MHRA Data Strategy 2024 -2027
Published 18 September 2024
1. Executive Summary
I am delighted to introduce the MHRA’s first Data Strategy at a moment of profound change and opportunity. We are seeing data and digital tools transform almost every aspect of our work and our lives. Big data, advanced analytics, and emerging digital technologies have been compared to a ‘fourth industrial revolution’, whilst artificial intelligence and machine learning have been likened to ‘the new electricity’.
Never before has science and technology converged with such velocity and potential for tangible impact across a range of clinical conditions and medical products. It is therefore timely that we have reflected upon the opportunities and challenges we face in relation to digital, data, and technology both within our organisation and across the broader health data ecosystem.
Having considered this landscape, we have developed a strategy which defines our vision for data, digital technology, and real-world evidence over the coming years. In doing so, we have sought to differentiate our strategy, recognising the highly specialist and in some cases unique aspects of our organisation, as a sovereign regulator of medical products and a hub for regulatory science and innovation. Our Data Strategy is based on five core themes, each underpinned by specific deliverables.
Running through fiscal years 2024 to 2027, the MHRA’s Data Strategy looks outwards, seeking to optimise our offering for our stakeholders and our customers, and enable innovation throughout the ecosystem. Our approach aspires, wherever it is possible and appropriate, to use data to streamline, deduplicate, and harmonise our regulatory processes and procedures.
By delivering this strategy, working in close collaboration with partners both within the UK and internationally, we will use data to deliver timely, proportionate, and scientifically robust regulatory decisions which facilitate early access to medical products and safeguard public health.
Dr June Raine DBE
Chief Executive Officer
2. Context
Data and digital technologies have transformed our society and continue to touch upon almost every aspect of our lives. Innovations in data science offer opportunities to support and improve our regulatory decisions across the medical product lifecycle.
However, significant challenges exist particularly relating to data access and linkage, uncertainty around data quality, integration of heterogenous data sources across care systems, and implementation of modern tools upon underlying legacy technology stacks. For example, barriers exist to routinely linking primary and secondary care data, and reliably identifying medical devices which limits our ability to consistently generate robust evidence in certain contexts.
These challenges are neither new nor unique to MHRA but impact upon the entire health data ecosystem and require a cohesive, collaborative, and unified approach to develop the capabilities required to achieve data-driven innovation, improve evidence generation, and support sustainable healthcare and clinical research.
Both in the UK and globally, the data landscape is complex, multi-modal and continuously growing. Conventional Real-World Data (RWD), acquired in the context of healthcare delivery and outside of the randomised controlled trials (RCT), such as electronic health records (EHR) and registry data are now being complemented by ‘omics’ modalities such as genomics, transcriptomics, proteomics and metabolomics, as well as biomedical images, and near-continuous monitoring from wearables and Internet of things devices. We also recognise the critical importance of quality-of-life measurements including patient-reported outcomes to support the ambition of a more personalised regulatory framework.
While the promise of a cradle-to-grave patient record should be achievable given the UK health system, siloing and fragmentation across the health data ecosystem are a persistent and significant obstacle. Addressing these issues, improving data quality and fitness-for-purpose, and integrating evidence from across the four nations of the UK, will be critical to effective and equitable solutions to the most pressing public health needs including precision medicine, long-term conditions, and rare diseases.
This Data Strategy capitalises upon our new One Agency structure and defines our approach to harnessing the potential of data and digital technologies across the medical product lifecycle. Realising our ambitions, will require us to extend and diversify the data we draw upon to inform our decisions, optimise and integrate our own data assets, and embrace novel analytical and methodological approaches. The strategy recognises the vital public service we provide to patients and other stakeholders, including academia, industry, and the third sector, and considers how delivery of this service will be optimised by effective, collaborative, and proactive approaches to data and technology.
3. Lay Summary
The vision of our Strategy is to enable an agile, responsive, and forward-looking approach to ensure we continue to regulate proactively, enable innovation, and safeguard public health. To achieve this, our Data Strategy will identify the opportunities and challenges for data and technology both within our organisation and from the broader ecosystem.
Our Data Strategy is contextualised by several recent reports including Cumberlege (2020), Goldacre (2022), McLean (2023), and Whitehead (2024), in addition to the Life Science Vision (2021), Department of Health Social Care (DHSC) ‘Data saves lives: reshaping health and social care with data’ policy paper, and our own Corporate Plan 2023 – 2026.
