. A similar observation was made in a recently published DCMS and OAI commissioned report on AI (Westgarth, et al., 2022). This report also notes that the value of AI is derived from its application to solve an existing problem. Yet, patent protection for AI-related inventions is available across all technology areas. Patent inventions embodying algorithms or software are allowable if they make a technical contribution. The UKIPO, like the European Patent Office (EPO), grants many thousands of patents for computer and software implemented inventions including in the field of AI (UKIPO, 2019). Thus, they do provide protection for innovators in this field. The UKIPO’s Manual of Patent Practice provides extensive guidance on the patenting of software and computer implemented inventions
-
as start-ups grow into the small and medium enterprise (SME) category, understanding of patents and copyrights improves, but understanding of the IP framework for trade secrets remains weaker
-
there appear to be two communities of interest using and/or developing AI technology and the UK IP framework. The technology sector community tends to focus on patents and relies on patent attorney firms while the media sector is served by copyright solicitors
-
currently there is a wide gap between these communities with little mixing of expertise. Integrating these two areas of professional expertise would help early-stage AI companies develop commercial strategies to realise the full potential of their key assets in terms of protection, fundraising and exploitation
The UK has established a strong reputation as a place to incubate AI businesses. However, it is seen as too small a market to grow large companies and to secure the larger investments needed for major long-term growth. Many young companies plan an early exit or a move to the far larger USA market with its reputation for risk-taking and large investment. The UK too often loses the larger-scale economic benefits of AI business growth to larger economies. Challenges in scaling up for UK innovative businesses is also highlighted in the Evidence paper of the UK Innovation Strategy (BEIS, 2021).
Abbreviations
-
AI - Artificial Intelligence
-
BEIS - Department for Business, Energy, and Industrial Strategy
-
CEO - Chief Executive Officer
-
COVID-19 - Coronavirus Disease caused by the SARS-CoV-2 virus
-
CTO - Chief Technology Officer
-
DCMS - Department for Digital, Culture, Media, and Sport
-
DTSA - Defend Trade Secrets Act
-
EPFL - École Polytechnique Fédérale de Lausanne
-
EPO - European Patent Office
-
EU European Union
-
GDP Gross Domestic Product
-
IBM International Business Machines
-
IP - Intellectual Property
-
IPO - Intellectual Property Office
-
IT - Information Technology
-
M&A - Mergers and Acquisitions
-
ML - Machine Learning
-
NLP - Natural Language Processing
-
OECD - Organisation for Economic Co-Operation and Development
-
ONS - Office for National Statistics
-
PCT - Patent Cooperation Treaty
-
PE - Private Equity
-
R&D - Research and Development
-
SME - Small and Medium Enterprise
-
UKIPO - UK Intellectual Property Office
-
USA - United States of America
-
USPTO - United States Patent and Trademark Office
-
WIPO - World Intellectual Property Organization
-
VC - Venture Capital
Introduction
The principal aim of this report is to investigate the current determinants in investment in AI worldwide and the role of IP. For growing companies in the UK, access to funding can be a major hurdle (Office for National Statistics (ONS), 2019). Furthermore, it is unclear whether the use of IP and the ability to protect innovative ideas serves as an attractor for investment.
This study aims to understand whether the UK IP framework plays a role in incentivising investment in AI technology and AI businesses in the UK. It also looks to understand the role of other factors in investment decisions in the UK relative to the IP framework.
We regard the IP framework as encompassing not only the formal framework as defined by the UKIPO and to a lesser extent the EPO, but also other wider dimensions. These dimensions include the related legal and commercial services profession and the judicial/legal enforcement framework in place for the protection and litigation of IP rights. It also accounts for the wider IP education and training ecosystem.
However, it should be noted that AI applications, products and services are international with global reach and opportunity beyond the UK.
We also examine the question of access to training datasets for AI models and the availability of such data for companies of different sizes and sectors. Furthermore, we consider how labelling or other modification of the data could potentially have an implication on its ownership.
Background and previous studies
The advent of AI is significantly changing the way we do business. It is reshaping how society as a whole works and it will continue to induce potentially major changes for years to come. AI has become an integral part of many modern production and manufacturing processes. In this setting, large amounts of data are being combined with extreme computing power to lead to improvements in efficiency, capacity, and output. To encourage implementation and adoption of AI based solutions, government policies must promote a trustworthy AI ecosystem and responsible AI R&D activities (Organisation for Economic Co-Operation and Development (OECD), 2019).
In this light, many governments worldwide are developing AI Frameworks. The UK Government National AI Strategy was recently announced and published by the Office for Artificial Intelligence (Department for Digital, Culture, Media, and Sport (DCMS), 2021). Such a nationally endorsed ecosystem supports SMEs in transitioning to an AI-focused environment and ensures that they remain competitive both within the UK and globally. This conversation extends to the role played by the IP framework, regulatory framework and financing in its wider Innovation Strategy (Department for Business, Energy, and Industrial Strategy (BEIS), 2021).
The UK government recognises the importance of AI to the UK economy through the establishment of several AI focused institutions. These include the Office for AI (OAI), a BEIS and DCMS joint initiative, and the AI Council, an independent expert committee providing an advisory role. The government also commissioned several reports, including the AI Roadmap (UK AI Council, 2021) and the National AI Strategy (DCMS, 2021) developed by OAI.
Furthermore, there has been significant parliamentary interest in this topic, as indicated by the Select Committee report (House of Lords, 2018). The work to date has not focussed attention on the potentially important area of IP, which may provide incentives for investment. IP, together with an enforcement regime, may influence investment by offering legal protection for innovation.
Questions to be addressed in this study
The UKIPO has the following aims and objectives in relation to this requirement.
- review the literature on the determinants of investments in AI, and the role of IP
- identify main actors of the AI sector in the UK and abroad and the nature of their contribution
- assess the determinants of AI investment
- provide evidence on whether IP plays a role in incentivising AI investment in the UK
- reflect differences between IP rights
How we define AI for the purpose of this study
We have considered the definition of AI for the purposes of this study, particularly with regards to the topic of investment. There are several definitions of AI (Council of Europe, n.d.; Wikipedia, 2021) that evolved over time and with the context in which it is considered.
-
Turing Test: The only thing that matters is the external behaviour of the machine. Does machinery show intelligent behaviour? (Turing, 1950)
-
John McCarthy: “Every aspect of learning or any other feature of intelligence can be so precisely described that a machine can be made to simulate it”. (McCarthy, 2011)
-
John R Searle: “The appropriately programmed computer with the right inputs and outputs would thereby have a mind in exactly the same sense human beings have minds.” But “even if a machine perfectly simulates human behaviour, there is still no reason to suppose it also has a mind.” (Searle, 1980)
For example, AI has been described as intelligence demonstrated by machines, as opposed to the natural intelligence displayed by humans or animals.
In the UK Government Industrial Strategy White Paper (BEIS, 2017), AI and the data-led economy are defined in the following terms.
Artificial intelligence (AI) is a set of technologies with the ability to perform tasks that would otherwise require human intelligence. These include visual perception, speech recognition, and language translation.
Machine learning (ML) is a type of AI that allows computers to learn rapidly from large datasets without being explicitly programmed. In many cases, the adoption of ML technologies brings benefits of improved productivity and cost-savings for a business.
Data-led economy a digitally connected economy that realises significant value from connected, large-scale data that can be rapidly analysed by technology to generate insights and innovation.
The 2018 House of Lords Select Committee on AI adopted an enhanced version of the above definition. It added: “that AI systems today usually have the capacity to learn or adapt to new experiences or stimuli”. We have taken this as a useful basis in adopting a definition appropriate for this report.
There is long-standing debate on whether machines can truly be intelligent. However, what matters here is whether a machine can simulate intelligence to the degree necessary to provide a particular set of functional capabilities.
There are many elements involved in what is considered to be intelligence, including abstraction, self-awareness, learning, planning, problem solving and critical thinking. In the context of an AI some of these are much more challenging to achieve at any point in time than others. Intensive R&D has been making, and continues to make, rapid progress tackling these challenges and realising solutions. AI development and its applications relies on the simulation of many elements traditionally contributing to intelligence at any given time to solve a problem.
AI has often been categorised on a scale from Narrow (or ‘weak’) to General (or ‘strong’) AI.
-
Narrow (or ‘weak’) AI
Narrow AI, which typically performs a single task or a set of closely related tasks, is the most common form of AI. Examples include weather apps, digital assistants, or data analysis to optimise a particular business function.
Although the functionalities of the AI in applications may be narrow and may focus on increasing efficiency, they have the power to transform systems. Developments continue to influence how businesses work and how we live on a global scale. Powerful examples include machine learning for the analysis of medical data, medical imaging, fraud detection, and driver behaviour monitoring and prediction. Recently, UK-based Monolith AI, together with Imperial College London, is using AI to determine the manufacturability of metal components (The Manufacturer, 2022).
Often, when a technique reaches mainstream use (e.g. optical character recognition), it is no longer considered as AI; this phenomenon is described as the ‘AI effect’.
-
General (or ‘strong’) AI
General AI, which relies on sentient machines emulating human intelligence, thinking strategically, abstractly, and creatively, is still in its infancy. In principle, General AI has the ability to handle a range of complex tasks and perhaps demonstrate empathy. While machines can perform some tasks better than humans (e.g., data processing), this fully realised vision of General AI does not yet exist in reality (Müller & Bostrom, 2016; Grace, et al., 2018; Gruetzemacher, et al., 2019; Avin, 2019). That is why human-machine synergy is crucial in today’s world, as AI remains an extension of human capabilities, not a replacement.
AI development sector and patenting trends
We note also that the (House of Lords, 2018) report refers to the “AI development sector, rather than an AI sector, on the grounds that it is mostly a particular sub-group within the technology sector which is currently designing, developing and marketing AI systems, but multiple sectors of the economy are currently deploying AI technology, and many more will likely join them in the near future.”
We have taken a similar view here. Rather than focus specifically just on end applications, we look also at the landscape of AI functionality developments, one stage back from the end applications. This is a space in which important tools and platforms are being developed and modules on which end-user applications are built. AI functionality platform examples include:
- computer vision platforms
- video recognition
- smart robot technology
- gesture control
- speech recognition
- speech translation
- machine learning platforms
- natural language processing
- virtual assistants
- recommendation systems
- context computing
A 2019 WIPO report shows that ML is the predominant AI technique used in over a third of AI patents filed worldwide (WIPO, 2019). This AI field has also experienced continued growth. The main areas of application are telecommunications (15%), Life Sciences (12%) and personal devices/computer-human interactions (11%). Telecommunications is a field of significant application of AI technologies. The main players focus on different AI fields and applications. Baidu focuses on deep learning, Toyota and Bosch on transportation and Philips and Siemens on life sciences. Whilst China figures highly overall, Chinese Universities also stand out. 17 of the top 20 academic players that patent are based in China (WIPO, 2019). Almost a quarter of peer-reviewed AI publications in 2019 originate from China alone (Zhang, et al., 2021).
There was 400% increase in the number of published AI patent applications worldwide over the last decade . There was a doubling of patenting activity by the UK AI sector over the same period, with 88% of AI patents first filed in the UK also protected elsewhere. In contrast, the USA and China had 53% and 19%, respectively, of patents protected elsewhere. UK-based applicants and inventors are ranked sixth worldwide in terms of the absolute level of AI patenting activity. Overall, there are more US-based applicants filing for AI-related patents in the UK than UK-based applicants. Technology related to neural networks has shown significant growth across the world over the past five years. The UK in particular has seen larger proportional increases than the global trends in AI-related technologies related to transport, image processing and telecommunications.
AI development stage
For the purposes of this report, we focus on AI technologies with good potential for economic effect and at a stage of early application requiring financial investment. Areas for high potential include applications of ML, computer vision, and natural language processing (NLP) as indicated in the chart below (Figure 4.1). This chart shows that a wide variety of AI technologies are applied across a wide range of industries. However, it is clear that generally speaking the most widely used forms of AI, irrespective of industry, are ML, computer vision and NLP.
