AI Barometer Part 3 - Recruitment and workforce management
Published 17 December 2021
1. Key messages
High impact context: For individuals, data-driven technologies in this sector can influence access to major opportunities, involve their sensitive personal data, and determine the nature and quality of their everyday work. For businesses, these tools can impact the performance of their workforce, the quality and diversity of their talent pipeline, the efficiency of their processes, and their ability to comply with their legal obligations.
Potential to address or perpetuate human biases: Real world applications in this sector have already demonstrated the potential for unfair outcomes for some groups. Conversely, data-driven technology can be used to increase the auditability, transparency and fairness of decision-making in these contexts - which our panel identified as one of the most promising, yet hard to achieve opportunities, with limited evidence this is a mature feature of current market offerings.
Disproportionate workplace monitoring: Our panel expressed considerable concern about the risks presented by algorithmic tools that monitor employees or automate aspects of performance management across both physical and digital environments. Such tools may help improve efficiency (e.g. in allocating tasks) or workplace safety (e.g. identifying worker proximity to hazards or for social distancing), but they also raise issues of accuracy, privacy, consent and fairness. While some of these challenges may be technical, many relate to the proportionality and appropriateness of how employers choose to collect and utilise data to achieve their goals.
Uneven distribution of benefits and risks: While the benefits of these technologies are accrued according to context (e.g. by recruiters, employers, managers, employees etc), the potential harms are not evenly distributed, with applicants and employees tending to primarily bear the direct risks. Employers typically bear legal risks that flow from these, as well as missing out on benefits when these risks are realised, such as access to a broad range of talent.
Unclear governance is the greatest barrier to responsible innovation: Developers and employers have to navigate a particularly complex governance landscape with respect to the use of data-driven technology in these contexts, which invokes data protection, equality, employment, and human rights laws, much of which is dependent on case law for correct interpretation. Many regulators have limited resource to address data-driven technology use across all sectors, and recruitment and employment contexts have historically appeared to be of lower priority.[footnote 1]
Governance barriers create costs: Our panellists told us that the absence of a specific governance framework for these contexts increases costs for developers and employers in seeking to comply with diffuse legal considerations, and results in an uneven playing field for SMEs who experience proportionally higher compliance costs. These factors indicate that there may be a role for enabling governance measures in this sector, such as context-specific guidance and better product assurance that helps employers navigate the market.
Spotlight: CDEI and REC recruitment guidance
CDEI is working with the Recruitment and Employment Confederation (REC) to develop practical guidance on the responsible adoption of data-driven tools in recruitment. CDEI’s Review into Algorithmic Bias highlighted that data-driven tools in recruitment present opportunities to standardise certain aspects of hiring that can be subject to human bias and lack of transparency. However, there are also a number of risks involved in the use of data-driven tools, such as algorithmic bias and accessibility issues. The guidance seeks to support organisations considering using data-driven tools in recruitment through mitigating risks and promoting fairness, transparency, privacy, and trust.
2. State of Play
Data-driven technology use is prevalent and growing across the recruitment and employment lifecycles, with commercially available tools offering to automate and simplify existing processes from candidate sifting to task allocation and productivity monitoring, promising greater efficiency, scalability, and consistency. 98% of Fortune 500 companies use some kind of data-driven systems to assist with recruitment, and some companies are using them to reduce the time-to-hire from weeks to days. Appetite for the use of data-driven technologies has grown significantly in the context of COVID-19, particularly given mass adoption of remote working arrangements for desk-based jobs, recruitment shifting online, and changing demand in labour markets. Beyond the pandemic,these technologies could drive more fundamental, long term shifts to working patterns.
A thriving ecosystem of firms and in-house development has emerged in recent years to exploit the availability of vast quantities of personal data for use in these areas. In recruitment, firms offer automated recruitment services that deploy a range of technologies across the numerous stages of the recruitment pipeline:
-
For job hunters, recruitment platforms can offer better job search, automated CV matching, and automation of aspects of the application process, such as form-filling.
-
At the sourcing stage, algorithmic systems are able to automate job description creation, enable targeted identification and advertising to applicants, and chatbots can automate aspects of a recruiter’s interactions with candidates by collecting their information.
-
When sifting applications, data-driven tools can screen for appropriate qualifications, automatically implement methods that can reduce discrimination (e.g. name-blinding), provide ‘gamified’ assessment platforms to assess candidate skills, and rank or score applicants based on their submissions.