Our Science Strategy identifies Data Science as one of five key themes. This Data Strategy consolidates and builds upon the scientific deliverables placed under that theme. Support for Centres of Excellence in Regulatory Science is a critical deliverable within this Data Strategy, and the launch of our regulatory science and innovation network funding call with Innovate UK and Office of Life Sciences (OLS) exemplifies our commitment to driving innovation in this space.
An overarching goal of this Strategy is to ensure we develop the infrastructure, architecture, and expertise to deliver timely access to Real-World Data (RWD) which can in turn result in actionable Real-World Evidence (RWE), and leverage this to support a broad base of regulatory data science including methodology development. This should ensure the evidence generated is sufficiently rigorous to support scientifically- robust regulatory decisions across the product life cycle in the context of uncertainty and ambiguity.
Key to delivering our ambitions will be the development of our people, skills, tools, and technologies to ensure that we can access, integrate, and interrogate data for maximal impact. Capability building is therefore a core element of our Strategy.
Finally, our Data Strategy will drive forward our ambitions to capitalise on artificial intelligence and advanced analytical methodologies to both streamline our business activities and generate actionable insights which support our regulatory mission.
As the regulator of medicines, medical devices, and blood products, decision-making is at the heart of our organisation. This document sets out our plans to improve the ways in which we use data to make these decisions. In particular, this strategy will support us to draw upon the wide range of data sources from across the United Kingdom and beyond and help us to enable earlier access to innovative products through proactive approaches to monitoring post-marketing safety. Another key objective is to improve our operational performance by harnessing the potential of data to enhance the timeliness, transparency, and predictability of our decision-making. Patient safety and public health are central to this plan and we will ensure that progress is communicated to all of our stakeholders as we move to delivering this strategy.
4. Digital and Technology
Our core approach to digital and technology underpins, enables, and catalyses the deliverables within our Data Strategy. The approach is centred upon the application of innovative technologies across the organisation, eradication of legacy applications, and embedding a robust and sustainable approach to cybersecurity.
We recognise the importance of novel technologies as a catalyst and driver for change and creating new opportunities to improve our operational performance. Integrating automation and AI tools should improve the timeliness and predictability of our services.
We will seek wherever possible to democratise our solutions across the organisation for example by establishing a self-service-based infrastructure and procuring low- and no-code solutions to enable greater productivity. Effectively delivering this will require us to modernise our core technology stack for example by migration from bespoke to standardised platforms and embracing cloud-based infrastructure aiming to improve our resilience and sustainability.
Cybersecurity is a critical component of our strategy and, recognising the significant threats we and comparable organisations face, will be based on a ‘zero-trust’ paradigm. We will establish a Cyber Security Operations Centre and deliver an application portfolio which is ‘secure-by-design’ - underpinned by automated solutions, global gold standards, and cyber-AI.
Partnership working, both nationally and internationally, is a prerequisite for the effective delivery of data, digital, and technological use-cases across the organisation. We will therefore work proactively with the technology industry, with partners and stakeholders from across HM Government, and with our global regulatory peers, to deduplicate wherever possible and ensure our approach is aligned to international best practice.