Figure 4.1 Patent families by co-occurrence of application field with AI type (Source: 2019 WIPO Technology Trend report on Artificial Intelligence
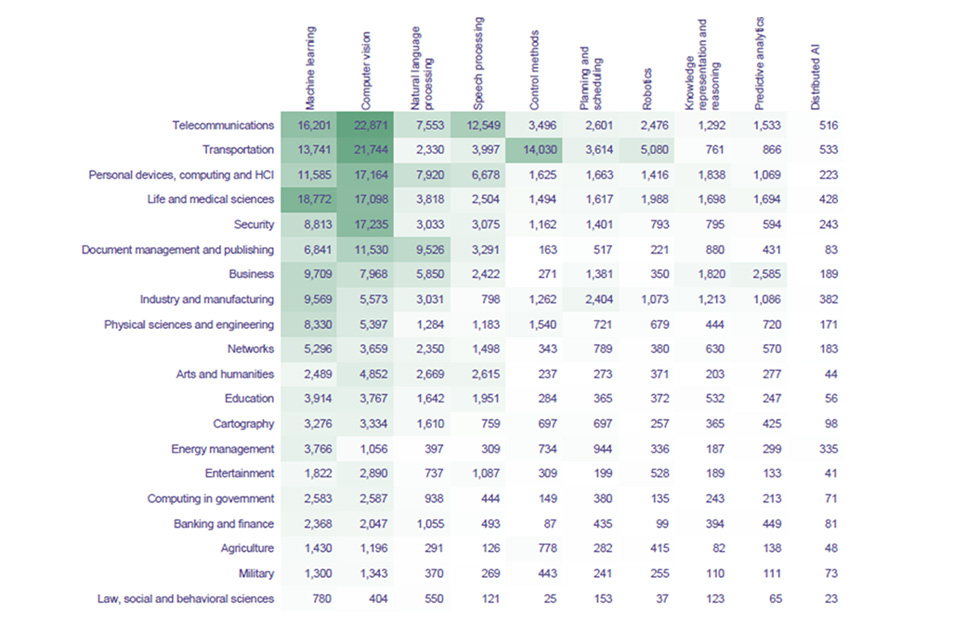
We note that what is considered AI technology (given the ‘AI Effect’) is itself a moving target. We focus on mature AI technologies that have become routine leaving aside future potential technologies. We thus retain a focus on the near-term applications that provide technical and economic advantage.
IP and intangible assets
IP in the UK is most frequently understood in terms of the legal rights available related to copyright, designs, patents and trade marks. However, within the commercial world of IP, wider intangible assets are frequently discussed. These may include registered and unregistered IP rights, as well as other assets (branding, software and training). The value of intangible assets is often poorly reflected on a company’s balance sheet (Haskel & Westlake, 2018).
In the context of this report we consider registered and unregistered IP to include the following:
Table 5.1 Overview of Intellectual Property Rights
Registered rights | Unregistered rights | Data/Database rights |
---|---|---|
Patents | Unregistered trade marks | Data* |
Utility models | Unregistered designs | Databases |
Registered trade marks | Copyright | |
Registered designs | know-how | |
Trade secrets |
The table above outlines both registered and unregistered IP rights, including database rights. While data in its pure form as facts, figures and information is not protectable as an IP asset, the collection of such data or independent works in the form of a database, is afforded database right protection under the Copyright and Rights in Databases Regulations 1997. Software code has copyright protection. Not all software and algorithms may be patented in the UK but software and algorithms or other similar mathematical methods are patentable if they provide a technical effect. Historically the US has taken a less restrictive approach to patent exclusions in this area compared to the UK and Europe. In an AI context, novel applications of AI may be patentable in the UK as well as basic functionalities but only if they provide a technical contribution
Improvements in productivity and automation also allow for improved use of human capital, whereby a highly educated workforce is refocused on higher value activities. A 2019 ONS report indicates that the largest capitalised intangible asset in the UK is computer software and databases (ONS, 2019). Investment in computer software accounted for a total investment of almost £31 billion in 2019. AI represents a significant subsection of this total. A recent DCMS report found that in 2020, 432,000 companies in the UK spent a total of £16.7 billion on AI technologies (DCMS, 2022). It also shows that average spend per business increases with size.
Current trends in investment in AI
Global trends in AI investment
Globally, pressure to adopt and develop new AI technologies increases, with AI incorporation seen across many industries (Judah, 2018; Mckinsey Digital, 2020; Business Insider, 2017). Businesses need to make investments in internal infrastructures to see significant cost savings relating to increased productivity or increased business opportunities related to AI adoption. Large multinationals in the pharmaceutical, biotech, automotive (Kearney, n.d.) and financial sectors (OECD, 2021) are also making significant investments in AI (Mckinsey Digital, 2020). Investments are made both internally and externally. AI start-ups are targets for rapid acquisition by known AI developers such as IBM but also by other industries such as pharmaceuticals and insurance companies. AI can not only offer significant cost savings in productivity but also open new business opportunities. For example, UK-based Deepmind was acquired by Google in 2014, Mellanox by Nvidia in 2019, and Swedish-based Vi-Health by UK-based Numan in 2021 (Mobihealthnews, 2021). At the same time, many successful UK AI companies have entered into development agreements with industry. For instance, AI-led pharmatech company Exscientia recently completed an IPO on Nasdaq with $510m in financing. The company also has agreements in place with Sanofi (Exscientia, 2022) and Bristol Myers Squibb (Exscientia, 2021).
A 2019 Gartner report based on a CIO Agenda survey showed that up to 14% of large corporates use AI in some form (Gartner, 2019). This survey gathered data from over 3,000 respondents across 89 countries and all major sectors in 2018. A 2020 McKinsey survey noted that approximately 50% of businesses surveyed adopted an AI functionality (Mckinsey Digital, 2020). This survey collected data from 2,395 respondents across companies of different sizes, industry, speciality and in different countries. The main areas of application of AI in such cases are chatbots, process optimisation, and fraud analysis across the insurance, software, retail, and IT sectors. Those already actively using AI are more likely to allocate more of their digital budgets for its continued use (Mckinsey Digital, 2020).
AI-related companies have on average higher valuations and raise more in individual investment rounds compared to others (MMC Ventures, 2019; IFC, 2020). However, the effectiveness of these investments can be improved by better guidance from governments and regulatory authorities on the design and implementation of AI technologies.
AI can be used in practically all industries and at many points along the value chain. Consequently, AI has attracted significant venture capital (VC) investment. Between 2012 and 2017, VC investments in AI-related venture activities rose from approximately US$600 million in 2012 to over US$14 billion in 2017 (IFC, 2020). There has been heavy investment in ML technologies across multiple industries (IFC, 2019). This is because ML is the most developed and mature AI field ready to be applied to existing products or services. This is also reflected in patent filing data (WIPO, 2019; UKIPO, 2019) and scientific publications (Zhang, et al., 2021).
There has been a significant increase in global investment in AI in the last few years. As indicated below in Figure 6.1, it has been estimated that global corporate investment in AI reached almost US$ 68 billion in 2020 (Zhang, et al., 2021).
Figure 6.1 Global corporate investment in AI 2015-2020; Source Statista and Artificial Intelligence Index Report 2021 (Zhang, et al., 2021)
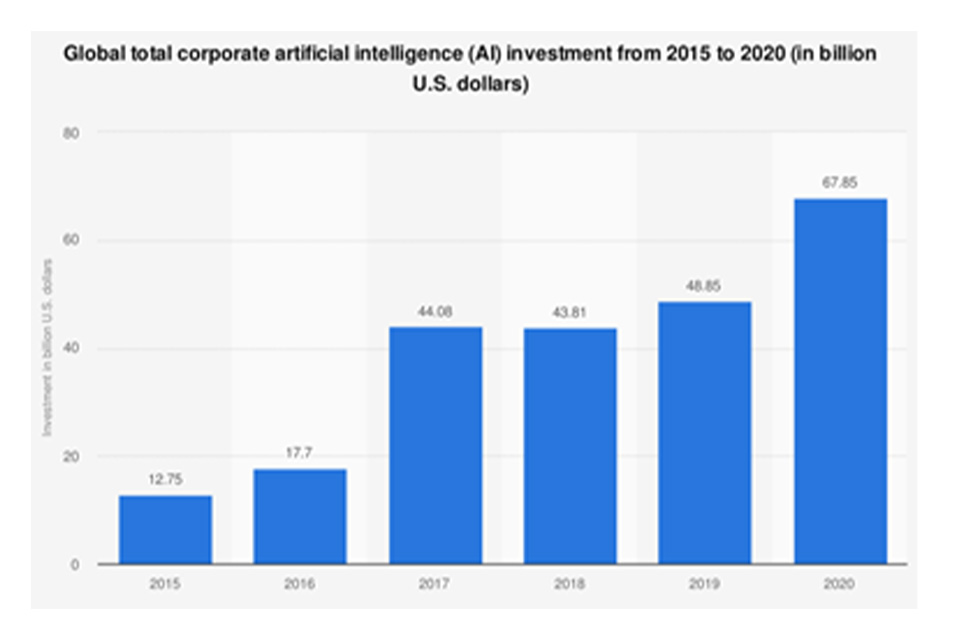
China and the USA lead investment in the AI space (Figure 6.2). There is very little investment in emerging markets, with the exception of India, although most studies have looked at developed economies (IFC, 2020). Total investment in AI in China reached CNY 175 billion in 2020 (about GBP 20 billion) from CNY 101 billion in 2019 (about GBP 11 billion). Average investment value increased from CNY 151 million (about GPB 17 million) to CNY 266 million (about GBP 29 million) (Statista, 2021).
Figure 6.2 Private Investment in AI in 2020 by country - Source: Artificial Intelligence Index Report 2021
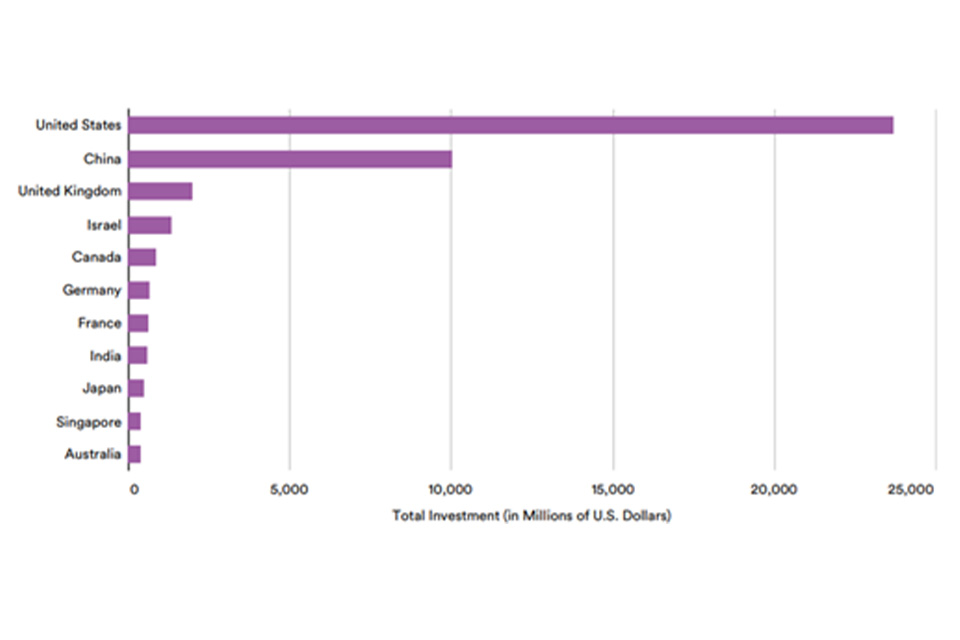
Figure 6.2 above suggests that China is behind the US in terms of total private investment in AI in 2020. However, the Chinese government investing heavily in AI R&D at both a local and national level. Consequently, a significant amount of investment in AI in China will come both directly and indirectly from government.
Average investment size in China is higher than the USA. US AI start-up businesses raised around US$4.4 billion from 155 investments, while in China a similar amount was raised in 19 investments (ABI Research, 2017). A Chinese Governmental Statement “A Next generation artificial intelligence development plan” includes an IP strategy for AI (Chinese State Council , 2017; New America, 2017; Nesta, 2017). This strategy provides certainty and confidence for businesses to act and begin investing in AI. Since 2017 China set the goal for significant breakthroughs by 2025 and world leader position in AI by 2030 (South China Morning Post, 2018). China has had a head start on both AI-related research and patenting (Figure 6.3). The former Pentagon chief software officer stated that the USA “will have no competing fighting chance against China in 15-20 years” (van der Merwe, 2021).