-
At interview, some software uses voice and image recognition combined with inferential biometric technology, claiming to be able to detect desirable candidate qualities and behaviours through posture, tone, and scoring candidate answers. It can also provide more basic functionality such as automated interviews where the panel need not be present (‘asynchronous video interviews’) and automated transcription.
-
At the selection stage, some tools offer automated ‘background checks’ that scan publicly available web content for information on the applicant, and provide recommendations on what kind of an offer a candidate is likely to accept.
The types of data-driven technologies in the workplace is very diverse, but tends to be focused around a few key use cases:
-
Management automation tools can increase the speed and efficiency of task allocation, such as warehouse picking, or allocating gig economy workers to deliveries.
-
Monitoring tools are increasingly prevalent, to detect employee activity (e.g. keystroke logging), presence (e.g. ‘at-desk’ detection), ensure safety (e.g. location tracking via image recognition or wearables), as well as more advanced applications that claim measurement of employee productivity (e.g. based on their use of email and other software), engagement (e.g. based on data like sick leave taken), or wellbeing (e.g. based on inferential biometrics/’emotion detection’).
-
In some instances, most notably on app-based gig economy platforms, the two use cases above are often combined, with workers allocated jobs, scored, and incentivised through monitoring of their performance.
-
Beyond the direct management of employees, some software also offers organisation-level predictive analytics, for example, understanding levels of worker attrition/’churn’, or more controversially, predicting the risk of employees unionising.
Across both contexts, data-driven technology can also support evaluation, for example scoring how inclusive or diverse the outcome of a business process like recruitment is, or monitoring decision-making processes for fairness and consistency - although these use cases appear less prominent in the market.
While not yet mature, the market in this sector includes many commercially available products often bundled as broader product suites, as well as significant instances of in-house development, most notably in tech-heavy companies such as large online retailers or prominent app-based startups.
Spotlight: illustrative market offerings
There are a large number of vendors that offer data-driven solutions for recruitment across the sourcing, screening, interviewing, selection and evaluation stages of the pipeline. Examples include: Textio’s “augmented language” service, that analyses job descriptions for exclusionary language; Loxo, Fetcher and Paradox using non-traditional communication channels to access candidates who may not appear on job boards; Mya and Wade and Wendy, and many others, using conversational chatbots to screen candidates for high-volume roles; Myinterview and XOR offering video interviewing software to assess candidates; and, Herefish and Talkpush providing automated onboarding processes for successful candidates.
Vendors also offer worker monitoring solutions through a wide variety of surveillance tools including: software monitoring, remote control takeover, keystroke logging, screen monitoring, internet monitoring, call tapping, location tracking, webcam surveillance, audio recording, email monitoring, IM monitoring, mobile device access, user action alerts, and time tracking. Some companies, for example FlexiSPY and SPYERA, provide offerings across almost all of these use cases. Others offer based on more specific use cases, for example Sneek offers webcam monitoring only. Whilst there are a wide range of workplace monitoring solutions available, as a market it appears less developed than that of recruitment software. Linked to this, it seems that more companies develop their own monitoring solutions in house than develop their own recruitment tools, perhaps adding to the relative immaturity of the workplace monitoring marketplace.
Spotlight: technology governance in the workplace
Employers using data-driven technology with respect to their workforce and recruitment have to navigate a patchwork of legislation obligations that extend beyond data governance, as highlighted in a recent TUC/AI Law Hub report. Some of the most notable include:
-
The right to equal treatment and work without discrimination (Equality Act 2010), with implications for technologies that may exhibit algorithmic bias.
-
Requirements under health and safety legislation, that apply in particular to technologies aimed at monitoring or improving safety outcomes in the workplace.
-
The right to privacy under the Human Rights Act 1998 (HRA), particularly in the context of monitoring employees when working from home.
-
Obligations under the Employment Rights Act 1996 around acting fairly when dismissing employees of over two years’ continuous service, with implications for technologies that measure or manage employee performance or productivity.
3. Opportunities
-
Innovation in this area promises numerous potential benefits to employers, employees and job applicants. For employers and recruiters, these tools can generate new kinds of data about their employees and job applicants, which can be used to automate processes, improve management practices, monitor employee performance, and bring consistency and standardisation to business processes. For employees, they can provide easier access to job opportunities, provide personalised professional development support, and potentially reduce bias in recruitment and the workplace.