5. Strategic Themes
- Support data-driven innovation, early access, and interdisciplinary data science to underpin our regulatory framework
- Enable effective, timely, and proportionate regulatory decision-making through Real-World Evidence
- Develop, extend, and integrate our capabilities in data and digital technologies
- Establish, embed, and expand synergistic partnerships across the data ecosystem
- Safely and responsibly harness the potential of artificial intelligence and advanced analytics throughout the product lifecycle
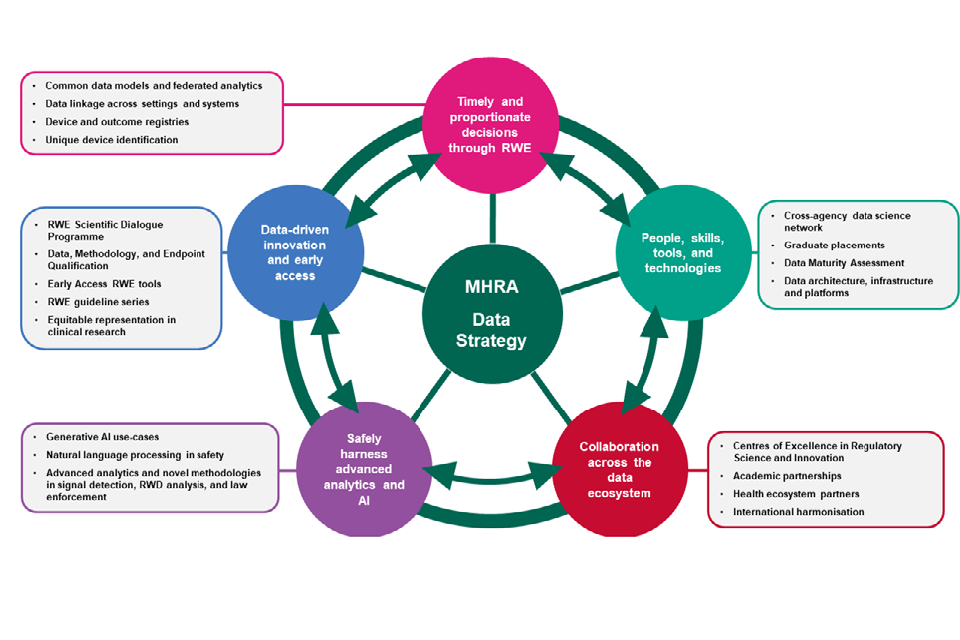
Data Strategy 2024 - 2027 - Plan on a page
6. Theme 1: Support data-driven innovation, early access, and interdisciplinary data science to underpin our regulatory framework
The Pro-innovation Regulations of Technologies review (2023) articulated the critical role of the MHRA within the broader life science ecosystem. Harnessing the potential of data, digital tools, and evidence generation is key to enabling innovation at multiple points in the medical product lifecycle, from initial discovery and development through to post-market surveillance and lifecycle management.
The UK’s strengths are exemplified by our rich health data landscape, extensive capabilities in genome sequencing and interpretation, research-intensive higher education institutions, and collaborative approach to inter-disciplinary science.
Randomised clinical trials (RCT), though the gold standard for demonstration of efficacy, often do not yield representative information about the effectiveness and safety of products across all potentially exposed patients under real-world conditions. We increasingly recognise the synergy and complementarity of Real-World Evidence (RWE), derived from analysis of Real-World Data (RWD) with randomised studies. These types of approaches can streamline and enhance product development, reduce ambiguity, and potentially enable earlier access by facilitating a more proactive approach to post-authorisation surveillance.
Box 1: Challenges in evidence generation for our stakeholders
- Characterising benefit/risk profiles whilst also achieving timely market access in the context of unmet medical need
- Harmonising studies for regulatory requirements and health technology appraisal across multiple territories
- Minimising study burdens on participants, investigators, and sites
- Situations where randomisation is unethical or unfeasible, including certain rare diseases or conditions which result in irreversible morbidity and/or mortality
- Ensuring equity of access to participation in clinical research
- Generalisability and external validity of clinical trials
- Ensuring endpoints robustly predict clinically-meaningful measurements of how patients feel, function, and survive
- Characterisation of safety, in particular long-latency and rare adverse events
- Stratifying patients according to prediction of efficacy and toxicity, including precision medicine and pharmacogenomics
- Delivering proactive vigilance and risk management in the real-world setting
This Strategy aims to identify opportunities to consolidate and harmonise our approach to evidence generation and encourage the uptake of novel methodologies where appropriate, with the aim of promoting efficient and patient-centric approaches to product development. By optimally deploying these methodologies and tools across well-considered hypotheses, investigators should be able to mitigate biases and deliver relevant, actionable evidence for the evaluation of benefits and risks across the spectrum of patients who use the product.
Box 2: Opportunities for RWD throughout the product lifecycle
- Defining disease epidemiology including incidence and prevalence e.g. for orphan designation
- Understanding natural history and disease heterogeneity
- Characterising treatment patterns and standard of care
- Identification and validation of surrogate endpoints
- Pragmatic trials
- External control arms
- Identification and recruitment of potential clinical trial participants
- Informing clinical trial design
- Development and validation of predictive models including training of AI/ML
- Pharmacogenomic and precision medicine approaches
- Product utilisation studies
- Signal detection, contextualisation, and validation
- Post-authorisation safety and effectiveness studies
- Evaluating the impact of risk minimisation measures
We recognise that innovators require clarity from the regulator to enable them to develop and apply novel approaches to evidence generation.