Figure 6.3 Geographic breakdown of origin of global AI journal publications 2000-2020. Source: Artificial Intelligence Index Report 2021 (Zhang, et al., 2021)
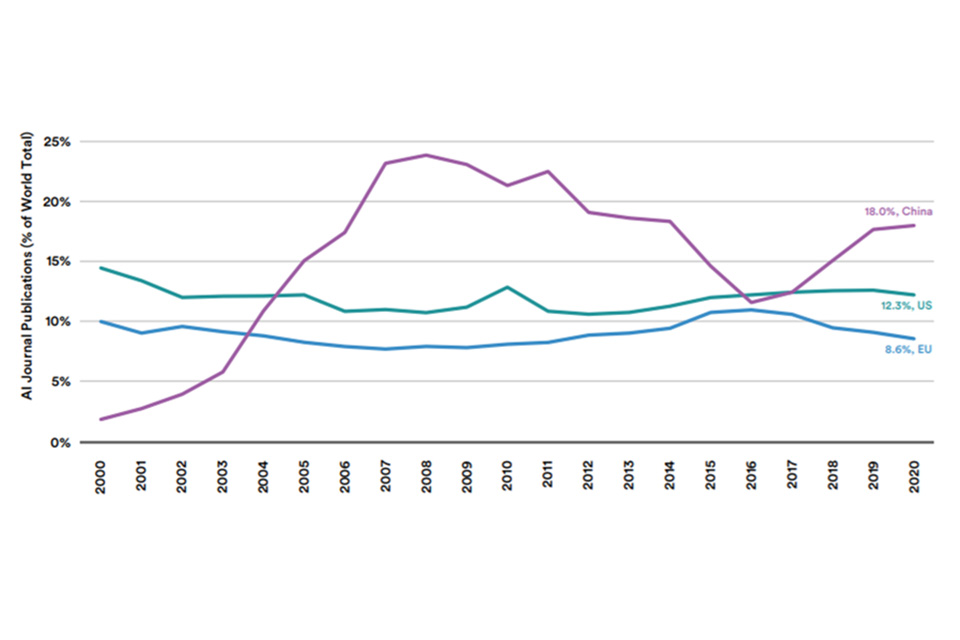
AI investment in the USA, China and the EU accounted for over 93% of the total private equity investment in AI between 2011-2018 (OECD, 2019). In this period, UK-directed investment in AI accounted for a total of 55% of total European investment. On the basis of investment as a percentage of GDP, the USA leads at 0.12%, with China and UK at 0.085% each.
Globally, the main AI hubs for development are Silicon Valley, New York, Beijing, Boston, London, and Shenzhen. Silicon Valley is the top hub for start-up businesses and is the seat for many VCs investing in this area.
Although India is the second largest emerging market, it previously lagged behind other markets such as China. However, this may be changing as it has been anticipated that investment in AI in India will reach approximately US$1 billion by 2023 (Tejaswi, 2021).
As it stands, India ranks in 8th position in terms of private investment in AI, behind Europe and particularly the USA and China. However, a recent State of AI report (Deloitte , 2021) suggests that most Indian businesses plan to increase their AI spend. This report is based on a survey of over 300 senior executives in India. The report shows that Indian start-ups are seeing rapid returns on earlier investments, giving them a competitive edge. Businesses in the retail & consumer sector, telecoms and financial services sectors demonstrate high levels of AI adoption. However, those in the pharmaceutical and manufacturing sectors, as well as hospitality, are lagging behind in their AI adoption. Furthermore, India is recorded as one of the countries experiencing highest growth in AI hiring from 2016-2020, as well as the highest AI skills penetration rate, ahead of both US and China, in second and third position respectively (Zhang, et al., 2021). A 2021 report estimates that AI has the potential to add US$957 billion of additional gross value to India by 2035 (Accenture, 2021). In 2019 the Indian government formed a committee to push for development of a formal AI policy and strategy to achieve India’s AI ambitions (Zhang, et al., 2021).
Looking at academic research in AI, China has taken a clear lead on developing AI-based innovations in universities. In 2019 over 100 of the top 500 AI-focused academic patent applicants worldwide were from China (WIPO, 2019). 20 were from the US and Korea, and four from Japan and Europe. However, while China leads in applied areas (facial recognition and NLP), it lags behind in hardware development required to build this AI ecosystem. As a result, China currently relies on western companies such as Nvidia, Arm, Intel, AMD as well as numerous earlier stage AI chip producers. To date the main challenger from China on AI chip production has been Baidu.
Between 2019 and 2020, there was a move towards applications in drug discovery, autonomous vehicles, gaming educational applications (Figure 6.4). These are likely to have grown due to the effects of COVID-19 and the associated global lockdowns on working habits, leisure activities and economies.
Figure 6.4 Change in focus for AI application fields between 2019 and 2020. Source -Artificial Intelligence Index Report 2021 (Zhang, et al., 2021)
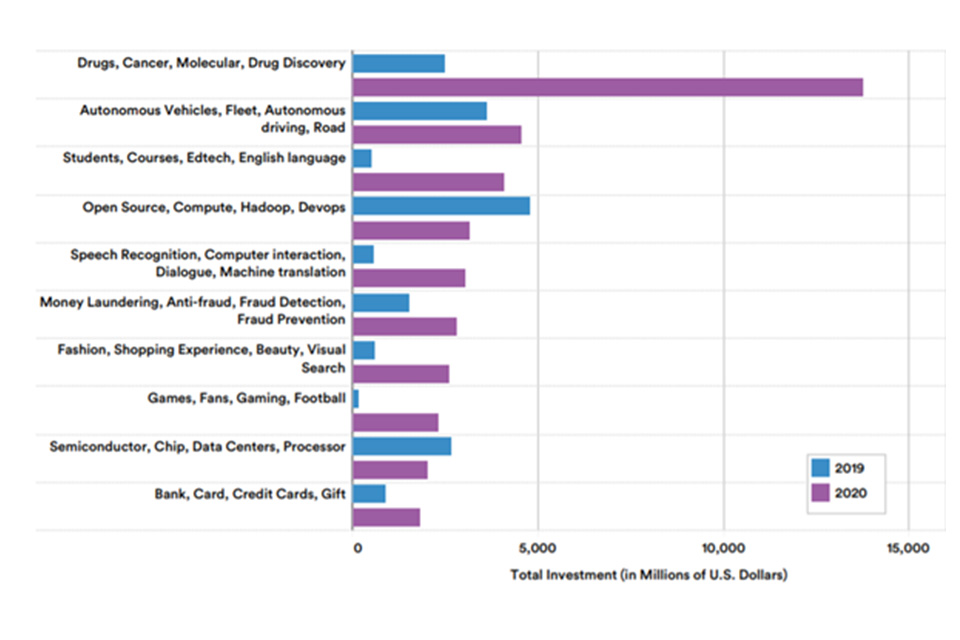
AI in the UK
The UK is above average in its AI readiness level (Bughin, et al., 2019; McKinsey & Company, 2019; Digis, 2021). This index relies on seven AI enablers. Three are AI-specific: AI research activities, AI startup investment and automation potential. The remaining four are foundations for AI: digital absorption, innovation foundation, human capital and ICT connectedness. Overall, the UK is doing relatively well in terms of AI research activities, AI startup investment, digital absorption, innovation foundation and ICT connectedness. However, it is lagging behind in terms of automation potential and human capital. The UK is also a leader in the AI rollout in Europe along with Scandinavia. It has been estimated that, by 2030, wider implementation of AI in the UK could result in a 22% boost to the UK economy (current GDP). The UK was ranked second behind Singapore in terms of readiness for AI adoption in a government AI readiness index (Oxford Insights, 2019). However, significant investment is still required, especially in scaling up its current digital assets and competencies. The study found that the relative readiness of the UK in the roll out of AI is dependent on seven enablers, namely:
- current research activities
- availability of start-up investment
- potential for automation in industry
- digital absorption
- innovation foundation
- human capital
- ICT
However, the study also found that while the UK has good native research in AI, investment is still lower than in China and the USA. Furthermore, adoption by UK businesses remains low. In other words, the UK is good at developing AI solutions but is lacking in the wider scale adoption of these solutions (Oxford Insights, 2019).
A report commissioned by DCMS found that around 15% of UK businesses (totalling 432,000) have adopted at least one AI technology. 2% are currently piloting AI and 10% plan to adopt at least one AI technology in the future (DCMS, 2022).
This report showed that AI solutions for data management and analysis are most prevalent in the UK, with 9% of firms having adopted them. This is followed by NLP and generation (8%), NLP (7%), AI hardware (5%), computer vision and image processing and generation (5%). The IT and telecommunications and legal sectors currently have the highest rate of adoption, respectively 29.5% and 29.2%. The sectors with the lowest adoption rates are hospitality (11.9%), health (11.5%), and retail (11.5%).
It also showed that large companies are more likely to adopt multiple AI technologies in the UK. However, there are innovative companies using multiple AI technologies across all size categories. Larger companies are more likely to use four or five AI technologies to assist in their business activities.
The level of investment in AI in the UK, mainly through VC, PE and M&A activity, is higher than elsewhere in Europe, the number of AI patents filed in the UK is lower than in France and Germany (Bughin, et al., 2019). However, the fact that UK AI activities are typically early stage may be related to this. Earlier concept and development stage AI innovations are predominantly focused on the software and algorithms. As such, they are more easily protected by copyright or trade secret. Later stage AI applications will have a technical effect and, in this respect, can generally be protected by patents.
The AI business sector tends to be dominated by the large multinationals. The majority of high-volume patent assignees are based in the USA (Microsoft, Alphabet, IBM, Facebook, Yahoo, Intel, Amazon, Apple and Qualcomm) (Cowan & Hinton, 2018). They are followed by Japan (Sony, Fujifilm, NEC and Xerox), the European Union (Siemens and Philips) and finally Korea (Samsung).
In 2017, DCMS and BEIS commissioned an independent report on the growth of AI in the UK (Hall & Pesenti, 2017). The report provides recommendations on the use of IP by universities and research institutes generating AI related inventions. The ability to transfer the inventions and IP needs to be improved. This would ensure that universities are not only seeking to protect AI inventions but also actively seeking to commercialise and monetize these. In this context, the review recommends standardisation of spin-out activities and common licensing practices. Furthermore, there may be ways of further incentivising corporate investors at an earlier stage by co-developing criteria and terms.
The development of a targeted National AI Strategy (DCMS, 2021) by the UK government is seen as a timely and positive step. This is in line with similar strategies being announced elsewhere in Europe (Die Bundesregierung, 2020; Rijksoverheid, 2019; Government of Ireland, 2021). However, there are still fundamental issues to be resolved for the UK to become an AI “superpower” within ten years (Hayes, 2021). These include an increased openness from AI suppliers about how the core IP works.
The recent UK National Data Strategy consultation is also relevant in an AI context (DCMS, 2020). It sets out core pillars for best use of data in the UK, which include data quality, skills, availability and its responsible use. However, some reports question whether the UK’s aim to become an AI superpower goes against important AI regulation on data control (Verdict, 2021). Article 22 of the GDPR regulation, covering regulation of AI, was incorporated into UK law through the Data Protection Act 2018. But there are concerns that following exit of the UK from the European Union, aspects of this regulation may be modified or removed (Pierides & Roxon, 2021). This would prioritise innovation above regulation. EU regulation and legislation surrounding the implementation of AI and data sharing are seen as fundamental parts of AI innovation and growth (European Commission, 2020).
The Alan Turing Institute, the British Standards Institution (BSI) and the National Physical Laboratory (NPL) will lead a new government initiative (DMCS and OAI, 2022). The aim of this initiative is to develop AI global technical standards and practical tools for businesses to roll-out AI innovations. This would thereby contribute to placing the UK in a leading position in the global adoption of AI.
Who are the main actors and actor types in relation to investment in AI
The different actor types listed below have different levels of involvement in the AI ecosystem. Some businesses receive investment to develop an AI system for their own use or for others. Others make the investment internally for developing an AI capability. Likewise, external institutional investors may invest in a wide range of AI businesses, across multiple points of the value chain and across multiple application areas. The relative importance of AI for individual businesses also changes across actors. For some it will be the main product or service they produce. For others, the AI stack may simply provide a small part of a much larger software-led offering, including other cutting-edge technologies. For the purpose of simplicity, we have grouped actors into a small number of general clusters recognising that there may be overlapping between, and sub-groups within, clusters.
We identify four types of investors in AI:
-
government investment, which for this report we assume to be led by factors other than the IP framework (as government defines the framework through legislation). However, the output of academic investment by government bodies will be considered together with IP-related requirements for investment or grant funding
-
companies making direct internal investment in products or services to create AI-related functionality by using their own internal resources
-
companies making external investments, where they acquire products or services from third parties, have venture funds, or fund external research
-
professional Investors such as venture capital or seed funding investors, where the investment is primarily for financial gain
We seek to understand whether investment decisions are affected by the perceived ability to protect or secure them through the current UK IP framework.
In addition, investment can be considered along the AI component value chain as investors may invest in one or more of the components of AI. We identify six components within the AI value chain:
1. Chips and hardware. Companies developing semiconductor chips that support AI applications (examples would be Intel and Qualcomm).
2. Platform and infrastructure. A hardware architecture or software framework that allows AI software to run (such as Google’s AI platform, Microsoft Azure and TensorFlow).
3. Frameworks and algorithms. AI frameworks allow faster and easier development of AI applications (examples would be Microsoft CNTK and Caffe).
4. Enterprise solutions. A category of enterprise software that harnesses advanced AI techniques to lead digital transformation (examples would be Digital Genius and Afiniti).
