Meet the data quality dimensions
Measurements driving continuous improvement
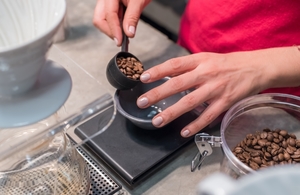
Data is valuable if it is of good quality. Data quality dimensions are the characteristics against which we measure quality.
In this article, we will look at these dimensions, what they track and why each is important.
Data quality dimensions
To ensure that data is trustworthy, it is important to understand the dimensions of data quality. Data quality dimensions will help you assess if your data is good enough to use or if you need to make improvements. A single dimension will not be sufficient to assess the quality of your data. You will need to select the dimensions that will describe it as fit for the purpose it’s intended to be used.
While we recognise that organisations may define different quality dimensions, we recommend these six dimensions, as defined by the Data Management Association UK (DAMA(UK):
1. Accuracy
We have accuracy when data reflects reality. For example, this can refer to correct names, addresses or represent factual and up to date data. A likely place for errors to occur is right at the start, during data collection. Look closely at each data field to see if the values look plausible. The height of a person recorded as 15 cm is clearly wrong.
Real-world information can change over time. This makes accuracy quite challenging to monitor. A change in the personal circumstances of a claimant may affect the housing benefit that the person is entitled to. You should regularly review data that is likely to change over time.
High data accuracy allows you to produce analytics than can be trusted; it also leads to correct reporting and confident decision-making.
2. Completeness
Data is considered complete when all the data required for a particular use is present and available to be used. It’s not about ensuring 100% of your data fields are complete. It’s about determining what data is critical and what is optional.
Consider patient records consisting of personal details and medical history. Missing information on allergies is a serious data quality problem because the consequences can be severe. On the other hand, if there are gaps in email addresses, this may not have an impact on patient care.
Completeness applies not only at the data item level but also at the record level in terms of assessing whether your data set meets your expectations of what’s comprehensive. This measure helps you understand if missing data will have an impact on its use and affect the reliability of insights that you gather from it.
Completeness is not the same as accuracy as a full data set may still have incorrect values. You may have full information about people claiming benefits, but this does not mean that the information is correct.
3. Uniqueness
Uniqueness measures the number of duplicates. Data is unique if it appears only once in a data set. A record can be a duplicate even if it has some fields that are different. For example, two patient records may have different addresses and contact numbers, but if they both refer to the same patient there is duplication. In this instance, it is likely that healthcare providers may miss critical information because it is in the duplicate.
Duplication is a particular risk when combining data sets. You should always check your data for uniqueness. Unique records build trust in the data.
4. Consistency
Consistency is achieved when data values do not conflict with other values within a record or across different data sets. For example, the first characters in a postcode should correspond to the locality of the address. Similarly, date of birth for the same person in two different data sets should be the same.
Consistent data improves the ability to link data from multiple sources. This, in turn, supplements your data set and increases the utility of the data.
5. Timeliness
Timeliness indicates whether the data is available when expected and needed. Timeliness means different things for different uses. In a hospital setting, timeliness is critical in ensuring the most up to date data in a bed allocation system. However, it may be acceptable to use previous quarterly figures from healthcare records to forecast care needs and plan health and social care services.
Data quality may diminish over time. For example, someone might provide the correct address or job title when the data is captured, but if the same individual changes their address or job these data items will become outdated.
Timeliness is important as it adds value to information that is particularly time sensitive. Timely data during the pandemic made health care provisions more responsive and saved lives.
6. Validity
Validity is defined as the extent to which the data conforms to the expected format, type, and range. For example, an email address must have an ‘@’ symbol; postcodes are valid if they appear in the Royal Mail postcode list; month should be between one and twelve.
Having valid data means that it can be used with other sources. It also helps to promote the smooth running of automated data processes.
However, we should not take for granted that valid values are always accurate. For example, in a system storing the colour of people’s eyes, a value of ‘pale ’is invalid. A value of ‘blue’ for a person’s eye colour would be valid but incorrect if it is actually brown.
Using data quality dimensions
Every organisation should have some means of measuring and monitoring data quality. You should identify which data items are critical to your operations and measure them using the appropriate dimensions. This will help identify issues and plan for improvements. Assessing data quality is an on-going process; measure the quality of your data regularly as data can change over time.
These dimensions are widely used and endorsed by industry professionals. In some cases, though, a different set of dimensions may be more appropriate. For example, for the quality of statistical outputs, refer to the quality dimensions defined by the European Statistical System in its Quality Assurance Framework, which are specifically designed for this purpose.
You can learn more about data quality dimensions from the Government Data Quality Framework. For guidance on how to use them effectively, watch out for our forthcoming training course on ‘Data Quality Action Plans’.
The Government Data Quality Hub (DQHub) is developing tools, guidance, and training to help you with your data quality initiatives. Please visit our website for articles, tools and case studies.
We also offer tailored advice and support across government. Contact us by emailing dqhub@ons.gov.uk.