Data science in GAD helps clients
GAD uses data science to identify patterns, gain detailed insights and offer better advice to clients.
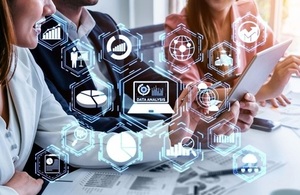
Data science experts at the Government Actuary’s Department (GAD) use a wide suite of technologies to help interrogate data, infer patterns and communicate results. These include using sophisticated ‘machine learning’ algorithms to identify and analyse complex patterns in data. This emphasis on data science is an important and growing part of GAD’s client offer.
Insights and data
GAD uses machine learning approaches to identify and gain insights into patterns and drivers of change within large and multi-faceted data sets. These insights enable us to provide an improved predictive quality to our modelling.
We combine actuarial judgement and advanced technical output with data visualisation tools to create powerful visual representations. This leads to greater understanding and the ability to deliver clear and relevant messages and better advice to our clients.
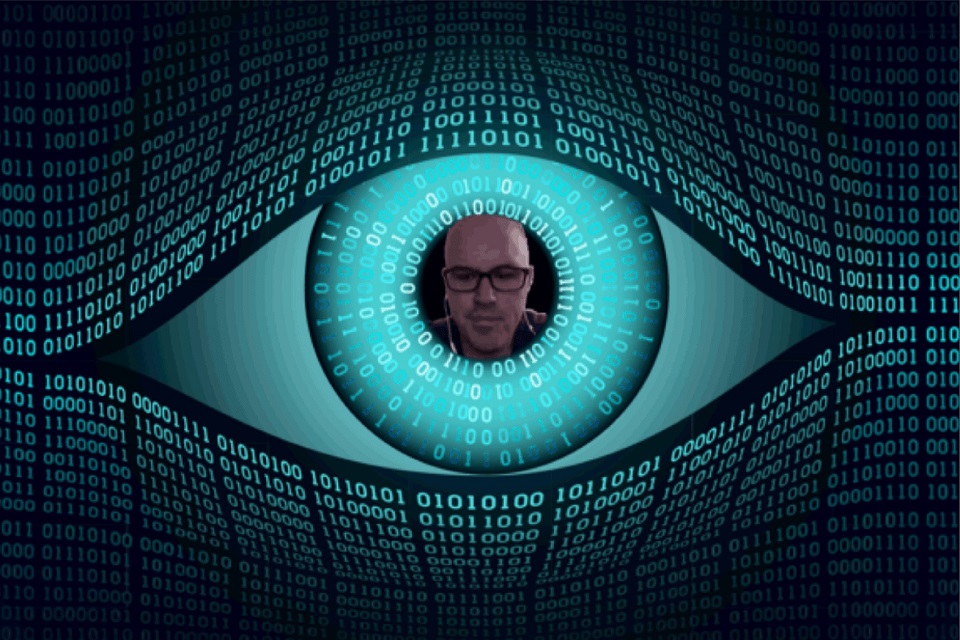
Data science in action
Student loans
Working alongside UK Government Investments (UKGI) on preparing and implementing the sale of the student loans book, GAD used machine learning techniques to analyse earnings paths of student loans borrowers. This approach helped us gain a better understanding of what was driving graduate earnings, and how these changed over time.
We used that information to confirm the robustness of UKGI’s existing model while also identifying areas to prioritise for possible refinement. Demonstrating robust modelling performance to investors increases their confidence in the behaviour of the underlying asset which could ultimately lead to increased proceeds for government from the sale of the student loans book.
NHS medical negligence claims
NHS Resolution helps the NHS to manage claims that arise when things go wrong. GAD advises on the likely costs relating to such claims and provides insights from the data to help understand the risks of future clinical negligence claims. We used machine learning techniques to help identify how relationships between multiple factors in the data drive likelihoods of settlement outcomes of claims. This provided additional assurance on the current modelling methods as well as exploring potential benefits of alternative modelling approaches.