5. Vertical Industry Solutions. Vertical (specific market sector) AI solutions (in specific market sectors) are about seeking to unlocking entirely new opportunities rather than just optimizing existing opportunities. Examples would be: Benevolent AI in healthcare; Zest finance in finance and insurance; and FarmersEdge in agriculture.
6. Corporates. Large corporations (such as Ford and Boeing in automotive and transport; and Disney in retail and media).
We also considered whether the type of AI application (see 4.1) may provide a third dimension. However, we found no evidence from our interviewees that this factor made any relevant difference.
In summary, investment determinants, including the IPO framework, are likely to be dependent on combinations of the type of investor and the target market sector. For this reason, our interviews covered a range of different types and sectors.
A recent study by Deloitte (Deloitte Development LLC, 2021) analyses MedTech start-ups, many of which incorporate AI capabilities. The study shows that early stage investment is mainly comprised of founder self-investment, friends & family and angel investors. VCs and institutional investors or corporate venture arms play a more marginal role. Venture Scanner data (2019) showed that most VC investment focuses on ML. A record high $44bn had been invested by Q3 2021 with 72% at seed and early stage. There have also been record exits in 2021 of 94 AI companies by Q3.
Drivers of investment in AI
Skills and talent
An important determinant for AI development in the UK is the large pool of AI and broader software talent available. This is partly due to the success of the UK in attracting talent from other countries –being a desirable destination for those developing AI innovations. In 2019, the UK had the second largest number of software developers in Europe (15% of the total). London is the largest technology hub in Europe. Importantly, the UK has an additional benefit in attracting innovators in the AI space, namely by being an English-speaking country. When US developers enter the European market, they typically move to the UK (Bughin et al., 2019). This has been further strengthened by the foundation of a new AI Council in 2019, which established doctoral training activities in AI throughout the UK. Similarly, the UK counts important research institutes, including the Alan Turing Institute, the Leverhulme Centre and the Ada Lovelace Institute, all focusing on AI R&D.
Research
Despite strong academic research leading to a large potential workforce and significant investment in the area, the UK generates a low number of successful AI related businesses compared to the USA (Bughin, et al., 2019). The Select committee report on Artificial Intelligence provides recommendations to tackle this shortfall (House of Lords, 2018). It notes that universities and research institutes need to introduce clear and commonplace practices for licensing IP and forming spin-out companies. There also appears to be an associated lag in adoption of AI at scale in the UK compared to Europe and the USA, as indicated in Figure 7.1 (Bughin, et al., 2019).
Figure 7.1 AI technology diffusion in the UK versus Europe and the USA based on % share of companies adopting AI at scale. Based on source (Bughin, et al., 2019)
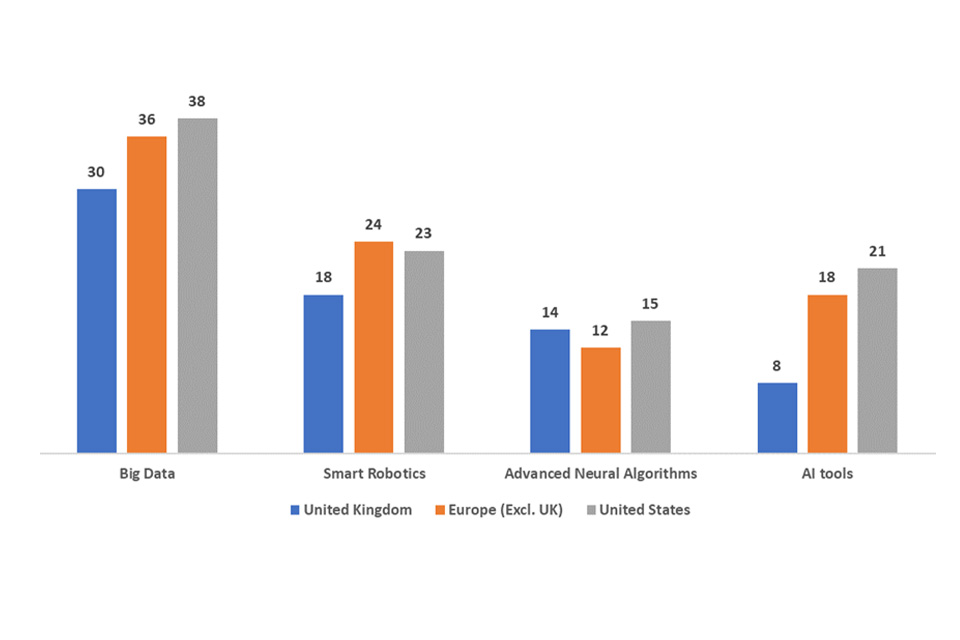
Scale-up and funding culture
There appears to be a trend amongst early-stage UK SMEs to plan an exit through large multinational acquisition rather than seek to grow organically. Early-stage UK deep tech businesses tend to exit before a second or third round of funding, compared to their US counterparts. For example, in the Tech Nation Future Fifty Programme for digital businesses (Tech Nation, 2001), the number of exits completed by startups accelerated by Future Fifty reached 55 as of mid-2021 (Statista, 2022). However, this is also partially due to the difficulties experienced by UK-based deep tech growth companies in trying to gain series-B funding. Many move their base to the USA, specifically due to the ease of access to a well-established funding ecosystem (British Business Bank, 2021).
The UK market may be viewed as too small with limited access to funding sources to scale up. The European market has varying regulations and language requirements. In contrast, the US market represents a large homogenous and English-speaking market with significant capital available and large companies looking to acquire start-ups.
This may also be part of a global trend of rapid exit for AI companies through M&A, as indicated in Figure 7.2 (CB Insights, 2021). Furthermore, it has been estimated that in 2021 the VC value from IPOs of AI and ML semiconductor companies reached over US$10 billion (TechMonitor, 2021).
Figure 7.2 Rate of company exits for AI start-ups worldwide from 2019-2021 and type of exit. Based on source - CB Insights Artificial Intelligence in Numbers Report (CB Insights, 2021)
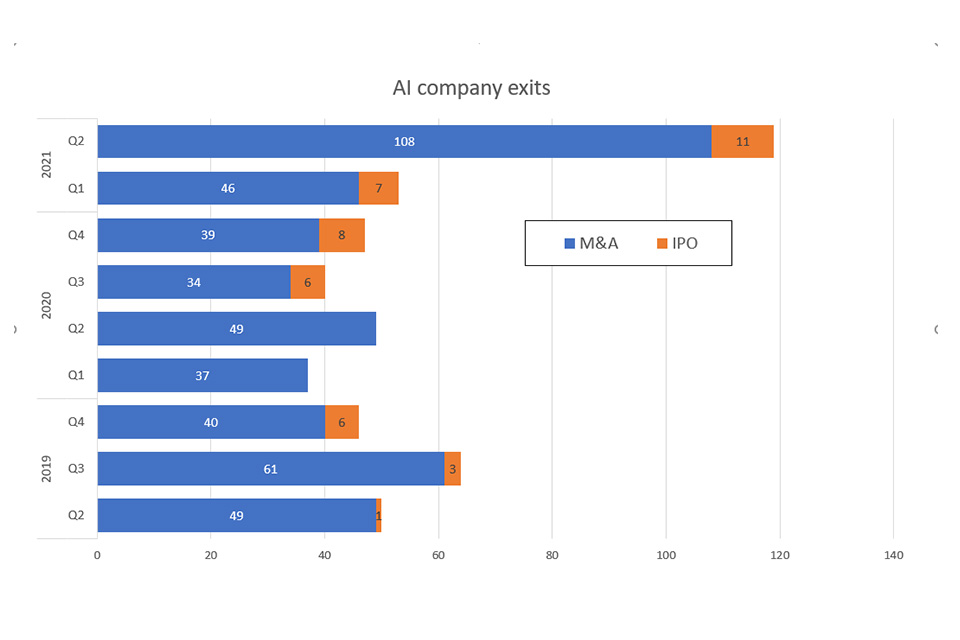
Regulatory framework
In a written evidence report to the House of Lords, Balderton Capital indicated that the main risks to AI development and use (Wise, 2017). Balderton Capital considers that failure to introduce a proper regulatory framework may see international operators take advantage of gaps in the UK market. That would undermine the UK human capital base and potentially increase national security risks. This may already be occurring as much of the patented UK AI-related R&D activities are outsourced or owned by large multinationals (Cowan & Hinton, 2018).
At a national level, several actions can be taken to strengthen and regulate the AI development and use (World Bank, 2021). This can be done through the establishment of a clear regulatory framework and strategy. This would include strengthening the national or regional IP regime where required, modifying competition rules/policy as well as regulatory sandboxing. The state may also provide policy clarity to businesses, as China has done (European Commission, 2021; Chinese State Council , 2017; House of Lords, 2018). By providing regulatory certainty, it is possible to enable businesses to proceed with confidence.
Availability of training data
A further limiting factor, for countries such as the UK, is the insufficient availability of unique training data. This is due to numerous reasons including failures to recognise the value of such data, as well as data protection considerations. Even if a unique AI technology or solution is developed, there may be a lack of suitable training data available in the UK. This contrasts with more open markets such as the US, or state-controlled markets such as China. Thus, there may be opportunities for large companies to license their data for use by third parties. Government departments and organisations may also be able to offer the use of large amounts of (anonymised) data to UK businesses. This approach may help avoid the situation observed in Canada (Cowan & Hinton, 2018). Although significant government investment was made in AI research, most of the final patented inventions are owned by large multinationals not headquartered in Canada.
IP protection
A recent study shows that the widespread adoption of AI across multiple industries and sectors may have an effect on the requirement for IP protection of AI technology (Hilty, et al., 2020). This is because of the very rapid innovation cycles and rapid product or system replacement. It may not make sense to invest in formally protecting a software system by patent if it becomes obsolete before the patent is even granted. As a result, the standard assumption that IP protection is itself an incentive for further innovation may no longer be valid.
Furthermore, the difficulty in protecting software inventions in a timely manner, especially with a patent, may limit software start-ups’ ability to attract investment. It may also hinder their ability to generate revenues through commercial use of the IP rights, for example, licensing deals. As a result, IP protection in the AI space may be justified to protect the rewards from investment in AI to create and invent.
This justification resonates strongly with many in the investment community. A strong IP portfolio, comprising a mixture of varied IP rights, demonstrates business maturity of the borrower. Importantly, it also provides recourse for the investor to recover some of its investment if the business is unsuccessful.
However, major technological changes are minimal due to very rapid development times and continued incremental improvements. Most changes lead the increased calculation speed and improved training data sets. Despite this, original and novel AI software and algorithms could be protected by copyright or trade secrets and related technical contributions by patents.
Countries developing AI, including the UK, will need to develop coherent national strategies and frameworks to avoid IP leakage (Cowan & Hinton, 2018). This includes providing mechanisms such as patent pools or specialist investment funds to ensure they can remain competitive against the US and China. In this context, there remains the question of whether the UK IP framework in a wider definition could help mitigate some of these perceived risks.
Literature survey-based conclusions
Based on our survey of the literature to date, as well as an increasing understanding of economic activities in AI, it is possible to draw a number of conclusions:
-
machine learning is by far the leading area in the AI space and is the type of AI most widely adopted across industry. This is largely because in each case it is solving an industry or business specific need, thereby increasing productivity, and reducing costs
-
in the UK, most investment in start-ups and SMEs comes from the founders, family and friends, angel investors and, in some instances, VC firms. However, at an early stage, VCs are likely to be more limited in the amount of funding they provide and the type of companies they are willing to invest in (GrantTree, 2021)
-
development of, and consequently investment in, AI is predominantly focused on the USA and China. However, within the European context, the UK is leading development activity and investment in AI
-
partnership and cooperation across AI research are limited, while conflict and litigation also appear to be quite low level (WIPO, 2019)
-
additionally, in comparison to several countries including Germany, France, Japan and South Korea, the patent output of the UK is lower (Bughin, et al., 2019). This may be because the UK appears to struggle to turn AI research into successful business (House of Lords, 2018). Several factors may explain this. Some UK activities are early-stage and not sufficiently developed for patenting. It may also be due to UK companies choosing to file in the USA or Europe instead of the UK. However, the lifecycle of software is generally short (~5 years) and early-stage developments can be superseded by new releases and upgrades
-
in the broader context of AI investment in the UK and also further afield, it is clear that, while IP may be part of the equation, it is by far not the most important factor (Bughin, et al., 2019). Access to human skills capital, access to data for training AI, and a reliable legal and regulatory system are also very important prerequisites for investment
-
a company’s decision to invest in the UK seems to be less influenced by the UKIPO/EPO framework than by related professional legal and consulting services. These include UKIPO IP audit and IP access grants enabling commercial advice for early-stage businesses (Mathys & Squire LLP, 2021). Mediation services, IP rights enforcement, trust in the judicial system, and the general regulatory environment also seem to matter more
-
additional economic levers, including tax incentives, can also influence larger companies’ decisions to locate in the UK (TechUK, 2021; BEIS and DCMS, 2019; OECD, 2021). Tax incentives include patent boxes or R&D tax credits
-
an open response to a Government Consultation paper highlighted the important role of IP in cementing the UK position in AI and attracting additional talent and investment (Lord Clement Jones, 2022). They consider several options for amendment of the current IP framework, some of which reflect those of respondents we interviewed. These options included introducing a reduced copyright protection term for AI generated copyright works and leveraging business incentives. They also discuss other options to incentivise data owners to licence training data for use
-
AI is still a heavily white male dominated area. Only 22% of all AI employees in the UK are female. However, 45% of all UK AI start-ups have a female co-founder (Aashind, 2021). Furthermore, according to the 2021 AI Index Report, 45% of North American AI graduates interviewed were white (Zhang, et al., 2021). This demonstrates a lack of diversity in the AI space that may influence on opportunities for the widest possible development and implementation of AI
In-person interviews and questions
In order to gain the most from the industry interaction, face to face interviews were conducted for all the discussions. Typically, these meeting lasted 60 minutes or over, using MS Teams or Zoom calls. It should be noted that during the study period, various Covid-19 restrictions were in place. However, the face-to-face approach was found to enable very open discussions. It also allowed interviewees to express opinions that would have been constrained by a written format.