-
Opportunities identified as being of the greatest perceived benefit but the hardest to achieve would primarily benefit workers (compared to employers or recruiters). The most significant of these is the potential for data-driven technology to support the evaluation and audit of fair outcomes from recruitment and management processes, and so help reduce the impact of human biases on such decisions. Recruitment-based opportunities were generally perceived by our panel to be easier to achieve, reflecting that recruitment processes are often sequential, modular, and typically follow common patterns.
-
The challenges associated with such opportunities are both conceptual and technical; for example, training a CV scoring system to ‘read’ and value different forms of qualifications and experience of people from diverse backgrounds is likely to require both appropriately labelled data, and appropriate methodologies for labelling such skills and experience in a way that truly reflects applicants’ capabilities. Similarly, use cases that seek to infer employees’ wellbeing or improve staff retention involve quantifying complex and subjective states that may not generalise well across workforces - for example, using sick leave data to understand staff engagement may fail to appropriately account for the impact of chronic illness or disability.
-
Our panel identified particular challenges in using data-driven technologies for performance management-related use cases, because of the difficulties inherent to measuring performance and productivity quantitatively in many work contexts.
-
Achieving opportunities gets harder when technologies aren’t integrated well into business processes, with tools often being deployed in ‘pre-AI workflows’. For example, in recruitment, technologies such as CV matching and candidate scoring are somewhat constrained by the design and description of jobs themselves, which often follow established templates. Approaching job design more flexibly around the core skills needed may enable a wider range of people to do a role in a number of different ways, allowing employers to engage with a wider profile of candidates that these technologies can help identify. Accordingly, the difficulty of attaining some of these benefits may decrease not only with advances in technology, but also as they are dovetailed with existing processes in more sophisticated ways.
Spotlight: APPG report on AI at work
In November 2021, the All-Party Parliamentary Group on the Future of Work published a report on the role, opportunities and concerns around the use of data-driven technologies in the workplace, with a focus on how governance could be used to stimulate “human-centred AI and the creation of better work for all”.
Opportunities quadrant
This quadrant is based on a panel survey rating the major opportunities in this sector over the next three years. This diagram is not exhaustive and reflects a review of existing policy literature, workshop discussion, further socialising and additional research and analysis. See our methodology for further detail.
4. Risks
-
Our panel noted that the risks of data-driven technology in this sector are not borne equally among its different actors; while there can be significant legal risks for developers and employers, most direct risks are borne by applicants and employees. These parties generally wield the least influence in the design and deployment of the systems in question, meaning design decisions are less informed by those directly experiencing many of the risks. The most significant risks are the capacity for algorithmic systems to perpetuate and entrench human bias and resultant discrimination in recruitment and employment decision-making, and the impacts associated with increased workplace monitoring. These major risk themes are considered in more detail below.
-
The most significant risks are the capacity for algorithmic systems to perpetuate and entrench human bias and resultant discrimination in recruitment and employment decision-making, and the impacts associated with increased workplace monitoring. These major risk themes are considered in more detail below.
-
Panellists noted several factors that exacerbate these risks, such as the relative lack of transparency around the performance of tools (often on the basis of commercial confidentiality) and the comparative infancy of scientific evidence for the validity of algorithmic measures of productivity or employee engagement.
-
While there are technical factors that make machine learning systems prone to exhibiting bias present in training data, many of the more significant risks in this sector relate to developer and employer choices around system design, appropriate boundaries around use cases (e.g. to prevent ‘mission creep’), and the decisions around how to collect, use and share data.
-
In part, this is due to challenges in giving effect to data protection legislation. In particular, consent models for data collection and processing experience significant failure in this sector due to asymmetric power relationships between employers and both employees and applicants. As noted in the government’s data reform consultation, consent may be over-relied upon as a purpose.
-
Another contributing factor is the maturity of digital and data skills needed to appropriately procure and use some algorithmic tools, particularly those providing recommendations or predictive capabilities. For example, unlike some contexts such as medical care, where professionals are accustomed to dealing with uncertainty and potential inaccuracy in the use of diagnostic tools, it is less clear that recruiters and employers typically enjoy similar skillsets, meaning the tools may be used inappropriately.
-
Cyberattacks can present additional risks due to the use of data-driven technologies, but these were considered to be lower than in some other sectors by our panel. In part this was because use cases in this sector tend not to be in safety or time-critical processes, or do not necessarily involve the additional collection and storage of sensitive personal data significantly beyond the considerable volumes normally collected for recruitment and management purposes. However, the impacts of data breaches and changes in algorithmic decision-making functionality could still result in considerable harms for individuals and organisations.
