AI Barometer Part 1 - Summary of findings
Published 17 December 2021
1. Foreword
After a year of intense disruption and change, data-driven technologies promise solutions to some of the most significant challenges facing the UK - both old and new. Understanding how we can best leverage technologies to address major shifts in labour markets and the ways that we work, deliver education in trying circumstances, or decarbonise our transport infrastructure will be crucial to building back better.
The AI Barometer is the CDEI’s contribution to understanding this complex picture; an analysis of the most pressing opportunities and risks associated with AI and data use - and crucially, the barriers that prevent us from realising the potential of data-driven technologies.
Building on last year’s report, this edition looks across three key UK sectors which have been severely affected by the pandemic, and where data-driven technologies may hold some of the keys to our economic and social recovery: recruitment and workforce management, transport and logistics, and education. The Barometer uses a novel approach rooted in innovative comparative research tools and community engagement with over 80 expert panellists to cut through the complex and often overwhelming volume of commentary and debate about the potential of data-driven technologies in these sectors. Alongside it, CDEI is also publishing the results of a business innovation survey, looking at key trends around the adoption of data-driven technologies among UK businesses.
These technologies offer a wealth of potential benefits, but our findings indicate that many of the most promising opportunities are also amongst the hardest to achieve, such as system-level applications to reduce carbon emissions, creating safer working environments, scaling up the provision of high quality education, and improving fairness in recruitment and management contexts. This report identifies the major, cross-sectoral barriers that hold us back from doing so.
Foremost among these barriers is ensuring data-driven technologies are trustworthy, and consequently trusted by users, organisations, markets and the public. The impacts of low trust in data-driven technologies have been highly visible in recent years, tangibly inhibiting innovation and ultimately depriving people of the benefits of these technologies. The right starting point for building trust is ensuring data-driven technology is implemented and used in a way that is trustworthy.
Where organisations lack the confidence to develop and deploy technologies, both they and society miss opportunities. Industry finds it particularly challenging to navigate how the patchwork of law and regulation applies in the myriad contexts data-driven technologies are used, with clear demand for better context-specific guidance and advice, to encourage innovation and avoid technology users and developers needing to be ‘detectives in case law’.
The advent of the government’s National Data Strategy, data reform consultation, and AI Strategy provides a crucial opportunity to identify and implement solutions to these barriers. The CDEI is already addressing many of these challenges, working with partners to facilitate responsible data sharing, better public sector AI and data use, and laying the foundations for a strong AI assurance ecosystem. These findings will inform the Centre’s future work programme and vision of what responsible innovation needs to flourish in the UK.
Edwina Dunn
Interim Chair of the Centre for Data Ethics and Innovation
2. Summary of findings
This report highlights the most promising opportunities presented by data-driven technologies across three sectors, describes the risks associated with their use, and crucially - the barriers that hold us back from innovating responsibly.
Responsible innovation is an approach to implementing data-driven technology that ensures it is built and used in a way that is ethical, trustworthy and ultimately effective. In practice, it often means finding ways of giving life to high level ethical principles in the way organisations develop, deploy and evaluate the impact of AI and data use on the ground.
2.1 Most promising opportunities
Not all opportunities presented by data-driven technology are equal. They vary both in the scale of potential benefits, and in how hard they are to realise. Our analysis focuses on identifying the class of highest benefit, hardest to achieve opportunities. These are the most unlikely to be realised without intervention, due to a variety of technical, market, governance or trust-based challenges.
For each sector, we looked at the issues currently facing the sector, and the ‘prize to be won’ if data-driven technologies can be successfully leveraged to address them:
-
Recruitment and Workforce Management: Market unpredictability caused by the pandemic has created different labour demands and cost pressures on employers. Data-driven technologies promise improved talent pipelines, better performance management, and more standardised business processes. Both for those seeking employment and for those in work, these tools can provide easier access to job opportunities, personalised professional development support, and in the longer term could potentially reduce discrimination.
-
Transport and Logistics: Data-driven technologies have significant potential to improve energy efficiency, drive down emissions, and yield better environmental outcomes, in what is traditionally a carbon-intensive sector. Mobility also sits at the heart of an efficient economy, and strong transport infrastructure also helps drive improved social mobility. Smart infrastructure can also play a key role in addressing logistics challenges - especially at borders, where it can smooth the transition of goods at a time when the UK is building new trade relationships.