Two questionnaires were designed to facilitate the interviews with these specific stakeholder groups in the AI value chain. One questionnaire was directed to company actors (both SMEs and Corporate) and another to investors. The questionnaires are provided in Appendix 2 of this report. The aim was to understand their respective views on activities and investment in AI. It was also important to ensure that the questions and format of the interviews were sufficiently similar to avoid creating any bias.
In the first instance a series of stakeholders across the following groups were selected:
-
SMEs
-
large corporates
-
academics
-
accelerators/ Innovation organisations
-
investors
In each target organisation, we typically sought to interview the CEO, CTO, Chief IP officer or similar senior person. This is because they are most likely to have an awareness of the development, use and protection of AI in the business. They are also most likely to have an opinion on the main determinants in the growth of AI based activities and investment. A list of the stakeholder organisations, businesses and investors interviewed is provided in Appendix 1.
These stakeholders were approached directly, where direct email addresses or phone numbers were available. Where no direct contact details were available, we sought to connect to relevant groups via LinkedIn and other platforms. Where the potential interviewee expressed an interest in participating, we then followed up via email and/or phone calls. Interview invitations were sent out in batches, with a number of invitations sent per week and interviews over the subsequent two weeks.
A total of 96 individuals from different businesses and organisations were approached, with 34 responding and 21 interviewed. This equates to an overall response rate of close to 22%. Thirteen respondents indicated that they did not wish to participate and were not interviewed. An overview of the breakdown of those interviewed is shown in Table 9.1 below.
Table 9.1 Overview of number of respondents, interviewees, and responses rates in this study
Numbers | % | |
---|---|---|
Total contacted | 96 | |
Overall response rate | 34 | 35% |
% Interviewed (from contacted) | 21 | 22% |
% Interviewed (from responded | 21 | 62% |
% Declined | 6 | 6% |
The response rate amongst companies and investors was similar at 33% and 31%, respectively. Over half (61%) of respondents were companies and 13% were investors (Figure 9.1). Investors included a mix of angel investors, venture capital and corporate venture. A smaller proportion of interviewees also belonged to accelerators (9%), universities (9%) and other AI related organisations (9%). Among companies, 42% were large, 36% SME and 21% micro. A significant majority of respondents interviewed were male (86%), as indicated in Figure 9.2.
Interview participants
Figure 9.1 Split of respondents interviewed in this study according to entity type
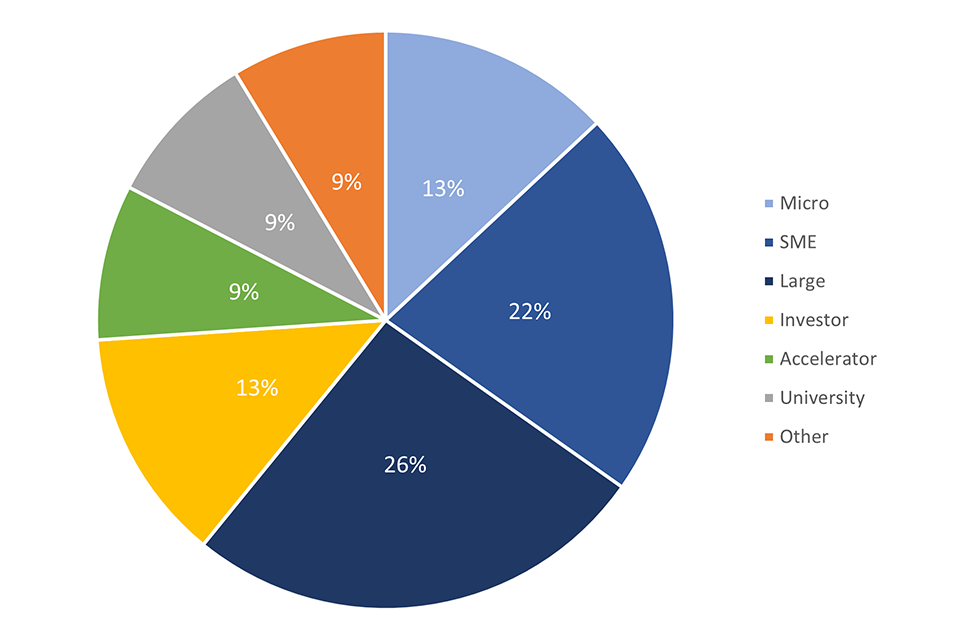
Figure 9.2 Gender split of those interviewed
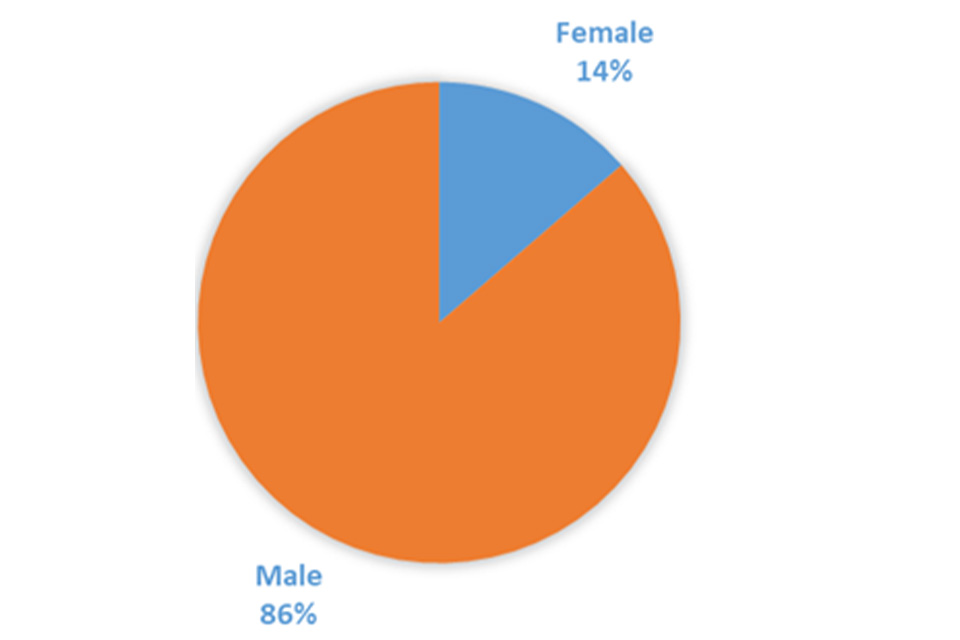
Despite the wide range of different industry interests amongst stakeholders interviewed, responses tended to converge.
It has been highlighted in several interviews undertaken as part of this study that it is not always possible to protect AI inventions by patent. Many software and AI companies, especially start-ups and SMEs, often rely on a combination of copyright and trade secret used in tandem with confidentiality agreements.
However, this has also raised the following questions:
1. Is the current set of IP rights sufficient to protect the innovations arising from this rapidly evolving AI field and software more broadly?
2. Is it time to consider a new type of IP right focused on the protection of AI inventions (Pinsent Masons , 2021; Deamer, 2022)?
3. Could amendments be made to current copyright legislation to address obstacles specific to current software and AI developments?
The UKIPO launched a consultation on the topic of copyright and patents in AI in October 2021 (UKIPO, 2021).
Such questions have been raised in several reports and studies. A recent 2020 study by the USPTO sought to answer some of these questions on patenting AI inventions (USPTO, 2020). In this study, several respondents indicated that current IP rights were sufficient, with any gaps being filled with suitable confidentiality agreements. Others indicated that a new form of IP right might be needed to protect data associated with ML in larger companies. They also recommended to make this data available to others to allow other market entrants to train their own AI.
Access to external data has been raised by a large corporate interviewee. This company uses commercially provided customer data from other parties and labels for training purposes. As this data is gradually passed through a chain, labelled and therefore gradually modified (in some cases by numerous parties), an important question was raised about: “the point at which it no longer resembles the original data provided and as such, where numerous parties will have modified that data through the chain, who actually owns the rights to that data?”
Conclusions from in-person interviews
This section highlights the main conclusions derived from the interviews with stakeholders undertaken as detailed above in section 9. The main insights from the interviews are summarised below and broken down into specific topics: IP, human skills capital and staffing, investment, and AI application. Capturing views from targeted and stratified face-to-face interviews has provided many insights that would not have been obtained through responses to written questionnaires.
We note that having undertaken more than 20 interviews, the same issues and topics were repeated by different interviewees in different sectors.
Intellectual property
-
the majority of micro and SME companies interviewed perceived that they could not get patents for their software developments. Technology sector participants had a generally perceived misconception that ‘core AI software is not patentable’. A similar observation was made in a recently published DCMS and OAI commissioned report on AI (Westgarth, et al., 2022). This report also notes that the value of AI is derived from its application to solve an existing problem. Yet, patent protection for AI-related inventions is available across all technology areas. Patent inventions embodying algorithms or software are allowable if they make a technical contribution. The UKIPO, like the EPO, grants many thousands of patents for computer and software implemented inventions including in the field of AI (UKIPO, 2019). Thus, they do provide protection for innovators in this field. The UKIPO’s Manual of Patent Practice provides extensive guidance on the patenting of software and computer implemented inventions
-
companies that were purely developers for third parties working at the pre-application stage felt limited in their ability to protect their inventions by patents. Conversely, those developing their own AI system to solve a specific problem felt they demonstrated technical effects, which could be more easily patented
-
amongst micro and SME companies we observed an imbalance in the understanding of the patent process, copyright and trade secrets (see Figure 10.1). Patents were the most readily understood with copyright and trade secrets being much lower down in both understanding and perceived value. Whilst in early software development stages, companies relied on copyright and trade secrets without a full understanding of the rights or legal protection they provided
Figure 10.1 Perceived imbalance in understanding of relative importance of different IP rights for different actors. Scales image under licence from Canva.com
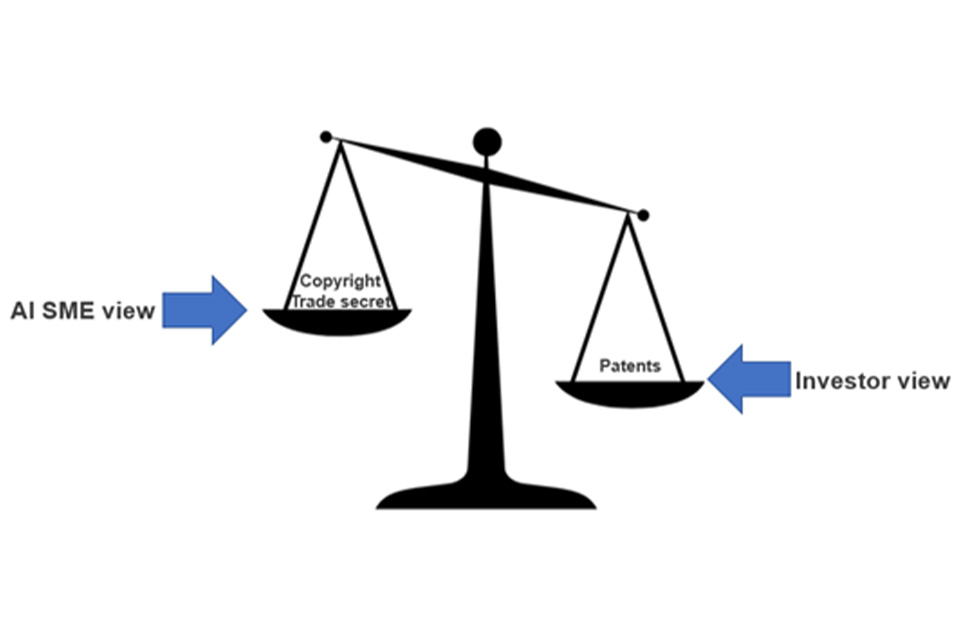
-
start-ups in early (pre-application) AI development stages have a dilemma since their main assets are software which is not patentable as such (in the UK). Without patents, it is more difficult for investors to obtain an objective measure of the inventiveness of software code leading to a patent bias for investors. It is then harder for some early-stage software and AI companies to raise money and leads to ‘patent anything or make filings’ to satisfy investors. Yet, in an early-stage company the budget required for patent filing is difficult to justify
-
some companies feel that their US counterparts have an advantage in ability to patent software, including AI
-
the view from some SME’s, especially early-stage companies, is that they have to rely on copyright and trade secrets. However, their understanding of those legal rights is low and the technology investor community does not view these rights as very secure. This contrasts with companies in the media sector that view copyright as their main asset, which is also well understood by media investors
-
it appears that the technology /science sector (patent-centric) and media sector (copyright-centric) are now merging with the advent of AI. The support infrastructure in terms of service providers and expertise has developed separately over time to support each of the sectors with little overlap. Thus, the technology/science sector needs to access service and expertise traditionally focused on the media sector
-
one respondent indicated that rather than developing a new right for AI, it might be appropriate to simply create a parallel version of copyright law applying to AI. The current copyright law would protect the written word, imagery etc while a new alternative copyright would protect software, AI and similar technology assets
-
companies’ reliance on different elements of the UK IP framework changes as they change in size, as illustrated in Figure 10.2
-
companies want consistency in global IP frameworks.