Spotlight: Consent in workplace contexts
The potential for consent mechanisms to fail in employment contexts has been recently highlighted in international contexts. In March 2021, Amazon drivers working in the US were asked to sign a ‘biometric consent’ form or otherwise lose their jobs. The updated contracts asked drivers to agree to the use of facial recognition and collection of biometric and location data through cameras installed in delivery vans.
In the UK, consent as defined under UK GDPR must be freely given, specific, informed and unambiguous, with specific requirements around prominence and clarity of consent requests, around the right to withdraw consent easily and at any time, and around contracts that are conditional on consent. GDPR Recital 43 emphasises that where contracts are dependent on consent but not necessary for performance, consent will not have been freely given, and that obtaining freely given consent will be more difficult in the context of a relationship between parties where there is a ‘clear imbalance’ between the data subject and the controller.
Risks quadrant
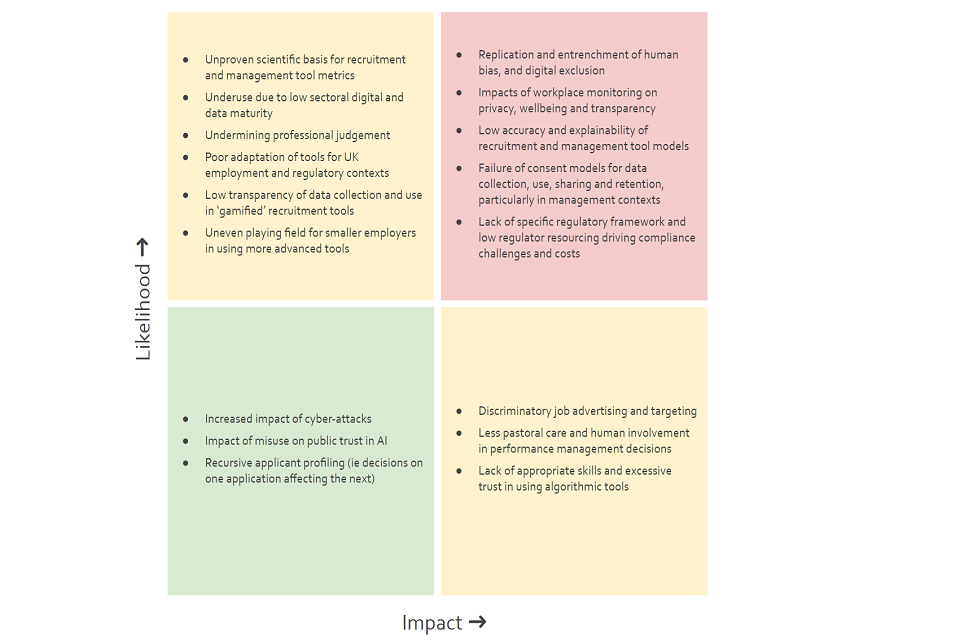
This quadrant is based on a panel survey rating the major risks in this sector over the next three years. This diagram is not exhaustive and reflects a review of existing policy literature, workshop discussion, further socialising and additional research and analysis. See our methodology for further detail.
Major risk theme - impacts of workplace monitoring
-
While profiling and targeting using data-driven technology is commonplace in online contexts, the prevalence and growth of digital monitoring of workers in workplace contexts is relatively new. In physical spaces, image recognition and wearables can be used to detect workers’ presence, location, proximity to hazards, to each other, and estimated levels of attention or drowsiness. In digital contexts, software can measure workers’ interaction with productivity suites (e.g. email) and hardware (e.g. vehicle telematics), as well as logging keystrokes or browsing history. The data from this monitoring can be combined into various metrics that some vendors claim can be used as accurate measures of productivity, engagement, or other types of performance. In some international contexts, such measures have been used to automatically dismiss staff, although this practice would be unlikely to comply with UK law.
-
Workplace monitoring will often engage a variety of legal and ethical implications, including data protection, privacy, and equality law, carrying the risk of affecting individuals’ rights, and with unclear impacts on worker wellbeing. The impact of these technologies is unevenly distributed across the workforce. While monitoring of digital working environments is growing, it appears particularly common in lower-paid or manual labour, such as gig economy or social work.