-
Education: Educators face increasing pressure to provide high quality education across complex student needs and have been managing additional challenges in adapting to the impacts of the pandemic. Data-driven technologies can help reduce the administrative burden on teachers, improve consistency of teaching and marking, and in the longer term, promise to enable scalable personalised learning. In aggregate, our panel highlighted these factors can contribute to improved social mobility as high quality education becomes easier to access.
Our analysis highlights patterns in use cases that can affect how difficult it is to achieve their full potential. Easier to achieve use cases often involve:
-
Low-risk automation where decisions have minor/no direct impact on individuals.
-
Augmentative recommendations (where data-driven assessments provide an additional data point for human decision-makers to consider)
-
Automation of discrete tasks within a broader system (e.g. candidate matching)
-
Integration into systems where both input and output is data-controlled (e.g. controlling traffic lights based on real-time traffic data)
Harder to achieve use cases tend to involve:
-
Integration into complex systems and environments (e.g. long-term planning)
-
Integration with interpersonal human decision-making (e.g. people management, understanding student needs)
-
Qualitative judgements of human performance (e.g. productivity, marking humanities subjects)
-
Using data-driven approaches to combat bias and discrimination
High-benefit opportunities by sector
Recruitment and Workforce Management | Transport and Logistics | Education | |
Harder to achieve | * Lower bias and improved fairness in recruitment and management * Better retention and pastoral support through wellbeing insights * Better talent pipelines (e.g. via increased diversity) * Improved employee safety |
* Reduced environmental impact and better fuel optimisation * Better system planning e.g. through predicting future mobility needs or predictive maintenance of infrastructure * Better access to mobility e.g. via ‘mobility as a service’ * Improved safety due to AI-driven robotics e.g. on roads and in moving freight |
* More scalable teaching provision via tailored learning pathways * Reduced administrative burdens such as marking workload * Metacognition - understanding what learning approaches, environments, and interventions work best |
---|---|---|---|
Easier to achieve | * Improved recruitment efficiency * Job search automation and better matching for applicants * Efficient planning and resource allocation * Greater remote working flexibility |
* Better mobility such as reduced journey times due to data-driven navigation, better managed traffic, and more accessible transport options * Automated and efficient transport network management (e.g. traffic flow management) * Better logistical efficiency (e.g. management of distribution/ delivery fleets and tasks) * Improved reliability and transparency of supply chains |
* Improved education system management (e.g. improved and novel insights into the performance of the sector) * Predicting risk (e.g. of dropping-out or failing assessments) enabling teachers and parents to make timely interventions |
2.2 Most significant risks
Much technology use introduces new risks or exacerbates existing ones, and it will be difficult to make the most of data-driven technology without mitigating the most serious of these, many of which appear commonly across all sectors examined. These risks drive barriers to responsible innovation by disincentivising use or damaging public trust, and are often borne unevenly by different groups.
Prominent across all sectors examined was the concern that problematic or controversial use of AI or data could harm public and organisational trust in AI itself. In particular, this is due to the pervasive risk of algorithmic bias and consequent discrimination, the potential for consent mechanisms failing to give data subjects meaningful control over their data, the explainability of AI-supported decision making, and how the technologies affect people’s privacy and autonomy. Underlying these drivers were concerns that regulatory systems may not yet be fully adapted to mitigating the risks of data-driven technology, and may struggle to do so in a timely way due to the scale of this challenge.
Common significant risks across sectors
While the use of AI and data presents unique challenges for each sector, our analysis found that a large number of risks were common to every sector examined. This table reflects how risks that are common across sectors were perceived in relative terms by our expert panels, from higher to lower risk, as reflected in the risk quadrants within each sector chapter.
2.3 Barriers to responsible innovation
Our expert panels (consisting of representatives from across the public sector, industry, academia and civil society) identified the following common issues as some of the greatest barriers facing responsible innovation in data-driven technology.