-
AI companies were often founded through university spinouts or by PhD students. One respondent mentioned that the UK is favoured due to the lower costs of patent filings and high level of expertise amongst UK patent attorneys. However, the general view amongst UK SMEs interviewed was that the route of filing for patent protection is often prohibitively expensive and slow. Consequently, most SMEs have chosen to keep filings to a minimum to reduce costs
-
we note, from speaking to a number of interviewees, that it is not clear where the UK sits on trade secret protection. There were questions about whether the current Trade Secrets (Enforcement) Regulations 2018 (Crown Copyright, 2018) will remain as such following departure of the UK from the EU. Other questions related to trade secret protection in the UK compares to the USA (US Government Publishing Office, 2016) or to the EU (European Parliament, 2016). One interviewee indicated that the existence of this protection should be better highlighted
Figure 10.2 Perception of value and benefit of IP changing depending on the actor and stage of business
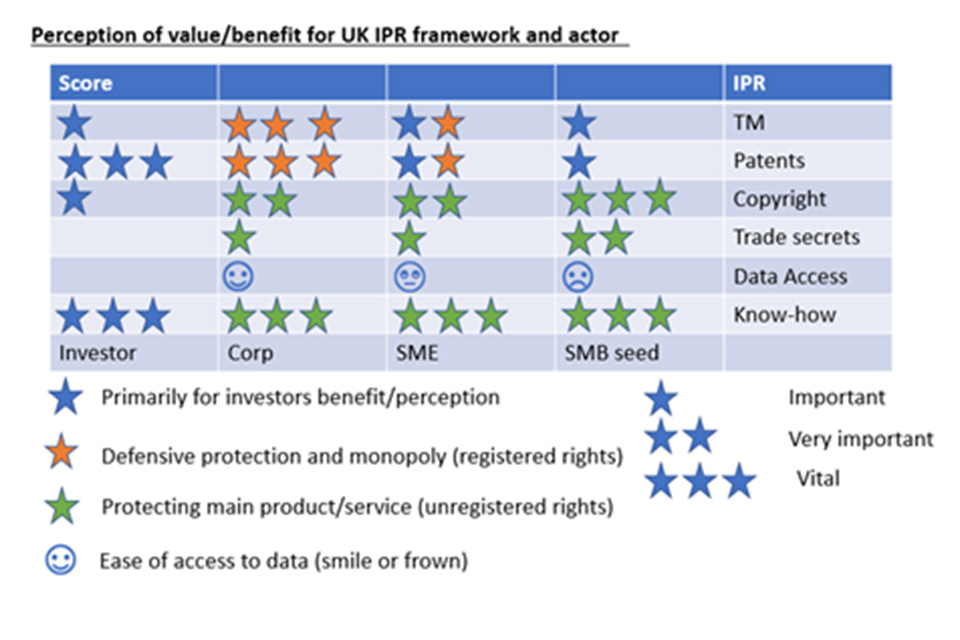
Human capital and staffing
-
the UK ecosystem is generally viewed positively with good source of grants, investment, and skills
-
human resources and skills are fundamental to all sectors, company sizes and investors. The interviewees were unanimous that resourcing was the most important aspect for AI
-
London and Cambridge were cited by international companies as AI hubs. Ecosystems were cited as being important in attracting further talent, by interviewees both inside and outside the UK. Ecosystem are defined as co-location of companies, universities and research
-
in many cases, qualified AI staff are difficult to find and, in most businesses, it is not sufficient to only have AI experience. Most businesses also need someone with experience of specialist fields in which AI may be applied, such as medical imaging or drug discovery. This multi-disciplinary approach allows for the most novel uses of AI
-
many larger SMEs regularly present at conferences, publish articles and participate to hackathons to showcase their activities and abilities alongside large multinationals such as Google. This is a requirement for many AI researchers seeking to establish their names in the field and wanting to share their work outside the company. This may not always sit easily alongside company IP policy requiring a high degree of secrecy protection around trade secrets
-
many businesses interviewed had AI teams comprising people in the UK and in other countries. Visa issues after the UK left the EU may have made travel more difficult to and from Europe. However, the ease at which people have adapted to working remotely during the COVID-19 pandemic has enabled development teams to be located around the world.
Investment and funding
-
most VC investors want to see companies with patents, whilst corporate investors are more interested in technology and the team
-
many SMEs view patents as important in their ability to attract and satisfy investors. However, other rights such as copyrights or trade secrets are less recognised by investors, especially VCs
-
the UK grant funding system, including Innovate UK, Innovate UK Edge grants and UKIPO grants, was viewed as very helpful at early development stage
-
the great majority of start-ups plan to exit as soon as possible. The UK is not viewed as having the market size or capital availability to scale up a business. The USA is viewed as having both market size, capital and large companies looking to acquire start-ups. Challenges in scaling up for UK innovative businesses is also highlighted in the Evidence paper of the UK Innovation Strategy (BEIS, 2021)
-
a number of companies using AI for drug design and binding site identification were interviewed and this appears to be a growing area. This is reflected in an AI Index Report which states that the Drug Discovery field was the area receiving most private AI investment in 2020 (Zhang, et al., 2021).
-
AI is being widely applied in the MedTech sector, especially in drug-discovery, potentially spurred by the COVID-19 pandemic
Data
-
access to data is essential for all sectors. Yet, larger established companies have access to more of their own data whereas start-ups often have limited access to data, which can slow development. Improving common access to data or data sharing would help start-ups accelerate developments and create more of a level playing field. Large companies have a potential monopoly on main data resources.
-
most SMEs interviewed noted that they had sufficient access to AI training data through commercially available datasets as well as in-house synthesised datasets. They also noted that a government supported, or industry supported, pooling of anonymised data for training would be strongly welcomed. Nevertheless, they recognise that it may be difficult to persuade industry to participate. In that respect, China has a clear advantage in its ability to gather and use large amounts of training data relative to western nations (WIPO, 2019). This suggestion is in line with recommendations made in an independent report Commissioned by DCMS and BEIS (Hall & Pesenti, 2017). This report recommends that training data should be more readily available and government and industry should aim to develop “data trusts” to facilitate data sharing
-
many companies use a mixture of commercially available (purchased) data sets and synthetically created data sets for training their AI system. Larger corporate businesses may have access to large amounts of data in-house which they own, thereby giving them an advantage. This is particularly the case in the pharma and biotech industries
-
in sectors where personal information is widely used, as in the medical sector, a particular data ownership structure is preferred. In this case, individuals would retain ownership of their personal data but allow for use in certain permitted circumstances
-
an interviewee in the consumer product and medical application field indicated they had access to large amounts of data through the devices their clients use. However, large amounts of data require cleaning and labelling to be exploited in useful ways. In some cases, regulatory controls also limit access to potentially available data
Relevance of being in the UK
-
the UK ecosystem and availability of funding is generally looked upon favourably compared to elsewhere in Europe. However, companies would typically seek funding outside the UK for scaling a business
-
remote working means that many team members do not need to be in the UK with the majority of respondents having international teams (Figure 10.3)
-
a large corporate investor for an Asian multinational noted that they perceive little differences between the UK and Europe (the UK is viewed as part of Europe) in terms of investment. The determinant is, in most cases, a good and well-developed technology with a commercial application
-
another large corporate indicated that any IP protection change for AI-related inventions and creations would need to occur at a larger international or European level. A singular “special” AI right provided in the UK alone would be of little interest or use to many internationally operating businesses
Figure 10.3 Typical UK start-up AI resources across Europe with multiple team members and teams scattered around Europe. European map image supplied under licence from Canva.com
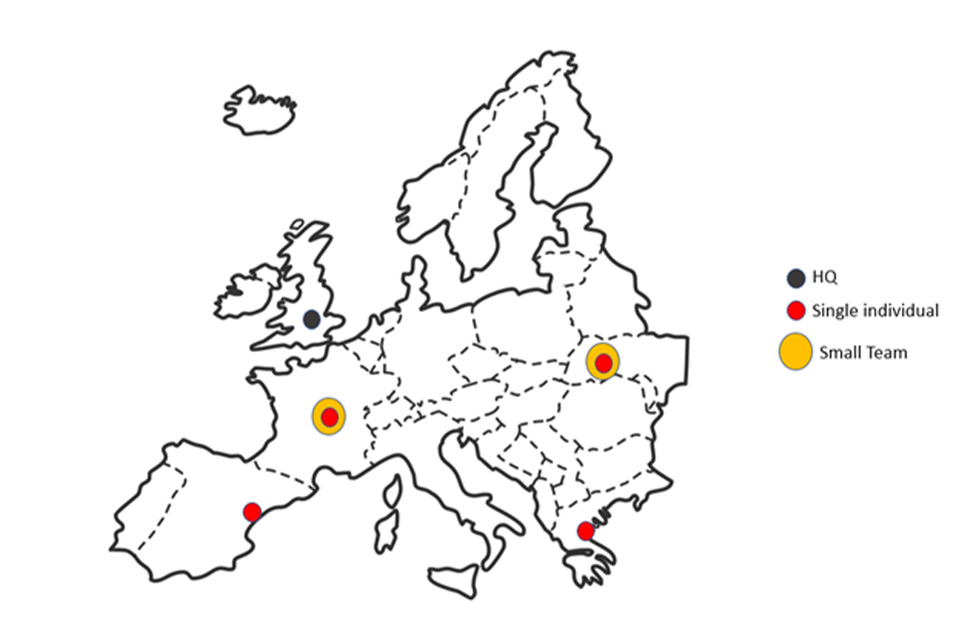
Overall headline conclusions
-
the UK IP Framework is not a fundamental consideration for investment by the participants in this study
-
other factors currently dominate investment decisions. The availability of human capital particularly PhD graduates, AI know-how within the UK workforce and the reputation of technology hubs and universities are particularly important. Government grants and access to early-stage funding are also essential
-
the participants regarded the UK as a good location to invest in a start-up AI business for several reasons. The UK has a relative strength of the above factors in relation to other locations. In particular, the majority of UK participants had graduated from UK universities with AI qualifications
-
the UK IP framework was broadly regarded as trusted and reliable and therefore not of concern regarding investment decisions. However, it should be noted that early stage start-ups did not have much ‘in-house’ experience of using the IP framework
-
participants from the technology sector noted a propensity for investors to require patents to support an investment round. Yet, their main asset, AI-related software, is viewed as not patentable. This latter point is a common misconception, as the presence of a technical effect does provide potential patentability
-
investors in early-stage AI companies viewed patents as offering a third-party opinion on the uniqueness of the company offering. In some cases, it was considered an ‘asset insurance’ which could be sold in the event the company ceased trading
-
within the early-stage technology sector companies, there was a good understanding of the patent system, but a much weaker understanding of copyright
-
all the participants had a relatively weak understanding of the IP framework related to trade secrets
-
technology sector companies were typically served by patent attorney firms, with a focus on obtaining patents
-
participants from the media sector were very familiar with the copyright framework and would typically use the services of a copyright solicitor
-
there appear to be two communities of interest using and/or developing AI technology and the UK IP framework. The technology sector community tends to focus on patents and relies on patent attorney firms while the media sector is served by copyright solicitors
-
currently there is a historical gap (See Figure 10.4) between these communities with little mixing of expertise. Bringing together combined patent and copyright expertise to focus on the AI sector would be particularly helpful for early-stage companies. This way, they could develop commercial strategies to realise the full potential of their assets both in terms of protection, fundraising and subsequent exploitation
Figure 10.4 Under the current IP Framework, software sits uncomfortably between the patent world and the copyright world, which have little real overlap
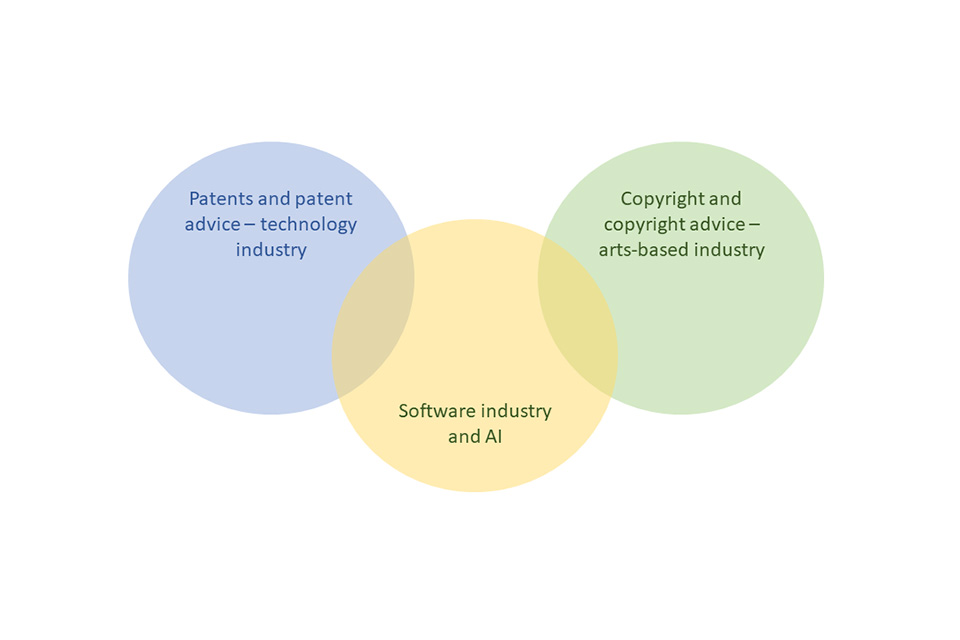
- the UK has established a strong reputation as a place to incubate new technologies, including AI. The UK is seen as too small a market to scale-up and too hard to get the larger rounds of investment needed for major long-term growth. Many young companies choose early exit or moving to the USA. It is a far larger market and has a reputation for risk and as the place to get large investment. This poses the risk of the UK losing out in the larger-scale economic benefits of AI business growth to other much larger economies.