-
The ‘gamification’ of certain work types appears to have grown in recent years and over the course of the pandemic, whereby performance monitoring technology directly feeds back into workers’ environments and task allocations. For example, some warehouse contexts incentivise workers to beat picking times and use that data to optimise future efficiency targets (usually upwards), and similar mechanisms are common in gig economy app platforms, where worker ratings or more desirable work assignments are distributed based on performance.
-
The scientific basis for many metrics derived from monitoring is unproven; while this arguably may be easier to demonstrate with some types of labour (e.g. deliveries), establishing valid measurements of concepts such as productivity and engagement becomes increasingly challenging with more complex work. There are no widely accepted standards for quantifying such measures apparent among vendors, who typically do not disclose their methodologies or software auditing for commercial reasons.
-
In governance terms, data collection and use for monitoring purposes usually takes place either under consent or another legitimate purpose under UK GDPR Article 6. Processing must be lawful, fair and transparent, accurate, limited to a clear purpose, and in line with data minimisation principles.
-
Providing meaningful consent in this context is highly challenging, meaning employees and applicants may have little meaningful control over whether they are subject to such tools or how their data is used. Employees must effectively choose another employer in order to opt out, or applicants forgo access to prominent job ‘marketplaces’. Unlike online service contexts, where people can take measures to anonymise themselves if they wish to opt out, such measures are either impractical or illegal in employment contexts (e.g. providing false personal data). This imbalance is exacerbated where work is less secure.
-
Similarly, employers may in practice find it challenging to meet Article 5 requirements with more intrusive monitoring systems, or where their approach lacks a clear definition or purpose for data collection and processing - which third party vendors cannot directly provide.
Spotlight: Employee perceptions of monitoring technologies
With the mass shift of workforce to remote working, as a result of the COVID-19 pandemic, there has been a surge in demand for employee surveillance software. In April 2020, global demand for these technologies rose by 87%. Emerging research suggests that employees and workers are unaware of the kinds of new monitoring technology which employers could introduce and find the technology uncomfortable. When polled by Prospect, 80% of workers said they would be uncomfortable with camera monitoring, 74% would be uncomfortable with electronic wearable tracking, and 66% would be uncomfortable with keystroke monitoring of their remote work. Prospect’s research also found that 48% of workers thought that introducing these technologies would damage their relationship with their manager (this rose to 62% with younger workers). Research by the Chartered Institute of Personnel and Development (CIPD) and the Trade Union Congress (TUC) paints a similar picture, with the CIPD’s work finding 73% of employees feel the introduction of these technologies would damage trust between workers and employers, and the TUC finding similarly for 65% of employees. Other research by the CIPD suggests that, amongst other things, lack of trust in developing technologies leads to lower employee engagement with technologies. This implies that the lack of trust employees have in these technologies could act as a barrier to realising their potential benefits.
5. Barriers
Barriers to responsible innovation are the issues that prevent us from maximising the benefits of data-driven technologies, and which prevent harms from being appropriately mitigated.
Lack of guidance and low regulator capacity
-
There is a broad and complex legal patchwork governing data-driven technology in recruitment and management contexts, including data protection, equality, employment, and human rights laws, and their respective bodies of case law. Our panellists told us that the absence of a specific regulatory framework for these contexts increases costs for developers and employers in seeking to comply with diffuse legal considerations, and results in an uneven playing field for SMEs who experience proportionally higher compliance costs.
-
Employers may be disincentivised from investing in these technologies out of concern around exposure to potentially unlawful practices, and find it hard to navigate an opaque market (detailed below).
-
Many regulators have limited resource to address data-driven technology use across all sectors, and recruitment and employment contexts appear to be of lower priority.
-
These factors indicate that there may be a role for enabling and standardising governance measures in this sector, such as context-specific guidance and better product assurance that helps employers navigate the market.
Recruitment software developer said:
When we don’t have clear governance, we need to be detectives in case law to ensure our products are compliant
Spotlight: Regulators and AI
The impacts of data-driven technology are increasingly high priorities for many regulators. The Equality and Human Rights Commission recently consulted on a draft 2022 strategy which proposes prioritising AI and emerging digital technologies, as well as fairness in workplace contexts. The ICO frequently publishes Commissioner Opinions on digital technologies and recently consulted on an AI and data protection risk toolkit designed to assist risk practitioners identify and mitigate data protection risks that AI system use can involve.