Early dialogue and engagement are being embraced by other global regulators, for example through the US FDA’s Advancing RWE Program. Through the deliverables underpinning Theme 1, we will support innovators in their evidence generation strategies by establishing a Scientific Dialogue Programme to provide support on protocol development for real world studies and subsequently introduce a Data, Methodology, and Endpoints Qualification process. This process should place the patient perspective at the heart of the development process and ensure that studies capture meaningful measurements of how patients feel and function.
Our ambition here is to deliver system-wide global thought leadership and enable innovators to embark on novel evidence generation strategies with a clear view of how these methodologies may support regulatory decision-making underpinned by trustworthy and compliant data.
To achieve this, we will pro-actively engage with our stakeholders to ensure a shared vision for evidence generation across the product life cycle, and to work with ecosystem partners, such as the Health Technology Appraisal bodies, NHS and Devolved Nations.
To deliver Theme 1, we will:
-
Continue our series of guidance documents on RWD/RWE and engage with stakeholders and global regulators to improve international harmonisation.
-
Develop a Scientific Dialogue Programme for RWD/RWE to improve clarity around regulatory expectations for innovative approaches to evidence generation.
-
Understand and describe patterns of underrepresentation in clinical research, thereby defining a harmonised view of inclusivity to improve generalisability and equity of access.
-
Pilot a Data, Methodology, and Endpoints Qualification process to support innovative evidence generation strategies across the product lifecycle.
-
Identify opportunities and deliver clear routes for real-world data to support early access to innovative medicines and devices whilst upholding safety through proactive surveillance in market.
7. Theme 2: Enable effective, timely, and proportionate regulatory decision-making through Real-World Evidence
The Independent Medicines and Medical Devices Safety Review (2020) provides a strong and clear frame of reference for this Data Strategy. The deliverables are fundamental to our ambitions to be an integral component of a healthcare system which learns and continuously improves, thus ensuring the public trusts our decisions and the patient voice is central.
Fulfilling our role as sovereign regulator requires us to make timely and scientifically-robust regulatory decisions which carefully balance the potential benefits and risks of medical products in the context of uncertainty and ambiguity. We must also detect signals of adverse events rapidly and accurately, then contextualise and validate these signals to determine the need for regulatory action. These decisions are reliant on the application of specialist expertise and judgement, underpinned by a comprehensive review of the available scientific evidence.
Evidence generation depends on high-quality, relevant data to enable an unbiased decision on whether the product is safe and effective. Where regulatory interventions are made, we need to be able to monitor the consequences and effectiveness of such actions in real-world clinical practice.
The data ecosystem which can support this is complex, distributed, and heterogeneous. Vast quantities of data, across many modalities, are potentially available – but must be linked, evaluated, and interrogated, to generate trustworthy and actionable evidence.
To fully harness this potential, we need to consider both the data architecture within our organisation and the broader ecosystem, evaluating how we utilise our own data assets, identifying our data needs and proactively address these requirements. In doing so, we recognise the critical importance of data governance to uphold trust in the robustness of our processes and systems, ensuring confidence in decisions we make.
Box 3: Requirements for RWD to support regulatory decision-making in benefit risk-evaluation
-
Timely access with predictable timelines and governance requirements
-
Adequately characterised data quality enabling assessment of fitness-for-purpose
-
Harmonised standards and ontologies permitting interoperability
-
Data which is representative of the UK population
-
Capture of data over a sufficient time course to address the scientific and regulatory questions of interest
-
Linkage and integration of data generated in different care settings and systems
-
Adequate capture of medical product exposure, clinically meaningful outcomes, and relevant covariates including potential confounders
UK health data is an unparalleled potential resource in terms of its scale, diversity, depth, longitudinality, and longevity – however, addressing key challenges around quality, linkage, and interoperability will be key to capitalising on this potential.
Considerations for medicines and medical devices are likely to be distinct and therefore require specific and strategic approaches. For example, traceability of medical devices and linkage to long-term outcomes is a persistent challenge which should be addressed by unique device identification and integration of this information in a structured way into longitudinal records. Adequately understanding safety signals for medical devices also requires additional contextual information about the user, operator, and procedure.
Access to secondary care prescribing is limited in some areas and this presents an obstacle to understanding the impact and consequences of regulatory action for specific issues.
Other leading global regulators have established initiatives to access RWD for regulatory decision-making and in some cases to generate a broader resource to support public health and wider evidence generation activities. A key element of using such data to generate informative and reliable evidence is consistently and thoroughly evaluating the data’s relevance, quality, and fitness-for-purpose.