References
Aashind, A., 2021. Curious Artificial Intelligence Statistics UK [2021]. [Online]
[Accessed 26 January 2022].
ABI Research, 2017. Artificial Intelligence Investment Monitor 2017. [Online]
Accenture, 2021. Rewire for Sucess - Boosting Indias AIQ, Bangalore: Accenture.
Avin, S., 2019. Exploring artificial intelligence futures.. Journal of AI Humanities.
BEIS and DCMS, 2019. Policy Paper - AI sector Deal. [Online]
[Accessed 06 December 2021].
British Business Bank, 2021. Small Business Equity Tracker 2021, Sheffield: British Business Bank.
Bughin, J. et al., 2019. Artificial intelligence in the United Kingdom: Prospects and challenges, London: Mckinsey & Company.
Business Insider, 2017. How 9 industries are being transformed by UK innovation around the world. [Online]
[Accessed 29 November 2021].
CB Insights, 2021, PDF 4.3MB. Artificial Intelligence In Numbers Q2 2021. [Online]
[Accessed 26 January 2022].
Chinese State Council , 2017. A Next Generation Artificial Intelligence Development Plan. [Online]
[Accessed 30 November 2021].
Cornell University, INSEAD & WIPO, 2020. The Global Innovation Index 2020: Who will finance innovation?, Ithaca, Fontainebleu & Geneva: Cornell University, INSEAD & WIPO.
Council of Europe, n.d. What is AI? [Online]
[Accessed 13 10 2021].
Cowan, P. & Hinton, J., 2018. Intellectual Property and artificial intelligence: What does the future hold? , London: Law Business Research .
Crown Copyright, 1997. The Copyright and Rights in Databases Regulations 1997. [Online]
[Accessed 20 January 2022].
Crown Copyright, 2018. The Trade Secrets (Enforcement, etc.) Regulations 2018. [Online]
[Accessed 06 December 2021].
DCMS & OAI, 2022. New UK initiative to shape global standards for Artificial Intelligence. [Online]
[Accessed 20 January 2022].
Deamer, L., 2022. New report claims fixing copyright would boost AI startup scene. [Online]
[Accessed 27 January 2022].
Deloitte , 2021. State of AI in India, Mumbai: Deloitte Touche Tohmatsu India LLP.
Deloitte Development LLC, 2021, PDF 1,548KB. Can medtech start-ups show us where the industry is headed? Insights from Medtech Innovator and industry leaders. [Online]
[Accessed 11 10 2021].
Die Bundesregierung, 2020. Artificial Intelligence Strategy of the German Federal Government, Berlin: Die Bundesregierung.
Digis, 2021. State of Artificial Intelligence in Europe: Statistics 2021. [Online]
[Accessed 10 February 2022].
DMCS and OAI, 2022. New UK initiative to shape global standards for Artificial Intelligence. [Online]
[Accessed 20 January 2022].
European Commission, 2020. White Paper On Artificial Intelligence - A European approach to excellence and trust, Brussels: European Commission.
European Commission, 2021. Europe fit for the Digital Age: Commission proposes new rules and actions for excellence and trust in Artificial Intelligence. [Online]
[Accessed 09 February 2022].
European Parliament, 2016, PDF 505KB. DIRECTIVE (EU) 2016/943 OF THE EUROPEAN PARLIAMENT AND OF THE COUNCIL. [Online]
[Accessed 02 12 2021].
Exscientia, 2021. Bristol Myers Squibb exercises option to develop Exscientia’s AI-designed, immune-modulating drug candidate. [Online]
[Accessed 13 January 2022].
Exscientia, 2022 . Exscientia and Sanofi Establish Strategic Research Collaboration to Develop AI-driven Pipeline of Precision-Engineered Medicines. [Online]
[Accessed 13 January 2022].
Gartner, 2019. Top trends on the Gartner Hype cycle for Artificial Intelligence, 2019. [Online]
[Accessed 15 10 2021].
Government of Ireland, 2021. AI - Here for Good: National Artificial Intelligence Strategy for Ireland. [Online]
[Accessed 05 December 2021].
Grace, K. et al., 2018. When will AI exceed human performance? Evidence from AI experts. Journal of Artificial Intelligence Research, Volume 62, pp. 729-754.
GrantTree, 2021. UK Start-up funding guide. [Online]
[Accessed 27 January 2022].
Gruetzemacher, R., Paradice, D. & Lee, K. B., 2019. Forecasting Transformative AI: An Expert Survey. arXiv preprint, p. arXiv:1901.08579..
Hall, W. & Pesenti, J., 2017. Growing the artificial intelligence industry in the UK , London: Crown Copyright.
Haskel, J. & Westlake, S., 2018. Capitalism without Capital - The rise of the intangible economy. 1 ed. New Jersey: Princeton University Press.
Hayes, J., 2021. UK’s AI masterplan vs reality. [Online]
[Accessed 5 December 2021].
Hilty, R., Hoffmann, J. & Scheuerer, S., 2020. Intellectual Property Justification for Artificial Intelligence. Intellectual Property Justification for Artificial Intelligence. Draft chapter. Forthcoming in: J.-A. Lee, K.-C. Liu Artificial Intelligence & Intellectual Property, Oxford, Oxford University Press, 2020, p. 20.
HM Government, 2017. Industrial Strategy - Building a Britian fit for the future , London: Crown Copyright.
HM Government, 2021. National AI Strategy , London: Crown Copyright.
House of Lords, 2018. Select Committee on Artificial Intelligence - AI in the UK: ready, willing and able?, London: House of Lords.
IFC, 2019. Artificial Intelligence: Investment Trends and Selected Industry uses, Washington DC: International Finance Corporation.
IFC, 2020. Artificial Intelligence in Emerging Markets, Washington DC: IFC.
International Finance Corporation, 2019. Artificial Intelligence and 5G Mobile Technology can drive investment opportunities in emerging markets , Washington DC: IFC .
Judah, N., 2018. The global impact of AI across industries. [Online]
[Accessed 29 November 2021].
Kearney, n.d. Investing in AI-driven industrial automation. [Online]
[Accessed 29 November 2021].
Lexology, 2021. What are digital twins and what are the legal issues with them?. [Online] [Accessed 15 February 2022].
Lord Clement Jones and Christian Gordon-Pullar, 2022. Artificial Intelligence and Intellectual Property: incentivize human innovation and creation. [Online] [Accessed 20 January 2022].
Lord Clement Jones, 2022. Artificial Intelligence and Intellectual Property: incentivize human innovation and creation. [Online] [Accessed 20 January 2022].
Mathys & Squire LLP, 2021. IPO launches IP Access grant funding scheme to help innovative UK-based SMEs. [Online] [Accessed 27 January 2022].
McCarthy, J., 2011. What is AI? / Basic Questions. [Online] [Accessed 14 February 2022].
McKinsey & Company, 2019. Notes from the AI Frontier - Tackling Europe’s Gap in Digital and AI, Brussels: McKinsey & Company.
Mckinsey Digital, 2020. Global Survey: The state of AI in 2020 , s.l.: McKinsey & Company .
MMC Ventures, 2019. The State of AI 2019: Divergence.” MMC Ventures Report in Partnership with Barclays. [Online] [Accessed 30 09 2021].
Mobihealthnews, 2021. UK men’s health start-up Numan acquires Swedish health tech company Vi-Health. [Online] [Accessed 13 January 2022].
Müller , V. C. & Bostrom, N., 2016. Future progress in artificial intelligence: A survey of expert opinion. Fundamental issues of artificial intelligenc, pp. 555-572.
Nesta, 2017. New Generation of Artificial Intelligence Development Plan. [Online] [Accessed 30 November 2021].
New America, 2017. China’s Plan to ‘Lead’ in AI: Purpose, Prospects, and Problems. [Online] [Accessed 30 November 2021].
OECD, 2019. Artifical Intelligence in society, Paris: OECD Publishing.
OECD, 2021. Government support for business research and innovation in a world in crisis. In: OECD Science, Technology and Innovation Outlook 2021: Times of Crisis and Opportunity. Paris: OECD.
OECD, 2021. OECD Business and Finance Outlook 2021 : AI in Business and Finance, Paris: OECD.
ONS, 2019. Investment in intangible assets in the UK by industry: 2019, London: Open Government Licence v3.0.
ONS, 2019. Investment in intangible assets in the UK by industry: 2019, London: Open Government Licence v3.0.
ONS, 2019. UK innovation survey 2019: main report. [Online] [Accessed 26 January 2022].
Oxford Insights, 2019. Government Artificial Intelligence Readiness. [Online] [Accessed 26 January 2022].
Pierides, M. & Roxon, C., 2021. Legislative Approaches to AI: European Union v. United Kingdom. [Online] [Accessed 05 December 2021].
Pinsent Masons, 2021. UK considers new IP right for AI. [Online] [Accessed 27 January 2022].
Rijksoverheid, 2019. Strategic Action plan for Artificial Intelligence , Den Haag: Ministerie van Economische Zaken en Klimaat.
Searle, J. R., 1980. Minds, brains, and programs. Behavioral and Brain Sciences 3, Volume 3, pp. 417-457.
South China Morning Post, 2018. China plans to be a world leader in Artificial Intelligence by 2030. [Online] [Accessed 15 October 2021].
Statista, 2021. Value of investments into AI in China 2016-2020. Sourced from: Deloitte, Intel; various sources (Shenzhen AI Industry Association); ITJuZi. [Online][Accessed 25 January 2022].
Statista, 2022. Number of exits made by start-ups after participating in selected accelerators in the United Kingdom as of July 2021. [Online] [Accessed 21 January 2022].
TechMonitor, 2021. AI semiconductor funding rockets as demand for advanced chips grows. [Online]
[Accessed 26 January 2022].