Lack of market transparency
- The level of market maturity and the integration of these technologies into existing workflows is relatively nascent, meaning employers may struggle to identify products that meet their needs and obligations. The efficacy of data-driven recruitment and management systems and their ability to address issues such as bias is often opaque, and many vendors cite commercial confidentiality as a reason for not submitting their products to external scrutiny. Limited publicly-available or comparable data on the effectiveness of these systems can make it hard to measure whether they are more effective than existing systems or compare them against each other.
Unclear scientific validity and impact
- The scientific validity of many of the metrics claimed to be measured by various platforms (e.g. productivity, or employee engagement) remains unproven. The use of inferential biometrics to detect indicators of desirable interview candidate behaviours is particularly contested.[footnote 2]
-
The impact of different types and combinations of monitoring and gamification technologies on employee wellbeing is poorly understood. In many instances, developers have few direct connections to the subjects of the technology (i.e. applicants and employees), meaning the engagement and input of data subjects is low.
-
The cumulative impact of technology over the various stages of sequential processes like a typical recruitment pipeline, and onward into employment is poorly understood. For example, smaller barriers to access created by technology for a particular person or group (e.g. a neurodivergent person) at the advertising, sifting, and interview stages may amount to a more significant barrier when considered holistically. As the market matures and systems become increasingly interoperable across stages, their cumulative impact will become even less clear.
Low quality and biased historical data
- The data used by employers to train algorithmic systems (e.g. for recruitment sifting) is likely to reflect historic biases in recruitment practices (such as preferring male candidates), and can be difficult to compensate for as machine learning systems may find proxy variables when blinded from seeing protected characteristics. The accuracy and explainability of a given AI system will vary across deployment contexts (i.e. when deployed for different employers) based on variations in the nature and quality of their datasets.
Spotlight: Inferential biometrics in recruitment
A number of recruitment providers have offered facial analysis to infer emotions or personality traits in prospective employees, but concerns surrounding the accuracy and scientific basis of inferential biometrics has led to some providers such as HireVue removing such features from their offerings. Following a complaint by the nonprofit Electronic Privacy Information Center filed with the Federal Trade Commission in 2019, HireVue announced that an algorithmic audit of its software showed it did not exhibit bias, but nevertheless removed the feature as ‘visual analysis has far less correlation to job performance than other elements of [their] algorithmic assessment’. HireVue’s software continues to analyse characteristics of candidates on the basis of other metrics such as their language and speech patterns.
Sensitivity to public trust
- As detailed in this chapter’s Spotlights, there have been numerous well-publicised instances of AI tools leading to discriminatory recruitment and employment outcomes that are likely to have negatively affected public trust in these technologies. Several employee surveys appear to show similar concern at the erosion of privacy and trust at work that can arise from poorly implemented data-driven technologies.[footnote 3] This can be aggravated by the failure of consent models in many employer-employee contexts, as power imbalances in such relationships often mean any consent given for the use of data-driven technologies is unlikely to be meaningful, and there is little directly-applicable guidance to steer appropriate data collection and use.
Spotlight: different approaches to introducing workplace technology
In 2019, Microsoft released a Productivity Score that allows managers to track employee’s activities for data such as contribution in group chats, number of emails sent, and level of collaboration in shared documents. The new features received negative media coverage, with some privacy researchers suggesting this effectively represented the introduction of remote workplace surveillance. Microsoft responded that the Productivity Score was not a work monitoring tool and is instead about ‘discovering new ways of working’, and emphasised that data was provided at the user level to enable insights for IT administrators about technology and infrastructure usage.
In December 2020, the Royal Mail Group (RMG) and the Communication Workers Union (CWU) signed an agreement that included the rollout of new technology and automation, following a two year dispute. The resulting framework introduced a number of key principles about how new technologies will be deployed, and included agreement on the rollout of “scan in/scan out” technology, and the trialling of a Resource Scheduler, as well as protections for employees in the context of new technologies.
-
Other employment sector bodies such as CIPD and ACAS have published research and guidance, but this usually does not constitute advice on interpreting regulatory provisions. ↩
-
For example, Meredith Whittaker (AI Now Institute), in The Washington Post (2019), ‘A face-scanning algorithm increasingly decides whether you deserve the job’, also AI Now Institute (2019), 2019 Report ↩
-
For example, Prospect (2020), ‘Workers are not prepared for the future of working from home’; Skillcast (2020), ‘Remote-working Compliance YouGov Survey’; BBC coverage of similar worker surveys ↩