Establishment of a federated clinical data network within the UK would support evidence generation for regulatory decision making at scale and at pace but should be built in such a way to leverage ongoing initiatives and deliver outputs relevant across health system partners.
Box 4: Challenges in the UK Health Data Landscape
-
Siloing and fragmentation of data across systems, healthcare settings and regions, with many subnational and disease area specific data sources
-
Large number of disparate initiatives delivering distinctive offerings using inconsistent approaches
-
Regional variation leading to limited generalisability and perpetuation of health inequalities
-
Distinctive approaches across the four nations limiting UK-level analysis
-
Lack of consistent data standards and format limiting interoperability
-
Variability in data platforms and infrastructure creating challenges for end-users
-
Variable data quality and limited transparency around fitness-for-purpose of datasets
-
Limited opportunities to link data and integrate multiple data modalities
-
Complex and varying approaches to data governance leading to long-lead times for access and a lack of predictability
-
Specific problems with certain classes of products such as traceability of medical devices and certain care settings such as prescribing in secondary care and the private sector
MHRA’s Clinical Practice Research Datalink is a critical component of the UK health data ecosystem
The Clinical Practice Research Datalink (CPRD) is our RWD research service. CPRD makes available anonymised patient data from a UK-wide network of GP practices for research benefiting public health. Access to data is controlled via a robust data governance framework and secure research environments.
CPRD currently encompasses 65 million patients from all four nations of the UK, including 19 million currently registered patients. Primary care data are linked to a range of other health related data to provide a longitudinal, representative UK population health dataset, with 25% of patients having at least 20 years of follow-up time.
CPRD data have been critical for the monitoring of medical product safety by the MHRA for over 35 years. A key strength of the CPRD databases is that they have been specifically created with research and regulatory requirements in mind. This means that CPRD has a documented data quality strategy, rigorous data quality checks, timestamping of data with no overwriting, metadata, versioning, archiving, and value-added data variables and algorithms.
CPRD is increasingly used in clinical trial recruitment and in support of primary care led decentralised trials. The Data Analytics Recruitment Tool (DART) is a delivery platform to support patient recruitment for this innovative work.
An independently validated Observational Medical Outcomes Partnership (OMOP) Common Data Model (CDM) version of the larger primary care databases is also available along with the Observational Health Data Science and Informatics (OHDSI) Atlas tool for federated analyses. CPRD has also undertaken mapping and conversion of its primary care data into the Sentinel CDM.
CPRD has developed both high and medium-fidelity synthetic datasets that can be used for a variety of purposes including training and validation of machine learning algorithms. CPRD has also been supporting the MHRA’s regulatory science ambitions by validating applications of high-fidelity synthetic data for sample size boosting and as external control arms.
In line with the Goldacre Review recommendations, CPRD aims to move to a predominantly trusted research environment (TRE) based model of data access. This is a major change to CPRD’s model of data access that requires careful consideration of archiving, provisioning of storage and compute, interoperability, linkages, and affordability.
While CPRD data have been invaluable for both the MHRA and the wider research community, the primary care data alone provide only a partial picture of the patient care pathway. This makes linkage to secondary care and other health-related datasets critical. CPRD currently is linked to secondary care, death registration and cancer registry data, but the frequency and timeliness of linked data updates need to be increased.
Expanding the programme of linkage will vastly increase the public health utility and impact of CPRD data, but this will only be feasible in partnership with NHS England and equivalent bodies in the devolved nations. Additionally, from a safety surveillance perspective, there are some key data gaps that could be addressed via new linkages e.g. secondary care prescribing.
To deliver Theme 2, we will:
-
Evaluate the role of common data models and federated analytics to generate RWE within the UK data ecosystem for both medicines and medical devices to address regulatory questions in a timely and scientifically robust manner.
-
Deliver the necessary secondary legislation and associated guidance around medical device identification to support traceability across the medical device lifecycle.
-
Map, evaluate, and strategically engage with major device and outcome registries, identifying opportunities to enhance our safety activities by leveraging RWD from across the UK.