- Trust
Low trust in AI and data use inhibits innovation. Organisations may avoid adoption of new technologies, or choose to delay or cancel innovative work due to fears about public backlash. A lack of trust among the general public can mean fewer users or less data available at the system level to generate truly transformative applications that address societal challenges, as well as damaging trust in the organisations and institutions that deploy such technology. Recent years have provided many examples of how problematic applications of AI and data use can damage public trust significantly, ultimately depriving people of the benefits of technology. The sectors examined in this edition showed particular sensitivity to public trust, not just in terms of data subjects (e.g. pupils in the education sector) but also customers and users of such technologies trusting technology (e.g. teachers).
- Unclear governance
Data-driven technology often engages a broad range of legal and regulatory mechanisms, including data protection, equalities and human rights laws, and context specific codes of practice and case law. Navigating this complex landscape is a significant challenge for developers and users, as well as data subjects. Implemented well, appropriate regulation and broader governance measures can bring clarity, and give confidence to innovators. However, across all sectors examined, panellists reported challenges in understanding how high-level principles apply in specific sectoral or application contexts, with the consequence that organisations are less willing to make full use of data and data-driven technologies due to the risk of non-compliance or doing harm, or the costs of guaranteeing compliance. In some instances, regulator resourcing can act as a bottleneck to developing guidance and providing clarity in a timely way, particularly around novel use cases.
- Misaligned market incentives and poor market information
Our panels highlighted that in several markets, vendor offerings are often not aligned with customer needs. For example, in education, applications of AI and data-driven technology are often targeted at ‘low hanging fruit’ use cases, and are focused on replacing rather than augmenting educator functions in ways that can be problematic or inappropriate to implement. In recruitment and workforce management contexts, most use cases are aimed at increasing the efficiency of such processes for employers, with many of the most promising opportunities around fairer processes represented in few market offerings. Panellists also reported significant difficulties for customers in navigating data-driven technology markets due to poor levels of accessible market information and comparable standards, particularly in recruitment, workforce management and education contexts. A lack of customer confidence in data-driven tools disincentivises custom and investment, making it harder for responsible innovators to succeed in the market, and a lack of customer knowledge increases the risk of inappropriate technology use (see next barrier).
- Unclear scientific validity of novel data insights
Many of the applications examined across the three sectors use new forms of data (e.g. ‘second generation’ biometrics) and algorithmically generated metrics or inferences (for example measuring a data subject’s performance, engagement or alertness). The scientific basis for these may not be well established, leading to inaccurate performance from the system in question (for example, assessing an employee to be performing badly). These technologies are nevertheless being deployed in real-world contexts (often by customers that may not fully understand the impact of a tool on data subjects), acting as a major risk driver for damaging trust in data-driven technologies and the organisations using them.
- Low digital and data maturity
In addition to typical digital maturity issues such as access to modern technologies and good quality data, data-driven tools are often not fully integrated into how organisations or services work, which can cause technology use to have unintended negative consequences. For example, data-driven employee performance insights may not be reliably and fairly integrated into management processes. Underlying low levels of maturity are factors such as continuing high demand for data skills across the economy, meaning that many organisations struggle to find the talent they need, and gaps in our data-sharing infrastructure, from interoperable standards to governance frameworks that enable organisations to share ethically and with confidence.
Spotlight: Lifting the barriers to responsible innovation
The barriers identified below point to possible solutions, such as better governance measures, clearer context-specific guidance and a potentially important role for better data-driven product assurance. The CDEI will continue to play an important role in helping address these challenges. Over the next year, we will be prioritising three themes in our work, to help foster responsible innovation:
-
Data sharing: We will facilitate responsible data sharing across the economy, including piloting new forms of data stewardship and governance.
-
Public sector innovation: We will support and facilitate the responsible development, deployment and use of AI and data across the public sector, with a focus on the most high impact use-cases.
-
AI assurance: We will help lay the foundations for the development of a strong AI assurance ecosystem in the UK, helping organisations to have confidence to innovate responsibly with AI and data, by fostering an emerging industry in AI assurance services.
Our work programme has already started to address some of the specific challenges facing data-driven technology in these sectors, working with the Centre for Connected Autonomous Vehicles on the legal framework needed to support self-driving vehicles; with the Recruitment and Employment Confederation on governance guidance for data-driven recruitment tools; and exploring future work in education. More broadly, the government’s National Data and AI Strategies, and ongoing consultation on “Data: A new direction”, provide crucial opportunities to address many of the governance barriers identified. The CDEI will continue to support this important work.