TechUK, 2021. Cloud Computing and Data costs to be covered under expanded R&D tax credit. [Online] [Accessed 06 December 2021].
Tejaswi, M., 2021. India expected to invest $1 billion in AI by 2023. The Hindu, 26 April, pp.
The Economic Times, 2021. India Inc. intends to increase spending on AI: Deloitte report. The Economic Times, 16 December.
The Manufacturer, 2022. Monolith AI and Imperial College London to use £500k Innovate UK grant to create AI that can assess metal part manufacturability. [Online] [Accessed 21 Jan 2022].
Turing, A. M., 1950. I. - Computing Machinery and Intelligence,. Mind, LIX(236), pp. 433-460.
UK AI Council, 2021, PDF 3.3MB. AI Roadmap. [Online] [Accessed 14 10 2021].
UKIPO, 2019. Artificial Intelligence A worldwide overview of AI patents and patenting by the UK AI sector, Newport: UKIPO.
UKIPO, 2021. Artificial Intelligence and IP: copyright and patents. [Online] [Accessed 27 January 2022].
UKIPO, 2021. Economic Analysis of the role of Intellectual Property (IP) on investments in Artificial Intelligence (AI), Newport: UKIPO.
US Government Publishing Office, 2016. S.1890 - Defend Trade Secrets Act of 2016. [Online] [Accessed 02 12 2021].
USPTO, 2020. Public Views on Artificial Intelligence and intellectual property policy , Alexandria : USPTO .
van der Merwe, B., 2021. Weekly data: How China became an AI powerhouse. InvestmentMonitor, 9 November, pp.
Verdict, 2021. UK attempts to join the global AI arms race – but at a cost to regulation. [Online] [Accessed 05 December 2021].
Wikipedia, 2021. [Online]
WIPO, 2019. WIPO Technology Trend 2019 - Artificial Intelligence, Geneva: WIPO.
WIPO, 2019. World Intellectual Property Report; Intangible Capital in Global Value Chains - Selected AI Categories and terms. [Online] [Accessed 15th October October 2021].
Wise, J., 2017. Balderton Capital (UK) LLP - Written Evidence (ALC0232). [Online] [Accessed 07 October 2021].
World Bank, 2021. Harnessing artificial intelligence for development in the post Covid-19 era - A review of national AI strategies and policies. [Online] [Accessed 8 October 2021].
Zhang, D. et al., 2021. The AI Index 2021 Annual Report, Stanford: Stanford University.
Appendix
Appendix 1: Actors interviewed
Senior representatives of the following stakeholder organisations, businesses and investors were interviewed in the course of this study:
IP Offices
UKIPO
Companies by size, AI type, field and application
Company | Type | AI Type | Field | Application |
---|---|---|---|---|
Aistetic | Micro | Computer Vision | Medtech | Retail / Digital Health |
Move.ai | Micro | Neural Networks | Entertainment | Movie & gaming industry |
Peptone | Micro | Machine Learning | Medtech | Drug Discovery |
Agile Analog | Small | Machine Learning | Chip production | Circuit design |
Brainomix | Small | Machine Learning | Medtech | Medical Imaging |
Neu.ro | Small | Machine Learning | Medtech | AI based digital therapy |
Arctic Shores | Medium | Machine Learning | Recruitment Tech | Recruitment |
Oxbotica | Medium | Computer Vision | AV | Autonomous Vehicles |
Benevolent AI | Large | Machine Learning | Medtech | Drug Discovery |
Onfido | Large | Machine Learning | Fintech | Security in Financial sector |
Amadeus | Large (multinational) | Machine Learning | IT | Travel industry systems |
GSK | Large (multinational) | Machine Learning | Medtech | Drug discovery |
Nvidia | Large (multinational) | Machine Learning | Chip production | Computer systems |
Philips | Large (multinational) | Machine Learning | Medtech | Consumer products |
Investors
-
Samsung Ventures – Corporate investor
-
Sure Valley Ventures – VC
-
Capital Enterprise – Seed funding
Universities and research organisations
-
Goldsmiths University of London
-
University of Cambridge
Miscellaneous organisations (think-tanks, incubators, accelerators)
-
Alliance for IP (media/copyright)
-
Naomi Korn Associates (copyright consultancy)
-
Scale.ai (start-up/accelerator)
-
Tech Nation (accelerator)
Figure A1.1 below shows a breakdown for the companies’ respondents by type of AI used. The majority uses ML, while others use Computer Vision or Neural Networks
Figure A1.1 Chart showing the type of AI used by interviewed companies
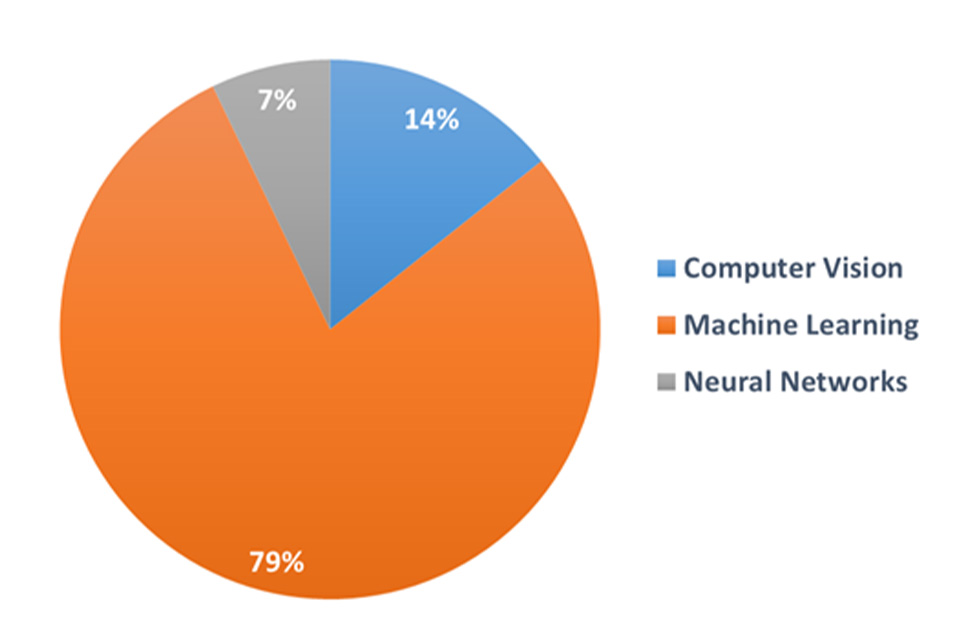
Figure A1.2 indicates the type of AI being used by companies’ respondents and the fields of AI application. One of the main areas is in MedTech. Recent reports indicate the significant growth of this field in recent years, specifically in drug discovery (Zhang, et al., 2021).
Figure A1.2 Overview of companies’ respondents by type of AI used, field of application and company size
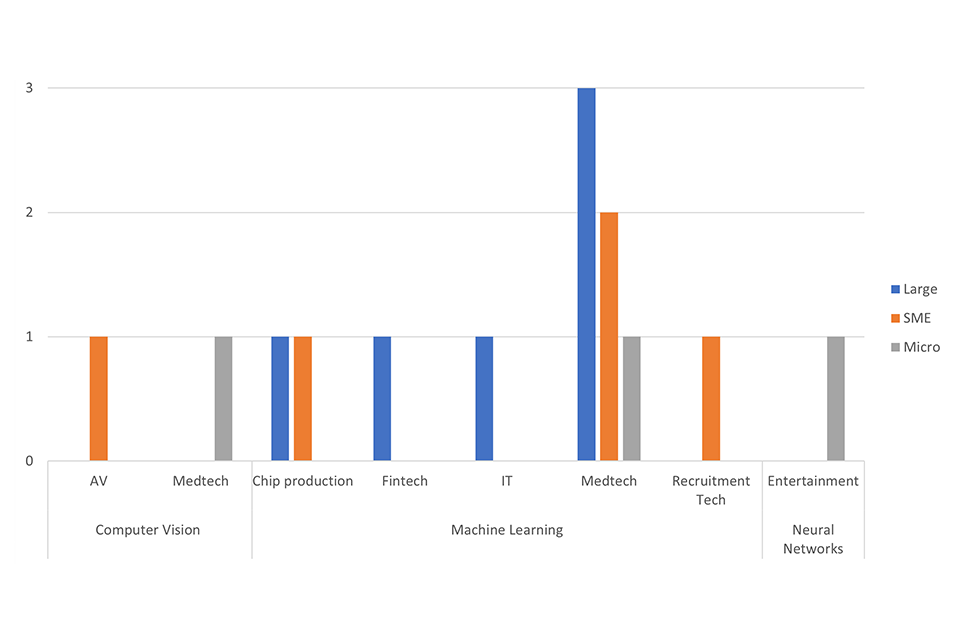
Appendix 2: Questions to support interviews
Questions for SMEs/Corporates
The following questions were used to support the face-to-face interviews with SMEs and Corporates.
A. Personal information
a. Name of Company:
b. Name of person interviewed:
c. Role of person interviewed (needs to be an IP Manager/CTO or similar)
d. Name of interviewer:
e. Date and time:
f. Gender
g. Ethnicity
B. General information
a. In what field is your business active?
b. Is your business involved in innovation?
c. What are the big trends/changes happening in your market at the moment?
d. What do you see happening in the future, say 5 years from now?
e. Do you have staff based in the UK, if so, approximately how many?
f. Why do you have people in the UK?
g. What are the advantages of being in the UK?
1. Are there disadvantages to being in the UK?
C. AI use/investment and determinants
a. How would you define AI in your business context?
b. What benefits does AI provide, now or future?
c. Do you use / invest in AI in your business?
i. If yes,
1. For what purpose (research activities, marketing, …)?
2. What factors have acted as a barrier to adopt AI?
3. Do you have AI expertise within your company?
a. Are they based in the UK?
i. if yes, what are the advantages and disadvantages of having staff in the UK?
4. Do you buy/licence AI applications? And if so, what type of rights?
d. Are there any economic factors which stop you from investing in AI now?
e. Do you work with partners research or developing AI capabilities?
f. Do you fund any external AI developments, if so, in which country is the development being conducted?
D. IP use and role in business decisions including AI investment
a. Do you own any registered IP rights such as patents, trade marks or designs? Specify which rights you own.
i. If yes,
1. How important are patents to your business?
2. Do patents play a role in your business decisions? [All the time, Some of the time, Never]
a. If no, why not?
3. How important are designs to your business?
4. Do designs play a role in your business decisions? [All the time, Some of the time, Never]
a. If no, why not?
5. How important are trade marks to your business?
6. Do trade marks play a role in your business decisions? [All the time, Some of the time, Never]
a. If no, why not?
b. Do you have unregistered rights such as copyright or trade secrets?
i. If yes,
1. What types of rights?
2. How important are copyrights to your business?
3. Do copyrights play a role in your business decisions? [All the time, Some of the time, Never]
a. If no, why not?
4. How important are trade secrets to your business?
5. Do trade secrets play a role in your business decisions? [All the time, Some of the time, Never]
a. If no, why not?
c. Does the UK IP framework incentivise you to invest in AI or AI projects?
1. If yes, how and in what way is the IP framework an incentive for you to invest in IP?
2. If no, why is the IP framework not an incentive to invest?
d. Is there anything about the UK IP framework that you would like to see changed?
Questions for inventors
The following questions were used to support the face-to-face interviews with Investors:
Name of Company
Name of person interviewed
Role of person interviewed
Interviewed by
Date
Time
-
what type of investor are you? - e.g. angel, VC, corporate
-
in what areas to you invest?
-
what are the big trends/changes happening in your market at the moment?
What do you see happening in the future, say 5 years from now? -
do you invest in AI? What type?
-
where are your main investments/ portfolio companies?
-
what proportion of portfolio companies relate to AI or ML based technologies?
-
do you have AI expertise in your investment team? How many are UK based?
-
what are the advantages of being in the UK?
-
are there disadvantages to being in the UK?
-
what are the main factors you look at when considering investing in a business?
-
what sort of due diligence do you conduct before investing? What are the main requirements?
-
do you consider registered IP rights such as patents, trade marks or designs when doing your due diligence of potential investment?
-
how important are registered rights to you in your investment decision?
-
what do you see as the main benefits of registered rights in commercial terms?
-
do you consider unregistered rights such as copyright or trade secrets?
-
what is your view on know-how and skills retention?
-
does the UK IP framework incentivise you to invest in AI or AI projects?
-
if yes, how and in what way is the IP framework an incentive for you to invest in IP?
-
if no, why is the IP framework not an incentive to invest?
-
is there anything about the UK IP framework that you would like to see changed?
-
if this was changed, would it incentivise you to invest more money in AI in your business?
-
are there any economic factors which stop you investing in AI now?