-
Scope opportunities to expand and improve linkage between care settings and data sources to generate actionable regulatory evidence
8. Theme 3: Develop, extend, and integrate our capabilities in data and digital technologies
Our Data Strategy provides an opportunity to identify and address the challenges we face in ensuring we have the requisite capabilities to realise the full potential of data and digital technologies. A broad range of capabilities will be required to deliver our ambitions for data science and evidence generation. We will develop our people and skill mix to ensure we have the specialist expertise to address requirements across data architecture, software engineering, analytics, data science, and machine learning. Key to achieving this will be embedding a culture of collaboration, inter-connectedness, and shared best practices.
Box 5: Core priorities for technological capabilities
- Cloud-based infrastructure
- Eradication of legacy applications and technical debt
- Cybersecurity
- Information governance
- Process automation
- Innovative tools including AI
- Environmental sustainability
HM Government’s Digital, Data and Technology approach and the Open Data Institute Data Skills Framework will support us to develop a resilient, capable, and multidisciplinary data workforce. Drawing upon the considerable external expertise in the broader ecosystem will also support our ambitions and ensure our strategic direction is appropriate and consistent. Like many comparable organisations we are faced with legacy systems, technical debt, and data islands which may limit our scope for achieving operational efficiency and constrain innovation. We are proactively addressing this through investment in legacy eradication and architecture development.
Establishing a robust organisation-wide data model, underpinned by our RegulatoryConnect system, should deliver a secure and trustworthy ‘single point of truth’, minimise duplication, and prevent siloing. Development of a rich suite of tools and technologies is an additional critical capability which will be required, recognising that this will require us to refresh and realign our underlying systems. The development of a modern, flexible and modular architecture will underpin these capabilities and be supported by secure cloud-based solutions. This architecture will facilitate the operationalisation of systems, such as our new vigilance platform SafetyConnect, which will consolidate and extend our capability to deliver proactive and agile safety decisions.
Secure integration of our systems with appropriate third-party solutions will provide additional opportunities to improve our responsiveness. Underpinning this, an organisation-wide data governance framework will be essential in ensuring the appropriate utilisation of our data assets. Taking the steps required to drive theme 3, will benefit patients and stakeholders by ensuring that our people and our digital tools are able to deliver in a timely, predictable, and transparent manner.
To deliver Theme 3, we will:
-
Establish a Cross-Agency Data Science network to share expertise, encourage collaboration and foster innovation.
-
Strengthen our involvement with the Graduate Programme, proactively identifying opportunities for digital and data focussed placements.
-
Conduct a Data Maturity Assessment programme to evaluate our internal data assets from across the organisation.
-
Implement an Agency-wide data architecture based on the principle of ‘collect once, use many times’ to enable us to gain a comprehensive and end-to-end view of any specific medical product.
-
Operationalise data management environments to productionise our data using best practices for data stewardship.
9. Theme 4: Establish, embed, and expand synergistic partnerships across the data ecosystem
The UK has a diverse and flourishing life science ecosystem including academia, industry, the third sector, and public institutions. Our goal is to engage effectively and constructively with partners and stakeholders, aligned on a shared purpose of population health and wellbeing.
The Goldacre review affirmed the vital importance of partnership working, both within the UK and globally. We will further develop an outward-facing and proactive approach to engagement, seeking wherever possible to streamline, de-duplicate, and align our ambitions through a harmonised national and international approach.
We will establish synergistic partnerships and collaborations needed to achieve the goals set out within this strategy. Partnering with academic institutions should support our ambitions for a pipeline of data talent and enable us to collaborate on methodological research which underpins key regulatory priorities.
Opportunities also exist for using the agency’s data knowledge to support international collaboration especially in vigilance. Building on the knowledge and work done to date through SafetyConnect, there are further opportunities to support safety signalling in low- and middle-income countries.
Centres of Excellence in Regulatory Science and Innovation
Delivering this ambitious programme of regulatory science will require extensive collaboration across the broader ecosystem. To enable this, we will work with our network of Centres of Excellence in Regulatory Science and Innovation to deliver tangible progress in data science for regulatory needs.
Over recent years, regulators worldwide have recognised that access to new data sources and associated analytical methodologies offer unparalleled opportunities to manage uncertainty through better evidence generation. Examples of such programmes include the European DARWIN and FDA Sentinel initiatives.
The network should provide MHRA with decision-ready evidence and methodology development to contextualise and validate signals of adverse events, reduce ambiguity in benefit-risk evaluation, enable timely and proportionate regulatory decisions, and facilitate monitoring of the consequences and impact of regulatory interventions such as risk minimisation measures. Collectively this effort should enable innovative product development and early market access through proactive approaches to safety and surveillance.