2.4 Scope and Methodology
The scope and approach of our research focused on the following:
-
The impact of AI and data-driven technologies: We looked at the potential impact of technologies involving the use and collection of data, data analytics, machine learning, and other forms of artificial intelligence. We refer to these broadly as ‘data-driven technologies’, and refer to more specific technologies where relevant.
-
A focus on the barriers to responsible innovation: We set out to understand what factors may prevent us from achieving the full benefits of data-driven technology. We used policy and academic literature to identify the opportunities and risks presented by data-driven technology in each sector over the next three years, to frame discussion with our expert panels.
-
Sector selection driven by current UK challenges: We focused on three sectors that had been radically affected by the pandemic, to understand how data-driven technology can help address some of the most pressing economic and social challenges facing the UK today. Using a sectoral approach frames our findings within boundaries that policymakers and regulators are familiar with.
-
An expert, community-driven view: Understanding the ethical impacts of AI and data use is an interdisciplinary endeavour. We convened expert panels made up of different communities within each sector, which ensured our work was informed by a diverse set of expertise and perspectives. Each panel was composed of a balanced set of experts and stakeholders within each sector, typically including representatives from government, regulators and other arms-length bodies, tech industry, sector organisations (e.g. service providers, businesses using data-driven technology), membership bodies, academia, and civil society organisations. Over 80 expert panellists contributed to our surveys and workshops. We used comparative survey tools so our panels could meaningfully assess and rank a large number of technological impacts, and used the results to provoke discussion in a series of expert panel workshops. This report reflects the input of these panels.
-
Balancing interests: In considering potential benefits, our panels took into account the wide range of stakeholders who stand to benefit from data-driven technologies - or bear their risks. For example, in the transport and logistics sector, this included national and local public bodies responsible for transport and environmental outcomes, people and businesses with mobility service needs, mobility service providers, and technology developers and vendors. Our panellists also considered the trade-offs between short and longer term benefits - for example, the convenience of short cab journeys via data-driven platforms vs the longer term environmental impact of such transport use.
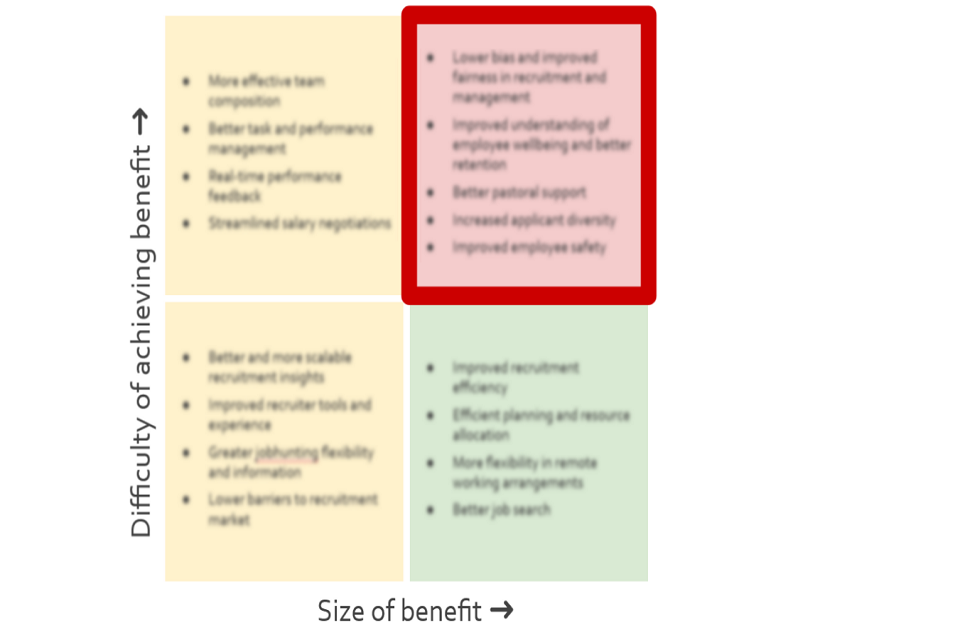
Quadrant analysis: We use quadrants to break down opportunities of data-driven technology based on how much benefit they present vs how hard they will be to realise. Our analysis focuses on high benefit, hard to achieve opportunities, as they will be the hardest to realise without intervention.