To deliver Theme 4, we will:
-
Leverage our Centres of Excellence in Regulatory Science and Innovation to deliver tangible progress in data science for regulatory needs.
-
Support international harmonisation of terminology, nomenclature, and data standards by working collaboratively with partners such as ICH and IMDRF.
-
Establish academic partnerships which will develop and apply novel analytical methodologies to improve the benefit-risk evaluation of medical products.
-
Collaborate with partners across the UK to ensure collection and integration of decision-ready regulatory medical product data.
10. Theme 5: Safely and responsibly harness the potential of artificial intelligence and advanced analytics throughout the product lifecycle
Artificial intelligence and machine learning (AI/ML), alongside advanced analytical techniques such as modelling and simulation, are revolutionising our ability to make data-driven decisions across multiple sectors, creating opportunities for scientific discovery, and promising system-wide improvements in efficiency, productivity, and safety. These tools can sift vast quantities of heterogeneous data, discover unexpected patterns and associations, and integrate diverse data modalities which would previously have required distinct analytical approaches.
While there are considerable opportunities to apply and refine the more established AI/ML approaches, such as supervised and unsupervised learning, novel technologies, such Generative AI and Large Language Models (LLMs), are also subject to substantial current interest and the Central Data and Digital Office has recently published a Generative AI Framework for HM Government.
AI/ML approaches offer us tantalising possibilities to extract actionable insights from the wealth of data produced across the health ecosystem. They may also enable us to streamline our processes, improve our operational performance, and innovate across our digital estate.
Nevertheless, these promising tools require a thoughtful and considered approach to their application, particularly in a regulatory and evidence generation context. Quality and representativeness of the underlying data used to train and evaluate AI/ML models is critical, and this will be a key area of focus.
Box 6: Key issues in AI/ML for medical product development and regulation
- Data – quality, representativeness, and fitness for purpose
- Ethical development and application
- Intended purpose and context-of-use
- Model training and validation best practices
- Bias/variance trade-off: overfitting and underfitting
- Performance evaluation
- Reproducibility
- Uncertainty quantification
- Generalisability and subgroup performance
- Interpretability and explainabilility
- Distribution drift and continuous learning
- Human/AI interface
- Regulatory compliance
- Cybersecurity and resilience
- Governance and oversight
We will be mindful of the importance of fairness, consistency and trust, all of which underpin the application of novel technologies. Following Dame Margaret Whitehead’s review of equity in medical devices, we will ensure that clear frameworks are in place to support the validation of AI/ML performance across the patient groups which will be affected by them.
It is critical with all analytical methodologies, but particularly so for AI/ML, that the uncertainty and reproducibility is considered and quantified. We will consider how these tools can be best integrated with human expertise and judgement, ensuring that there is clear accountability where such tools are utilised in product development and across multiple regulatory touchpoints.
To deliver Theme 5, we will:
- Evaluate the use of natural language processing to improve our pharmacovigilance systems and operations.
- Establish a cross-functional task force to explore the operational potential of Generative AI and LLMs to augment our processes.
- Investigate the role of advanced analytical methods, including causal inference and AI/ML, for the analysis of RWD and generation of RWE to reduce ambiguity in benefit-risk evaluation.
- Evaluate the potential of novel methodologies analytical approaches to adverse event signal detection for both medicines and medical devices.
- Investigate the potential of advanced analytical approaches in support of the prevention, detection and investigation of threats to the UK public from medicines crime.
11. Abbreviations
AI/ML | Artificial Intelligence / Machine Learning |
---|---|
CDM | Common Data Model |
CERSI | Centre of Excellence in Regulatory Science and Innovation |
CPRD | Clinical Research Practice Datalink |
DARWIN | E.U. Data Analysis and Real-World Interrogation Network |
DHSC | Department of Health and Social Care |
EHR | Electronic Health Record |
FDA | U.S. Food & Drug Administration |
GenAI | Generative Artificial Intelligence |
HTA | Health Technology Appraisal |
LLM | Large Language Model |
MHRA | Medicines and Healthcare products Regulatory Agency |
NLP | Natural Language Processing |
OHDSI | Observational Health Data Sciences and Informatics |
OLS | Office for Life Sciences |
OMOP | Observational Medical Outcomes Partnership |
RWD | Real-World Data |
RWE | Real-World Evidence |
TRE | Trusted Research Environment |
UKRI | United Kingdom Research and Innovation |